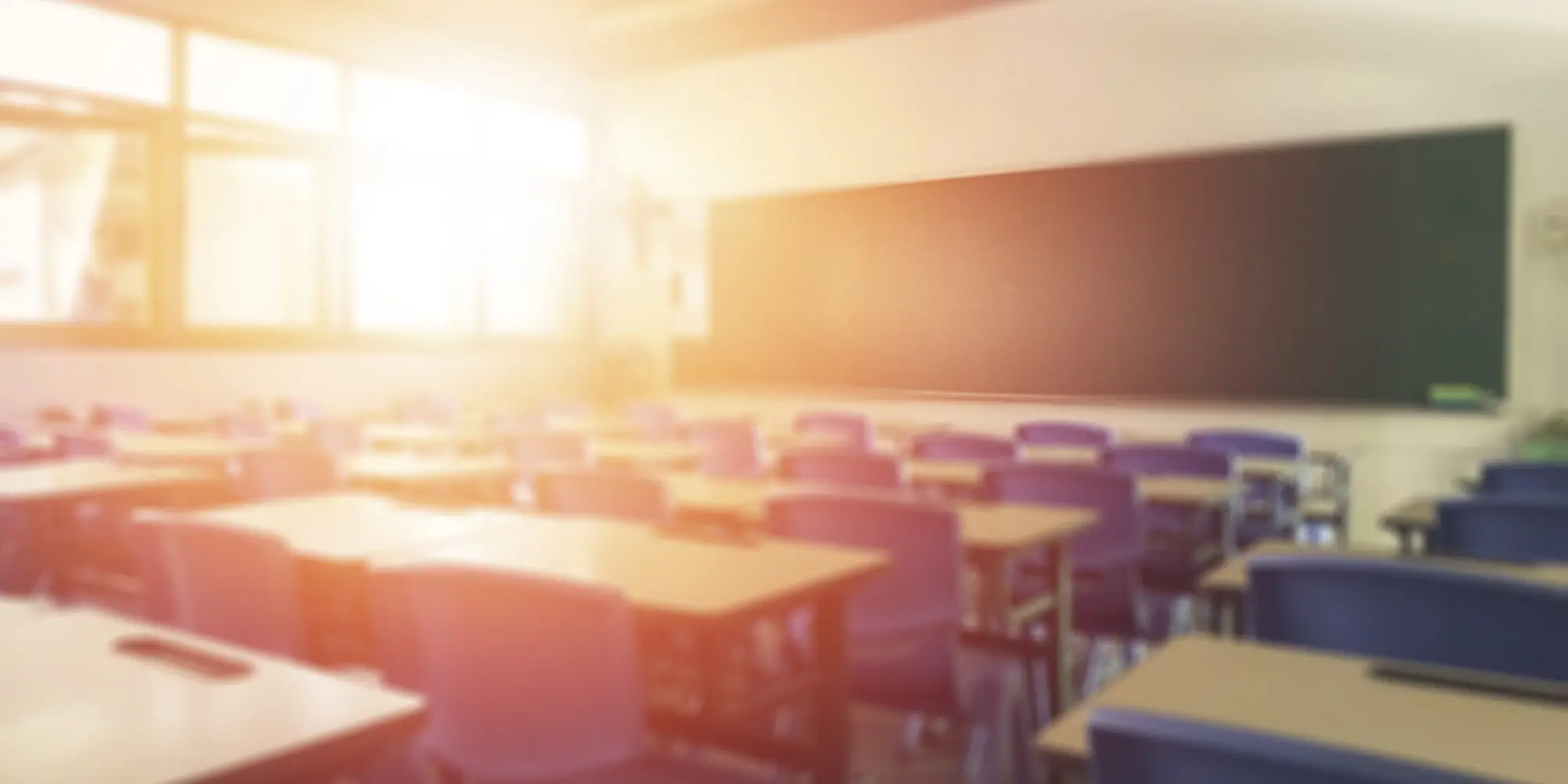
The Future of Classroom Experimentation
Media Inquiries
With access to some of the best digital tools and learning systems ever seen, it's a wonder that there is currently no easy way for teachers to conduct experiments to see what is working best in their classrooms. Carnegie Mellon University and its partners were recently awarded a nearly $3 million National Science Foundation grant to fund a new framework for adaptive experimentation in classrooms and digital learning spaces like CMU's Open Learning initiative(opens in new window) (OLI) and the Carnegie Learning K-12 platform(opens in new window).
