Upcoming Workshops
PFEW (Pittsburgh Formal Epistemology Workshop) & Center for Formal Epistemology Talks
For more information about upcoming PFEW events email: Adam Bjorndahl, Krzysztof Mierzewski or Francesca Zaffora Blando.
More PFEW workshops will be posted next semester.
Past Workshops, Conferences and Talks
Friday, April 18, 2025
Damini Kusum (CMU)
Title: Solomonoff Induction and Algorithmic Randomness
Abstract: For sequential prediction tasks, Solomonoff's (1978) celebrated result guarantees the inductive success of the “simplicity” prior by showing that the predictive probabilities induced by any optimal semimeasure converge to the posterior of the true probability almost surely. Hutter and Muchnik (2004) have shown that one can construct a Martin-Löf random sequence from an optimal semimeasure such that this convergence does not hold for the uniform measure. Since the study of algorithmic randomness can be used to characterize “almost-sure” convergence theorems, one can ask whether stronger notions of randomness suffice for Solomonoff convergence. I show that it is possible to establish an upper bound on the strength of randomness required for convergence. Furthermore, while difference randomness may prima facie appear to be the intuitive notion to test for convergence, I discuss the possibility of a negative result.
April 3–5, 2025
The Center for Formal Epistemology at Carnegie Mellon University presents:
Progic 2025: The 12th Workshop on Combining Probability and Logic
Special focus: Theoretical Approaches to Learning and Learnability
Logic and probability provide two distinct frameworks for modeling how rational agents ought to draw inferences and learn from the available data in the face of uncertainty. The aim of this conference is to provide an interdisciplinary forum for bridging these two perspectives and, in particular, to explore ways in which one may combine insights and techniques from probability and logic to provide an analysis of rational learning and the conditions under which successful inductive learning can be achieved.
This workshop is primarily aimed at logicians, philosophers, mathematicians, statisticians, and computer scientists. Topics will include logical and computability-theoretic aspects of probabilistic inference, logics for probabilistic and causal reasoning, inductive logic, statistical models of learning (including Bayesian approaches), and topological approaches to learning and learnability.
Plenary Speakers:
- Dan Roy (Toronto)
- Sean Walsh (UCLA)
- Kun Zhang (CMU)
If you have any questions, please contact Krzysztof Mierzewski, Francesca Zaffora Blando or Kevin Kelly.
Friday, March 28, 2025
Alexander Meehan (University of Wisconsin-Madison)
Title: What conditional probability could (probably) be
Abstract: According to orthodox probability theory, when B has probability zero, the conditional probability of A given B can depend on the partition or sub-sigma-field that B is relativized to. This relativization to sub-sigma-fields, a hallmark of Kolmogorov's theory of conditional expectation, is traditionally seen as appropriate in a treatment of conditioning with continuous variables, and it is what allows the theory to preserve Total Disintegrability, a generalization of the Law of Total Probability to uncountable partitions. In this talk, I will argue that although the relativization of conditional probability to sub-sigma-fields has advantages, it also has an underrecognized cost: it leads to puzzles for the treatment of iterated conditioning. I will discuss these puzzles and some possible implications for the foundations of conditional probability.
This talk is based on joint work with Snow Zhang (UC Berkeley).
Friday, March 21, 2025
Patrick Rooyakkers (University of Bristol)
Title: Dutch Strategies, Accuracy-Dominance, Lockean Beliefs, and their Plannings
Abstract: Suppose we consider an agent with both numerical credences and all-or-nothing beliefs. This agent might also have a plan about how she is going to update her beliefs upon receiving new evidence. What rational requirements on such a plan can be justified from an epistemic value point of view? Plan Almost Lockean Revision is the claim that it is rationally required that one's planned beliefs are exactly one's sufficiently high conditional credences. We start by reviewing arguments available for Plan Lockean Revision in the current literature, ultimately concluding that they are non-optimal. We provide a better argument to the effect that the belief updating rule that is expected to be the best according to one's current credences is exactly Plan Almost Lockean Revision, that is, we prove a Qualitative Greaves-Wallace Theorem. Furthermore, building on the work of (Rothschild, 2021), we investigate the dutchbookability of Lockean betting behavior for all-or-nothing beliefs and their plannings, ultimately proving a qualitative version of the dutch strategy theorem which leads to the development of novel dutch-strategy/accuracy-dominance arguments for Lockean norms on belief/belief-planning pairs.
Friday, March 14, 2025
Leszek Wroński (Jagiellonian University)
Title: Elimination counterexamples — a blow to accuracy monism?
Abstract: We study the situations where an agent's epistemic state, presumably, improves, and yet its inaccuracy increases. We show that the phenomenon is, in a precise sense, frequent, and that it is frequently displayed by all the popular inaccuracy measures found in the literature. Lastly, we investigate how this fact can be used as an argument against accuracy monism.
Friday, February 28, 2025
Igor Douven (French National Centre for Scientific Research)
Title: The Social Learnability of Natural Concepts
Abstract: According to a recent proposal, natural concepts are those represented by the cells of an optimally partitioned similarity space, where optimality is defined in terms of various design criteria, including the criterion that natural concepts should be easily learnable. While computational studies have shown that natural concepts are indeed more easily learned than non-natural ones in contexts of individual learning, the proposal also suggested that natural concepts should facilitate social learning, helping communities converge on shared meanings through interaction. We test this hypothesis using neural agent-based models that combine artificial neural networks with models of opinion dynamics to simulate how communities learn conceptual structures through social interaction. Our simulations demonstrate that natural concepts are learned more easily and reliably than non-natural ones in social contexts, thereby providing further support for the optimal partitioning account.
Friday, February 14, 2025
Shawn Simpson (University of Pittsburgh)
Talk Title: William James’s “The Will to Believe”: A Decision-Theoretic Analysis
Abstract: A fairly common view is that one ought not believe that for which one is lacking sufficient evidence. In William James’s time, a view of this sort – often referred to as evidentialism – was quite popular. James, however, thought evidentialism was too restrictive. There were at least some circumstances, he contended, in which one had a right to believe an evidentially unwarranted hypothesis. In this paper, I present and clarify James’s argument against evidentialism as presented in his 1896 lecture “The Will to Believe” and do so with an eye toward understanding the argument from a decision-theoretic perspective. I argue that we can interpret at least part of James’s essay as a contribution to decision theory, where James is introducing a special class of decision problems, namely the class in which the acts about which an agent must make a decision are acts of believing. Recasting James’s argument this way, we can see how James might actually have made a decision- theoretic argument against Clifford’s evidentialism. Whether such an argument works out in the end, however, hinges on whether one really can “will to believe” anything.
Friday, January 17, 2025
Daniel Herrmann (University of Groningen)
Talk Title: Deference and Decision
Abstract: The debate between evidential decision theory (EDT) and causal decision theory (CDT) has long centered on cases like Newcomb's Problem. In particular, causalists have claimed that EDT's violation of (what I’ll call) "Deference to the Foreseen"—the principle that you should take actions you know a better-informed version of yourself would endorse—seems to count heavily against it. In this talk I’ll present joint work with Gerard Rothfus showing that this dialectic is incomplete. We identify another compelling principle of rational choice that we call "Deference to the Seen," which says that an act's value should only depend on outcomes consistent with your current evidence. Crucially, whether a decision theory satisfies either principle depends on the learning rule with which one pairs it. After formalizing these principles, decision theories, and learning rules within a common formalism we prove that no conservative decision theory—one that agrees with EDT and CDT when they agree—can satisfy both principles with respect to any single learning rule. Specifically, assuming Bayesian updating, EDT satisfies Deference to the Seen but violates Deference to the Foreseen, while CDT exhibits exactly the opposite pattern. We then show how CDT can satisfy Deference to the Seen when paired with a modified learning rule we call "aggressive conditioning”, though necessarily at the cost of violating deference to the foreseen. These results illuminate a tension at the heart of the EDT-CDT debate: any conservative decision theory, paired with any learning rule, must choose between deferring to evidence that rules out possibilities and deferring to hypothetical better-informed perspectives. At the end of the talk, I’ll take a step back, and gesture at how I (in contrast to Gerard) think we should proceed in light of these results. In particular, I’ll sketch an argument that we should treat Deference to the Foreseen how we treat dominance reasoning: only appropriate under specific independence conditions.
Friday, December 6, 2024
Mario Günther (MCMP, CMU)
Talk Title: Legal Proof Should Be Justified Belief of Guilt
Abstract: We argue that legal proof should be tantamount to justified belief of guilt. A defendant should be found guilty just in case it is justified to believe that the defendant is guilty. Our notion of justified belief implies a threshold view on which justified belief requires high credence, but mere statistical evidence does not give rise to justified belief.
Monday, November 25, 2024
Jan-Willem Romeijn (University of Groningen)
Talk Title: "Overfitting in statistics and machine learning”, or: "The complexities of complexity"
Based on joint work with Daniel Herrmann (University of Groningen) and Tom Sterkenburg (LMU Munich)
Abstract: Machine learning (ML) methods seem to defy statistical lore on the risks of overfitting. They often have many more adjustable parameters than are needed for fitting the data perfectly but, after showing predictive loss in the realm of normal overfitting, highly overparameterized ML models show surprisingly good predictive performance. In my talk I will review several attempts by statisticians and computer scientists to explain this so-called "double descent phenomenon" and distill three philosophical lessons that each derive from seeking continuity between statistics and ML. One is that our conception of model capacity needs an update, as it harbors a variety of ideas about complexity. A further lesson is that we have to flip the script on the problem of underdetermination: the use of unidentified statistical models offers predictive advantages, and this invites a fresh look at our empiricist ideals. A final lesson relies on De Finetti's representation theorem and on basic insights into the problem of induction: understanding the success of machine learning requires that we delve into processes of model and data construction.
Thursday, November 22, 2024
Aydin Mohseni (CMU)
Talk Title: Why are the Social Sciences Hard?
Abstract: We present two novel hypotheses for why the human sciences are hard: (1) we are pre-committed to a very specific domain of prediction tasks in the human sciences, which limits a powerful strategy for making scientific progress—changing the prediction task to something more tractable; and (2) due to evolutionary pressures, human baseline performance is relatively high for many of the ‘low-hanging fruit’ prediction tasks concerning human behavior, making progress beyond this baseline challenging. We provide a formal framework for reasoning about the difficulty of disciplines and the impressiveness of their achievements in terms of their success at particular prediction tasks.
This is joint work with Daniel Herrmann.
Thursday, November 7, 2024
Branden Fitelson (Northeastern University)
Talk Title: Probabilities of Conditionals and Conditional Probabilities—Revisited
Abstract: Lewis’ (1976) triviality argument against The Equation (also known as Adams’ thesis) rests on an implausible assumption about the nature of (epistemic) rational requirements. Interestingly, Lewis (1980) later rejected this assumption. In his discussion of the Principal Principle, Lewis makes a weaker and more reasonable assumption about the nature of rational requirements. In this paper, I explain how to apply the insights of Lewis (1980) to repair Lewis (1976). This leads to a more reasonable rendition of the equation—one that is (a) immune to triviality and (b) a better candidate for a (bona fide) rational requirement.
Friday, November 1, 2024
Samuli Reijula (University of Helsinki)
Talk Title: Modeling cognitive diversity in group problem solving
Abstract: According to the diversity-beats-ability theorem, groups of diverse problem solvers can outperform groups of high-ability problem solvers (Hong and Page 2004). This striking claim about the power of cognitive diversity is highly influential both within and outside academia, from democratic theory to management of teams in professional organizations. Our replication and analysis of the models used by Hong and Page suggests, however, that both the binary string model and its one-dimensional variant are inadequate for exploring the trade-off between cognitive diversity and ability. Diversity may sometimes beat ability, but the models fail to provide reliable evidence of if and when it does so. We suggest ways in which these important model templates can be improved.
There’s a paper that the talk is based on, and more information about my research can be found at www.samulireijula.net.
Friday, October 25, 2024
Max Noichl (University of Bamberg and University of Vienna)
Talk Title: Towards Empirical Robustness in Network Epistemology
Abstract: One of the central papers in simulation studies of science argues that less communication can lead to higher reliability. More generally, simulation studies have been used to explore which communication networks enhance collective reliability and speed of convergence. However, this literature has largely concentrated on relatively simple network structures (e.g., cycles, wheels, full graphs), which bear little resemblance to real social networks. Does less communication often lead to higher reliability also in real social networks? In this talk, we provide the first results concerning the empirical robustness of these findings with respect to real social networks.
We develop a novel method to perform this empirical robustness analysis. First, we use citation graphs to depict empirical networks commonly discussed in the literature as examples of lagging discovery—i.e., one concerning the bacterial causes of peptic ulcers and another concerning the prolonged history of the perceptron. Second, we develop a new method to generate a sample of “similar” networks, based on the optimization of generative network models toward the sampled empirical ones. Third, we work out the collective reliability and speed of convergence of these communication networks by running simulations. Finally, we analyze the data about these networks to determine which outcomes can be expected in real networks and which network properties (e.g., degree heterogeneity, clustering, etc.) strongly affect collective reliability and speed of convergence.
