Upcoming Events
April 3, 2025 | 4:00pm–6:00pm | Posner A35
Morris H. DeGroot Memorial Lecture
Edward Kennedy
Carnegie Mellon University
Talk Title: Minimax optimality in causal inference
Abstract: In this talk I will survey some recent work on minimax optimality in causal inference problems. We consider minimax optimality in smooth, structure-agnostic, and combined models, for both average and heterogeneous/conditional causal effects. In smooth models, higher-order influence function-based methods can yield optimal rates, which roughly resemble optimal rates for simpler quadratic functionals in smooth models (depending on assumptions about the covariate distribution). In structure-agnostic models, simpler doubly robust estimators are optimal. For particular combined models, the optimal rate is in between, and requires non-trivial bias correction involving regressions on estimated nuisance functions. For heterogeneous causal effects, minimax optimal rates interpolate between rates for functional estimation and nonparametric regression / density estimation, illustrating how these effects behave as a regression/functional hybrid.
April 4, 2025 | 12:00pm–2:00pm | Baker Hall Patio (overlooking Frew St.)
Carnival Alumni Luncheon
You are invited to join the Department of Statistics & Data Science current faculty and staff for a lunch and opportunity to reconnect! This event is open to Stat & DS alumni, graduate students, faculty and staff.
We hope to see you there!
Schedule of all Carnival events
April 7, 2025 | 4:00–5:00pm | BH A53 (Steinberg)
Rafael Frongillo
University of Colorado Boulder
Talk Title: Fantastic Surrogate Loss Functions and Where to Find Them
Abstract: Loss functions are used in many contexts throughout statistics and machine learning, primarily to train models from data and to evaluate existing models. But which loss function should one choose? It turns out that every choice of loss function corresponds to a particular statistic of the underlying conditional label distribution, the one that the loss "elicits". Minimizing the loss over the data will pull the model toward this statistic. For example, in ordinary least-squares regression, this statistic is the conditional mean. To design fantastic (statistically consistent) loss functions, therefore, we must understand which losses elicit which statistics. Unfortunately, some important statistics are not elicited by any loss function, or the only loss functions are highly discontinuous and not suitable for training. For this reason, we need to take a step further and design *surrogate* loss functions, which is where our journey begins. The talk will give an overview of loss function design, and introduce new techniques to design surrogate loss functions for both continuous and discrete prediction tasks. If time permits we will conclude with a few variations, such as loss functions to evaluate generative models or to learn from quantum data.
Bio: Rafael (Raf) Frongillo is an Associate Professor of Computer Science at the University of Colorado Boulder. His research lies at the interface between theoretical machine learning and economics, primarily focusing on information elicitation mechanisms, which incentivize humans or algorithms to predict accurately. Before Boulder, Raf was a postdoc at the Center for Research on Computation and Society at Harvard University and at Microsoft Research New York. He received his Ph.D. in Computer Science at UC Berkeley, advised by Christos Papadimitriou and supported by the NDSEG Fellowship.
April 14, 2025 | 4:00–5:00pm | BH A53 (Steinberg)
Alex Tartakovsky
AGT StatConsult, Los Angeles, California USA
Talk Title: Optimal Change Detection and Identification for General Stochastic Models: Recent Results and Future Challenges
Abstract: Changepoint problems deal with detecting changes in a process that occur at unknown points in time or space. A conventional approach to the sequential changepoint detection problem is to design a detection rule that minimizes the expected detection delay of a real change subject to a bound on the false alarm rate. However, in many applications, there are multiple data streams or channels/sensors, and it is also necessary to identify a subset of affected streams where the change occurs. In this case, there are multiple post-change hypotheses that have to be tested (isolated). In other words, the problem reduces to joint detection and identification and the adequate optimality criterion is to minimize the average delay to detection-identification under all possible hypotheses and all change points subject to the constraint imposed on the false alarm and false identification risks. In this talk, we will discuss minimax and pointwise problems for general statistical models that include dependent and nonidentically distributed observations. The developed general theory allows us to design nearly optimal change detection-identification rules when the probabilities of false alarm and misidentification are small. We will also discuss optimal reliable change detection approaches that are targeted to minimizing probabilities of errors in fixed time windows under a given false alarm rate. We then apply the developed change detection rules to the problems of rapid detection and localization of epidemics, including COVID-19; detection and estimation of traces of dim space objects with unknown orbits with telescopes; and track initiation in object tracking systems. The results show that the developed algorithm allowed for the detection-identification of the region with an outbreak of the COVID several days prior to the imposition of quarantine protocols.
Bio: After earning his Ph.D. in 1981, Dr. Tartakovsky served as a Senior Research Scientist and later as Department Head at the A.L. Mints Institute of Radio Technology (Russian Academy of Sciences). He also held a professorship at the Moscow Institute of Physics and Technology (PhysTech). Since 2015, he has been the president of AGT StatConsult in Los Angeles, CA. From 2017 to 2022, he led the Space Informatics Laboratory at PhysTech. Prior to this, he was a Professor in the Department of Statistics at the University of Connecticut (2013-2016) and a Professor of Mathematics and Associate Director of the Center for Applied Mathematical Sciences at the University of Southern California (1997-2013).
He is an expert in theoretical and applied statistics, applied probability, sequential analysis, and changepoint detection. His research spans applications in statistical image and signal processing, video surveillance, object detection and tracking, information fusion, network security, detection and tracking of malicious activities, pharmacokinetics/pharmacodynamics, and early epidemic detection using sequential hypothesis testing and changepoint methods. He is the author of three books, several book chapters, and over 150 peer-reviewed articles. He is a Fellow of the Institute of Mathematical Statistics and a senior member of IEEE. Among his several awards is the 2007 Abraham Wald Prize in Sequential Analysis.
April 21, 2025 | 4:00–5:00pm | BH A53 (Steinberg)
Ivan Diaz
NYU Grossman School of Medicine
Talk Title: TBD
Abstract: TBD
April 25, 2025 | 1:30–2:30pm | BH A53 (Steinberg)
Joel Leja
The Pennsylvania State University
Talk Title: TBD
Abstract: TBD
May 9, 2025 | 10:00am–12:30pm | Chosky Theater
Graduate Diploma Ceremony & Reception
May 10, 2025 | 4:00–5:00pm | Weigand Gym
Undergraduate Diploma Ceremony
May 10, 2025 | 5:00–6:30pm | P/W/McKenna Room, UC
Undergraduate Reception
Past Events
March 17, 2025
Tracy Ke
Harvard University
Talk Title: A Statistically Provable Approach to Integrating LLMs into Topic Modeling
Abstract: The rise of large language models (LLMs) raises an important question: how can statisticians leverage their expertise in the AI era? Statisticians excel in developing resource-efficient, theoretically grounded models. In this talk, we use topic modeling as an example to illustrate how such expertise can enhance the processing of LLM-generated data.
Traditional topic modeling is applied to word counts without considering contextual meaning. LLMs, however, produce contextualized word embeddings that capture deeper semantic relationships. We leverage these embeddings to refine topic modeling by representing each document as a sequence of word embeddings, modeled as a Poisson point process. Its intensity measure is expressed as a convex combination of K base measures, each representing a topic. To estimate these topics, we propose a flexible algorithm that integrates traditional topic modeling methods, incorporating net-rounding before and kernel smoothing after. A key advantage of this approach is its compatibility with any existing bag-of-words topic modeling method as a plug-in module, requiring no modifications.
Assuming each topic is a beta Hölder smooth intensity measure in the embedded space, we establish the convergence rate of our method. We also derive a minimax lower bound and show that our method attains this rate when beta is in a certain range. Finally, we validate our approach on multiple datasets, demonstrating its advantages over traditional topic modeling techniques in capturing word contexts.
