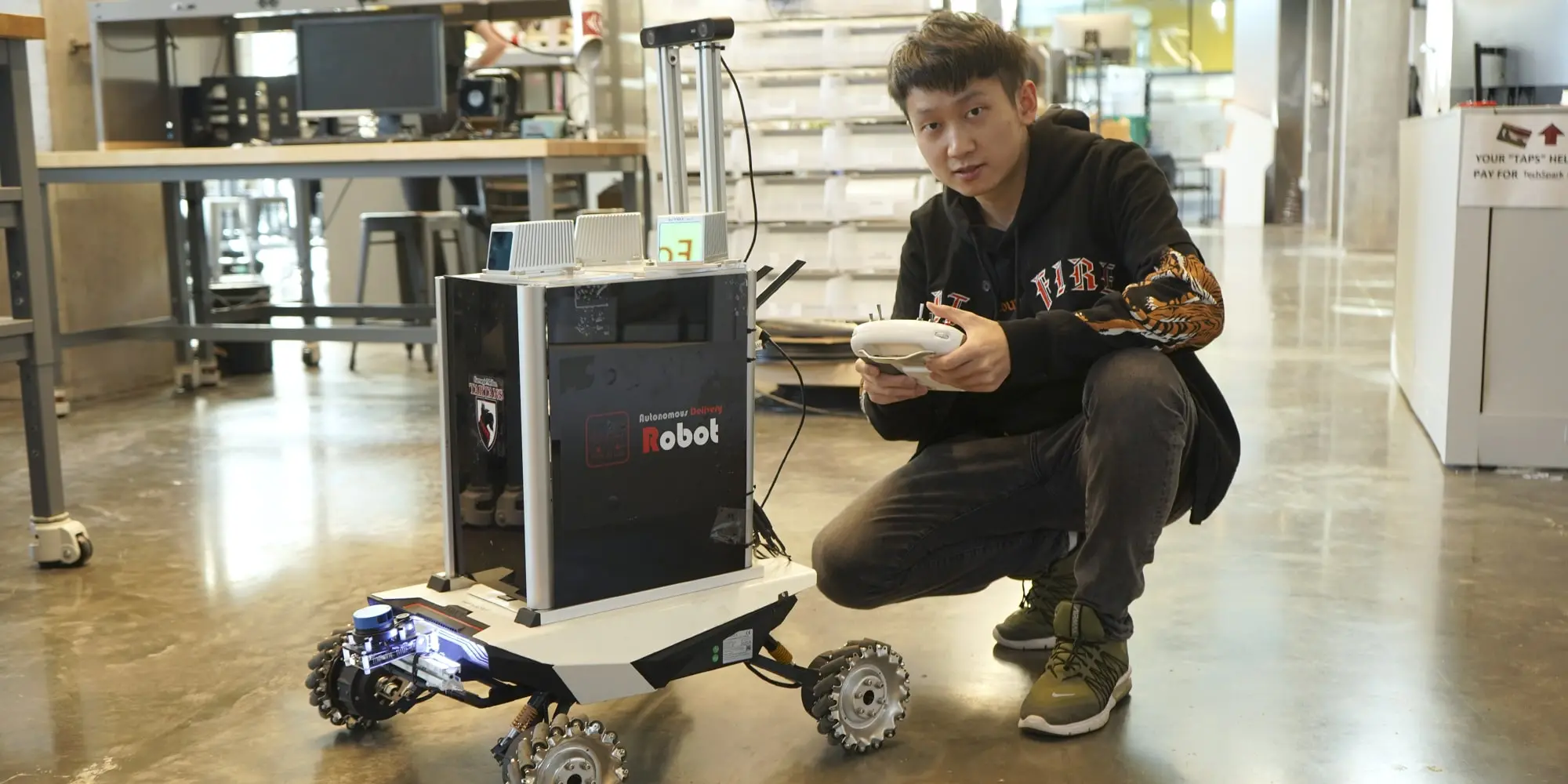
Robots Can Learn To Safely Navigate Warehouses
Media Inquiries
Robots have been working in factories for many years. But given the related safety concerns to the tasks they perform, most operate inside cages or behind safety glass to limit or prevent interaction with humans.
In warehouse operations, where goods are continuously sorted and moved, robots can be neither caged nor stationary. And while large corporations like Amazon have already incorporated robots into their warehouses, they are highly customized and costly systems where robots are designed to work within one facility on predefined grids or well-defined pathways under the guidance of specific, centralized programming that carefully directs their activity.