This is joint work with Ignacio Quintana and Hein Duijf.
Friday, October 4, 2024
Ahmee Christensen (Department of Mathematics, UC Berkeley)
Title: Logics of Knowability
Christensen Talk Slides [pdf]
Abstract: Knowability has a significant history in the field of epistemic logic dating back to the introduction of Fitch's paradox in 1963. More recently it has received attention from the perspectives of dynamic epistemic logic and epistemic temporal logic. However, existing treatments of knowability either address a knowability-like concept that deviates from an intuitive understanding of knowability in some important way or take knowability to be a complex notion merely definable in the language. In this paper, we take 'it is knowable that' as a primitive modality and offer axiomatizations of two knowability logics—one of knowledge and knowability and one of pure knowability. We prove that these logics are sound and complete with respect to a distinguished subclass of birelational frames. These proofs are hopefully of technical interest, as well; because knowability is, we argue, best understood as a non-normal modality, the completeness proofs require novel model constructions.
Friday, September 20, 2024
Daniel Halpern (EconCS Group, Harvard University)
Title: Aggregating Preferences with Limited Queries
Abstract: Social choice theory studies how to aggregate individual preferences into a collective decision for society. Traditionally, this assumes full access to each individual’s complete set of preferences. However, modern online platforms promoting civic participation, such as pol.is, aim to solve social choice problems that do not fit neatly into this framework. These platforms aggregate complex preferences over a vast space of alternatives, rendering it infeasible to learn any individual's preferences completely. Instead, preferences are elicited by asking each user a simple query about a small subset of their preferences.
In this talk, I will present a simple model for analyzing what is possible in these scenarios, along with a variety of positive and negative results . It covers two recent papers:
Contributions include:
- Positive algorithmic results: Efficient algorithms that produce representative outcomes with limited queries.
- Information-theoretic impossibilities: Fundamental limits on what can be learned, regardless of the number of queries.
- Query-complexity lower bounds: Situations where, even if it is possible in theory to achieve a desired outcome, an exponential number of queries may be required, making it practically infeasible.
No prior knowledge of social choice will be assumed.
The Center for Formal Epistemology at Carnegie Mellon University presents:
Saturday, September 21 and Sunday, September 22, 2024
Time: Saturday from 8:30am to 4:45pm and Sunday from 8:30am to 12pm
Location: Steinberg Auditorium, Baker Hall A53
An interdisciplinary workshop aimed at exploring philosophical, methodological and mathematical aspects of probabilistic reasoning, from Bayesian conceptions of rationality, to chance and its relation to credence, to logical and computational considerations in probabilistic learning.
Speakers:
- Gordon Belot (Michigan)
- Cameron Freer (MIT)
- Kevin Kelly (CMU)
- Krzysztof Mierzewski (CMU)
- Brian Skyrms (UC Irvine)
- Snow Zhang (UC Berkeley)
If you have any questions, please contact Krzysztof Mierzewski, Kevin Kelly, or Lisa Everett.
Friday, September 6, 2024
Siddharth Namachivayam (Philosophy Department, Carnegie Mellon University)
Title: Topological Semantics For Asynchronous Common Knowledge
Abstract: Common knowledge as usually defined has the property that it must arise synchronously, i.e. someone cannot know P is common knowledge without everyone knowing P is common knowledge. Recent work by Gonczarowski and Moses 2024 (G&M 2024) proposes redefining common knowledge so it can arise asynchronously at different times for different agents. Why? We think of common knowledge as guiding coordination. So if we would like to guide coordination asynchronously, we ought to define common knowledge asynchronously. In this talk, we analyze a Byzantine generals-like learning game where agents must coordinate on correctly reporting a proposition is true asynchronously. We argue the worlds where agents can successfully coordinate in some equilibrium of this game using no retractions should be thought of as the worlds where the proposition can become asynchronous common knowledge. In the course of doing so, we develop a purely topological semantics for asynchronous common knowledge which makes no explicit reference to time. Our topological semantics correspond to detemporalizing G&M 2024’s semantics but also naturally admit a notion of asynchronous common belief. We conclude by showing the worlds where agents can successfully coordinate in some equilibrium of our learning game using a fixed budget of retractions coincides with the worlds where the proposition is true and can become asynchronous common belief.
Friday, April 19, 2024
Caspar Oesterheld (CMU, Computer Science)
Title: Can de se choice be ex ante reasonable in games of imperfect recall? A complete analysis
Abstract: In this paper, we study games of imperfect recall, such as the absent-minded driver or Sleeping Beauty. We can study such games from two perspectives. From the ex ante perspective (a.k.a. the planning stage) we can assess entire policies from the perspective of the beginning of the scenario. For example, we can assess which policies are ex ante optimal and which are Dutch books (i.e., lose money with certainty when it would be possible to walk away with a guaranteed non-negative reward). This perspective is conceptually unproblematic. The second is the de se perspective (a.k.a. the action stage), which tries to assess individual choices from any given decision point in the scenario. How this is to be done is much more controversial. Multiple different theories have been proposed, both for how to form beliefs and how to choose based on these beliefs. To resolve such disagreements, multiple authors have shown results about whether particular de se theories satisfy ex ante standards of rational choice. For example, Piccione and Rubinstein (1997) show that the ex ante optimal policy is always “modified multiself consistent”. In the terminology of the present paper (and others in this literature), they show that the ex ante optimal policy is always compatible with choosing according to causal decision theory and forming beliefs according to generalized thirding (a.k.a. the self-indication assumption).
In this paper, we aim to give a complete picture of which of the proposed de se theories match the ex ante standards. Our first main novel result is that the ex ante optimal policy is always compatible with choosing according to evidential decision theory and forming beliefs according to generalized double-halfing (a.k.a. compartmentalized conditionalization and the minimal-reference-class self-sampling assumption). Second, we show that assigning beliefs according to generalized single-halfing (a.k.a. the non-minimal reference class self-sampling assumption) can avoid the Dutch book of Draper and Pust (2008). Nevertheless, we show that there are other Dutch books against agents who form beliefs according to generalized single-halfing, regardless of whether they choose according to causal or evidential decision theory.
Friday, March 29, 2024
Mariangela Zoe Cocchiaro (Jagiellonian University, Kraków)
Title: Fail again. Fail better. But fail how?
Abstract: As the so-called ‘Defeat’ assumption in the epistemology debate suggests, peer disagreement often functions as a sort of litmus paper for detecting the presence of a defective attitude. In this talk, I scrutinize the exact nature of this defective attitude—and of the credal version of ‘Defeat’ stemming from it—when we operate in a fine-grained model of belief. Firstly, I show how the question as to the nature of the defectiveness of the credences in these cases falls within the scope of the epistemology debate. Then, after claiming that the fairly obvious appeal to inaccuracy comes with philosophically heavy commitments, I turn to what credences are taken to be for a principled answer.
2024 Pitt-CMU Graduate Student Philosophy Conference
Saturday, March 16, 2024
Baker Hall A36, Adamson Wing
9am-6pm
In the Spring semester of each academic year, graduate students from three Pittsburgh area departments (Pitt Philosophy, Pitt HPS, and CMU Philosophy) come together to organize a conference to exhibit promising work from graduate students around the world in all areas of contemporary philosophy. The conference also includes a keynote by a prominent philosopher; past keynote speakers include L.A. Paul, Hartry Field, Bas van Fraassen, Michael Strevens, and Stephen Yablo.
This year’s conference is hosted by CMU and will take place on the 16th of March, 2024. The keynote address will be given by Elisabeth Camp.
Friday, March 15, 2024
Jessica Collins (Columbia University)
Title: Imaging is Alpha + Aizerman
Abstract: I give a non-probabilistic account of the imaging revision process. Most familiar in its various probabilistic forms, imaging was introduced by David Lewis (1976) as the form of belief revision appropriate for supposing subjunctively that a hypothesis be true. It has played a central role in the semantics of subjunctive conditionals, in causal decision theory, and, less well known to philosophers, in the computational theory of information retrieval. In the economics literature, non-probabilistic imaging functions have been called “pseudo-rationalizable choice functions”. I show that the imaging functions are precisely those which satisfy both Sen’s Alpha Principle (aka “Chernoff’s Axiom”) and the Aizerman Axiom. This result, a version of which was proved in Aizerman and Malishevksy (1981) allows us to see very clearly the relationship between non-probabilistic imaging and AGM revision: see diagram, AGM revision is Alpha + Beta. Mark Aizerman (1913-1992) was a Soviet cyberneticist at the Institute for Control Sciences, Moscow.
Friday, March 1, 2024
Alexandru Baltag (ILLC, University of Amsterdam)
Title: The Dynamic Logic of Causality: from counterfactual dependence to causal interventions
Abstract: Pearl's causal models have become the standard/dominant approach to representing and reasoning about causality. The setting is based on the static notion of causal graphs, but it also makes an essential use of the dynamic notion of causal interventions. In particular, Halpern and Pearl used this setting to define and investigate various notions of actual causality.
As noted by many, causal interventions have an obvious counterfactual flavour. But... their relationship with the counterfactual conditionals (a la Lewis-Stalnaker) has remained murky. A lot of confusion still surrounds this topic.
The purpose of this talk is threefold:
1. understand interventions as dynamic modalities (rather than conditionals);
2. elucidate the relationship between intervention modalities and counterfactual conditionals;
3. formalize and completely axiomatize a Causal Intervention Calculus (CIC), that is general enough to allow us to capture both interventions and causal conditionals, but also expressive enough to capture the various notions of actual causality proposed in the literature.
Friday, February 9, 2024
Sven Neth (University of Pittsburgh)
Title: Against Coherence
Abstract: Coherence demands that an agent does not make sequences of choices which lead to sure loss. However, Coherence conflicts with the plausible principle that agents are allowed to be uncertain about how they will update. Therefore, we should give up coherence.
Friday, December 8, 2023
Josiah Lopez-Wild (UC Irvine)
Title: A Computable von Neumann-Morgenstern Representation Theorem
Abstract: The von Neumann-Morgenstern Representation Theorem (hereafter “vNM theorem”) is a foundational result in decision theory that links rational preferences to expected utility theory. It states that whenever an agent’s preferences over lotteries satisfy a set of natural axioms, they can be represented as maximizing expected utility with respect to some function. The theorem provides us with a behavioral interpretation of utility by grounding the notion in choice behavior. This talk presents a computable version of the vNM theorem. Using techniques from computable analysis I show that a natural computability requirement on the agent's preferences implies that there is a computable utility function that the agent maximizes in expectation, and that in some cases this computability condition is also necessary. I discuss the philosophical significance of computable representation theorems for decision theory, and finish with a discussion of other representation theorems that I suspect can be effectivized.
Friday, November 17, 2023
Marta Sznajder (Institute Vienna Circle, University of Vienna)
Title: Janina Hosiasson-Lindenbaum: Building a career in inductive logic in the 1920s and 1930s
Abstract: Janina Hosiasson-Lindenbaum (1899-1942) was a Polish philosopher working on inductive reasoning and the interpretation of probability. As a member of the Lvov-Warsaw School, she was an active participant in the logical empiricist movement, broadly construed. Most of her philosophical work concerned the logical aspects of inductive reasoning and the nature of probability. In this talk, I will present her academic career from the historical and philosophical perspective.
In spite of her extensive publication record - spanning over more than thirty articles, conference talks, texts in popular magazines, and book translations - and her wide network of academic contacts, she never held a post at a university and remained a high school teacher between obtaining her PhD in 1926 and the beginning of World War II. I will give an overview of the strategies she used to promote her work and establish herself as an internationally recognized philosopher, as well as the obstacles that she faced - culminating with the failed efforts to obtain refugee scholar funding from the Rockefeller Foundation in 1940.
While Hosiasson-Lindenbaum has been recognised as an early adopter and developer of subjectivism, her philosophical work spans a much broader range. As it turns out, she was engaged in some ways with almost all significant developments in philosophical theories of probability and confirmation of the interwar decades: as a critic and a commentator, and as a highly original philosopher.
Friday, November 10, 2023
Michael Cohen (Tilburg University)
Title: Imperfect Recall from Descartes to Monty Hall
Abstract: The overall aim of this talk is to draw interesting connections between assumptions foundational to Bayesian Epistemology and principles of dynamic epistemic logic. Various authors have observed that both Dutch book and accuracy based arguments for Bayesian conditioning require a partition assumption from the learning experience. Roughly, for such arguments to work, the agent must know there is a set of propositions that partitions the epistemic space, and that the proposition learned in the learning experience comes from that partition. Schoenfield, Bronfman, Gallow and others have connected this partition assumption to epistemic introspective principles ("KK-like" principles, applied to learning), although the exact logical formulation of those principles remains informal. In this talk, I present a general logical framework to analyze the logical properties of (Bayesian) learning experiences, using dynamic epistemic logic. I argue that Perfect-Recall is an important epistemic principle at the heart of these Bayesian matters. In such epistemic logic formulation, Perfect-Recall is not really about memory, but about the agent's general ability to know how they came to know what they know. Following the existing literature, I use Monty Hall style cases to demonstrate the connection between Perfect-Recall and the partition assumption.