March 13-14, 2025 | Carnegie Mellon University
WiDS Pittsburgh @ CMU: Bringing Together the Region’s Data Science Community
We are excited to welcome you to WiDS Pittsburgh @ CMU, returning in March 2025 as a premier gathering for data scientists across the Pittsburgh region. This event brings together students, researchers, industry professionals, and organizations to explore the latest advancements in data science, foster connections, and highlight impactful work happening in our community. WiDS Pittsburgh @ CMU is part of the global WiDS initiative, which originated at Stanford University and has grown to include more than 150 satellite events worldwide.
This event is open to anyone who is interested in data science and its transformative potential. Students and junior professionals are also welcome to share their resumes with WiDS partners and sponsors.
Join us to celebrate data science's power to drive discovery and innovation across Pittsburgh and beyond.
February 26, 2025
Sabina Sloman
University of Manchester
Talk Title: Confronting scientific uncertainty: Bayesian experimental design and inference with limited prior knowledge
Abstract: The goal of many scientists is to resolve uncertainty about some aspect of a target system, while the nature of other aspects of the system is both unknown and subject to change. Accomplishing this goal requires identifying which observations would be the most informative (experimental design) and learning from actual observations of the system (inference). Many scientific disciplines rely on Bayesian methods for experimental design and inference. However, these methods are often not compatible with scientists’ uncertainty about other aspects of the target system and the possibility of inaccurate prior knowledge (misspecification). In this talk, I will present work showing the effect of misspecification on the design of experiments (Bayesian experimental design) and inference when conditions are subject to change (Bayesian transfer learning). In the context of Bayesian experimental design, I will show that learning under possible misspecification requires rethinking commonly-used objective functions. In the context of Bayesian transfer learning, I will apply these results to the anticipation and mitigation of the phenomenon of “negative transfer”. I will conclude by presenting a bird’s-eye view on the evolving relationship between statistical models and scientific objectives, in particular the impact of highly expressive machine learning models on scientific models and modeling practice.
Bio: I am a postdoc in the Department of Computer Science at the University of Manchester, supervised by Dr. Samuel Kaski. I am a member of the Manchester Centre for AI Fundamentals and the ELLIS Society. Much of my research is done in collaboration with the Finnish Center for Artificial Intelligence. I received my Ph.D. in Social and Decision Sciences (concentration in Cognitive Decision Sciences) in 2022 from Carnegie Mellon University, where I was advised by Dr. Daniel Oppenheimer. My dissertation investigated the robustness of Bayesian experimental design to misspecification.
February 24, 2025
John Cherian
Stanford University
Talk Title: Statistical methods for assessing the factual accuracy of large language models
Abstract: The deployment of machine learning in high-stakes settings has raised fundamental questions about the reliability and fairness of black-box models. For example, does a model treat different groups equitably, or can we quantify model uncertainty before taking action on each prediction? While numerous assumption-lean methods appear to address these types of questions, their guarantees can often be misaligned with practitioners’ needs. My research program aims to resolve the inherent tension of model-free statistical inference: the generic validity of such methods is appealing, but without a well-specified model, it is challenging to identify guarantees that are also useful for decision-making.
To illustrate my approach, this talk will primarily focus on a set of new conformal inference methods for obtaining validity guarantees on the output of large language models (LLMs). Prior work in language modeling identifies a subset of the text that satisfies a high-probability guarantee of factuality. These methods work by filtering a claim from the LLM’s original response if a scoring function evaluated on the claim fails to exceed some estimated threshold. Existing methods in this area suffer from two deficiencies. First, the guarantee is not conditionally valid. The trustworthiness of the filtering step may vary based on the topic of the response. Second, because the scoring function is imperfect, the filtering step can remove many valuable and accurate claims. Our work addresses both of these challenges via two new conformal prediction methods. First, we show how to issue an error guarantee that is both valid and adaptive: the guarantee remains well-calibrated even though it can depend on the prompt (e.g., so that the final output retains most claims). Second, we will show how to optimize the accuracy of the scoring function used in this procedure, e.g., by ensembling multiple scoring approaches. This is joint work with Isaac Gibbs and Emmanuel Candès.
Bio: John Cherian is a 5th year Ph.D. student at Stanford University supported by the Hertz Foundation. Advised by Emmanuel Candès, he works on problems in model-free inference and uncertainty quantification. He also consults for The Washington Post, where he applies this research to night-of election models. Prior to the Ph.D., John spent three years at D.E. Shaw Research improving molecular dynamics simulations for structural biology and drug discovery.
February 19, 2025
Ang Yu
University of Wisconsin-Madison
Talk Title: Nonparametric Causal Decomposition of Group Disparities
Abstract: We introduce a new nonparametric causal decomposition approach that identifies the mechanisms by which a treatment variable contributes to a group-based outcome disparity. Our approach distinguishes three mechanisms: group differences in 1) treatment prevalence, 2) average treatment effects, and 3) selection into treatment based on individual-level treatment effects. Our approach reformulates classic Kitagawa-Blinder-Oaxaca decompositions in causal and nonparametric terms, complements causal mediation analysis by explaining group disparities instead of group effects, and isolates conceptually distinct mechanisms conflated in recent random equalization decompositions. In contrast to all prior approaches, our framework uniquely identifies differential selection into treatment as a novel disparity-generating mechanism. Our approach can be used for both the retrospective causal explanation of disparities and the prospective planning of interventions to change disparities. We present both an unconditional and a conditional decomposition, where the latter quantifies the contributions of the treatment within levels of certain covariates. We develop nonparametric estimators that are root-n consistent, asymptotically normal, semiparametrically efficient, and multiply robust. We apply our approach to analyze the mechanisms by which college graduation causally contributes to intergenerational income persistence (the disparity in adult income between the children of high- vs low-income parents). Empirically, we demonstrate a previously undiscovered role played by the new selection component in intergenerational income persistence.
Bio: Ang Yu is a Ph.D. candidate in sociology at the University of Wisconsin-Madison and holds a master’s degree in statistics from the same university. His methodological interests lie in causal inference, particularly mediation/longitudinal estimands and causal machine learning. His substantive areas of research include social stratification, sociology of education, and health disparities.
February 17, 2025
Zackary Dunivin
Indiana University
Talk Title: Beyond NLP: A computational mixed-methods approach to social complexity
Abstract: This job talk presents two studies showcasing how a combination of computational methods, crowdsourced text, creative statistical analysis, and close reading can gain purchase on complex social phenomena. Zackary will report results from "Black Lives Matter protests shift public discourse" (PNAS 2022), which uses multisource crowdsourced data to show how BLM leveraged street protests to change the ways we talk about race and politics. He will also present analyses from “Moving beyond bias mitigation to bias negotiation: Leveraging sociocultural representations in LLMs for transformative social AI” (unpublished). Through a combination of LLM-as-classifier analysis and ethnography of “interviews” with LLMs, he argues that sociocultural schemas, including stereotypes, should be privileged, rather than purged from LLMs. Sophisticated conceptions of social identity and structural power dynamics combined with ethical alignment and the ability reasoning from multiple perspectives can enable social AI to confront entrenched inequalities and strive toward more informed, compassionate, and just collective futures.
Bio: Zackary Dunivin received his doctorate in Complex Systems and Sociology from Indiana University in 2024. His work centers computational tools, frequently supported by statistical methods, to enable qualitative and quasi-qualitative analyses of cultural phenomena. His research has involved NLP, agent-based modeling, time series analysis, Bayesian modeling, and ethnography, and engaged such substantive areas as social movements, social identity, cognitive science, race and gender studies, and media studies. Presently a post-doctoral fellow at UC Davis, Zackary’s research has been published in PNAS, Psychological Review, and the Journal of Medical Internet Research. Recently he has been studying open-source organizations and social aspects of LLMs. Future research plans involve continued work on computational approaches to culture and qualitative research, the use of LLMs as tools for social science, the role of AI in society, and organizations as a complex system.
