Alison L. Barth
Maxwell H. and Gloria C. Connan Professor in the Life Sciences
Address:
159C Mellon Institute
Department of Biological Sciences
Carnegie Mellon University
4400 Fifth Avenue
Pittsburgh, PA 15213
Phone: 412-268-1198
Fax: 412-268-8423
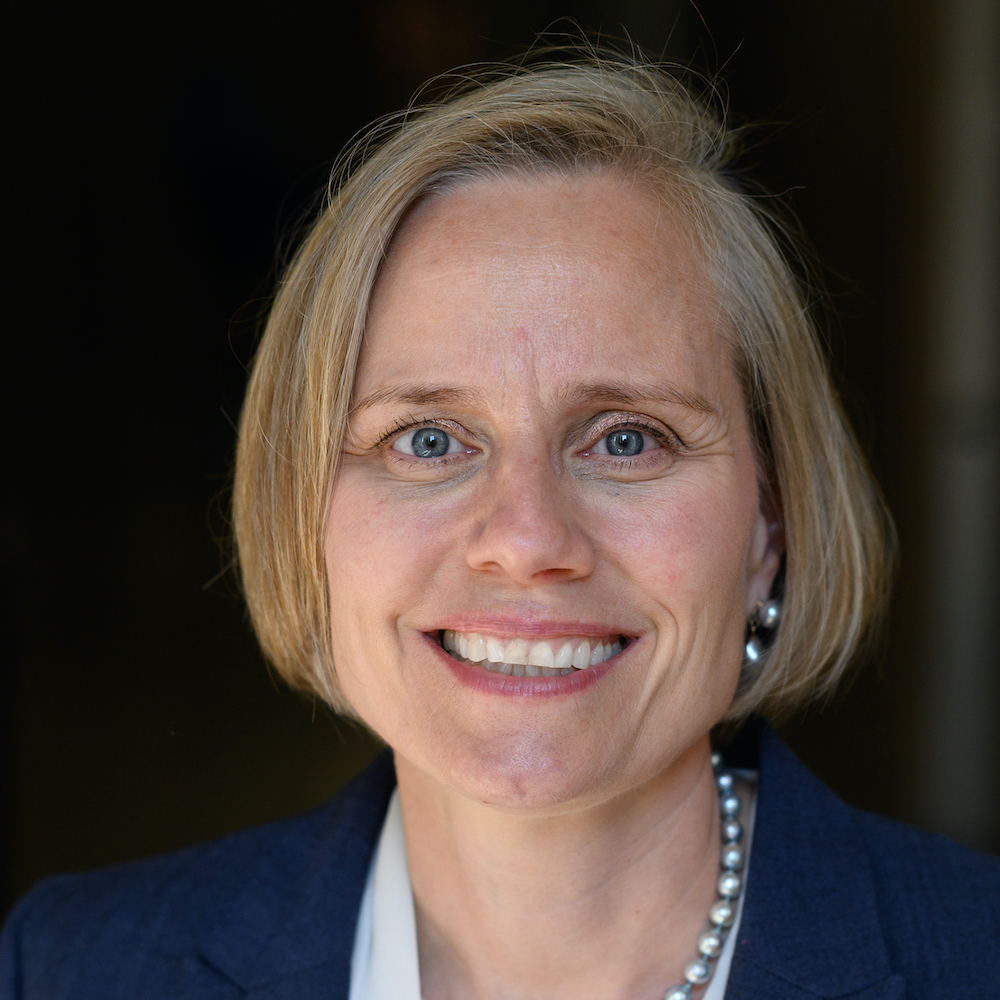
Education
Postdoctoral Appointment, Stanford University School of Medicine
Research
How does experience shape the brain?