Friday, October 27, 2023
Jeff Barrett (University of California, Irvine)
Title: Algorithmic randomness, probabilistic laws, and underdetermination (joint work with Eddy Chen)
Abstract: We consider two ways one might use notions of algorithmic randomness to characterize probabilistic physical laws like those encountered in quantum mechanics. The first is as generative chance* laws. Such laws involve a nonstandard notion of chance. The second is as probabilistic* constraining laws. Such laws impose randomness constraints that every physically possible world must satisfy. This algorithmic approach to physical randomness allows one to address a number of long-standing issues regarding the metaphysics of laws. In brief, since many histories permitted by traditional probabilistic laws are ruled out as physically impossible, it provides a tighter connection between probabilistic laws and their corresponding sets of possible worlds. But while the approach avoids one variety of empirical underdetermination, it reveals other varieties of underdetermination that are typically overlooked.
Friday, October 6, 2023
Saira Khan (Center for Philosophy of Science, University of Pittsburgh)
Title: Deliberation and Normativity in Decision Theory
Abstract: The prescriptions of our two most prominent strands of decision theory, evidential and causal, differ in general classes of problems known as Newcomb problems and decision instability problems. Attempts have been made at reconciling the two theories through deliberational models (Eells 1984; Skyrms 1982; Huttegger, forthcoming; Joyce 2012; Arntzenius 2008). However, philosophers have viewed deliberation very differently. In this talk, I consider how deliberational decision theory differs in the kinds of normative prescriptions it offers when compared with our traditional decision theories from Savage (1954), Jeffrey (1965) and their intellectual predecessors. This raises questions about whether deliberation is an appropriate method for reconciling evidential and causal decision theory.
Friday, September 29, 2023
Kevin T. Kelly (Carnegie Mellon University)
Watch the recording
PaDSCode: A^.$9hv$
Title: General (Distribution-free) Topological Characterization of Statistical Learnability
Abstract: In purely propositional models of learning, the possible propositional information states that the inquirer might encounter determine a topology on possible worlds that may be called the information topology. An important insight of topological learning theory is that learnability, both deductive (infallible) and inductive (fallible) can be characterized in terms of logical complexity relative to the information topology. Much follows, including a novel justification of Ockham's razor. However, none of that applies literally to statistical inference, in which one receives propositional information about a sample related to the world only by chance. Nonetheless, there are strong intuitive analogies between propositional and statistical learning that suggest a deeper connection. My former Ph.d. student Konstantin Genin [University of Tuebingen] ingeniously discovered such a connection by proving that there is a unique topology on statistical worlds such that learnability (almost surely or in chance) is characterized exactly in terms of complexity definable in that topology. Furthermore, the topology has a natural, countable basis whose elements may be thought of as propositional information states directly about the statistical world under study for purposes of proving negative results about statistical learnability. Alas, Genin's beautiful result is not fully general: it assumes that the chance that a sample hits exactly on the geometrical boundary of an elementary sample information state is necessarily zero, which essentially restricts the result to the discrete and continuous cases. This talk presents an extension of Genin's seminal result to the distribution-free case. The new result depends on a generalized concept of convergence in probability that makes sense even when the propositional information received from the sample is not itself subject to chance, which is of independent interest as an antidote to "chance bloat": the questionable frequentist tendency to assume that every sample event has a definite chance. This is very recent, unpublished work from my current book draft. For background, Genin's theorem is summarized in:
The Topology of Statistical Verifiability. (2017)
Konstantin Genin, Kevin T. Kelly.
In proceedings of TARK 2017, Liverpool.
Friday, September 15, 2023
Zoé Christoff (University of Groningen)
Title: Majority Illusions in Social Networks
Abstract: The popularity of an opinion in one’s circles is not necessarily a good indicator of its popularity in one’s entire community. For instance, when confronted with a majority of opposing opinions in one’s direct circles, one might get the impression that one belongs to a minority. From this perspective, network structure makes local information about global properties of the group potentially inaccurate. However, the way a social network is wired also determines what kind of information distortion can actually occur. We discuss which classes of networks allow for a majority of agents to be under such a ‘majority illusion’.
Friday, April 7, 2023
Kevin Dorst (MIT)
Title: Do Gamblers Commit Fallacies?
Abstract:The “gambler's fallacy" is the widely-observed tendency for people to expect random processes to “switch"—for example, to think that after a string of tails a heads is more likely. Is it irrational? Understood narrowly, it is—but we have little evidence that people exhibit it. Understood broadly, I'll argue that it follows from reasonable uncertainty combined with rational management of a limited memory.
Homotopy Type Theory 2023
The Second International Conference on Homotopy Type Theory (HoTT 2023) will take place Monday, May 22 to Thursday, May 25 at Carnegie Mellon University in Pittsburgh, USA.
Invited Speakers
- Julie Bergner (University of Virginia, USA)
- Thierry Coquand (Chalmers University, Sweden)
- András Kovács (Eötvös Loránd University, Hungary)
- Anders Mörtberg (Stockholm University, Sweden)
There will also be a special Vladimir Voevodsky Memorial Lecture given by Michael Shulman (University of San Diego, USA).
Scientific Committee
- Thorsten Altenkirch (University of Nottingham, UK)
- Steve Awodey (Carnegie Mellon University, USA)
- Benno van den Berg (University of Amsterdam, Netherlands)
- Dan Christensen (University of Western Ontario, Canada)
- Nicola Gambino (University of Manchester, UK), chair
- Hugo Herbelin (INRIA, France)
- Peter LeFanu Lumsdaine (Stockholm University, Sweden)
- Maria Emilia Maietti (University of Padova, Italy)
- Emily Riehl (Johns Hopkins University, USA)
Sponsorship
HoTT 2023 is an official ASL-sponsored meeting.
We are happy to announce a grant from the National Science Foundation which will provide modest student travel awards to attend HoTT 2023. Women and members of minority groups are strongly encouraged to apply. Students and recently graduated postdocs can be supported, whether they submit a paper or not.
Local Committee
- Mathieu Anel
- Carlo Angiuli
- Steve Awodey (chair)
- Jonas Frey
- Andrew Swan
The meeting is being hosted by the HoTT group at CMU.
Contact: hott2023conference@gmail.com.
Friday, March 24, 2023
Jingyi Wu (Department of Logic and Philosophy of Science, UC Irvine)
Title: Modeling Injustice in Epistemic Networks
Abstract: I use network models to explore how social injustice impacts learning in a community. First, I simulate situations where a dominant group devalues evidence from a marginalized group. I find that the marginalized group ends up developing better beliefs. This result uncovers a mechanism by which standpoint advantages for the marginalized group can arise because of testimonial injustice. Interestingly, this model can be reinterpreted to capture another kind of injustice—informational injustice—between industrial and academic scientists. I show that a subgroup of scientists can learn more accurately when they unilaterally withhold evidence.
Tuesday, March 21, 2023
Gert de Cooman (Foundations Lab, Ghent University)
Title: Cooperation, Maximin, and the Foundations of Ethics
Abstract: I intend to discuss the representation of indifference in inference and decision making under uncertainty, in the very general context of coherent partial preference orderings, or coherent sets of desirable options.
I’ll begin with a short discussion of the basic model—coherent sets of desirable options, and intend to show how it can capture many relevant aspects of so-called conservative probabilistic inference. In particular, I’ll explain how this model leads in particular to coherent lower and upper previsions or expectations, conditioning, and coherent conditional (precise) previsions.
I’ll then discuss how a notion of indifference can be introduced into this context, and what its effects are on desirability models, through representation results. In this context, the different notions of *conservative inference under indifference* and *updating under indifference* make their appearance.
I’ll then present a number of examples: specific useful and concrete instances of the abstract notion of option space and the abstract notion of indifference. Such as:
- observing an event and conditioning a coherent set of desirable gambles on this observation;
- observing the outcome of a measurement and Lüders’ conditionalisation in quantum mechanics;
- exchangeability and de Finetti’s representation theorem in an imprecise probabilities context.
Friday, February 24, 2023
Derek Leben (Carnegie Mellon University)
Title: Cooperation, Maximin, and the Foundations of Ethics
Abstract: A Social Contract view about meta-ethics proposes that normative principles can be causally and functionally explained as solutions to cooperation problems, and they can therefore be evaluated by how effectively they solve these problems. However, advocates of the Social Contract view have often not specified details about what counts as a cooperation problem, and what solutions to it would look like. I propose that we define cooperation problems as interactions where there exists at least one Strong Pareto-Improvement on every pure Nash Equilibrium (willfully ignoring mixed solutions). We will explore a range of solutions to this problem, and how these solutions correspond to various normative principles. In the past, I have advocated the Maximin principle as an optimal solution to cooperation problems, but this turns out to be incomplete at best, and mistaken at worst. I will end with a plea for help from others who are more knowledgeable and intelligent than I am.
Friday, February 10, 2023
Adam Bjorndahl (Carnegie Mellon University)
Title: Knowledge Second
Abstract: Classical philosophical analyses seek to explain knowledge as deriving from more basic notions. The influential "knowledge first" program in epistemology reverses this tradition, taking knowledge as its starting point. From the perspective of epistemic logic, however, this is not so much a reversal as it is the default—the field arguably begins with the specialization of "necessity" to "epistemic necessity"; that is, it begins with knowledge. In this context, putting knowledge *second* would be the reversal.
In this talk I will motivate, develop, and explore such a "knowledge second" approach in epistemic logic, founded on distinguishing what a body of evidence actually entails from what it is (merely) believed to entail. I'll import a logical framework that captures exactly this distinction and use it to define formal notions of "internal" and "external" justification; these will then be applied to yield new insights into old topics, namely the KK principle and the regress problem. I will close with some remarks about the "definition" of knowledge and/or extensions of this framework to the probabilistic setting.
Friday, January 27, 2023
Tessa Murthy (Carnegie Mellon University)
Title: From Borel's paradox to Popper's bridge: the Cournot principle and almost-sure convergence
Abstract: Adherents to non-frequentist metaphysics of probability have often claimed that the axioms of frequentist theories—in particular, the existence of a limiting relative frequency in infinite iteration of chance trials—can be derived as theorems of alternate characterizations of probability by means of convergence theorems. This project constitutes a historical analysis of a representative such attempt: Karl Popper's "bridge" between propensities and frequencies as discussed in his books Logic of Scientific Discovery and Realism and the Aim of Science. I reconstruct the motivation for Popper's argument, focusing on its relationship to the so-called "Borel paradox" outlined by van Lambalgen in his doctoral dissertation. I then discuss the structure of, reception toward, and debate around the argument. Richard von Mises argued that Popper assumes an empirical reading of the law of large numbers which is circular in context. The crucial problem is that SLLN is an almost-sure theorem; it has a measure zero exclusion clause. But taking measure-zero sets to be probability-zero sets is a frequentist assumption. It presupposes von Mises' limit axiom, which is exactly what Popper is attempting to derive.
Two features of the debate, however, have not received substantial historical consideration. First, Popper was evidently aware of his assumption about the law of large numbers, and even joined von Mises in criticizing Frechet's more heavy-handed application of it. Second, statistical convergence theorems like SLLN require that trials satisfy independence conditions (usually i.i.d.); without presupposing also von Mises' second axiom, it is not clear why this antecedent condition should be assumed. My primary contributions are (1) an explanation of why this mutual misunderstanding between von Mises and Popper arose, and (2) a charitable interpretation of Popper's assumptions that better justifies the undiscussed premise that experimental iterations that give rise to Kollektivs satisfy the antecedent of the SLLN. For the first, I discuss Popper's use of the "Cournot principle" and evaluate whether it permits a nonfrequentist to infer that null sets are "impossible events." For the second, I introduce Popper's n-freedom criterion and his reference to Doob's theorem. Though this historical investigation paints Popper's approach as more statistically informed than is commonly thought, it does not get him entirely out of trouble. I close by evaluating Popper's metaphysics of probability as a hybrid view, in which his assumption of the Cournot principle functions as a moderate frequentist preconception that does not entail the stronger axioms used by canonical frequentists like von Mises and Reichenbach.
Friday, December 9, 2022
Nevin Climenhaga (Dianoia Institute of Philosophy, Australian Catholic University)
Title: Are Simpler Worlds More Probable?
Abstract: Some philosophers have suggested that simpler worlds are more intrinsically probable than less simple worlds, and that this vindicates our ordinary inductive practices. I show that an a priori favoring of simpler worlds delivers the intuitively wrong result for worlds that include random or causally disconnected processes, such as the tosses of fair coins. I conclude that while simplicity may play a role in determining probabilities, it does not do so by making simpler worlds more probable.
