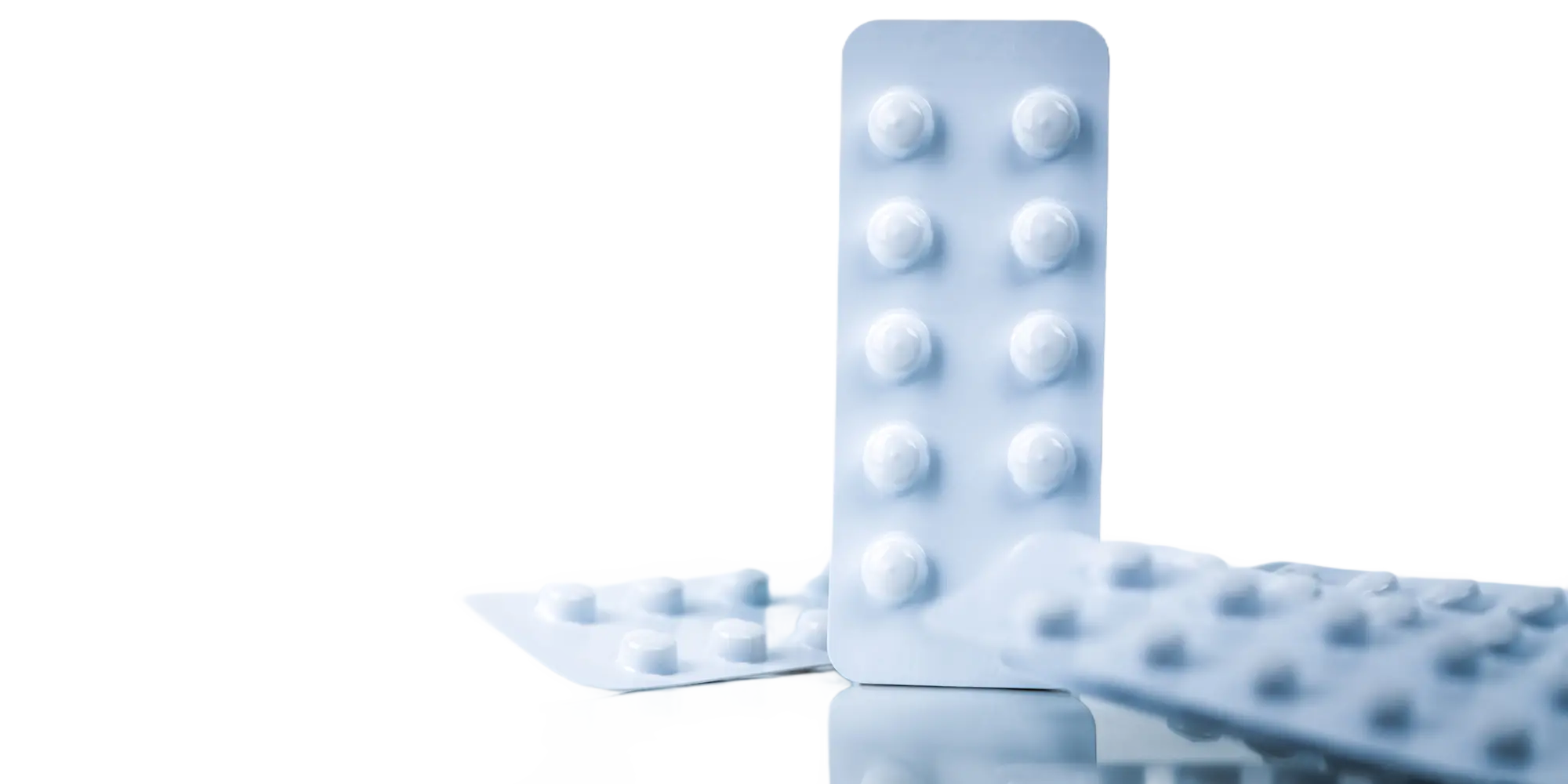
Collaboration on AI-powered Patient Safety Research Flags Drug Side Effects
Media Inquiries
A recent capstone project by an interdisciplinary team of graduate students from Heinz College of Information Systems and Public Policy(opens in new window) at Carnegie Mellon University and the University of Pittsburgh School of Medicine used artificial intelligence to examine health care data and look for patterns to detect potentially dangerous drug interactions.
Four CMU master’s degree students used AI and machine learning to review databases housed at the University of Pittsburgh to look for signals of adverse drug events, adverse drug reactions or medication errors in millions of records from more than 600,000 patients from across three years of routinely collected patient care data.
“We learned all these applied skills in class — data cleaning, data analysis — but then in real-world applications, you start to see all these obstacles that you have to know how to get around,” said Quentin Auster, a student in the Heinz College’s Master of Public Policy and Management: Data Analytics(opens in new window) program. He led the capstone project team that also included Joanna Sam and (now-graduate) Alex Liu of the Master of Information Systems Program in Business Intelligence & Data Analytics(opens in new window); and Yue (Zoey) Sun, a student in the Master of Health Care Analytics & Information Technology(opens in new window) Program.