February 10, 2025
Tijana Zrnic
Stanford University
Talk Title: AI-Assisted Approaches to Data Collection and Inference
Abstract: Recent breakthroughs in AI offer tremendous potential to reduce the costs of data collection. For example, there is a growing interest in leveraging large language models (LLMs) as efficient substitutes for human judgment in tasks such as model evaluation and survey research. However, AI systems are not without flaws—generative language models often lack factual accuracy, and predictive models remain vulnerable to subtle perturbations. These issues are particularly concerning when critical decisions, such as scientific discoveries or policy choices, rely on AI-generated outputs. In this talk, I will present recent and ongoing work on AI-assisted approaches to data collection and statistical inference. Rather than treating AI as a replacement for data collection, our methods leverage AI to strategically guide data collection and improve the power of subsequent inferences, all the while retaining provable validity guarantees. I will demonstrate the benefits of this methodology through examples from computational social science, proteomics, and more.
Bio: Tijana Zrnic is a Ram and Vijay Shriram Postdoctoral Fellow at Stanford University, affiliated with Stanford Data Science and the Department of Statistics. Tijana obtained her Ph.D. in Electrical Engineering and Computer Sciences at UC Berkeley and a BEng in Electrical and Computer Engineering at the University of Novi Sad in Serbia. Her research establishes foundations to ensure data-driven technologies have a positive impact; she has worked on topics such as AI-assisted statistical inference, performative prediction, and mitigating selection bias.
February 5, 2025
Anastasios Angelopoulos
UC Berkeley
Talk Title: Statistical Foundations of Trustworthy AI Engineering
Abstract: My research has been almost entirely devoted to a single question: How can we build trustworthy systems from untrustworthy AI algorithms? Answering this question is difficult because modern AI models can be wrong in unpredictable ways. From data, these models learn biases, spurious associations, and imperfect world-models that are difficult to debug due to their statistical nature. But to use AI in critical applications---from legal and financial institutions to power plants to hospitals, where safety, and lives are at stake---we need trust. Part of what holds us back is a lack of formally grounded but practical statistical methodology for ensuring that we are able to use AI reliably, even when the underlying model may have flaws.The talk will have two halves. In the first half, I will discuss conformal risk control, a statistical framework for reliable decision-making using black-box models. In the second half, I will discuss AI evaluations for aligning AI with human preferences and safety. I will focus both on the foundational statistical methodology underlying these techniques and also the large-scale deployments that have resulted, and the opportunities for future research that arise.
Bio: Anastasios Nikolas Angelopoulos is a sixth-year Ph.D. student at the University of California, Berkeley. Previously, he obtained a B.S. in Electrical Engineering at Stanford University. His research concerns statistical infrastructure for reliable and safe deployment of AI, including conformal prediction, prediction-powered inference, and the development of Chatbot Arena, an open-source platform measuring the alignment of AI to human preferences.
February 3, 2025
Aishik Ghosh
UC Irvine
Talk Title: A high-dimensional paradigm of frequentist statistics in particle physics
Abstract: Particle physics research relies on making statistical statements about Nature. When quantum effects and virtual particles are the norm, it may not even make sense to talk about events; rather, only their probabilities. In this talk, I will motivate the need for high-dimensional non-parametric statistics in particle physics to facilitate new discoveries within the lifetime of existing experiments. I will then discuss my six-year research program that led to the design of the first statistical method capable of being applied to real-world particle physics problems. This method incorporates uncertainty quantification to create a robust test statistic, implements Neyman inversion for valid confidence intervals, and develops visualization tools to interpret what the neural networks are learning in high dimensions and diagnose potential mismodeling. We apply this technique to a flagship Higgs measurement at the Large Hadron Collider, achieving a 260% improvement in our ability to reject the null hypothesis. This technique is now being integrated into open-source statistics software and deployed on DOE supercomputers as a service, with the potential for use in numerous frequentist analyses across particle physics experiments.
I will also discuss upcoming and future work that combines Bayesian and frequentist tools to address certain challenges in testing non-nested hypotheses and develops physics-informed priors to improve the sensitivity of existing statistical methods, including a collaboration with my colleagues at CMU. Finally, I will explore the connections between my uncertainty quantification work and algorithmic bias that affects society, as well as how I have contributed to public policy to mitigate these risks.
Bio: Dr. Aishik Ghosh is a postdoctoral scholar at UC Irvine and an affiliate at Berkeley Lab, focusing on the development of statistical techniques and uncertainty quantification tools for the physical sciences. These including applications in particle physics, astrophysics, and, more recently, inverse problems in climate modeling. He has collaborated with the Organization for Economic Cooperation and Development (OECD) on topics such as trustworthy AI and science policy. Dr. Ghosh is interested in algorithmic fairness and has published work on the dangers of misquantified biases in AI algorithms. He obtained his PhD from the University of Paris-Saclay in France.
January 29, 2025
Ankit Pensia
UC Berkeley
Talk Title: Modern Algorithmic Statistics: Reliability with Minimal Resources
Abstract: Modern data science pipelines are often severely constrained in resources, both statistical (e.g., poor-quality input data due to outliers) as well as computational (e.g., limited runtime or memory). Simultaneously adapting to these constraints necessitates new algorithmic solutions for even basic statistical tasks. In this talk, I will present two such results in the field of high-dimensional statistics.First, I will discuss parameter estimation for sub-Gaussian data in the presence of arbitrary outliers. For many important problems in this class, existing algorithms were either robust or polynomial-time, but not both. We resolve this issue by providing the first polynomial-time robust algorithms for covariance estimation, linear regression, and covariance-aware mean estimation. Our results are obtained via new structural results about semidefinite relaxations. Next, I will discuss the problem of robust sparse mean estimation. Moving beyond polynomial runtime as the benchmark, I will show how to bridge the gap, in fine-grained runtime, between robust and non-robust algorithms. I will conclude with connections to other notions of resource constraints, such as privacy and communication budget.
Bio: Ankit Pensia is a research fellow at the Simons Institute for the Theory of Computing at UC Berkeley. Previously, he was a Herman Goldstine Postdoctoral fellow at IBM Research. He obtained his PhD in Computer Science at the University of Wisconsin-Madison under the supervision of Po-Ling Loh, Varun Jog, and Ilias Diakonikolas. His current research interests include algorithmic robust statistics, high-dimensional probability, decentralized detection, and differential privacy.
January 27, 2025
Yeshwanth Cherapanamjeri
MIT
Talk Title: Statistical Challenges in Modern Machine Learning and their Algorithmic Consequences
Abstract: The success of modern machine learning is driven, in part, by the availability of large-scale datasets. However, their immense scale also makes the effective curation of such datasets challenging. Many classical estimators, developed under the assumption of clean, well-behaved data, fare poorly when deployed in these settings. This unfortunate scenario raises several statistical as well as algorithmic challenges: What are the statistical limits of estimation in these settings and can they be realized computationally efficiently? In this talk, I will compare and contrast the task of addressing these challenges in two natural, complementary settings: the first featuring extreme noise and the second, extreme bias. In the first setting, we consider the problem of estimation with heavy-tailed data where recent work has produced estimators achieving optimal statistical performance. However, these solutions are computationally impractical and their analysis, tailored to the specific problem of interest. I will present a simple algorithmic framework that has resulted in state-of-the-art estimators for a broad class of heavy-tailed estimation problems. Next, I consider the complementary setting of extreme bias under the classical Roy model of self-selection bias where bias arises due to the strategic behavior of the data-generating agents. I will describe algorithmic approaches to counteract this bias, yielding the first statistically and computationally efficient estimators in this setting. Finally, I will conclude the talk with future directions targeting the construction of good datasets when the data is drawn from a diverse and heterogeneous range of sources with varying quality and quantity.