Friday, December 2, 2022
Yanjing Wang (Peking University)
Title: Knowing How to Understand Intuitionistic Logic
Abstract: In this talk, we propose an approach to “decode” intuitionistic logic and various intermediate logics as (dynamic) epistemic logics of knowing how. Our approach is inspired by scattered ideas hidden in the vast literature of math, philosophy, CS, and linguistics about intuitionistic logic, which echoed Heyting’s initial conception of intuitionistic truth as “knowing how to prove.” This notion of truth is realized by using a bundled know-how modality based on some formalized Brouwer–Heyting–Kolmogorov interpretation. Our approach reveals the hidden, complicated epistemic information behind the innocent-looking connectives by providing intuitive epistemic readings of formulas in intermediate logics. As examples, we show how to decode inquisitive logic and some version of dependence logic as epistemic logics. If time permits, we show how similar ideas can be applied to deontic logic.
Tuesday, November 22, 2022
Sander Beckers (University of Tübingen)
Title: Causal Explanations and XAI
Abstract: Although standard Machine Learning models are optimized for making predictions about observations, more and more they are used for making predictions about the results of actions. An important goal of Explainable Artificial Intelligence (XAI) is to compensate for this mismatch by offering explanations about the predictions of an ML-model which ensure that they are reliably action-guiding. As action-guiding explanations are causal explanations, the literature on this topic is starting to embrace insights from the literature on causal models. Here I take a step further down this path by formally defining the causal notions of sufficient explanations and counterfactual explanations. I show how these notions relate to (and improve upon) existing work, and motivate their adequacy by illustrating how different explanations are action-guiding under different circumstances. Moreover, this work is the first to offer a formal definition of actual causation that is founded entirely in action-guiding explanations. Although the definitions are motivated by a focus on XAI, the analysis of causal explanation and actual causation applies in general. I also touch upon the significance of this work for fairness in AI by showing how actual causation can be used to improve the idea of path-specific counterfactual fairness.
Friday, November 18, 2022
Tom Sterkenburg (MCMP, LMU Munich)
Title: Machine learning and the philosophical problem of induction
Abstract: Hume's classical argument says that we cannot justify inductive inferences. Impossibility results like the no-free-lunch theorems underwrite Hume's skeptical conclusion for machine learning algorithms. At the same time, the mathematical theory of machine learning gives us positive results that do appear to provide justification for standard learning algorithms. I argue why there is no conflict here: rather, there are two different conceptions of formal learning methods, that lead to two different demands on their justification. I further discuss how these different perspectives relate to prominent contemporary proposals in the philosophy of inductive inference (including Norton's material theory and Schurz's meta-inductive justification of induction), and how they support two broader epistemological outlooks on automated inquiry.
Friday, November 4, 2022
Krzysztof Mierzewski (Carnegie Mellon University)
Title: Probing the qualitative-quantitative divide in probability logics
Abstract: Several notable approaches to probability, going back at least to Keynes (1921), de Finetti (1937), and Koopman (1940), assign a special importance to qualitative, comparative judgments of probability ("event A is at least as probable as B"). The difference between qualitative and explicitly quantitative probabilistic reasoning is intuitive, and one can readily identify paradigmatic accounts of each type of inference. It is less clear, however, whether there are any natural structural features that track the difference between inference involving comparative probability judgments on the one hand, and explicitly numerical probabilistic reasoning on the other. Are there any salient dividing lines that can help us understand the relationship between the two, and classify intermediate forms of inference lying in between the two extremes?
In this talk, I will explore this question from the perspective of probability logics. Probability logics can represent probabilistic reasoning at different levels of grain, ranging from the more 'qualitative' logic of purely comparative probability to explicitly 'quantitative' languages involving arbitrary polynomials over probability terms. I will identify a robust boundary in the space of probability logics by distinguishing systems that encode merely additive reasoning from those that encode additive and multiplicative reasoning. The latter include not only languages with explicit multiplication, but also languages expressing notions of probabilistic independence and comparative conditional probability.
As I will explain, this distinction tracks a divide in computational complexity: for additive systems, the satisfiability problem remains NP-complete, while systems that can encode even a modicum of multiplication are robustly complete for ETR (the existential theory of the reals). I will then address some questions about axiomatisation by presenting new completeness results, as well as a proof of non-finite-axiomatisability for comparative probability. For purely additive systems, completeness proofs involve familiar methods from linear algebra, relying on Fourier-Motzkin elimination and hyperplane separation theorems; for multiplicative systems, completeness relies on results from real algebraic geometry (the Positivstellensatz for semi-algebraic sets). If time permits, I will highlight some important questions concerning the axiomatisation of comparative conditional probability.
We will see that, for the multiplicative probability logics as well as the additive ones, the paradigmatically 'qualitative’ systems are neither simpler in terms of computational complexity, nor in terms of axiomatisation, while losing in expressive power to their explicitly numerical counterparts.
This is joint work with Duligur Ibeling, Thomas Icard, and Milan Mossé.
Friday, October 21, 2022
Maryam Rostamigiv (Carnegie Mellon University)
Title: About the type of modal logic for the unification problem
Abstract: I'll talk about the unification problem in ordinary modal logics, two-modal logic fusions, and multi-modal epistemic logics. Given a formula A and a propositional logic L, in a unification problem we should find substitutions s such that s(A) is in L. These substitutions are known as unifiers of A in L. When they exist, we investigate various methods for constructing minimal complete sets of unifiers of a given formula A, and we discuss the unification type of A based on the cardinality of these minimal complete sets. Then, I will present the unification types of several propositional logics.
Friday, October 7, 2022
Brittany Gelb (Rutgers University) and Philip Sink (Carnegie Mellon University)
Title: Modal Logic Without Possible Worlds
Abstract: We will present a semantics for modal logic based on simplicial complexes that instead of possible worlds uses an "Agent Perspective". Philip will explain the details of the formalism, including a novel soundness and completeness proof. Brittany will follow up with some applications of these models to a distributed setting. Additionally, she will show how we can use tools from algebraic topology to show a variety of things including the nonexistence of bisimulations.
The Center for Formal Epistemology at Carnegie Mellon University presents:
Learning, Randomness, and Complexity
An interdisciplinary workshop aimed at exploring the connections between inductive inference, complexity, and computation, including the theory of algorithmic randomness and its philosophical ramifications.
Saturday, October 8 and Sunday, October 9, 2022
Adamson Wing, Baker Hall
If you have any questions, please contact Francesca Zaffora Blando, Kevin Kelly, or Lisa Everett.
Friday, September 23, 2022
Mikayla Kelley, Stanford University
Title: A Contextual Accuracy Dominance Argument for Probabilism
Abstract: A central motivation for Probabilism—the principle of rationality that requires one to have credences that satisfy the axioms of probability—is the accuracy dominance argument: one should not have accuracy dominated credences, and one avoids accuracy dominance just in case one satisfies Probabilism. Up until recently, the accuracy dominance argument for Probabilism has been restricted to the finite setting. One reason for this is that it is not easy to measure the accuracy of infinitely many credences in a motivated way. In particular, as recent work has shown, the conditions often imposed in the finite setting are mutually inconsistent in the infinite setting. One response to these impossibility results—the one taken by Michael Nielsen—is to weaken the conditions on a legitimate measure of accuracy. However, this response runs the risk of offering an accuracy dominance argument using illegitimate measures of accuracy. In this paper, I offer an alternative response which concedes the possibility that not all sets of credences can be measured for accuracy. I then offer an accuracy dominance argument for Probabilism that allows for this restrictedness. The normative core of the argument is the principle that one should not have credences that would be accuracy dominated in some epistemic context one might find oneself in if there are alternative credences which do not have this defect.
Friday, September 9, 2022
Francesca Zaffora Blando, Carnegie Mellon University
Title: Randomness as the stable satisfaction of minimal randomness conditions
Abstract: What are the weakest properties you would expect an infinite sequence of zeroes and ones to possess if someone told you that that sequence is random (think: maximally irregular and patternless)? Perhaps you would expect that sequence to be uncomputable or, with a little more reflection, bi-immune. Perhaps you would expect it to satisfy the Strong Law of Large Numbers (namely, you would expect the limiting relative frequency of 0, and of 1, along that sequence to be 1/2). None of these properties is, by itself, sufficient for randomness. For instance, the sequence 01010101… satisfies the Strong Law of Large Numbers, yet it is very regular. But what if, similarly to what von Mises did when defining collectives, we instead required these properties to be satisfied stably? In other words, what if we required them to be preserved under an appropriate class of transformations? Would this suffice to obtain reasonable randomness notions? In this talk, I will discuss some work (very much) in progress that addresses this question and its connections with von Mises’ early work on randomness.
Friday, May 6, 2022
Philip Sink (CMU)
Title: A Logical Model of Pluralistic Ignorance
Abstract: Much of the existing literature on pluralistic ignorance suggests that agents who find themselves in such a situation must consider themselves "special" in one way or another (Grosz 2018, Bjerring et al. 2014). Agents have to recognize their own dishonesty, but believe everyone around them is perfectly honest. This argument is taken to show that pluralistic ignorance is irrational. Modifying work from Christoff 2016, we use a simple logical model to show that these arguments for the irrationality of pluralistic ignorance depend on various introspection assumptions. We will finish by putting forth various scenarios where agents can be honest, headstrong, or something similar (generally taken to be impossible under pluralistic ignorance) but are nonetheless consistent if one relaxes introspection assumptions. This shows that agents can see themselves as no different from their friends and still be in a situation of pluralistic ignorance with sufficiently weak introspection assumptions.
Friday, April 29, 2022
Marina Dubova, Indiana University
Watch the Zoom recording
View the slides
Title: "Against theory-motivated data collection in science"
Abstract: We study the epistemic success of data collection strategies proposed by philosophers of science or executed by scientists themselves. We develop a multi-agent model of the scientific process that jointly formalizes its core aspects: data collection, data explanation, and social learning. We find that agents who choose new experiments at random develop the most accurate accounts of the world. On the other hand, the agents following the confirmation, falsification, crucial experimentation (theoretical disagreement), or novelty-motivated strategies end up with an illusion of epistemic success: they develop promising accounts for the data they collected, while completely misrepresenting the ground truth that they intended to learn about. These results, while being methodologically surprising, reflect basic principles of statistical learning and adaptive sampling.
Friday, April 8, 2022
Tom Wysocki, University of Pittsburgh
Title: "Causal Decision Theory for the Probabilistically Blind"
Abstract: If you can’t or don’t want to ascribe probabilities to the consequences of your actions, classic causal decision theory won’t let you reap the undeniable benefits of causal reasoning for decision making. I intend the following theory to fix this problem.
First, I explain in more detail why it’s good to have a causal decision theory that applies to non-deterministic yet non-probabilistic decision problems. One of the benefits of such a theory is that it’s useful for agents under bounded uncertainty. I then introduce the underdeterministic framework, which can represent non-probabilistic causal indeterminacies. Subsequently, I use the framework to formulate underdeterministic decision theory. On this theory, a rational agent under bounded uncertainty solves a decision problem in three steps: she represents the decision problem with a causal model, uses it to infer the possible consequences of available actions, and chooses an action whose possible consequences are no worse than the possible consequences of any other action. The theory applies to decisions that have infinitely many mutually inconsistent possible consequences and to agents who can’t decide on a single causal model representing the decision problem.
Friday, March 25, 2022
Alicja Kowalewska, Carnegie Mellon University
Watch a recording of this talk
Title: "Measuring story coherence with Bayesian networks" (joint work with Rafał Urbaniak, University of Gdańsk)
Abstract: When we say that one’s views or story are more or less coherent, we seem to think of how well their individual pieces fit together. However, explicating this notion formally turns out to be tricky. In this talk, I’ll describe a Bayesian network-based coherence measure, which performs better than its purely probabilistic predecessors. The novelty is that by paying attention to the structure of the story encoded in the network, we avoid considering all possible pairs of subsets of a story. Moreover, our approach assigns special importance to the weakest links in a story, to improve on the other measures’ results for logically inconsistent scenarios. I’ll discuss the performance of the measures in relation to a few philosophically motivated examples and the real-life case of Sally Clark.
Friday, February 25, 2022
Pablo Zendejas Medina, University of Pittsburgh
Title: "Rational Inquiry for Qualitative Reasoners"
Abstract: If you believe something, you're also committed to believing that any future evidence to the contrary would be misleading. Thus, it would seem to be irrational to inquire further into a question that you already have a belief about, when you only care about the accuracy of that belief. This is a version of the Dogmatism Paradox. In this talk, I'll show how the paradox can be solved, even granting its core assumptions, if we make the right assumptions about belief revision and about how belief licenses action. Moreover, the argument generalizes: it turns out that given these assumptions, inquiry is always rational and often even rationally required. On the resulting view, an opinionated inquirer believes that they won't encounter defeating evidence, but still inquires in case they turn out to be mistaken.
