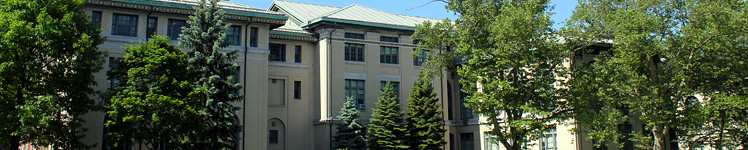
Computational Epistemology
David Hume begins his Enquiry Concerning Human Understanding with a sharp dichotomy between "relations of ideas" and "matters of fact". Relations of ideas are necessary and can be decided a priori in finite time with demonstrative certainty. Matters of fact concern unbounded future experience a posteriori and can never be certain. Relations of ideas concern mathematics and logic. Matters of fact succumb only to the methods of natural science. This momentous first distinction is still central to contemporary philosophy, in spite of the celebrated demise of the analytic/synthetic distinction. Philosophy of mathematics is where one expects to hear about algorithms, undecidability, and incompleteness, whereas the philosophy of science concerns "rationality", "confirmation", and "probability".
According to computational epistemology, the entire, standard picture just described is a tragic mistake founded on a spurious distinction. For there exist both decidable and undecidable formal questions just as there exist decidable and undecidable empirical questions. And in both the formal and empirical domains there are also undecidable problems in which the answer is verifiable or refutable. And in both the formal and empirical domains there are problems that are neither verifiable nor refutable but in which it is possible to converge to the right answer. The first cut in philosophy should have been between these successively weaker notions of solvability rather than between the a priori and the a posteriori. For think about it: the formally undecidable "halting problem" of computability theory asks of an arbitrary computation if it will never halt. Hume's problem of induction asks whether bread will never cease to nourish. Both hypotheses are refutable but not verifiable and are universal with respect to concrete experimental outcomes. It is just that in the former case the experiments can be performed a priori (by a "mental simulation" of the computation step-by-step) and in the latter they cannot be (one must consult the "external" world). The similarities between the two cases outweigh the differences. For the difference amounts to this: the empirically undecidable problem of induction is like sucking in an infinite noodle that you'll never eat entirely, whereas the formally undecidable halting problem is like sucking on a jawbreaker that will never dissolve entirely even though it fits completely in your mouth. The fundamental issue is failure to completely "digest" one's input, and that is essentially the same in both cases. So where philosophy draws its central distinction, there is actually a strong, point-by-point analogy between formal and empirical truth-finding. Exploration of that analogy is what computational epistemology is really all about. It is no mere technical exercise. It is a new, unified paradigm for the epistemology of the formal and the empirical that should be familiar to every serious epistemologist.
Computational learning theory looks at scientific questions the way a computer scientist looks at formal questions. The focus is not on "support", "confirmation", "coherence", "rational degrees of belief" or "historical case studies", for if these things are not conducive to efficient truth-finding, why bother with them? Instead, the idea is to cut straight to the chase and to seek the most efficient possible truth-finding methods.
Kelly is the leading philosopher involved in computational learning theory and its application to traditional puzzles in epistemology and the philosophy of science. He has most recently constructed the first non-circular explanation of how Ockham's razor, the principle that one should prefer simple theories, facilitates finding theoretical truth. This work involves a general, mathematical definition of theoretical simplicity that provides a new and more comprehensive understanding of the nature of this elusive concept. Kelly has also investigated:
- the learning power of "belief revision methods"
- infinite empirical regresses
- (with former Logic and Computation Ph.D. student Oliver Schulte) the effective learnability of theories whose predictions cannot be derived by effective means
- the extent to which "rationality" prevents effective agents from finding the truth
- the extensive analogies between uncomputability and the problem of induction (also examined by Sieg in his work on Turing's thesis)
- the structural necessary and sufficient conditions for the existence of methods for verification, refutation, convergence to the truth, etc
- (with C. Glymour) relativistic learning theory, in which the truth depends in part on the agent
Glymour has applied the learning theoretic framework to characterize hypotheses about "cognitive architecture" that can be learned from observing combinations of cognitive deficits in people with brain injuries. He is now extending the analysis to resolve a longstanding debate in cognitive neuropsychology over the value of studies that compare aggregate features of distinct groups versus studies that collect records of individual deficits.
Also, much of Glymour's earlier work is unified by the theme of Ockham's razor in scientific practice. In particular, his extensive work on causal inference (with Spirtes and Scheines) is motivated by simplicity intuitions that can be explicated in terms of the analysis of Ockham's razor described above.
Also relevant to this general topic is Seidenfeld's ground-breaking work on convergence and non-convergence of opinion of multiple Bayesian agents.