In the following Q&A, John Stamper(opens in new window), an associate professor at the Human-Computer Interaction Institute(opens in new window) and the principal investigator (PI) on the project, expands on what his team of multidisciplinary researchers hopes to achieve. This interview has been edited and condensed.
Q. Why is it important for teachers to be able to do experiments?
A. Teachers of all kinds, from K-12, to college, to informal adult education instructors, already conduct experiments every day when they try different approaches to see what resonates with their students. We want to make it easy for them in a data-driven way that works with the learning platform they are already using.
Q. Why is it hard?
A. First, there is no one tool to facilitate this. We are creating a tool called EASI, pronounce it like "easy." That stands for "Experiments as a Service Infrastructure," an open-source back-end software that anyone will be able to use with their learning systems.
Second, it takes a lot of time. One of the most important things in any classroom is to use students' time wisely. In traditional experimentation, often called A/B testing, you assign students to one group or another — and of course one condition is going to be better than the other, so you've just assigned half of your students to the bad condition. We use adaptive experimentation, which gets students into the better condition faster.
Q. What is adaptive experimentation?
A. In A/B testing, each condition is typically given a similar number of participants. But in adaptive experimentation, the conditions adapt in real time as the experiment progresses. So, the better conditions, the ones that are working, get more participants. And that means better results and student outcomes. In addition, we can use machine learning to speed up scientific discovery, by testing more hypotheses at once, and putting more emphasis on the promising ideas.
Q. What if what works best on average isn't best for a particular student?
A. A key part of EASI is allowing tools for experimentation to be used for personalization. For example, imagine if the experimental data suggests explanation A is good for students who got the last problem wrong, and explanation B is good for students who got it right. EASI can personalize by delivering explanation A to students who got the last problem right, and explanation B to students who got it wrong. EASI enables sophisticated machine learning algorithms to personalize automatically but keeps human judgment in the loop by letting instructors and scientists see the data and interact with or override the algorithms.
Q. What does this look like in the classroom?
A. Let's say we want to have students learn geometry concepts. We would start with four groups. We would ask the first group of students to read some text and solve a set of problems; another group of students would watch a video first; a third group of students would be asked just to solve the problems without instruction; and a fourth group would be shown a completed problem and asked to solve a similar one.
We want to know which of those activities works best for our students. If we have a lot of participants, we can very quickly begin to see what's working for them. We can move the students from a group that isn't working into one that is, so no one is left behind.
Q. Your co-PIs come from very different backgrounds. Why is that important?
A. Joseph Jay Williams(opens in new window) (assistant professor at the University of Toronto) directs the Intelligent Adaptive Interventions lab, which bridges human-computer Interaction, psychology, statistics, and machine learning. This allowed them to provide the EASI component of a software framework (MOOClet) that allows for multidisciplinary scientists to collaborate on A/B experimentation, personalization, and crowdsourcing. Aaditya Ramdas(opens in new window) (assistant professor in CMU's Statistics and Machine Learning departments) is an expert on algorithms and tests for adaptive experimentation. Steven Ritter (founder and chief scientist at Carnegie Learning) has been a long-term partner with CMU, especially in trying to make experimentation easier. Jeffrey Carver(opens in new window) (professor at the University of Alabama) works on helping scientists use software tools to do research and will help us evaluate how EASI brings teams together to advance science in a way that improves the student experience. Norman Bier(opens in new window) (director of the Open Learning Initiative and the executive director of the Simon Initiative(opens in new window) at CMU) is an expert in learning engineering and the OLI platform, and has been using data to improve educational systems my entire career.
Q. How does this support learning engineering?
A. Learning engineering(opens in new window), the way we design and build learning environments, is an iterative process. In some ways, you could call what we are doing iterative experimentation, because as people are entering the experiment they get assigned based off past results. It's an opportunity to improve your experiments in real time — you don't have to start over with a new experiment to get better results, you can test more hypotheses than traditional experiments, accelerating science by automatically emphasizing the promising interventions.
Q. How can people get involved?
A. We will be making these tools available to teachers, psychological and learning scientists, statisticians, and machine learning researchers, so they can design, deploy and analyze data from these experiments, and test out different algorithms. We will also provide training and onboarding sessions for researchers and practitioners. If you're interested, email John(opens in new window) or Joseph(opens in new window).