Friday, February 11, 2022
Johanna Thoma, London School of Economics
Watch a recording of this talk
Title: "What’s wrong with pure risk paternalism?"
Abstract: A growing number of decision theorists have, in recent years, defended the view that rationality is permissive in the sense that there is rational leeway in how agents who value outcomes in the same way may choose under risk, allowing for different levels of ‘pure’ risk aversion or risk inclination. Granting such permissiveness complicates the question of what attitude to risk we should implement when choosing on behalf of another person. More specifically, my talk is concerned with the question of whether we are pro tanto required to defer to the risk attitudes of the person on whose behalf we are choosing, that is, whether what I call ‘pure risk paternalism’ is problematic. I illustrate the practical and theoretical significance of this question, before arguing that the answer depends less on one’s specific account of when and why paternalism is problematic more generally, and more on what kinds of attitudes we take pure risk attitudes to be.
Friday, January 28, 2022
Johan van Benthem, University of Amsterdam, Stanford University, and Tsinghua University
Title: "Venturing Further Into Epistemic Topology"
Abstract: Epistemic topology studies key ideas and issues from epistemology with mathematical methods from topology. This talk pursues one particular issue in this style: the nature of informational dependence. We combine two major semantic views of information in logic: as 'range' (the epistemic logic view) and as 'correlation' (the situation theory view), in one topological framework for knowledge and inquiry arising out of imprecise empirical observations. Technically, we present a decidable and complete modal base logic of information-carrying dependence through continuous functions. Our treatment uncovers new connections with other areas of mathematics: topological independence matches with 'everywhere surjective functions', stricter requirements of computability lead to a complete modal logic for Domain Theory with Scott topology. Finally, we move from topology to Analysis and offer a logical take on uniform continuity, viewed as a desirable form of epistemic know-how, modeled in metric spaces, but also in new qualitative mathematical theories of approximation in terms of entourages. Beyond concrete results, the talk is meant to convey a certain spirit: how epistemic topology can profit from venturing more deeply into mathematics.
References
- Baltag & J. van Benthem, 2021, A Minimal Logic of Functional Dependence
- 2021, Knowability and Continuity, A Topological Account of Informational Dependence, manuscript, ILLC Amsterdam.
- 2018, Some Thoughts on the Logic of Imprecise Measurement
Friday, December 10, 2021
Xin Hui Yong, University of Pittsburgh
Title: "Accidentally I Learnt: On relevance and information resistance”
Abstract: While there has been a movement aiming to teach agents about their privilege by making the information about their privilege as costless as possible, Kinney & Bright argue risk-sensitive frameworks (particularly Lara Buchak's, from Risk and Rationality) can make it rational for privileged agents to shield themselves from learning about their privilege, even if the information is costless and relevant. In response, I show that in this framework, if the agent is not certain if the information will be relevant, they may have less reason to actively uphold ignorance. I explore what the agent's uncertainty about the relevance of the information could describe, and what this may mean upshot-wise. For example, these educational initiatives may not be as doomed as Kinney & Bright suggest, and risk-sensitive frameworks like Buchak's can lead to situations where an agent would feel better off having had learned something at the same time that they may rationally decline to know it now. I aim to explore these upshots and what they say about elite group ignorance and the viability of risk-sensitive expected utility theory as a helpful explanation of elite agent ignorance.
Friday, November 19, 2021
Taylor Koles, University of Pittsburgh
Title: "Higher-Order Sweetening Problems for Schoenfield"
Abstract: I argue against a particular motivation for adopting imprecise credences advanced by Schoenfield 2012. Schoenfield's motivates imprecise credences by arguing that it is permissible to be insensitive to mild evidential sweetening. Since mild sweetening can be iterated, Schoenfield's position that our credences should be modeled by a set of precise probability functions ensures that even on her view it is at least sometimes impermissible to be insensitive to mild sweetening. Taking a lesson from the literature on higher-order vagueness, I argue that the better approach is to get off the slope at the first hill - a perfectly rational agent would not be insensitive to mild evidential sweetening.
Friday, November 5, 2021
Snow Zhang, New York University
Recording (paDSCode: 8ua%69nP)
Title: Updating Stably
Abstract: Bayesianism appears to give counter-intuitive recommendations in cases where the agent lacks evidence about what their evidence is. I argue that, in such cases, Bayesian conditionalization is rationally defective as an updating plan. My argument relies on a new norm for rational plans: self-stability. Roughly, a plan is self-stable if it gives the same recommendation conditional on its own recommendations. The primary goal of this talk is to give a precise formulation of this norm. The secondary goal is to show how this norm relates to other norms of rationality.
Friday, October 22, 2021
Kenny Easwaran, Texas A&M University
Watch the Recording
Title: "Generalizations of Risk-Weighted Expected Utility"
Abstract: I consider Lara Buchak’s (2013) “risk-weighted expected utility” (REU) and provide formal generalizations of it. I do not consider normative motivations for any of these generalizations, but just show how they work formally. I conjecture that some of these generalizations might result from very slightly modifying the assumptions that go into her representation theorems, but I don’t investigate the details of this.
I start by reviewing the formal definition of REU for finite gambles, and two ways to calculate it by sums of weighted probabilities. Then I generalize this to continuous gambles rather than finite ones, and show two analogous ways to calculate versions of REU by integrals. Buchak uses a risk-weighting function that maps the probability interval [0,1] in a continuous and monotonic way to the weighted interval [0,1]. I show that if we choose some other closed and bounded interval, the result is formally equivalent, and I show how to generalize it to cases where the interval is unbounded. In these cases, the modified REU can provide versions of maximin (if the interval starts at −∞), maximax (if the interval ends at +∞) as well as something new if both ends of the interval are infinite. However, where maximin and maximax are typically either indifferent or silent between gambles that agree on the relevant endpoint, the decision rules formally defined here are able to consider intermediate outcomes of the gamble in a way that is lexically posterior to the endpoint(s).
Finally, I consider the analogy between risk-sensitive decision rules for a single agent and inequality-sensitive social welfare functions for a group. I show how the formal generalizations of REU theory allow for further formal generalizations of inequality-sensitivity that might have some relevance for population ethics.
Friday, October 15, 2021
Francesca Zaffora Blando, Carnegie Mellon University
Title: Wald randomness and learning-theoretic randomness
Abstract: The theory of algorithmic randomness has its roots in Richard von Mises’ work on the foundations of probability. Von Mises was a fervent proponent of the frequency interpretation of probability, which he supplemented with a (more or less) formal definition of randomness for infinite sequences of experimental outcomes. In a nutshell, according to von Mises’ account, the probability of an event is to be identified with its limiting relative frequency within a random sequence. Abraham Wald’s most well-known contribution to the heated debate that immediately followed von Mises’ proposal is his proof of the consistency of von Mises’ definition of randomness. In this talk, I will focus on a lesser known contribution by Wald: a definition of randomness that he put forth to rescue von Mises’ original definition from the objection that is often regarded as having dealt the death blow to his entire approach (namely, the objection based on Ville’s Theorem). We will see that, when reframed in computability-theoretic terms, Wald’s definition of randomness coincides with a well-known algorithmic randomness notion and that his overall approach is very close, both formally and conceptually, to a recent framework for modeling algorithmic randomness that rests on learning-theoretic tools and intuitions.
Friday, September 24, 2021
Kevin Zollman, Carnegie Mellon University
Recording (paDSCode: x4%h1j^a)
Slides
Title: "Is 'scientific progress through bias' a good idea?"
Abstract: Some philosophers have argued for a paradoxical conclusion: that science advances because of the IRrationality of scientists. That is, by combining epistemically inferior behavior on the part of individual scientists with a social structure that harnesses this irrationality, science can make progress faster than it could with more rational individuals. Through the use of a simple computational model, I show how this is indeed possible: biased scientist do in fact make more scientific progress than an equivalent community which is unbiased. However, this model also illustrates that such communities are very fragile. Small changes in their social structure can move biased communities from being very good to being abysmal.
Center for Formal Epistemology Talk
Friday, September 10, 2021
Prof. Kevin Kelly will present his joint work with Hanti Lin (UC Davis) and Konstantin Genin (University of Tuebingen) on "Ockham's Razor, Inductive Monotonicity, and Topology” (the abstract is below).
Title: Ockham's Razor, Inductive Monotonicity, and Topology
Abstract: What is empirical simplicity? What is Ockham's razor? How could Ockham's razor find hidden structure in nature better than alternative inductive biases unless you assume a priori that the truth is simple? We present a new explanation. The basic idea is to amplify convergence to the truth in the limit with constraints on the monotonicity (stability) of convergence. Literally monotonic convergence to the truth is hopeless for properly inductive problems, since answering the question implies the possibility of false steps en route to the truth. *Inductive monotonicity* requires, more weakly, that the method never retracts from a true conclusion to a false one, which sounds like a straightforward epistemic consideration (i.e., it is weaker than Plato's requirement in the Meno that knowledge be stable true belief). We show (very easily) that inductively monotonic convergence to the truth implies that your inductive method satisfies Ockham's razor at *every* stage of inquiry, which projects a long-run criterion of success into the short run, with no appeal to an alleged short-run notion of "inductive support" of universal conclusions.
The main result is a basic proposition in point set topology. Statistics, ML, and the philosophy of science have bet their respective banks on probability and measure as the "right" concepts for explaining scientific method. We respond that the central considerations of scientific method: empirical information, simplicity, fine-tuning, and relevance are all fundamentally topological and are most elegantly understood in topological terms.
Watch, Listen or Read the recording
2021 CMU HoTT Graduate Student Workshop
This event is a gathering of graduate students, to exchange ideas related to homotopy type theory, logic, and category theory. The workshop will consist of a series of presentations, with opportunities for discussion. This event is meant to be friendly and informal, and talks on work in progress and unfinished ideas are welcome.
For more information, please visit the workshop website or contact Jonas Frey.
Center for Ethics & Policy
2021 Workshop on Political Philosophy in Bioethics
February 19-20, 2021
Organizer: Danielle M. Wenner
Annual Meeting of the Society for Exact Philosophy (SEP)
THIS EVENT HAS BEEN CANCELED
June 12-14, 2020
Organizer: Kevin Zollman
The Society for Exact Philosophy is an international scholarly association, founded in 1970, to provide sustained discussion among researchers who believe that rigorous methods have a place in philosophical investigations. To this end, the Society meets annually, alternating between locations in Canada and the U.S.
Call for Papers
The Society for Exact Philosophy invites submissions of papers in all areas of analytic philosophy for its 2020 meeting.
Paper submission deadline: March 1, 2020 (Notification by April 1) via Easychair
Models of Morality, Morality of Models
Center for Formal Epistemology Workshop
March 6-7, 2020
This workshop is free to attend. Please register at the webpage so we can ensure sufficient seating.
Organizers: David Danks, Kevin Kelly, Simon Cullen
Recent years have seen an explosion of research into the empirical bases of human moral judgment along with a corresponding interest in formal and computational models of human morality. At the same time, AI and robotics researchers aim to develop systems that are themselves capable of moral judgment, and so require some model of morality. With this workshop, we hope to spur new and generative collaborations between researchers pursuing these two parallel lines of inquiry.
Confirmed Speakers:
- Cristina Bicchieri (University of Pennsylvania)
- Simon Cullen (Carnegie Mellon University)
- Oriel FeldmanHall (Brown University)
- Seth Lazar (Australian National University)
- Annette Zimmerman (Princeton University)
Formal Methods in Mathematics / Lean Together 2020
January 6-10, 2020
Formal Methods in Mathematics / Lean Together 2020 is a gathering of those interested in the Lean interactive theorem prover, and, more generally, formal methods in mathematics and computer science.
The meeting is a successor to Lean Together 2019.
The first three days will focus on formal methods in pure and applied mathematics, including interactive theorem proving, automated reasoning, verification of symbolic and numeric computation, and general mathematical infrastructure.
The last two days will be devoted to specifically to the Lean Theorem Prover and its core library, mathlib. Users and library developers will have opportunities to present work in progress and discuss plans for the future.
Attendance is free and open to the public, but we ask you to let us know by December 6 if you plan to come. If you are tentatively planning to attend, please tell us now and update us if your plans change. You register via the web page above, or contact the organizers, Jeremy Avigad and Robert Y. Lewis.
The meeting is supported by grant FA9550-18-1-0325 from the Air Force Office of Scientific Research. The contents do not necessarily reflect the views of the AFOSR.