"For robots to be most useful in a warehouse, they will need to be smart enough to deploy in any facility easily and quickly; able to train themselves to navigate in new dynamic environments; and most importantly, be able to safely work with humans, as well as sizeable fleets of other robots," said Ding Zhao(opens in new window), the principal investigator and assistant professor of mechanical engineering at Carnegie Mellon University.
"Warehouse robots need to be smart enough to deploy quickly and navigate safely in new dynamic environments." — Ding Zhao
A team of CMU engineers and computer scientists have employed their expertise in advanced manufacturing, robotics and artificial intelligence to develop the warehouse robots of the future.
The collaboration was formed at the university's Manufacturing Future's Institute(opens in new window) (MFI), which funds research with grants from the Richard King Mellon Foundation. The foundation made a lead $20 million grant in 2016 and gave an additional $30 million in 2021 to support advanced manufacturing research and development at MFI.
Zhao and Martial Hebert(opens in new window), the dean of the School of Computer Science(opens in new window) and a professor at the Robotics Institute(opens in new window), are leading the warehouse robot project. They have investigated multiple reinforcement learning techniques that have shown measurable improvements over previous methods in simulated motion-planning experiments. The software used in their test robot has also performed well in path-planning experiments at Mill 19(opens in new window), MFI's collaborative workspace.
"Thanks to the advance in chips, sensors and advanced AI algorithms, we are at the cusp of revolutionizing the manufacturing robots," said Zhao. The team leverages previous work in self-driving cars to the development of warehouse robots that can learn multi-task path planning via safe reinforced learning, training robots to quickly adapt to new environments and operate safely with workers and human-operated vehicles.
MAPPER: Robots that can learn to plan their own pathways
The group first developed a method that could enable robots to continuously learn to plan routes in large, dynamic environments. The Multi-Agent Path Planning with Evolutionary Reinforcement(opens in new window) (MAPPER) learning method will allow the robots to explore by themselves and learn by trial and error in a manner similar to the way human babies accumulate more experience to handle various situations over time.
The decentralized method eliminates the need to program the robots from a powerful central command computer. Instead, the robots make independent decisions based on their own local observations. The robots' capabilities will enable their onboard sensors to observe dynamic obstacles within a 10-30-meter range. With reinforced learning, robots will continually train themselves how to handle unknown dynamic obstacles.
Such smart robots can enable warehouses to employ large fleets of robots more easily and quickly. Because the computation is done with each robot's onboard resources, the computation complexity will increase mildly as the robot number increases, which will make it easier to add, remove or replace the robots.
Energy consumption could also be reduced when robots travel shorter distances because they are able to independently learn to plan their own efficient paths. And the "decentralized and partially observable" setting will reduce the communication and computation energy when compared to classical centralized methods.
In November 2021, Ding Zhao and his students demonstrated their warehouse robot to Pennsylvania Sens. Ryan Aument, Joe Pittman and Pat Stefano; and Reps. Josh Kail and Natalie Mihalek, who were touring the College of Engineering.
RCE: Robots that prioritize safety while in pursuit of programmed goal
Another successful study applied the use of a constrained model-based reinforcement learning with the Robust Cross-Entropy(opens in new window) (RCE) method.
Researchers must explicitly consider safety constraints for a learning robot so that it does not sacrifice safety in order to finish tasks. For example, the robot needs to avoid colliding with other robots, damaging goods or interfering with equipment in order to reach its goal.
"Although reinforcement learning methods have achieved great success in virtual applications such as computer games, there are still a number of difficulties in applying them to real-world robotic applications. Among them, safety is premium," said Zhao.
Creating such safety constraints that always factored in all conditions goes beyond traditional reinforcement learning methods into the increasingly important area of safe reinforcement learning, which is essential to deploying such new technologies.
Mengdi Xu, a Ph.D. student at the CMU Safe AI Lab, works with an intelligent manufacturing manipulation robot.
The team evaluated their new RCE method in the Safety Gym, a set of virtual environments and tools for measuring progress toward reinforcement learning agents that respect safety constraints while training. The results showed that their approach enabled the robot to learn to complete its tasks with a much smaller number of constraint violations than state-of-the-art baselines. Additionally, they were able to achieve several orders-of-magnitude better sample efficiency when compared with constrained model-free RL approaches.
CASRL: Robots that can learn to adapt to current conditions
To further address how robots can navigate safely in typical warehouse environments where people and other robots are moving freely — or what researchers call nonstationary disturbances — the group employed the use of a Context-Aware Safe Reinforcement Learning(opens in new window) (CASRL) method, a meta-learning framework in which the robots can learn how to safely adapt to nonstationary disturbances as they occur.