Bio: Yeshwanth is a postdoctoral researcher at MIT where he is mentored by Constantinos Daskalakis. Previously, he completed his Ph.D at UC Berkeley under the guidance of Peter Bartlett. Yeshwanth is interested in statistical and algorithmic challenges that arise in the modern practice of machine learning. These include settings with extreme amounts of noise or bias, missing or partially observed data, and more recently, the impact of dataset construction on statistical performance.
January 22, 2025
Yuchen Hu
Stanford University
Talk Title: Policy Evaluation in Dynamic Experiments
Abstract: Experiments where treatment assignment varies over time, such as micro-randomized trials and switchback experiments, are essential for guiding dynamic decisions. These experiments often exhibit nonstationarity due to factors like hidden states or unstable environments, posing substantial challenges for accurate policy evaluation. In this talk, I will discuss how Partially Observed Markov Decision Processes (POMDPs) with explicit mixing assumptions provide a natural framework for modeling dynamic experiments and can guide both the design and analysis of these experiments. In the first part of the talk, I will discuss properties of switchback experiments in finite-population, nonstationary dynamic systems. We find that, in this setting, standard switchback designs suffer considerably from carryover bias, but judicious use of burn-in periods can considerably improve the situation and enable errors that decay nearly at the parametric rate. In the second part of the talk, I will discuss policy evaluation in micro-randomized experiments and provide further theoretical grounding on mixing-based policy evaluation methodologies. Under a sequential ignorability assumption, we provide rate-matching upper and lower bounds that sharply characterize the hardness of off-policy evaluation in POMDPs. These findings demonstrate the promise of using stochastic modeling techniques to enhance tools for causal inference. Our formal results are mirrored in empirical evaluations using ride-sharing and mobile health simulators.
Bio: Yuchen Hu is a Ph.D. candidate in Management Science and Engineering at Stanford University, under the supervision of Professor Stefan Wager. Her research focuses on causal inference, data-driven decision making, and stochastic processes. She is particularly interested in developing interdisciplinary statistical methodologies that enhance the applicability, robustness, and efficiency of data-driven decisions in complex environments. Hu holds an M.S. in Biostatistics from Harvard University and a B.Sc. in Applied Mathematics from Hong Kong Polytechnic University.
January 15, 2025
Yuchen Wu
Wharton School, University of Pennsylvania
Talk Title: Modern Sampling Paradigms: from Posterior Sampling to Generative AI
Abstract: Sampling from a target distribution is a recurring theme in statistics and generative artificial intelligence (AI). In statistics, posterior sampling offers a flexible inferential framework, enabling uncertainty quantification, probabilistic prediction, as well as the estimation of intractable quantities. In generative AI, sampling aims to generate unseen instances that emulate a target population, such as the natural distributions of texts, images, and molecules. In this talk, I will present my works on designing provably efficient sampling algorithms, addressing challenges in both statistics and generative AI. (1) In the first part, I will focus on posterior sampling for Bayes sparse regression. In general, such posteriors are high-dimensional and contain many modes, making them challenging to sample from. To address this, we develop a novel sampling algorithm based on decomposing the target posterior into a log-concave mixture of simple distributions, reducing sampling from a complex distribution to sampling from a tractable log-concave one. We establish provable guarantees for our method in a challenging regime that was previously intractable. (2) In the second part, I will describe a training-free acceleration method for diffusion models, which are deep generative models that underpin cutting-edge applications such as AlphaFold, DALL-E and Sora. Our approach is simple to implement, wraps around any pre-trained diffusion model, and comes with a provable convergence rate that strengthens prior theoretical results. We demonstrate the effectiveness of our method on several real-world image generation tasks. Lastly, I will outline my vision for bridging the fields of statistics and generative AI, exploring how insights from one domain can drive progress in the other.
Bio: Yuchen Wu is a departmental postdoctoral researcher in the Department of Statistics and Data Science at the Wharton School, University of Pennsylvania. She earned her Ph.D. in 2023 from Stanford University, where she was advised by Professor Andrea Montanari. Her research lies broadly at the intersection of statistics and machine learning, featuring generative AI, high-dimensional statistics, Bayesian inference, algorithm design, and data-driven decision making.
January 13, 2025
Jake Soloff
University of Chicago
Talk Title: Off-the-shelf algorithmic stability
Abstract: Algorithmic stability holds when model fitting is insensitive to small changes in the training data. It is often seen as a means to assumption-lean inference, since it has important implications for generalization, predictive inference, and other statistical problems, without requiring distributional assumptions on the data. To reap these benefits, we should not leave stability as yet-another questionable assumption, but we also should not restrict ourselves to using a handful of specific, mathematically tractable algorithms that have been shown to be stable. In this talk, we establish that bagging—averaging models trained on random subsets of data—automatically stabilizes any black-box algorithm, with finite-sample guarantees controlled by the fraction of samples used in each subset. These results extend beyond prediction to any statistical method with outputs in a Hilbert space, and to classification through a new 'inflated argmax' that adapts to model uncertainty. This talk is based on joint work with Rina Foygel Barber and Rebecca Willett.
Bio: Jake Soloff is a postdoc at the University of Chicago, mentored by Rina Foygel Barber and Rebecca Willett. He obtained his PhD in Statistics at UC Berkeley under Aditya Guntuboyina and Michael Jordan, receiving the Eric Lehmann Citation for outstanding dissertation in theoretical statistics. His research explores theoretical frontiers in nonparametric statistics, developing new frameworks for large-scale inference, stable learning, and statistical mechanism design.
December 6, 2024
David Shih
Rutgers University
Talk Title: Searching for the Unexpected from Colliders to Stars with Modern Machine Learning
This is a STAMPS hybrid event and will also be held via Zoom.
Abstract: Modern machine learning and generative AI are having an exciting impact on fundamental physics, allowing us to see deeper into the data and enabling new kinds of analyses that were not possible before. I will describe how we are using generative AI to develop powerful new model-agnostic methods for new physics searches at the Large Hadron Collider, and how these methods can also be applied to data from the Gaia Space Telescope to search for stellar streams. I will also describe how these same generative AI techniques can be used to perform a novel measurement of the local dark matter density using stars from Gaia as tracers of the Galactic potential.
Bio: David Shih is a Professor in the New High Energy Theory Center and the Department of Physics & Astronomy at Rutgers University. His current research focuses on developing new machine learning methods to tackle the major open questions in fundamental physics -- such as the nature of dark matter and new particles and forces beyond the Standard Model -- using big datasets from particle colliders and astronomy. His work has touched on many key topics at the intersection of ML and fundamental physics, including generative models, anomaly detection, AI fairness, feature selection, and interpretability. Shih is the recipient of an DOE Early Career Award, a Sloan Foundation Fellowship, the Macronix Prize, and the Humboldt Bessel Research Award.
November 18, 2024
Paul Gustafson
University of British Columbia, Department of Statistics
Talk Title: Bayesian Inference when Parameter Identification is Lacking: A Narrative Arc across Applications, Methods, and Theory
Abstract: Partially identified models generally yield “in between” statistical behavior. As the sample size goes to infinity, the posterior distribution on the target parameter heads to a distribution narrower than the prior distribution but wider than a single point. Such models arise naturally in many areas, including the health sciences. They arise particularly when we own up to limitations in how data are acquired. I aim to highlight the narrative arc associated with partial identification. This runs from the applied (e.g., broaching the topic with subject-area scientists), to the methodological (e.g., implementing a Bayesian analysis without full identification), to the theoretical (e.g., characterizing what is going on as generally as possible). As per many areas of statistics, there is good scope to get involved across the whole arc, rather than just at one end or other.
