Ph.D. Student Mingjun Sun Untangles the Complexity Puzzle in Asset Pricing
Researcher Uses Machine Learning to Decode Financial Markets
By John Miller
Media InquiriesIn his recent paper, “Complexity vs. Simplicity in Factor Pricing Models,” Mingjun Sun, a third-year doctoral student in Finance at the Tepper School of Business, studies the best way to construct factor pricing models. Sun is interested in financial machine learning, an emerging field that uses machine learning to study financial markets. While machine learning offers powerful tools, applying these methods in asset pricing poses unique challenges.
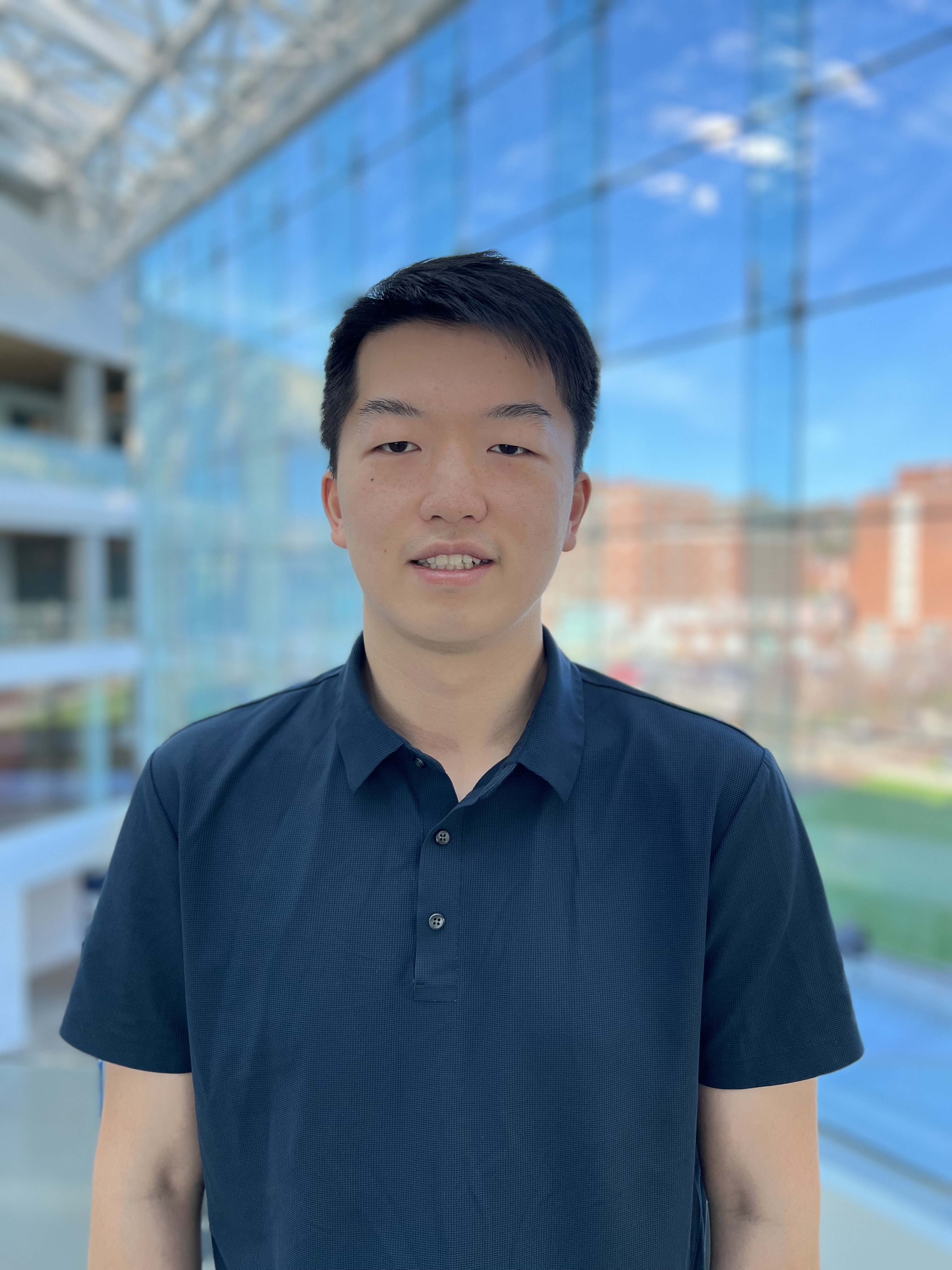
In finance, the stochastic discount factor, or SDF, is a critical tool used to explain asset prices by discounting future earnings based on their uncertainty. Traditional approaches, popularized by Eugene Fama and Kenneth French, construct the SDF using a small number of factors tied to firm characteristics such as size and book-to-market ratio. However, as the amount of available data has exploded in recent years, so have the number of potential characteristics to consider. This has led to debate over whether models should embrace greater complexity by incorporating a large number of factors.
Sun’s paper explores whether a complex SDF, with a large number of factors, or a simpler one, using only a select few dominant principal components, does a better job of explaining the cross-section of returns. His research sheds light on this tradeoff using a blend of empirical analysis and theoretical modeling.
Using U.S. equities data, Sun constructs complex and simple SDF models based on three different sets of factors: 153 factors, 11,936 factors formed through interactions of 153 characteristics, and 10,000 factors generated through random feature transformations of basic characteristics.
Sun finds that while constructing factors using non-linear transformations of firm characteristics improves pricing performance, approximately fifty dominant principal components are sufficient to summarize the cross-section of returns without losing out-of-sample accuracy. In other words, Sun shows that complexity matters, but so does parsimony. A factor pricing model with about fifty dominant principal components of a large number of factors can be just as effective as one with tens of thousands of factors. This finding has important implications for finance: it suggests that researchers and practitioners can achieve a balance between harnessing the richness of financial data and keeping models practical and manageable.
Sun develops a theoretical framework grounded in arbitrage arguments to support his empirical results. He shows that models with too many factors would imply the existence of near-arbitrage opportunities, which are highly profitable strategies rarely observed in real-world markets. His theory reinforces the empirical observation that a few dominant principal components can capture most of the relevant information in asset returns.
Sun’s work also uncovers important practical insights for researchers and practitioners. He finds that estimating factor pricing models over longer historical periods (e.g., 20 years) improves out-of-sample performance. Additionally, he demonstrates that different methods of constructing factors, such as value-weighting versus equal-weighting, can meaningfully impact results.
Sun’s work provides valuable insights into the ongoing debate about complexity and simplicity in asset pricing models. The paper suggests that while incorporating complexity through non-linear transformations of characteristics can enhance a model's predictive power, this complexity can be efficiently captured by a few dominant principal components.
In the future, Sun plans to investigate the applications of large language models to extract signals from unstructured financial data and improve quantitative investment strategies.