In addition to workers or other robots moving around a warehouse, the CARSL method would enable the robots to learn how to safely navigate other situations that could include inaccurate sensor measurements, broken robot parts or obstructions such as trash in the environment. The team also applies CARSL to manipulation of tools and interaction with humans, which can be directly applied to assembly in manufacturing.
"Nonstationary disturbances are everywhere in real-world applications, providing infinite variations of scenarios. An intelligent robot should be able to generalize to unseen cases rather than just memorize the examples provided by humans. This is one of the ultimate challenges in trustworthy AI," said Zuxin Liu, a third year Ph.D. student at the Safe AI Lab(opens in new window) at CMU, supported by the MFI award.
Zhao explained that the robot must learn to determine whether the previously trained planning policies are still suitable for the current situation. The robot updates policy based on the recent local observations in an online training fashion, so that it could be easily adapted to new situations with unseen disturbances, while also guaranteeing safety with high degree of probability. Given the past several minutes/seconds sensing data, the robot can automatically infer and model the potential disturbances based on the data and update the planning policy. Zhao's team further extends the method to task-agnostic online enforcement learning, which can continuously learn to solve unseen tasks with online reinforcement learning that not only is able to adapt to unseen, yet similar tasks, but also to identify and learn to solve distinct tasks.
In each of these studies, the new models and methods improved upon prior ways of training robots to move about safely and effectively in new and changing environments. Such incremental steps are essential to achieving the goal of a verifiable level of trustworthiness required for better warehouse robots.
The team will continue working on the deployment of manufacturing logistics and assembly manipulation. Zhao will also work on a new project funded by MFI on generating safety and security-critical digital twins/metaverse, which will be a critical tool in the development of trustworthy intelligent manufacturing robots.
"The next generation of manufacturing is now." said Zhao.
Zhao's robotic research was also funded by the Pennsylvania Infrastructure Technology Alliance(opens in new window).
— Related Content —
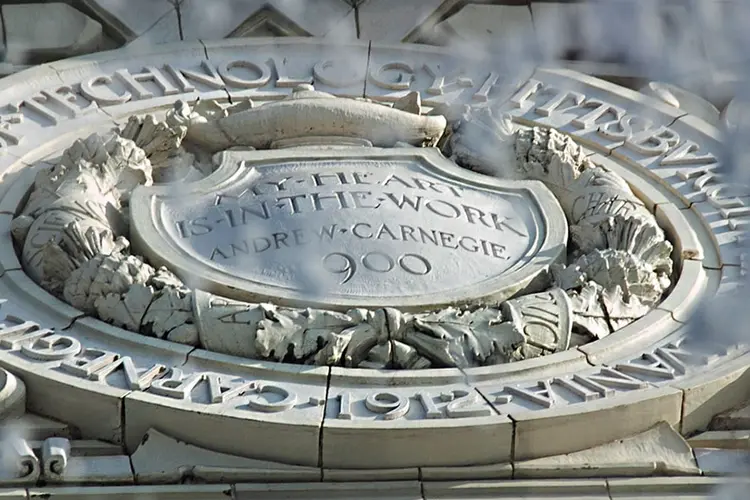
NSF 2021 CAREER College of Engineering Awardees Named
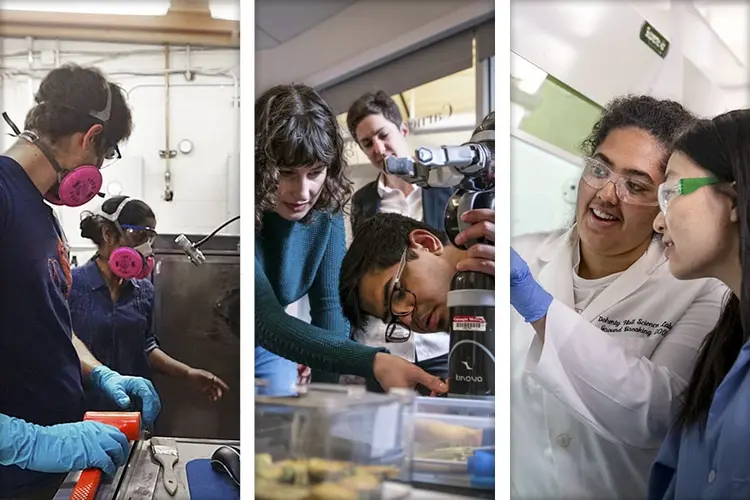