There will be a reception following the talk.
November 11, 2024
Xin Tong
University of Southern California, Data Sciences & Operations
Talk Title: Monoculture and Social Welfare of the Algorithmic Personalization Market under Competition
Abstract: Algorithmic personalization markets, where providers utilize algorithms to predict user types and personalize products or services, have become increasingly prevalent in our daily lives. The adoption of more accurate algorithms holds the promise of improving social welfare through enhanced predictive accuracy. However, concerns have been raised about algorithmic monoculture, where all providers adopt the same algorithms. The prevalence of a single algorithm can hinder social welfare due to the resulting homogeneity of available products or services. In this work, we address the emergence of algorithmic monoculture from the perspective of providers' behavior under competition in the algorithmic personalization market. We propose that competition among providers could mitigate monoculture, thereby enhancing social welfare in the algorithmic personalization market. By examining the impact of competition on algorithmic diversity, our study contributes to a deeper understanding of the dynamics within algorithmic personalization markets and offers insights into strategies for promoting social welfare in these contexts.
Bio: Xin Tong is an associate professor in the department of Data Sciences and Operations at the University of Southern California. His current research focuses on learning with partial information and asymmetry, social and economic networks, and Al ethics. He is an associate editor for JASA and JBES.
October 28, 2024
Kaizheng Wang
Columbia University, Industrial Engineering and Operations Research
Talk Title: Adaptive Transfer Clustering
Abstract: We develop a transfer learning framework for clustering given a main dataset and an auxiliary one about the same subjects. The two datasets may reflect similar but different grouping structures. We propose an adaptive transfer clustering (ATC) algorithm that automatically leverages the commonality in the presence of unknown discrepancy, by optimizing an estimated bias-variance decomposition. It applies to a broad class of statistical models, including Gaussian mixture models, stochastic block models, and latent class models. A theoretical analysis proves the optimality of ATC under the Gaussian mixture model and explicitly quantifies the benefit of transfer. Extensive simulations and real data experiments confirm its effectiveness in various scenarios. The talk is based on joint work with Zhongyuan Lyu and Yuqi Gu.
Bio: Kaizheng Wang is an assistant professor of Industrial Engineering and Operations Research, and a member of the Data Science Institute at Columbia University. He works at the intersection of statistics, machine learning, and optimization. He obtained his Ph.D. from Princeton University in 2020 and B.S. from Peking University in 2015.
October 21, 2024
Gennady Samorodnitsky
Cornell University, Department of Statistics and Data Science
Talk Title: Kernel PCA for learning multivariate extremes
Abstract: We provide general insights into kernel PCA algorithm that can effectively identify clusters of preimages when the data consists of a discrete signal with added noise. We then apply kernel PCA for describing dependence structure of multivariate extremes. Kernel PCA has been motivated as a tool for denoising and clustering of the approximate preimages. The idea is that such structure should be captured by the first principal components in a suitable function space. We provide some simple insights that naturally lead to clustered primages when the underlying data comes from a discrete signal corrupted by noise. Specifically, we use the Davis-Kahan theory to give a perturbation bound on the performance of preimages that quantifies the impact of noise in clustering a discrete signal. We then propose kernel PCA as a method for analyzing the dependence structure of multivariate extremes and demonstrate that it can be a powerful tool for clustering and dimension reduction. In this case, kernel PCA is applied only to the extremal part of the sample, i.e., the angular part of random vectors for which the radius exceeds a large threshold. More specifically, we focus on the asymptotic dependence of multivariate extremes characterized by the angular or spectral measure in extreme value theory and provide a careful analysis in the case where the extremes are generated from a linear factor model.
Bio: Gennady Samorodnitsky is a professor of Operations Research and Information Engineering at Cornell University. His research interests range from machine learning and differential privacy to extreme value theory, phase transitions between short and long range dependence, topology of random objects and interplay between probability and ergodic theory. He is a fellow of the Institute of Mathematical Statistics.
October 11, 2024
Gwendolyn Eadie
University of Toronto
Talk Title: Studying the Universe with Astrostatistics
Abstract: Astrostatistics is a growing interdisciplinary field at the interface of astronomy and statistics. Astronomy is a field rich with publicly available data, but inference using these data must acknowledge selection effects, measurement uncertainty, censoring, and missingness. In the Astrostatistics Research Team (ART) at the University of Toronto --- a joint team between the David A. Dunlap Department of Astronomy & Astrophysics and the Department of Statistical Sciences --- we take an interdisciplinary approach to analysing astronomical data from a range of objects such as stars, old clusters, and galaxies. In this talk, I will cover three ART projects that employ Bayesian inference techniques to: (1) find stellar flares in time series data from stars using hidden Markov models, (2) investigate the relationship between old star cluster populations and their host galaxies using hurdle models, and (3) discover potential "dark" galaxies within an inhomogeneous Poisson Process framework.
Bio: Gwendolyn Eadie is an Assistant Professor of Astrostatistics at the University of Toronto, jointly appointed between the Department of Statistical Sciences and the David A. Dunlap Department of Astronomy & Astrophysics. She is the founder and co-leader of UofT's Astrostatistics Research Team, and works on a range of projects that use hierarchical Bayesian inference to study galaxies, globular star clusters, stars, and fast radio bursts. She is also the current Chair of the Astrostatistics Interest Group of the American Statistical Association and the Chair of the Working Group on Astroinformatics & Astrostatistics of the American Astronomical Society.
October 7, 2024
Xiu Yang
Lehigh University, Systems Engineering
Talk Title: Challenges and Opportunities in Quantum Computing for Data Science
Abstract: Exploring the potential opportunities offered by quantum computing (QC) to speed up the solution of challenging application problems has attracted significant attention in recent years. A key barrier in developing QC methods is the error induced by the noise in the hardware as well as the statistical error in the measurement. In this talk, we will first introduce implementations of statistical methods like Bayesian inference on modeling and mitigating the error in prototype quantum circuits. Next, an alternative approach for a specific optimization problem will be presented to illustrate how uncertainty quantification can be conducted by identifying a new design of an algorithm. Finally, we will discuss the potential of QC to accelerate the development of AI/machine learning in training and implementing models.
Bio: Xiu Yang joined Lehigh from Pacific Northwest National Laboratory (PNNL) where he was a scientist since 2016. His research has been centered around modern scientific computing including uncertainty quantification, multi-scale modeling, physics-informed machine learning, and data-driven scientific discovery. Xiu has been applying his methods on various research areas such as fluid dynamics, hydrology, biochemistry, soft material, energy storage, and power grid systems. Currently, he is focusing on uncertainty quantification in quantum computing algorithms and machine learning methods for scientific computing. He received a Faculty Early Career Development Program (CAREER) Award from NSF in 2022 and Outstanding Performance Award from PNNL in 2015 and 2016. Xiu also served on the DOE applied mathematics visioning committee in 2019.
September 30, 2024
Kary Myers
Los Alamos National Laboratory, Department of Statistics
Talk title: Community Detection (the life kind, not the network science kind)
In this talk, I’ll share some behind-the-scenes career highs and lows that led to my current (very fun) role at Los Alamos National Laboratory. I’ll also offer thoughts on creating career resilience by expanding the definition of “communities” and bringing an intentionality to how you engage with them.