Organizer: Prof. Jeremy Avigad
International Conference on Homotopy Type Theory
12-17 August 2019
INVITED SPEAKERS
Ulrik Buchholtz (TU Darmstadt, Germany)
Dan Licata (Wesleyan University, USA)
Andrew Pitts (University of Cambridge, UK)
Emily Riehl (Johns Hopkins University, USA)
Christian Sattler (University of Gothenburg, Sweden)
Karol Szumilo (University of Leeds, UK)
SUMMER SCHOOL
There will also be an associated Homotopy Type Theory Summer School in the preceding week, August 7th to 10th. The instructors and topics will be:
- Cubical methods: Anders Mortberg (Carnegie Mellon University, USA)
- Formalization in Agda: Guillaume Brunerie (Stockholm University, Sweden)
- Formalization in Coq: Kristina Sojakova (Cornell University, USA)
- Higher topos theory: Mathieu Anel (Carnegie Mellon University, USA)
of type theory: Jonas Frey (Carnegie Mellon University, USA)Semantics -
Synthetic homotopy theory: Egbert Rijke (University of Illinois, USA)
SCIENTIFIC COMMITTEE
Steve Awodey (Carnegie Mellon University, USA)
Andrej Bauer (University of Ljubljana, Slovenia)
Thierry Coquand (University of Gothenburg, Sweden)
Nicola Gambino (University of Leeds, UK)
Peter LeFanu Lumsdaine (Stockholm University, Sweden)
Michael Shulman (University of San Diego, USA),
Geometry in Modal Homotopy Type Theory
March 11-15, 2019
Homotopy Type Theory (HoTT) is one of the tools to reason within a higher topos. The recent extensions of HoTT by Modalities has led to stronger relations to the use of higher toposes in Topology, Differential Geometry
20th Annual Pitt-CMU Graduate Student Philosophy Conference
Uncertainty and the Limits of Knowledge
March 16-17, 2019
Philosophical Issues in Research Ethics
Center for Ethics and Policy
November 2-3, 2018
The CEP’s second biennial workshop on ethics and policy will bring scholars together from across North America to discuss philosophical issues in research ethics. The goal of this workshop is to promote more philosophically rigorous work in the field of research ethics and to bring more philosophers into the research ethics community.
Logic, Information, and Topology Workshop
Center for Formal Epistemology
Saturday, October 20, 2018 - 9-6pm
Baker Hall 136A - Adamson Wing
Dynamic epistemic logic concerns the information conveyed by the beliefs of other agents. Belief revision theory studies rational belief change in light of new information. Formal learning theory concerns systems that learn the truth on increasing information. Topology is emerging as a particularly apt formal perspective on the underlying concept of propositional information. The talks in this workshop address the preceding themes from a range of overlapping perspectives.
Workshop Organizers:
Kevin Kelly
Adam Bjorndahl
Workshop Schedule
Invited Speakers:
Alexandru Baltag - Institute for Logic, Language and Computation (ILLC), Amsterdam
KNOWABLE DEPENDENCY: A TOPOLOGICAL ACCOUNT
If to be is to be the value of a variable, then to know is to know a functional dependence between variables. (Moreover, the conclusion may still be arguably be true even if Quine's premise is wrong...) This points towards a fundamental connection between Hintikka's Epistemic Logic and Vaananen 's so-called Dependence Logic (itself anticipated by the Independence-Friendly Logic of Hintikka and Sandu). The connection was made precise in the Epistemic Dependence Logic introduced in my 2016 AiML paper. Its dynamics captures the widespread view of knowledge acquisition as a process of learning correlations (with the goal of eventually tracking causal relationships in the actual world). However, when talking about empirical variables in natural sciences, the exact value might not be knowable, and instead only inexact approximations can be known. This leads to a topological conception of empirical variables, as maps from the state space into a topological space. Here, the exact value of the variable is represented by the output of the map, while the open neighborhoods of this value represent the knowable approximations of the exact answer. I argue that knowability of a dependency amounts in such an empirical context to the continuity of the given functional correlation. To know (in natural science) is to know a continuous dependence between empirical variables.
Eric Pacuit - University of Maryland
BELIEFS, PROPOSITIONS and DEFINITE DESCRIPTIONS
In this paper, we introduce a doxastic logic with expressions that are intended to represent definite descriptions for propositions. Using these definite descriptions, we can formalize sentences such as:
- Ann believes that the strangest proposition that Bob believes is that neutrinos travel at twice the speed of light.
- Ann believes that the strangest proposition that Bob believes is false.
The second sentence has both de re and de dicto readings, which are
distinguished in our logic. We motivate our logical system with a novel analysis of the Brandenburger-Keisler paradox. Our analysis of this paradox uncovers an interesting connection between it and the Kaplan-Montague Knower paradox.
(This is joint work with Wes Holliday)
Adam Bjorndahl - Carnegie Mellon University
THE EPISTEMOLOGY OF NONDETERMINISM
Propositional dynamic logic (PDL) is a framework for reasoning about nondeterministic program executions (or, more generally, nondeterministic actions). In this setting, nondeterminism is taken as a primitive: a program is nondeterministic iff it has multiple possible outcomes. But what is the sense of "possibility" at play here? This talk explores an epistemic interpretation: working in an enriched logical setting, we represent nondeterminism as a relationship between a program and an agent deriving from the agent’s (in)ability to adequately measure the dynamics of the program execution. More precisely, using topology to capture the observational powers of an agent, we define the nondeterministic outcomes of a given program execution to be those outcomes that the agent is unable to rule out in advance. In this framework, continuity turns out to coincide exactly with determinism: that is, determinism is continuity in the observation topology. This allows us to embed PDL into (dynamic) topological (subset space) logic, laying the groundwork for a deeper investigation into the epistemology (and topology) of nondeterminism.
Kasey Genin - University of Toronto
THE TOPOLOGY OF STATISTICAL INQUIRY
Taking inspiration from Kelly's The Logic of Reliable Inquiry (1996), Baltag et. al. (2015) and Genin and Kelly (2015) provide a general topological framework for the study of empirical learning problems. Baltag et. al. (2015) prove a key result showing that it is almost always possible to learn by stable and progressive methods, in which the truth, once in the grasp of a learning method, is never relinquished. That work is grounded in a non-statistical account of information, on which information states decisively refute incompatible possibilities. However, most scientific data is statistical, and in these settings, logical refutation rarely occurs. Critics, including Sober (2015), doubt that the gap between propositional and statistical information can be bridged. In Genin (2018), I answer the skeptics by identifying the unique topology on probability measures whose closed sets are exactly the statistically refutable propositions. I also show that a statistical analogue of progressive learning can also be achieved in the more general setting. That result erects a topological bridge on which insights from learning theory can be ported directly into machine learning, statistics and the data-driven sciences.
Colin Zwanziger - Carnegie Mellon University
SPATIAL MODELS OF HIGHER-ORDER S4
Topological spaces provide a model for propositional S4 modal logic (McKinsey and Tarski 1944) in which the modal operators can be thought of as expressing verifiability and refutability (c.f. Schulte and Juhl 1996, Kelly 1996,...). It is natural to ask: is there a "spatial" notion of model which stands in the same relation to (modal S4) predicate logic as topology does to propositional logic?
Garner (2010) introduced ionads to provide a notion of "higher topological space". The sheaf semantics of Awodey and Kishida (2008) yields a special example of an ionad. A generalization of Garner's ionads is suggested here as a response to our question, in which the "points" will themselves often be mathematical structures (e.g. groups, rings,...), considered together with their isomorphisms. Any such generalized ionad is a model of (classical) higher-order S4 (by application of Zwanziger 2017). Furthermore, to any generalized ionad, we can associate a Grothendieck topos (analogous to the poset of opens of a topological space) that is generated canonically from a theory in the verifiable (geometric) fragment of first-order logic. Thus, generalized ionads may be of interest for applications to verifiability and refutability.
A One-Day PRASI Writing Workshop
October 7, 2018 - 9 am–5 pmScaife Hall 219
Workshop Organizer: Martha Harty, Carnegie Mellon University
Workshop Presenters:
- Mary Adams Trujillo
- Hasshan Batts
- Beth Roy
PRASI is sponsoring an all-day workshop supporting all kinds of people to accomplish all kinds of writing.
The Practitioners Research and Scholarship Institute (PRASI) is a multicultural group of conflict transformation practitioners and writers dedicated to producing literature that reflects the full diversity of our society. Started within the conflict resolution community with the goal of creating a written basis for the profession based in the lived experience of practitioners of many cultural backgrounds, PRASI writing workshops are open to people who desire to write across a broad spectrum of genres: creative, literary, academic, professional.
To register or for information, email Beth Roy.
Workshop on Foundations of Causal Discovery
Center for Formal Epistemology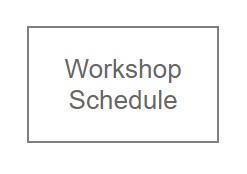
September 22-23, 2018
Baker Hall A53 Steinberg Auditorium
Workshop Organizers: Kevin Kelly and Kun Zhang
Rationale: It is well known that correlation does not directly imply causation. However, patterns of correlation or, more generally, statistical independence, can imply causation, even if the data are non-experimental. Over the past four decades, that insight has given rise to a rich exploration of causal discovery techniques. But there is an important epistemological catch. While it is true that one can sometimes deduce causation from the true independence relations among variables, in practice one must infer those relations from finite samples, and the chance of doing so in error is subject to no a priori bound. Furthermore, such inferences are sometimes based on assumptions that may be questioned, such as that causal pathways never cancel exactly, neutralizing their effects. This workshop brings together experts from philosophy, statistics, and machine learning, to shed fresh light on the special epistemological issues occasioned by causal discovery from non-experimental data.
This workshop is open to the public. For more information, contact the Carnegie Mellon Philosophy Department.
Invited Speakers:
Tom Claassen, Radboud University Nijmegen
Causal discovery from real-world data: relaxing the faithfulness assumption
Abstract: The so-called causal Markov and causal faithfulness assumptions are well-established pillars behind causal discovery from observational data. The first is closely related to the memorylessness property of dynamical systems, and allows us to predict observable conditional independencies in the data from the underlying causal model. The second is the causal equivalent of Ockham’s razor, and enables us to reason backwards from data to the causal model of interest.
A key motivation behind the workshop is the realisation that, though theoretically reasonable, in practice with limited data from real-world systems we often encounter violations of faithfulness. Some of these, like weak long-distance interactions, are handled surprisingly well by benchmark constraint-based algorithms such as FCI. Other violations may imply inconsistencies between observed (conditional) independence statements in the data, and cannot currently be handled effectively by most constraint based algorithms. A fundamental question is whether our output retains any validity when not all our assumptions are satisfied, or whether it is still possible to reliably rescue parts of the model.
In this talk we introduce a novel approach based on a relaxed form of the faithfulness assumption that is able to handle many of the detectable faithfulness violations while ensuring the output causal model remains valid. Effectively we obtain a principled form of error-correction on observed in/dependencies, that can significantly improve both accuracy and reliability of the output causal models in practice. True; it cannot handle all possible violations, but the relaxed faithfulness assumption may be a promising step towards a more realistic, and so more effective, underpinning of the challenging task of causal discovery from real-world systems.
Alexander Gebharter, University of Groningen
Uncovering constitutive relevance relations in mechanisms
Abstract: In this paper I argue that constitutive relevance relations in mechanisms behave like a special kind of causal relation in at least one important respect: Under suitable circumstances constitutive relevance relations produce the Markov factorization. Based on this observation one may wonder whether standard methods for causal discovery could be fruitfully applied to uncover constitutive relevance relations. This paper is intended as a first step into this new area of philosophical research. I investigate to what extent the PC algorithm, originally developed for causal search, can be used for constitutive relevance discovery. I also discuss possible objections and certain limitations of a constitutive relevance discovery procedure based on PC.
Kasey Genin, University of Toronto
Progressive Methods for Causal Search
Constraint-based methods for causal search typically start out by conjecturing sparse graphs with few causal relations and are driven to introduce new causal relationships only when the relevant conditional independencies are statistically refuted. Algorithms such as PC proceed by nesting sequences of conditional independence tests. Although several such methods are known to converge to the true Markov equivalence class in the limit of infinite data, there are infinitely many other methods that would have the same limiting performance, but make drastically different decisions on finite samples. Some of these alternative methods may even reverse the usual preference for sparse graphs for arbitrarily many sample sizes. What, then, justifies the standard methods? Spirtes et al. [2000] note that it cannot be the usual comforts of hypothesis testing since the error probabilities of the nested tests cannot be given their usual interpretation.
I propose a new way of justifying nested-test methods for causal search that provides both a stronger justification than mere point-wise consistency and a new interpretation for the error probabilities of the constituent tests. Say that a point-wise consistent method for investigating a statistical question is progressive if, no matter which answer is correct, the chance that the method outputs the correct answer is strictly increasing with sample size. Progressiveness ensures that collecting a larger sample is a good idea. Although it is often infeasible to construct strictly progressive methods, progressiveness ought to be a regulative ideal. Say that a point-wise consistent method is α-progressive if, for any two sample sizes n1 < n2, the chance of outputting the correct answer does not decrease by more than α. I show that, for all α>0, there exist α-progressive methods for solving the causal search problem. Furthermore, every progressive method must systematically prefer sparser causal models. The methods I construct carefully manage the error probabilities of the nested tests to ensure progressive behavior. That provides a new, non-standard, interpretation for the error probabilities of nested tests.