Research in the Barth Lab is focused on understanding how experience assembles and alters the properties of neural circuits in the cerebral cortex, in both normal and disease states. The lab has a specific focus on somatosensation in the mouse model system, where specific types of sensory input from the skin are used to drive neural activity to change the strength of synaptic connections and the firing output of cortical neurons. This neural plasticity can result in enhanced perceptual capabilities and influence subsequent learning. A detailed examination of how synapses are changed by experience is revealing fundamental principles about both perception and learning across many neural systems. In addition, researchers in the lab are using electrophysiological recordings, electron microscopy, and computational modeling to understand how functional networks are constructed and optimized in the neocortex. Experiments take advantage of transgenic mice to manipulate gene expression and label defined neural subsets and whole-cell recording and imaging to quantitate the electrical properties of cortical neurons. Ongoing projects include:
1. Algorithms for learning
How is information from the external world transformed by cortical circuits during both normal sensory processing as well as adaptively, during learning? Decades of research have focused on neural decoding – a stimulus focused-approach to estimate the probability or properties of a sensory stimulus from the pattern of spikes collected from increasingly large populations of neurons. In contrast, we are interested in neural algorithms: the small-scale, laminar and translaminar computations deployed by defined subtypes of neocortical neurons in the receipt and transfer of sensory input. This bottom-up approach seeks to identify biologically-grounded procedures by which neurons transform inputs to outputs, constrained by deep knowledge about the identity of molecularly-distinct subsets of neurons connected through highly-specified networks. Our experimental data drive predictions about how these processes can be altered during different brain states, such as attention, reward, or fatigue, as well as how they enable experience-dependent plasticity. Using state-of-the-art genetic tools for pathway-specific activation and cell-type specific electrophysiological recordings, we are developing insight into the low- and high-level computations that are carried out by increasingly large assemblies of neurons. We have identified critical cellular and synaptic control points, embedded in the 6-layered architecture of the circuit, that enable the cortical algorithm to adapt and change during behaviorally-relevant training. The sequence of these changes – which synapses, on which cells, in which layers - shed light onto how this neocortical circuit has become specialized for plasticity. Insights from biological systems can thus provide inspiration for engineered circuits for artificial intelligence.
2. High-throughput, fluorescence-based methods for synapse detection and connectomics
Anatomical methods for determining cell-type specific connectivity are essential to inspire and constrain our understanding of neural circuit function across development, during learning, and in disease states. We have developed new genetically-encoded reagents for fluorescence-synapse labeling and connectivity analysis in brain tissue that are designed for high-throughput, compartment-specific localization of synapses across diverse neuron types in the mammalian brain. High-resolution confocal image stacks of virally-transduced neurons can be used for 3D reconstructions of postsynaptic cells, automated detection of synaptic puncta, and multichannel fluorescence alignment of dendrites, synapses, and presynaptic neurites to assess cell-type specific connectivity. We are using these fluorescence-based reagents to quantitatively evaluate changes in synaptic connectivity during learning and in mouse models of neurological disorders such as Alzheimer’s and Parkinson’s disease. The vast number of fluorescently-labeled, input- and target-specified synapses we are collecting offers new and exciting opportunities for data analysis and machine learning.
3. Network assembly and optimization using principles of neural design
Neurons within the neocortex are connected in stereotyped ways to generate complex but reproducible patterns of activity. We are interested in how these principles enable effective information transfer and network plasticity. We are working with computer scientists to identify and adapt biological principles into engineered networks to inspire new architectures for information storage, working memory, and learning.
Publications
Barth, R.F., Buja, L.M., Barth, A.L., Carpenter, D.E., Parwani, A.V. A Comparison of the Clinical, Pathologic, Virologic, and Immunologic Features of SARS, MERS, and COVID-19 Viral Diseases. (2021) Archives of Pathology & Laboratory Medicine Oct 1;145(10):1194-1211. doi: 10.5858/arpa.2020-0820-SA.
Park, E., Audette, N.J. and Barth, A.L. Learning-dependent enhancement of persistent activity in the neocortex. (2021) 9th International Conference on Learning Representations (ICLR), Vienna Austria May 2021, Workshop Track Proceedings.
Kuljis, D.A., Micheva, K.D., Wegner, W., Bowman, R., LaBranche, M., Madison, D.V., Willig, K.I., and Barth, A.L. Gephyrin-lacking PV synapses on neocortical pyramidal neurons. (2021) International Journal of Molecular Sciences. Sep 17;22(18):10032. doi: 10.3390/ijms221810032.
Bernhard, S.M., Lee, J., Erskine, A., Hires, S.A. and Barth, A.L. Automated home-cage training drives sensory association learning. (2020) PLoS One Dec 2;15(12):e0232916. doi: 10.1371/journal.pone.0232916.