Bio: Kary Myers is a fellow of the American Statistical Association and currently leads a group of ~40 scientists and R&D engineers in the Space Remote Sensing and Data Science Group at Los Alamos National Laboratory (LANL). With support from an AT&T Labs Fellowship, she earned her Ph.D. from Carnegie Mellon’s Statistics and Data Science Department and her MS from their Machine Learning Department before joining LANL in 2006. She spent 15 years as a scientist in the Statistical Sciences Group at Los Alamos, including a few years as their deputy group leader and as the Deputy Director for Data Science in LANL’s Information Science and Technology Institute. She also served as LANL’s Intelligence and Emerging Threats Program Manager for Data Science. She’s been involved with a range of data-intensive projects, from analyzing electromagnetic measurements, to aiding large scale computer simulations, to developing analyses for chemical spectra from the Mars Science Laboratory Curiosity Rover. She served as an associate editor for the Annals of Applied Statistics and the Journal of Quantitative Analysis in Sports, and she created and organizes CoDA, the Conference on Data Analysis, to showcase data-driven research from across the Department of Energy.
September 20, 2024
STAMPS Research Center Launch Event
Tepper Quad, Simmons Auditorium B
Coffee & Refreshments at 3:30 PM, reception following the event.
STAMPS (STAtistical Methods for the Physical Sciences) is one of few university research groups specializing in foundational statistics and Al research for physical science applications ranging from particle and astrophysics to climate and environmental sciences. Starting fall of 2024, STAMPS is now becoming a CMU Research Center!
September 16, 2024
Maggie Niu
Preferential Latent Space Models for Networks with Textual Edges
Abstract: Many real-world networks contain rich textual information in the edges, such as email networks where an edge between two nodes is an email exchange. Other examples include co-author networks and social media networks. The useful textual information carried in the edges is often discarded in most network analyses, resulting in an incomplete view of the relationships between nodes. In this work, we propose to represent the text document between each pair of nodes as a vector counting the appearances of keywords extracted from the corpus, and introduce a new and flexible preferential latent space network model that can offer direct insights on how contents of the textual exchanges modulate the relationships between nodes. We establish identifiability conditions for the proposed model and tackle model estimation with a computationally efficient projected gradient descent algorithm. We further derive the non-asymptotic error bound of the estimator from each step of the algorithm. The efficacy of our proposed method is demonstrated through simulations and an analysis of the Enron email network.
Bio: Xiaoyue Maggie Niu is an Associate Professor of Statistics and Director of the Statistical Consulting Center at Penn State. Her research focuses on the development of statistical models that solve real world problems, especially with applications in health and social sciences. The methodological approaches she takes include Bayesian methods, social network models, and latent variable models. Another big part of her work is in the statistical consulting center. She collaborates with a variety of researchers on campus and mentors graduate students to work with them. Solving practically important problems and interacting with people from diverse backgrounds are the most enjoyable part of her work. She received her Ph.D. in Statistics from University of Washington and her B.S. in Applied Math from Peking University.
April 22, 2024
Anderson Zhang
Spectral Clustering: Methodology and Statistical Analysis
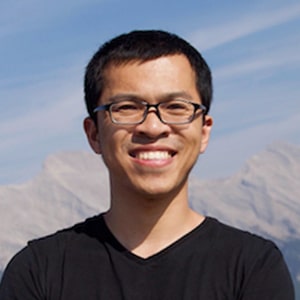
Abstract: Spectral clustering is one of the most popular algorithms to group high-dimensional data. It is easy to implement, computationally efficient, and has achieved tremendous success in many applications. The idea behind spectral clustering is dimensionality reduction. It first performs a spectral decomposition on the dataset and only keeps the leading few spectral components to reduce the data's dimension. It then applies some standard methods such as the k-means on the low-dimensional space to do clustering. In this talk, we demystify the success of spectral clustering by providing a sharp statistical analysis of its performance under mixture models. For isotropic Gaussian mixture models, we show spectral clustering is optimal. For sub-Gaussian mixture models, we derive exponential error rates for spectral clustering. To establish these results, we develop a new spectral perturbation analysis for singular subspaces.
Bio: Anderson Ye Zhang is an Assistant Professor in the Department of Statistics and Data Science at the Wharton School, with a secondary appointment in the Department of Computer and Information Science, at the University of Pennsylvania. Before joining Wharton, he was a William H. Kruskal Instructor in the Department of Statistics at the University of Chicago. He obtained his Ph.D. degree from the Department of Statistics and Data Science at Yale University. His research interests include network analysis, clustering, spectral analysis, and ranking from pairwise comparisons.
April 12, 2024
Alicia Carriquiry
Statistics and its Applications in Forensic Science and the Criminal Justice System
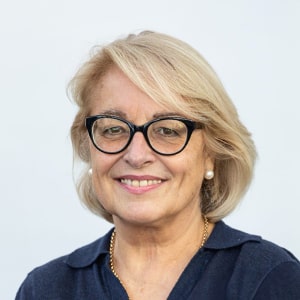
Abstract: Steve Fienberg was a pioneer in highlighting the role of statistical thinking in the civil and criminal justice systems and was an early critic of many forensic methods that are still in use in US courts. One of his last achievements was the creation of the Center for Statistics and Applications in Forensic Evidence (CSAFE), a federally funded NIST Center of Excellence, with the mission to build the statistical foundation – where possible – for what is known as forensic pattern comparison disciplines and digital forensics.
Forensic applications present unique challenges for statisticians. For example, much of the data that arise in forensics are non-standard, so even defining analytical variables may require out-of-the-box thinking. As a result, the usual statistical approaches may not enable addressing the questions of interest to jurors, legal professionals and forensic practitioners.
Today’s presentation introduces some of the statistical and algorithmic methods proposed by CSAFE researchers that have the potential to impact forensic practice in the US. Two examples are used for illustration: the analysis of questioned handwritten documents and of marks imparted by firearms on bullets or cartridge cases. In both examples, the question we address is one of source: do two or more items have the same source? In the first case, we apply “traditional” statistical modeling methods, while in the second case, we resort to algorithmic approaches. Much of the research carried out in CSAFE is collaborative and while mission-driven, also academically rigorous, which would have pleased Steve tremendously.
Bio: Alicia Carriquiry (NAM) is Professor of Statistics at Iowa State University. Between January of 2000 and July of 2004, she was Associate Provost at Iowa State. Her research interests are in Bayesian statistics and general methods. Her recent work focuses on nutrition and dietary assessment, as well as on problems in genomics, forensic sciences and traffic safety. Dr. Carriquiry is an elected member of the International Statistical Institute and a fellow of the American Statistical Association. She serves on the Executive Committee of the Institute of Mathematical Statistics and has been a member of the Board of Trustees of the National Institute of Statistical Sciences since 1997. She is also a past president of the International Society for Bayesian Analysis (ISBA) and a past member of the Board of the Plant Sciences Institute at Iowa State University.
April 5, 2024
Andrew Gelman
Bayesian Workflow: Some Progress and Open Questions
Abstract: The workflow of applied Bayesian statistics includes not just inference but also model building, model checking, confidence-building using fake data, troubleshooting problems with computation, model understanding, and model comparison. We would like to toward codify these steps in the realistic scenario in which researchers are fitting many models for a given problem. We discuss various issues including prior distributions, data models, and computation, in the context of ideas such as the Fail Fast Principle and the Folk Theorem of Statistical Computing. We also consider some examples of Bayesian models that give bad answers and see if we can develop a workflow that catches such problems.
Bio: Andrew Gelman is a professor of statistics and political science at Columbia University. He has received the Outstanding Statistical Application award three times from the American Statistical Association, the award for best article published in the American Political Science Review, the Mitchell and DeGroot prizes from the International Society of Bayesian Analysis, and the Council of Presidents of Statistical Societies award. His books include Bayesian Data Analysis (with John Carlin, Hal Stern, David Dunson, Aki Vehtari, and Donald Rubin), Teaching Statistics: A Bag of Tricks (with Deborah Nolan), Data Analysis Using Regression and Multilevel/Hierarchical Models (with Jennifer Hill), Red State, Blue State, Rich State, Poor State: Why Americans Vote the Way They Do (with David Park, Boris Shor, and Jeronimo Cortina), A Quantitative Tour of the Social Sciences (co-edited with Jeronimo Cortina), and Regression and Other Stories (with Jennifer Hill and Aki Vehtari).