The research team received access to the data through funding from the Pittsburgh Regional Autonomous Patient Safety(opens in new window) (RAPS) initiative of the Jewish Healthcare Foundation that established Carnegie Mellon’s Initiative for Patient Safety Research(opens in new window) (IPSR) in 2022 with a two-year $500,000 grant.
Detecting Adverse Events
The students mined association rules in the medication data using a method often applied to e-commerce data. Instead of suggesting similar products for customers to buy together, in this case they generated the rules from the data to guide the discovery of different medication combinations that may lead to adverse outcomes, said Rema Padman(opens in new window), Trustees Professor of Management Science and Healthcare Informatics in the Heinz College and faculty advisor of the capstone project. The team also collaborated with Ari Lightman(opens in new window), digital media and marketing professor in the Heinz College, and Alan Scheller-Wolf(opens in new window), Richard M. Cyert Professor of Operations Management in the Tepper School of Business(opens in new window), both faculty leads at IPSR who facilitated access to the data at the University of Pittsburgh.
Once these combinations are identified, researchers can determine those with the highest frequency within the hundreds of thousands of records in the dataset. Clinicians can use the information to verify the risk of an adverse reaction and identify alternative medications. Detecting and reviewing the importance of the anomalies in the data would be difficult without AI and machine learning, Padman said.
“These methods can really help to sift through the vast amounts of data, since every patient might be taking multiple medications, and with thousands of patients, there are many different combinations to examine,” she said. “We can apply these methods to extract some useful information.”
Uncovering Patterns Within The Data
The team from Carnegie Mellon collaborated with Richard D. Boyce, Associate Professor of Biomedical Informatics at the University of Pittsburgh, and his research team to obtain secure access to the data, including Pitt’s Medication Error Avoidance at Regional Scale(opens in new window) (MEARs) database and the U.S. Food and Drug Administration’s Adverse Event Reporting System database(opens in new window) (FAERS), and specialized domain knowledge about MEARs and medication-related errors.
The capstone team conducted research through an open-source approach in a highly secure virtual workbench using a combination of data science tools that included a user-friendly research web application for large-scale analytics developed by the Observational Health Data Sciences and Informatics (or OHDSI, “Odyssey”) collaborative. As the students progressed, they gained an understanding of patient journeys through visualization and analysis.
The team demonstrated their approach by narrowing their focus to patients taking colchicine, typically used to treat gout but increasingly prescribed to prevent coronary artery disease(opens in new window). Then, they looked for the antibiotic clarithromycin and medications like it that influence how the body breaks down colchicine.
Partners in Health Care Data
Carnegie Mellon University participates in the Pittsburgh Health Data Alliance(opens in new window), which unites the world-class computer science, artificial intelligence and medical research from CMU and University of Pittsburgh with clinical expertise and data from UPMC to facilitate innovation in digital health. The effort includes the Center for Machine Learning and Health(opens in new window) and projects focus on health care outcomes, consumer-oriented health care, and health care infrastructure and efficiencies.
Capstones, Explained
Experiential learning is more than a buzz term. Students at the Heinz College engage in hands-on projects with industry partners to solve real-world problems.