Bernhard, S.M., Lee, J., Zhu, M., Hsu, A., Erskine, A., Hires, S.A., and Barth, A.L. (2020). An automated homecage system for multiwhisker detection and discrimination learning in mice. BioRxiv 2020.04.27.063750.
Kuljis, D.A., Park, E., Myal, S.E., Clopath, C., and Barth, A.L. (2020). Transient and layer-specific reduction in neocortical PV inhibition during sensory association learning. BioRxiv 2020.04.24.059865.
Litvina, E., Adams, A., Barth, A.L., Bruchez, M.P., Carson, J., Dupre, K.B., Frank, L.M., Gates, K.M., Harris, K.M., Joo, H., Lichtman, J.W., Ramos, K.M., Sejnowski, T., Trimmer, J.S., White, S., Koroshetz, W. BRAIN Initiative: Cutting-edge tools and resources for the community. (2019) Journal of Neuroscience 16 October 39(42):8275-8284. doi: 10.1523/JNEUROSCI.1169-19.2019
Barth, A.L. and Ray, A. Progressive circuit changes during learning and disease. (2019) Neuron 9 October 104(1):37-46. doi: 10.1016/j.neuron.2019.09.032
Kuljis, D.A., Park, E., Telmer, C.A., Lee, J., Ackerman, D.S., Bruchez, M.P. and Barth, A.L. Fluorescence-based quantitative synapse analysis for cell-type specific connectomics. (2019) eNeuro 23 September. doi: 10.1523/ENEURO.0193-19.2019
Audette, N.J., Bernhard, S. M., Ray, A., Stewart, L.T., and Barth A.L. Rapid plasticity of higher-order thalamocortical inputs during sensory learning. Neuron. 2019 Jul 17;103(2):277-291.e4. doi: 10.1016/j.neuron.2019.04.037.
Reddy, J.W., Kimukin, I., Ahmed, Z., Stewart, L., Barth, A.L., Towe, E. and Chamanzar, M. High density, double-sided, flexible optoelectrical neural probes with embedded micro-LEDs. Frontiers in Neuroscience, 09 August https://doi.org/10.3389/fnins.2019.00745
Kuljis D.A., Zemoura K, Telmer C, Lee J, Park E, Xu W, Bruchez MP, and Barth AL. Quantitative synapse analysis for cell-type specific connectomics. BioRXiv, August 7, 10.1101/386912.
Beukema P, Cecil KL, Peterson E, Mann VR, Matsushita M, Takashima Y, Navlakha S, and Barth AL. TrpM8-mediated somatosensation in mouse neocortex. Journal of Comparative Neurology, Jun 15, 526(9):1444-1456.
Navlakha S, Bar-Joseph Z, and Barth AL. Network Design and the Brain. Trends in Cognitive Science, 22 Jan 2018.
Urban-Ciecko J, Jouhanneau JS, Myal SE, Poulet JFA, and Barth AL. Precisely Timed Nicotinic Activation Drives SST Inhibition in Neocortical Circuits. Neuron, 7 Feb 2018, 97(3): 611-625.
Pratt CP, Kuljis DA, Homanics G, Dudem S, Hollywood MA, Barth AL, Bruchez MP. Tagging of endogenous BK channels with a fluorogen-activating peptide reveals β4-mediated control of channel clustering in cerebellum. Front Cell Neurosci., 31 Oct 2017, 11:337.
Lee J, Barth AL. Constructing the external world. Neuron, 21 Jun 2017, 94(6): 1048-1050.
Audette NJ, Urban-Ciecko J, Matsushita M, Barth AL. POm thalamocortical input drives layer-specific microcircuits in somatosensory cortex. Cerebral Cortex, 10 Mar 2017, 1-17.
Urban-Ciecko, J. and Barth A.L. Somatostatin neurons in cortical networks (2016) Nature Reviews Neuroscience 17:401–409.
Ye, L., Allen, W.F., Thompson, K.R., Tian, Q., Hsueh, B., Ramakrishnan, C., Wang A.-C., Jennings, J., Adhikari, A., Witten, I.B., Barth, A.L., Luo, L. Halpern C.H., McNab, J., and Deisseroth, K. Long-range wiring and molecular signatures of prefrontal cortex neuronal populations mediating positive or negative valence experience. (2016) Cell Jun 16;165(7):1776-88.