Hanti Lin, University of California at Davis
How to Tackle an Extremely Hard Learning Problem: Learning Causal Structures from Non-Experimental Data without the Faithfulness Assumption or the Like
Most methods for learning causal structures from non-experimental data rely on some assumptions of simplicity, the most famous of which is known as the Faithfulness condition. Without assuming such conditions to begin with, Jiji Zhang (Lingnan University) and I develop a learning theory for inferring the structure of a causal Bayesian network, and we use the theory to provide a novel justification of a certain assumption of simplicity that is closely related to Faithfulness. Here is the idea. With only the Markov and IID assumptions, causal learning from non-experimental data is notoriously too hard to achieve statistical consistency but we show that (1) it can still achieve a quite desirable ''combined'' mode of stochastic convergence to the truth: having almost sure convergence to the true causal hypothesis with respect to almost all causal Bayesian networks, together with a certain kind of locally uniform convergence. We also show that (2) every learning algorithm achieving at least that joint mode of convergence has this property: having stochastic convergence to the truth with respect to a causal Bayesian network N only if N satisfies a certain variant of Faithfulness, known as Pearl's Minimality condition---as if the learning algorithm were designed by assuming that condition. This new theorem, (1) + (2), explains why it is not merely optional but mandatory to assume the Minimality condition---or to proceed as if we accepted it---when experimental data are not available. I will explain the content of this new theorem, give a pictorial sketch of proof, and defend the philosophical foundation of the underlying approach. In particular, I will argue that it is true to the spirit by which Gold and Putnam created learning theory in the 1960's. And I will argue that the proposed approach can be embraced by many people in epistemology, including reliabilists and---perhaps somewhat surprisingly---even evidentialists and internalists in epistemology.
Karthika Mohan, UC Berkeley
Graphical Models for Missing Data: Recoverability, Testability and Recent Surprises!
The bulk of literature on missing data employs procedures that are data-centric as opposed to process-centric and relies on a set of strong assumptions that are primarily untestable (eg: Missing At Random, Rubin 1976). As a result, this area of research is wanting in tools to encode assumptions about the underlying data generating process, methods to test these assumptions and procedures to decide if queries of interest are estimable and if so to compute their estimands.
We address these deficiencies by using a graphical representation called "Missingness Graph" which portrays the causal mechanisms responsible for missingness. Using this representation, we define the notion of recoverability, i.e., deciding whether there exists a consistent estimator for a given query. We identify graphical conditions (necessary and sufficient) for recovering joint and conditional distributions and present algorithms for detecting these conditions in the missingness graph. Our results apply to missing data problems in all three categories -- MCAR, MAR and NMAR -- the latter is relatively unexplored. We further address the question of testability i.e. whether an assumed model can be subjected to statistical tests, considering the missingness in the data.
Furthermore viewing the missing data problem from a causal perspective has ushered in several surprises. These include recoverability when variables are causes of their own missingness, testability of the MAR assumption, alternatives to iterative procedures such as EM Algorithm and the indispensability of causal assumptions for large sets of missing data problems.
Cosma Shalizi, Carnegie Mellon University
Lessons for causal discovery from Markov models
Causal discovery focuses on learning conditional independence relations in multivariate data. Results on independence testing seem to imply that this is very hard and perhaps unfeasible without strict assumptions. In this talk, I will go over analogies between the causal discovery problem and problems of learning the order and structure of different kinds of Markov models of time series and dynamical systems, which is also all about finding conditional independence relations in data. Here, however, there are a lot of positive results about model-selection consistency, and I will try to explore what insights Markov model discovery might have for causal discovery.
Caroline Uhler, Massachusetts Institute of Technology
From Causal Inference to Gene Regulation
A recent break-through in genomics makes it possible to perform perturbation experiments at a very large scale. The availability of such data motivates the development of a causal inference framework that is based on observational and interventional data. We first characterize the causal relationships that are identifiable from interventional data. In particular, we show that imperfect interventions, which only modify (i.e., without necessarily eliminating) the dependencies between targeted variables and their causes, provide the same causal information as perfect interventions, despite being less invasive. Second, we present the first provably consistent algorithm for learning a causal network from a mix of observational and interventional data. This requires us to develop new results in geometric combinatorics. In particular, we introduce DAG associahedra, a family of polytopes that extend the prominent graph associahedra to the directed setting. We end by discussing applications of this causal inference framework to the estimation of gene regulatory networks.
James F. Woodward, University of Pittsburgh
Flagpoles, Anyone? Independence, Invariance and the Direction of Causation
This talk will explore some recent ideas concerning the directional features of explanation (or causation). I begin by describing an ideal of explanation, loosely motivated by some remarks in Wigner’s Symmetries and Reflections. It turns out (or so I claim) that broadly similar ideas are used in recent work from the machine learning literature to infer causal direction (Janzig et al. 2102).
One such strategy for inferring causal direction makes use of what I call assumptions about variable independence. Wigner’s version of this idea is that when we find dependence or correlation among initial conditions we try to trace this back to further initial conditions or causes that are independent – we assume as a kind of default that it will be possible to find such independent causes. Applied to inferring the direction of causation, a related thought is that if a relationship involves 3 (or more) measured variables X, Y, and Z, or two variables X and Y and an unmeasured noise term U and two of these are independent but no other pairs are independent, then it is often reasonable to infer that the correct causal or explanatory direction is from the independent pair to the third variable.
A second strategy, which makes use of what I call value/ relationship independence, exploits the idea (also found in Wigner) that we expect that the causal or explanatory relationship between two variables (Xà Y,) will be “independent” of the value (s) of the variables that figure in the X or cause position of the relationship Xà Y. Here the relevant notion of independence (which of course is not statistical independence) is linked to a notion of value-invariance described in Woodward (2003): if the Xà Y relationship is causal, we expect that relationship to be invariant under changes in the value of X. According to this strategy for inferring causal direction, if Xà Y is independent of (or invariant under) changes in X and the X—Y relationship in the opposite direction (Y—>X) is not invariant under changes in Y, we should infer, ceteris paribus, that the causal direction runs from X to Y rather than from Y to X.
As said earlier, I take both strategies from the machine learning literature—they are not original with me. But I believe that both strategies have a broader justification in terms of interventionist ideas I have discussed elsewhere as well as methodological ideas like those found in Wigner. I believe it may be useful to put these ideas within this more general context. I will also argue that these ideas lead to a satisfying solution to Hempel’s famous flagpole problem which asks why we think that the length of a flagpole can be used to explain its shadow but not conversely.
References
- Janzing, D., Mooij, J., Zhang, K., Lemeire, J., Zscheischler, J., Daniusis, D., Steudel, B. and Schölkopf, B. (2012) “Information-geometric Approach to Inferring Causal Directions” Artificial Intelligence 182-183: 1-31.
- Wigner, E. (1967) Symmetries and Reflections
- Woodward, J. (2003) Making Things Happen: A Theory of Causal Explanation
Jiji Zhang, Lingnan University
SAT-based causal discovery of semi-Markovian models under weaker assumptions
Abstract: For constraint-based discovery of Markovian causal models (a.k.a causal discovery with causal sufficiency), it has been shown (1) that the assumption of Faithfulness can be weakened in various ways without, in a sense, loss of its epistemological purchase, and (2) weakening of Faithfulness may help to speed up methods based on Boolean satisfiability (SAT) solvers. In this talk, I discuss (1) and (2) regarding causal discovery of semi-Markovian models (a.k.a causal discovery without causal sufficiency). Time permitting, I will also examine the epistemological significance of the fact that unlike Faithfulness, weaker assumptions do not necessarily survive marginalization.
Kun Zhang, Carnegie Mellon University
Causal modeling, statistical independence, and data heterogeneity
Causal discovery aims to reveal the underlying causal model from observational data. Recently various types of independence, including conditional independence between observed variables and independence between causes and noise, have been exploited for this purpose. In this talk I will show how causal discovery and latent variable learning (or concept learning) can greatly benefit from heterogeneity or nonstationarity of the data--data heterogeneity improves the identifiability of the causal model, and even allows us to identify the true causal model in the presence of a large number of hidden variables that are causally related. Finally, I will discuss the implication of the result in machine learning with deep structure.
NASSLLI 2018
June 25-29, 2018We are excited to announce that in June 2018, the Department of Philosophy, with support from across the campus, will host the upcoming North American Summer School in Logic, Language and Information. NASSLLI is a biennial event inaugurated in 2001, which brings together faculty and graduate students from around the world, for a week of interdisciplinary courses on cutting edge topics at the intersection of philosophy, linguistics, computer science and cognitive science. The Summer School aims to promote discussion and interaction between students and faculty in these fields. High level introductory courses allow students in one field to find their way into related work in another field, while other courses focus on areas of active research. With its focus on formalization and on cross-disciplinary interactions, NASSLLI is a natural fit for us here at CMU. We are delighted to be hosting. The summer school will take place June 25-29, 2018, with preparatory events June 23-24.
Information, Causal Models and Model Diagnostics
April 14-15, 2018
Co-sponsored by the Info-Metrics Institute and Dietrich College of Humanities & Social Sciences
The fundamental concepts of information theory are being used for modeling and inference of problems across most disciplines, such as biology, ecology, economics, finance, physics, political sciences and statistics (for examples, see Fall 2014 conference celebrating the fifth anniversary of the Info-Metrics Institute).
The objective of spring 2018 workshop is to study the interconnection between information, information processing, modeling (or model misspecification and diagnostics) and causal inference. In particular, it focuses on modeling and causal inference with an information-theoretic perspective.
Background: Generally speaking, causal inference deals with inferring that A causes B by looking at information concerning the occurrences of both, while probabilistic causation constrains causation in terms of probabilities and conditional probabilities given interventions. In this workshop we are interested in both. We are interested in studying the modeling framework - including the necessary observed and unobserved required information - that allows causal inference. In particular we are interested in studying modeling and causality within the info-metrics - the science of modeling, reasoning, and drawing inferences under conditions of noisy and insufficient information - framework. Unlike the more 'traditional' inference, causal analysis goes a step further: its aim is to infer not only beliefs or probabilities under static conditions, but also the dynamics of beliefs under changing conditions, such as the changes induced by treatments or external interventions.
This workshop will (i) provide a forum for the dissemination of new research in this area and will (ii) stimulate discussion among research from different disciplines. The topics of interest include both, the more philosophical and logical concepts of causal inference and modeling, and the more applied theory of inferring causality from the observed information. We welcome all topics within the intersection of info-metrics, modeling and causal inference, but we encourage new studies on information or information-theoretic inference in conjunction with causality, model specification (and misspecification). These topics may include, but are not limited to:
- Causal Inference and Information
- Probabilistic Causation and Information
- Nonmonotonic Reasoning, Default Logic and Information-Theoretic Methods
- Randomized Experiments and Causal Inference
- Nonrandomized Experiments and Causal Inference
- Modeling, Model Misspecification and Information
- Causal Inference in Network Analysis
- Causal Inference, Instrumental Variables and Information-Theoretic Methods
- Granger Causality and Transfer Entropy
- Counterfactuals, Causality and Policy Analysis in Macroeconomics
PROGRAM COMMITTEE
- Richard Scheines, Co-Chair (CMU)
- Teddy Seidenfeld, Co-Chair (CMU)
- Amos Golan (American University)
CONFIRMED INVITED SPEAKERS AND DISCUSSANTS
- Thomas Augustin (Department of Statistics, University of Munich)
- Gert de Cooman (SYSTeMS Research Group, Ghent University)
-
J. Michael Dunn (Department of Philosophy, Indiana University Bloomington)
- Frederick Eberhardt (Division of Humanities and Social Sciences, Caltech)
- Erik Hoel (Department of Biological Sciences, Columbia University)
- Dominik Janzing (Max Planck Institute for Intelligent Systems)
-
Nicholas (Nick) Kiefer (Cornell)
- David Krakauer (Complexity, Info, Causality; Santa Fe Institute)
- Sarah E. Marzen (MIT Physics of Living Systems)
- Kun Zhang (Department of Philosophy, CMU)
Category Theory Octoberfest
October 28-29, 2017
View slides from Dana Scott’s Talk: What is Explicit Mathematics?
The 2017 Category Theory Octoberfest will be held on the weekend of Saturday, October 28 and Sunday, October 29 at Carnegie Mellon University in Pittsburgh. Following the tradition of past Octoberfests, this is intended to be an informal meeting, covering all areas of category theory and its applications.
Talks by PhD students and young researchers are particularly encouraged!
Details and travel information can be found here:
https://www.andrew.cmu.edu/user/awodey/CToctoberfest/Octoberfest.html
Registration:
There is no registration fee. Registration is optional, but participants are requested to contact the organizers in advance, especially if they would like to give a talk. To register and/or submit a talk, please send email to the organizers with the following information: your name, will you give a talk (yes or no), the title of your talk (if yes).