As the culmination of their learning, a team of students applies the skills they’ve learned. Over 15 weeks, students will work with their client and a faculty mentor to create an innovative and customized solution to a challenge the partner has identified. Check out examples of recent capstone projects(opens in new window).
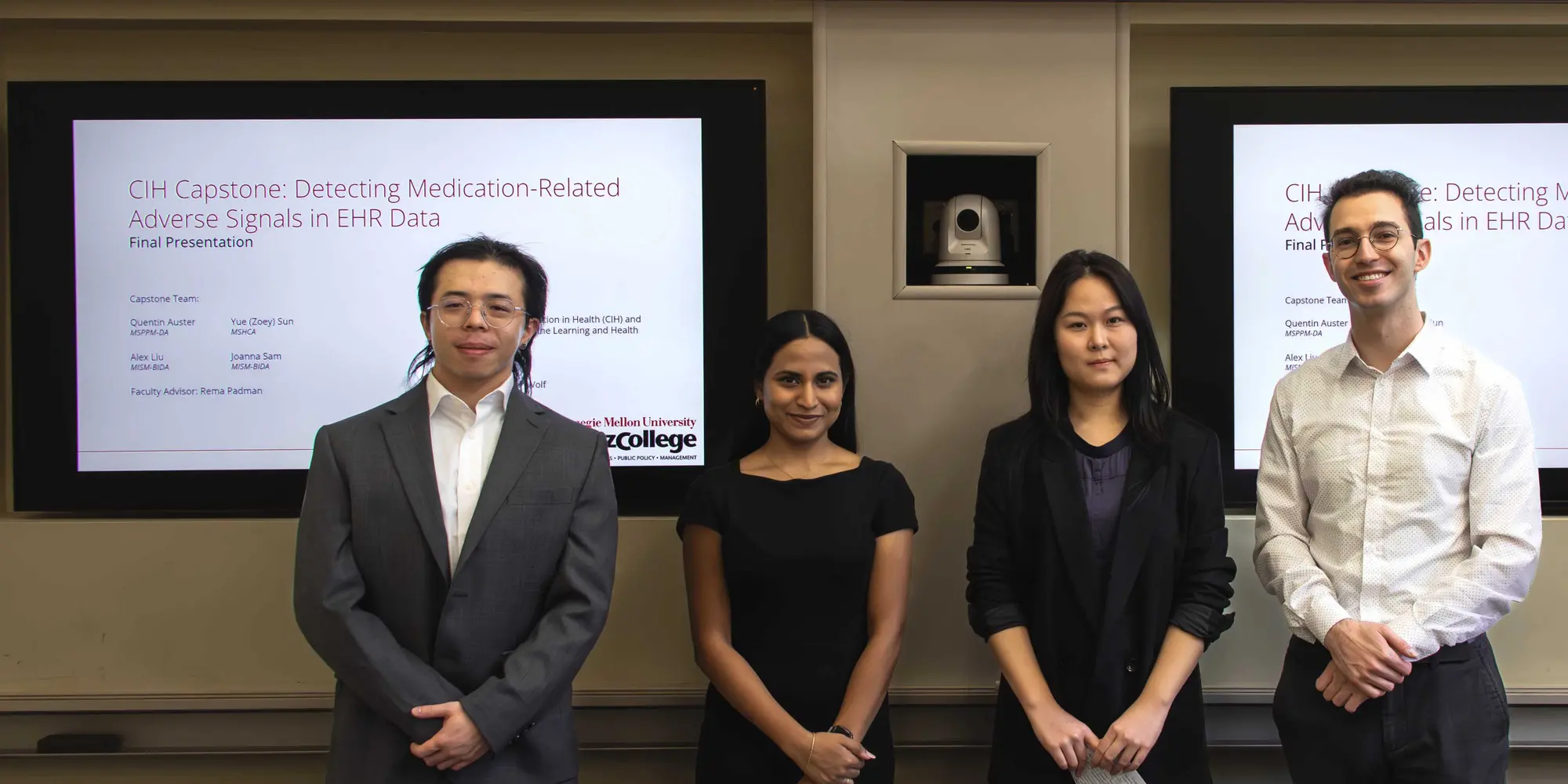
“If you are a doctor practicing every day, you should know this already, so you shouldn’t have prescribed this combination together,” Sun said. “We wouldn’t expect to see a lot of instances in the EHR (electronic health record) system, which added to the difficulty for us to research and study trying to find this combination.”
In applying association rule-mining to the EHR data, the team noticed the frequent pairing of colchicine with metoprolol, used to treat high blood pressure but potentially exacerbate a patient’s gout.
Their findings show that nuanced clinical judgment is necessary in the interpretation of data-derived medication patterns, said Sun, who also holds a doctorate in pharmacy, knowledge that was invaluable in helping the team decipher information on pharmaceuticals.
In future work, Padman said the approach used in this project can be evaluated using other known combinations of medications that result in adverse events or reactions, then generalized to detect new combinations that can be verified by domain experts.
“There’s really not a flag that’s specific to say ‘an adverse drug event happened here,’ so it’s a bit like if you lose your keys,” Auster said. “You're going to look around the streetlamp where you might have lost them. The streetlamp in this case was colchicine, which has actual signals of a place where we would expect adverse events to happen.”
Determining Future Solutions
Through the IPSR, Padman is also advising other Ph.D. students on similar patient safety-related research, all part of the Carnegie Mellon’s Center for Innovation in Health(opens in new window), led by Carl Kingsford(opens in new window), Herbert A. Simon Professor of Computer Science in the Computational Biology Department of the School of Computer Science(opens in new window).
“AI does not start at the beginning of the deep network,” Kingsford said. “You can’t train AI without data. You can’t do anything without setting up a problem. All that stuff is super-crucial, especially in the health care domain — structured data, unstructured data, weird specialized terms — that all have to be put into a model that can be used to train and apply AI.”
About 250,000 to 400,000 people die annually from preventable medical errors, said Karen Feinstein, CEO of the Jewish Healthcare Foundation(opens in new window). For now, because of the fragmented nature of the health care industry, she said investment in patient safety is difficult to incentivize, but research like this project can help interest in it gain momentum.
“Health care systems — and the data that evaluate their performance — are complex and confusing,” she said. “Employers and patients lack knowledge of the serious safety deficits that put them at risk and lack avenues to express their concerns about safety. In addition, the convoluted payment systems for health care do not reward exceptional performance in quality or safety and regulation has, so far, proved ineffective. This has put health care far behind other industries in their product and services safety. It does, however, leave the door wide open for entrepreneurs, and CMU is equipping students with the skills and insight to help drive the revolution of tech solutions for patient safety.”
@CarnegieMellon and @PittTweet are more than just neighbors in Oakland. This article from @PittMedMag explores how the two schools are collaborating to train the next generation of #BiomedicalLeaders. https://t.co/szKqEPAAYm
— Computational & Systems Biology at Pitt (@compbiopitt) February 23, 2024