Andrew has done research on a wide range of topics, including: why it is rational to vote; why campaign polls are so variable when elections are so predictable; the effects of incumbency and redistricting; reversals of death sentences; police stops in New York City, the statistical challenges of estimating small effects; the probability that your vote will be decisive; seats and votes in Congress; social network structure; arsenic in Bangladesh; radon in your basement; toxicology; medical imaging; and methods in surveys, experimental design, statistical inference, computation, and graphics.
March 25, 2024
Glenn Shafer
Modernizing Cournot’s Principle
Abstract: In everyday English, a forecast is something less than a prediction. It is more like an estimate. When an economist forecasts 3.5% inflation in the United States next year, or my weather app forecasts 0.55 inches of rain, these are not exactly predictions. When the forecaster gives rain a 30% probability, this too is not a prediction. A prediction is more definite about what is predicted and about predicting it.
We might say that a probability is a prediction when it is very close to one. But this formulation has a difficulty: there are too many high probabilities. There is a high probability against every ticket in a lottery, but we cannot predict that no ticket will win.
Game-theoretic statistics resolves this problem by showing how some high probabilities are simpler than others. The simpler ones qualify as predictions.
This story has roles for Cournot’s principle, Kolmogorov’s algorithmic complexity, and de Finetti’s previsione. See www.probabilityandfinance.com and my two books on the topic with Vladimir Vovk.
Bio: In the 1970s, Glenn launched the “Dempster-Shafer” theory. The Belief Functions and Applications Society, devoted to this theory, has been holding international conferences since 2010.
During the past 25 years, Glenn and Vladimir Vovk launched game-theoretic probability and statistics. Their two books on the topic appeared in 2001 and 2019.
Glenn has published more than 20 papers on the history of probability and statistics. His most recent book, The Splendors and Miseries of Martingales: Their History from the Casino to Mathematics, co-edited with Laurent Mazliak, was published by Birkhäuser in 2022.
Glenn served in the Peace Corps in Afghanistan. At the Rutgers Business School, he served as director of the doctoral program for ten years and as dean for four years.
March 18, 2024
Martin Wainwright
Challenges with Covariate Shift: From Prediction to Causal Inference
Abstract: In many modern uses of predictive methods, there can be shifts between the distributional properties of training data compared to the test data. Such mismatches can cause dramatic reductions in accuracy that remain mysterious. How to find practical procedures that mitigate such effects in an optimal way? In this talk, we discuss the fundamental limits of problems with covariate shift, and simple procedures that achieve these fundamental limits. Our talk covers both the challenges of covariate shift in non-parametric regression, and also in semi-parametric problems that arise from causal inference and off-policy evaluation.
Based on joint works with: Peter Bartlett, Peng Ding, Cong Ma, Wenlong Mou, Reese Pathak and Lin Xiao.
Bio: Martin Wainwright is the Cecil H. Green Professor in Electrical Engineering and Computer Science and Mathematics at MIT, and affiliated with the Laboratory for Information and Decision Systems and Statistics and Data Science Center.
His main claim to fame is that he was the graduate advisor of Nihar Shah, and postdoc advisor of Aaditya Ramdas, Pradeep Ravikumar (all esteemed faculty at CMU), and Sivaraman Balakrishnan. He has also received a number of awards and recognition including an Alfred P. Sloan Foundation Fellowship, best paper awards from the IEEE Signal Processing Society, the IEEE Communications Society, and the IEEE Information Theory and Communication Societies, the Medallion Lectureship and Award from the Institute of Mathematical Statistics, and the COPSS Presidents’ Award from the Joint Statistical Societies. He was a Section Lecturer with the International Congress of Mathematicians in 2014 and received the Blackwell Award from the Institute of Mathematical Statistics in 2017.
February 7, 2024
Shuangning Li
Inference and Decision-Making amid Social Interactions
Abstract: From social media trends to family dynamics, social interactions shape our daily lives. In this talk, I will present tools I have developed for statistical inference and decision-making in light of these social interactions.
- Inference: I will talk about estimation of causal effects in the presence of interference. In causal inference, the term “interference” refers to a situation where, due to interactions between units, the treatment assigned to one unit affects the observed outcomes of others. I will discuss large-sample asymptotics for treatment effect estimation under network interference where the interference graph is a random draw from a graphon. When targeting the direct effect, we show that popular estimators in our setting are considerably more accurate than existing results suggest. Meanwhile, when targeting the indirect effect, we propose a consistent estimator in a setting where no other consistent estimators are currently available.
- Decision-Making: Turning to reinforcement learning amid social interactions, I will focus on a problem inspired by a specific class of mobile health trials involving both target individuals and their care partners. These trials feature two types of interventions: those targeting individuals directly and those aimed at improving the relationship between the individual and their care partner. I will present an online reinforcement learning algorithm designed to personalize the delivery of these interventions. The algorithm's effectiveness is demonstrated through simulation studies conducted on a realistic test bed, which was constructed using data from a prior mobile health study. The proposed algorithm will be implemented in the ADAPTS HCT clinical trial, which seeks to improve medication adherence among adolescents undergoing allogeneic hematopoietic stem cell transplantation.
Bio: Shuangning Li is a postdoctoral fellow working with Professor Susan Murphy in the Department of Statistics at Harvard University. Prior to this, she earned her Ph.D. from the Department of Statistics at Stanford University, where she was advised by Professors Emmanuel Candès and Stefan Wager.
February 5, 2024
Brian Trippe
Probabilistic methods for designing functional protein structures
Abstract: The biochemical functions of proteins, such as catalyzing a chemical reaction or binding to a virus, are typically conferred by the geometry of only a handful of atoms. This arrangement of atoms, known as a motif, is structurally supported by the rest of the protein, referred to as a scaffold. A central task in protein design is to identify a diverse set of stabilizing scaffolds to support a motif known or theorized to confer function. This long-standing challenge is known as the motif-scaffolding problem.
In this talk, I describe a statistical approach I have developed to address themotif-scaffolding problem. My approach involves (1) estimating a distribution supported on realizable protein structures and (2) sampling scaffolds from this distribution conditioned on a motif. For step (1) Iadapt diffusion generative models to fit example protein structures from nature. For step (2) I develop sequential monte carlo algorithms to sample from the conditional distributions of these models. I finally describe how, with experimental and computational collaborators, I have generalized and scaled this approach to generate and experimentally validate hundreds of proteins with various functional specifications.
Bio: Brian Trippe is a postdoctoral fellow at Columbia University In the Department of Statistics, and a visiting researcher at the Institute for Protein Design at the University of Washington. He completed his Ph.D. in Computational and Systems Biology at the Massachusetts Institute of Technology where he worked on Bayesian methods for inference in hierarchical linear models. In his research, Brian develops statistical machine learning methods to address challenges in biotechnology and medicine, with a focus on generative modeling and inference algorithms for protein engineering.
January 31, 2024
Michael Celentano
Debiasing in the inconsistency regime
Abstract: In this talk, I will discuss semi-parametric estimation when nuisance parameters cannot be estimated consistently, focusing in particular on the estimation of average treatment effects, conditional correlations, and linear effects under high-dimensional GLM specifications. In this challenging regime, even standard doubly-robust estimators can be inconsistent. I describe novel approaches which enjoy consistency guarantees for low-dimensional target parameters even though standard approaches fail. For some target parameters, these guarantees can also be used for inference. Finally, I will provide my perspective on the broader implications of this work for designing methods which are less sensitive to biases from high-dimensional prediction models.