Organizers:
Modality and Method Workshop
June 9 and 10, 2017 - Center for Formal Epistemology
Margaret Morrison 103
This workshop showcases cutting-edge applications of modality to an intriguing range of methodological issues, including reference, action, causation, information, and the scientific method. Following the tradition of CFE workshops, it is structured to provide ample time for real interaction with, and between, the speakers.
All are welcome to attend.
For more information please email.
Workshop Speakers:
Alexandru Baltag Oxford University |
Title: Knowing Correlations: how to use questions to answer other questions Abstract: Informationally, a question can be encoded as a variable, taking various values ("answers") in different possible worlds. If, in accordance to the recent trend towards an interrogative epistemology, "To know is to know the answer to a question" (Schaffer), then we are lead to paraphrasing the Quinean motto: To know is to know the value of a variable. There are two issues with this assertion. First, questions are never investigated in isolation: we answer questions by reducing them to other questions. This means that the proper object of knowledge is uncovering correlations between questions. To know is to know a functional dependence between variables. Second, when talking about empirical questions/variables, the exact value/answer might not be knowable, and instead only "feasible answers" can be known: this suggests a topology on the space of possible values, in which the open neighborhoods of the actual value represent the feasible answers (knowable approximations of the actual value). A question Q epistemically solves question Q' if every feasible answer to Q' can be known if given some good enough feasible answer to Q. I argue that knowability in such an empirical context amounts to the continuity of the functional correlation. To know is to know a continuous dependence between variables. I investigate a logic of epistemic dependency, that can express knowledge of functional dependencies between (the values of) variables, as well as dynamic modalities for learning new such dependencies. This dynamic captures the widespread view of knowledge acquisition as a process of learning correlations (with the goal of eventually tracking causal relationships in the actual world). There are interesting formal connections with Dependence Logic, Inquisitive Logics, van Benthem's Generalized Semantics for first order logic, Kelly's notion of gradual learnability (as well as the usual learning-theoretic notion of identifiability in the limit), and philosophically with Situation Theory and the conception of "information-as-correlation". |
Adam Bjorndahl Carnegie Mellon University |
Title: Logic and Topology for Knowledge, Knowability, and Belief Abstract: In recent work, Stalnaker (2006) proposes a logical framework in which belief is realized as a weakened form of knowledge. Building on Stalnaker's core insights, and using frameworks developed in (Bjorndahl 2016) and (Baltag et al. 2016), we employ topological tools to refine and, we argue, improve on this analysis. The structure of topological subset spaces allows for a natural distinction between what is known and (roughly speaking) what is knowable; we argue that the foundational axioms of Stalnaker’s system rely intuitively on both of these notions. More precisely, we argue that the plausibility of the principles Stalnaker proposes relating knowledge and belief relies on a subtle equivocation between an "evidence-in-hand" conception of knowledge and a weaker "evidence-out-there" notion of what could come to be known. Our analysis leads to a trimodal logic of knowledge, knowability, and belief interpreted in topological subset spaces in which belief is definable in terms of knowledge and knowability. We provide a sound and complete axiomatization for this logic as well as its uni-modal belief fragment. We also consider weaker logics that preserve suitable translations of Stalnaker's postulates, yet do not allow for any reduction of belief. We propose novel topological semantics for these irreducible notions of belief, generalizing our previous semantics, and provide sound and complete axiomatizations for the corresponding logics. This is joint work with Aybüke Özgün. |
University of Pittsburgh |
Title: Classical Opacity Abstract: In Frege's well-known example, Hesperus was known by the Greeks to rise in the evening, and Phosphorus was not known by the Greeks to rise in the evening, even though Hesperus is Phosphorus. A predicate F such that for some a and b, a=b, Fa and not Fb is said to be opaque. Opaque predicates appear to threaten the classical logic of identity. The responses to this puzzle in the literature either deny that there are cases of opacity in this sense, or deny that one can use classical quantificational logic when opacity is in play. In this paper we motivate and explore the view that there are cases of opacity and that classical quantificational logic is valid even when quantifying in to opaque contexts. We develop the logic of identity given these assumptions in the setting of higher-order logic. We identify a key choice-point for such views, and then develop alternative theories of identity depending on how one makes this choice. In closing, we discuss arguments for each of the two theories. |
Melissa Fusco Columbia University |
Title: Deontic Modality and Classical Logic Abstract: My favored joint solution to the Puzzle of Free Choice Permission (Kamp 1973) and Ross's Paradox (Ross 1941) involves (i) giving up the duality of natural language deontic modals, and (ii) moving to a two-dimensional propositional logic which has a classical Boolean character only as a special case. In this talk, I'd like to highlight two features of this radical view: first, the extent to which Boolean disjunction is imperiled by other natural language phenomena not involving disjunction, and second, the strength of the general position that natural language semantics must treat deontic, epistemic, and circumstantial modals alike. |
Dmitri Gallow University of Pittsburgh |
Title: Learning and Value Change Abstract: Accuracy-first accounts of rational learning attempt to vindicate the intuitive idea that, while rationally-formed belief need not be true, it is nevertheless likely to be true. To this end, they attempt to show that the Bayesian’s rational learning norms are a consequence of the rational pursuit of accuracy. Existing accounts fall short of this goal, for they presuppose evidential norms which are not and cannot be vindicated in terms of the single-minded pursuit of accuracy. They additionally fail to vindicate the Bayesian norm of Jeffrey conditionalization; the responses to uncertain evidence which they do vindicate are not epistemically defensible. I propose an alternative account according to which learning rationalizes changes in the way you value accuracy. I show that this account vindicates the Bayesian’s norm of conditionalization in terms of the single-minded pursuit of accuracy, so long as accuracy is rationally valued. |
Franz Huber |
Title: The Modality underlying Causality Abstract: I will discuss the relationship between extended causal models, which represent two modalities (causal counterfactuals and normality), and counterfactual models, which represent one modality (counterfactuals). It is shown that, under a certain condition, extended causal models that are acyclic can be embedded into counterfactual models. The relevant condition is reminiscent of Lewis (1979) "system of weights or priorities" that governs the similarity relation of causal counterfactuals. In concluding I will sketch modal idealism, a view according to which the causal relationship is a mind-dependent construct. |
Kevin T. Kelly and Konstantin Genin |
Title: What is Statistical Deduction? Abstract: The philosophy of induction begins by drawing a line between deductive and inductive inference. That distinction is clear when empirical information can be modeled as a non-trivial proposition that restricts the range of theoretical possibilities—inference is deductive when every possibility of error is excluded by the premise. Recently, topological methods have been used with success to characterize the boundary between induction and deduction for propositional information of that kind. The basic idea is that that the possible, propositional information states constitute a topological space in which the deductively verifiable propositions are open sets. Then refutable propositions are closed sets, decidable propositions are closed-open, and more general topological concepts characterize the hypotheses that are decidable, verifiable, or refutable in the limit. A new justification of inductive inference emerges thereby—an inductive method is justified insofar as it achieves the best possible sense of success, given the topological complexity of the inference problem faced. That revealing, topological approach to empirical information does not apply directly to statistical inference, because statistical information typically rules out no theoretical possibilities whatever—the sample might just be very unlucky. For that reason, the received view in the philosophy of science has been that all statistical inference is inductive. However, some statistical inferences are evidently very similar to deductive inferences—e.g., rejecting a sharp null hypothesis or generating a confidence interval—whereas others are more similar to inductive inferences—e.g., accepting a sharp null hypothesis or selecting a statistical model. The basis for the analogy is that statistically deductive inferences are ''nearly deductive’’, in the sense that they are performed with a guaranteed low chance of error. The key to connecting the topological-propositional perspective on information with statistics is, therefore, to identify the unique topology for which the propositions that are verifiable with low chance of error are exactly the open propositions. In this talk, we show how to do just that. The result opens the door to a free flow of logical/topological insights into statistical methodology. |
Tamar Lando Columbia University |
Title: Topology and Measure in Logics for Point-Free Space |
Workshop on Exploitation and Coercion
Nov 4-5, 2016 - Center for Ethics & Policy
The Center for Ethics & Policy at Carnegie Mellon University invites paper abstracts for an inaugural Workshop on Ethics and Policy to be hosted November 4-5, 2016 at the CMU campus in Pittsburgh, PA. We are pleased to welcome Richard Arneson as our keynote speaker. In celebration of the 20th Anniversary of the publication of Alan Wertheimer's seminal work Exploitation, the theme for our inaugural workshop is "Exploitation and Coercion".
Attitudes and Questions Workshop
June 10 and 11, 2016 - Center for Formal Epistemology
Question embedding in natural language allows a subject to be related to a question by either a (traditionally) propositional attitude like knowledge and forgetting, or an (apparently) inherently question-oriented predicate like asking or wondering. Attitudes held of questions are an important locus of research into the semantics of both interrogative clauses and clause-embedding verbs, closely connected with the notion of the answerhood conditions of a question, and with the operations of composition involved in combining these types of predicates with semantically heterogeneous arguments. The attitudes that relate us to questions are also of considerable epistemic interest, touching on the nature of the knowledge relation and on the way that questions structure our inquiries. This workshop aims to bring together a diverse group of experts on the semantics and epistemic issues raised by these phenomena, to promote exchange of theoretical perspectives and approaches, and to help to move forward current work on questions and attitudes.
Workshop Speakers:
Harvard University |
Sensitivity to false answers in indirect questions Abstract: |
Duke University |
Reducibility, George's challenge, and Intermediate Readings: In search for an Alternative Explanation Abstract: |
Massachusetts Institute of Technology |
Mention Some, Reconstruction, and Free Choice Abstract: |
École Normale Supérieure, |
Plurality effects and exhaustive readings of embedded questions
|
Institut Jean Nicod & Ecole Normale Supérieure |
Predicting the presuppositions triggered by responsive predicates Abstract: |
Carnegie Mellon University |
Simplicity and Scientific Questions Abstract: Ockham’s razor instructs the scientist to favor the simplest theory compatible with current information. There is a broad consensus that simplicity is a principal consideration guiding inductive inference in science. But that familiar observation raises several subtle questions. When is one theory simpler than another? And why should one prefer simpler theories if there is no guarantee that simpler theories are — in some objective sense — more likely to be true? We present a model of empirical inquiry in which simplicity relates answers to an empirical question, and is grounded in the underlying information topology, the topological space generated by the set of possible information states inquiry might encounter. We show that preferring simple theories is a necessary condition for optimally direct convergence to the truth, where directness consists in avoiding unnecessary cycles of opinion on the way to the truth. Our approach relates to linguistics in two ways. First, it illustrates how questions under discussion can shape simplicity and, hence, the course of theoretical science. Second, it explains how, and in what sense, empirical simplicity can serve as a theoretical guide in empirical linguistics. |
Carnegie Mellon University |
The False Belief Effect for know wh and its Conceptual Neighbors Abstract: |
Harvard University |
"Differentiating Contents" CFE/Linguistics Workshop
Saturday, December 5, 2015 - Carnegie Mellon, Baker Hall, Dean’s Conference Room, 154R
A variety of phenomena have motivated researchers to distinguish between different types of linguistic content. One classical distinction is that made by Austin (1962) and Searle (1969) between the propositional content of utterances and their speech act force. Another classical distinction is that between assertoric and presupposed content (Frege 1893, Strawson 1950, Stalnaker 1974, inter alia). In recent years, a new distinction between at-issue and not at-issue content (Potts 2005, Simons et al. 2010) has been introduced, to some extent offered as a replacement for the asserted/presupposed distinction. One empirical domain where the at-issue/not at-issue distinction has been utilized by some researchers is in the study of evidentials, a category of linguistic forms which provide information about the speaker’s evidential relation to the (remaining) content of her utterance.
This one day workshop will bring together researchers with intersecting work on the nature of these distinctions, on the empirical evidence for them, and on how to model them.
Fifteenth conference on Theoretical Aspects of Rationality and Knowledge (TARK 2015)
Co-sponsored by the Center for Formal Epistemology
June 4-6, 2015 - Carnegie Mellon
Pitt/CMU Graduate Student Conference
March 20-21, 2015 - Carnegie Mellon
Locations: Mellon Institute, Room 348 (March 20) and Margaret Morrison, Room A14 (March 21)
Workshop on Simplicity and Causal Discovery
Co-sponsored by the Center for Formal Epistemology
June 6-8, 2014 - Carnegie Mellon
Modal Logic Workshop: Consistency and Structure
Co-sponsored by the Center for Formal Epistemology
Saturday, April 12, 2014 - Carnegie Mellon
Trimester: Semantics of Proofs and Certified Mathematics Trimester at the Institut Henri Poincare
April 7 - July 11, 2014 - Paris, France
Workshop: Philosophy of Physics
September 7, 2013
With Hans Halvorson (Princeton University) and James Weatherall (UC Irvine)
Conference: Type Theory, Homotopy Theory, and Univalent Foundations
September 23-27, 2013 - Barcelona, Spain
Workshop: Case Studies of Causal Discovery with Model Search
October 25-27, 2013 - Carnegie Mellon