Bio: Michael Celentano is a Miller Fellow in the Statistics Department at the University of California, Berkeley, advised by Martin Wainwright and Ryan Tibshirani. He received his Ph.D. in Statistics from Stanford University in 2021, where he was advised by Andrea Montanari. Most of his work focuses on the high-dimensional asymptotics for regression, classification, and matrix estimation problems.
January 29, 2024
Ying Jin
Model-free selective inference: from calibrated uncertainty to trusted decisions
Abstract: AI has shown great potential in accelerating decision-making and scientific discovery pipelines such as drug discovery, marketing, and healthcare. In many applications, predictions from black-box models are used to shortlist candidates whose unknown outcomes satisfy a desired property, e.g., drugs with high binding affinities to a disease target. To ensure the reliability of high-stakes decisions, uncertainty quantification tools such as conformal prediction have been increasingly adopted to understand the variability in black-box predictions. However, we find that the on-average guarantee of conformal prediction can be insufficient for its deployment in decision making which usually has a selective nature.
In this talk, I will introduce a model-free selective inference framework that allows us to select reliable decisions with the assistance of any black-box prediction model. Our framework identifies candidates whose unobserved outcomes exceed user-specified values while controlling the average proportion of falsely selected units (FDR), without any modeling assumptions. Leveraging a set of exchangeable training data, our method constructs conformal p-values that quantify the confidence in large outcomes; it then determines a data-dependent threshold for the p-values as a criterion for drawing confident decisions. In addition, I will discuss new ideas to further deal with covariate shifts between training and new samples. We show that in several drug discovery tasks, our methods narrow down the drug candidates to a manageable size of promising ones while controlling the proportion of falsely discovered. In a causal inference dataset, our methods identify students who benefit from an educational intervention, providing new insights for causal effects.
Bio: Ying Jin is a fifth-year Ph.D. student at Department of Statistics, Stanford University, advised by Emmanuel Candès and Dominik Rothenhäusler. Prior to this, she obtained B.S. in Mathematics from Tsinghua University. Her research focuses on devising modern statistical methodology that enables trusted inference and decisions with minimal assumptions, covering conformal inference, multiple testing, causal inference, distribution robustness, and data-driven decision-making.
January 24, 2024
Arkajyoti Saha
Inference for machine learning under dependence
Abstract: Recent interest has centered on uncertainty quantification for machine learning models. For the most part, this work has assumed independence of the observations. However, many of the most important problems arising across scientific fields, from genomics to climate science, involve systems where dependence cannot be ignored. In this talk, I will investigate conference on machine learning models in the presence of dependence.
In the first part of my talk, I will consider a common practice in the field of genomics in which researchers compute a correlation matrix between genes and threshold its elements in order to extract groups of independent genes. I will describe how to construct valid p-values associated with these discovered groups that properly account for the group selection process. While thesis related to the literature on selective inference developed in the past decade, this work involves inference about the covariance matrix rather than the mean, and therefore requires an entirely new technical toolset. This same toolset can be applied to quantify the uncertainty associated with canonical correlation analysis after feature screening.
In the second part of my talk, I will turn to an important problem in the field of oceanography as it relates to climate science. Oceanographers have recently applied random forests to estimate carbon export production, a key quantity of interest, at a given location in the ocean; they then wish to sum the estimates across the world’s oceans to obtain an estimate of global export production. While quantifying uncertainty associated with a single estimate is relatively straightforward, quantifying uncertainty of the summed estimates is not, due to their complex dependence structure. I will adapt the theory of V-statistics to this dependent data setting in order to establish a central limit theorem for the summed estimates, which can be used to quantify the uncertainty associated with global export production across the world’s oceans.
This is joint work with my postdoctoral supervisors, Daniela Witten (University of Washington) and Jacob Bien (University of Southern California).
Bio: Arkajyoti Saha is a postdoctoral fellow in the Department of Statistics, University of Washington. He received his Ph.D. in Biostatistics from the Johns Hopkins Bloomberg School of Public Health. His research lies at the intersection of machine learning, selective inference, and spatial statistics,with a focus on machine learning under dependence with applications in genomics and oceanography.
January 22, 2024
Satarupa Bhattacharjee
Geodesic Mixed Effects Models for Repeatedly Observed/Longitudinal Random Objects
Abstract: Mixed effect modeling for longitudinal data is challenging when the observed data are random objects, which are complex data taking values in a general metric space without either global linear or local linear (Riemannian) structure. In such settings, the classical additive error model and distributional assumptions are unattainable. Due to the rapid advancement of technology, longitudinal data containing complex random objects, such as covariance matrices, data on Riemannian manifolds, and probability distributions are becoming more common. Addressing this challenge, we develop a mixed-effects regression for data in geodesic spaces, where the underlying mean response trajectories are geodesics in the metric space and the deviations of the observations from the model are quantified by perturbation maps or transports. A key finding is that the geodesic trajectories assumption for the case of random objects is a natural extension of the linearity assumption in the standard Euclidean scenario to the case of general geodesic metric spaces. Geodesics can be recovered from noisy observations by exploiting a connection between the geodesic path and the path obtained by global Fréchet regression for random objects. The effect of baseline Euclidean covariates on the geodesic paths is modeled by another Fréchet regression step. We study the asymptotic convergence of the proposed estimates and provide illustrations through simulations and real-data applications.
Bio: I am a Postdoctoral Scholar in the Department of Statistics at Pennsylvania State University, working with Prof. Bing Li and Prof. Lingzhou Xue. I received my Ph.D. in Statistics at UC Davis advised by Prof. Hans-Georg Müller in September 2022.
My primary research centers around analyzing functional and non-Euclidean data situated in general metric spaces, which we refer to as random objects, with examples in brain imaging data, networks, distribution valued data, and high-dimensional genetics data.
January 17, 2024
Christopher Harshaw
Algorithm Design for Randomized Experiments
Abstract: Randomized experiments are one of the most reliable causal inference methods and are used in a variety of disciplines from clinical medicine, public policy, economics, and corporate A/B testing. Experiments in these disciplines provide empirical evidence which drives some of the most important decisions in our society: What drugs are prescribed? Which social programs are implemented? What corporate strategies to use? Technological advances in measurements and intervention -- including high dimensional data, network data, and mobile devices -- offer exciting opportunities to design new experiments to investigate a broader set of causal questions. In these more complex settings, standard experimental designs (e.g. independent assignment of treatment) are far from optimal. Designing experiments which yield the most precise estimates of causal effects in these complex settings is not only a statistical problem, but also an algorithmic one.
In this talk, I will present my recent work on designing algorithms for randomized experiments. I will begin by presenting Clip-OGD, a new algorithmic experimental design for adaptive sequential experiments. We show that under the Clip-OGD design, the variance of an adaptive version of the Horvitz-Thompson estimator converges to the optimal non-adaptive variance, resolving a70-year-old problem posed by Robbins in 1952. Our results are facilitated by drawing connections to regret minimization in online convex optimization. Time permitting, I will describe a new unifying framework for investigating causal effects under interference, where treatment given to one subject can affect the outcomes of other subjects. Finally, I will conclude by highlighting open problems and reflecting on future work in these directions.
Bio: Christopher Harshaw is a FODSI postdoc at MIT and UC Berkeley. He received his Ph.D. from Yale University where he was advised by Dan Spielman and Amin Karbasi. His research lies at the interface of causal inference, machine learning, and algorithm design, with a particular focus on the design and analysis of randomized experiments. His work has appeared in the Journal of the American Statistical Association, Electronic Journal of Statistics, ICML, NeurIPS, and won Best Paper Award at the NeurIPS 2022 workshop, CML4Impact.