Barth A., Burkhalter A., Callaway E.M., Connors B.W., Cauli B., DeFelipe J., Feldmeyer D., Freund T., Kawaguchi Y., Kisvarday Z ., Kubota Y., McBain C., Oberlaender M., Rossier J., Rudy B., Staiger J.F., Somogyi P., Tamas G., Yuste R. Comment on "Principles of connectivity among morphologically defined cell types in adult neocortex". (2016) Science Sep 9;353(6304):1108.
Chandrasekaran, S., Navlakha, S., Audette, N.J., McCreary, D., Souhan, J., Bar-Joseph, Z., and Barth, A.L. Unbiased, high-throughput analysis of experience-dependent changes in synaptic density in somatosensory cortex. (2015) Journal of Neuroscience Dec 16;35(50):16450-16462.
Pratt, C.P., He, J., Wang, Y., Barth, A.L., and Bruchez, M.P. Fluorogenic Green-Inside Red -Outside (GIRO) labeling reveals kinase-dependent control of BKalpha surface expression. (2015) Bioconjugate Chemistry Sep 16;26(9):1963-71.
Cai, X., Huang, H., Kuzirian, M.S., Snyder, L.M., Matsushita, M., Lee, M.C., Furguson, C., Homanics, G.E., Barth, A.L., and Ross, S.E. Generation of a KOR-Cre knockin mouse strain to study cells involved in kappa opioid signaling (2015) Genesis Nov 17. doi: 10.1002/dvg.22910. [Epub ahead of print]
Navlakha, S., Barth, A.L.*, and Bar-Joseph, Z.* Synapse pruning rates optimize construction of efficient and robust networks. (2015) PLoS Computational Biology July 28; 11(7): e1004347. *indicates co-corresponding authors
Urban-Ciecko, J., Fanselow, E.E., and Barth, A.L. Neocortical somatostatin neurons reversibly silence excitatory transmission via GABAb receptors (2015) Current Biology Mar 16;25(6):722-31.
Glazewski, S. and Barth, A.L. Stimulus intensity determines experience-dependent modifications in neocortical neuron firing rates. (2015) European Journal of Neuroscience Feb;41(4):410-9.
Urban-Ciecko, J., Wen, J.A., Parekh, P.K., and Barth, A.L. Experience-dependent regulation of presynaptic NMDARs enhances neurotransmitter release at neocortical synapses. (2014) Learning and Memory Dec 15;22(1):47-55.
Jouhanneau, J.S., Ferrarese, L., Estabanez L., Audette N., Brecht, M., Barth, A.L., and Poulet, J.F.A. Cortical cfos expression reveals broad receptive field excitatory neurons targeted by POm. (2014) Neuron Nov 13;84(5):1065-1078.
Czajkowski, R., Jayaprakash, B., Wiltgen, B., Rogerson, T., Karlsson, M.G., Barth, A.L., Trachtenberg, J., and Silva, A.J. Encoding and storage of spatial information in the retrosplenial cortex (2014) PNAS Jun 10;111(23):8661-6
Barth, A.L. and Kuhlman, S.J. The many layers of specification and plasticity in the neocortex. (2013) Neuron 79(5): 829-831.
Navlakha, S., Suhan, J., Barth, A.L., and Bar-Joseph, Z. A high-throughput machine learning framework to detect synapses in electron microscopy images. (2013) Proc. of the 21st Intl. Conf. on Intelligent Systems for Molecular Biology and 12th European Conf. on Computational Biology (ISMB/ECCB). Bioinformatics. Jul 1;29(13):i9-17.
Wen, J.A. and Barth, A.L. Initiation, labile, and stabilization phases of experience-dependent plasticity at neocortical synapses. (2013) Journal of Neuroscience 33(19):8483-93.
Benedetti, B.L.*, Takashima, Y.*, Wen, J.A., Urban-Ciecko, J., and Barth, A.L. Asymmetric wiring of layer 2/3 neurons drives sparse and reliable firing during neocortical development. (2012) Cerebral Cortex Aug 23. [Epub ahead of print] *these authors contributed equally to this work.
Poulet, J.F.A. and Barth, A.L. Experimental evidence for sparse firing in the neocortex. (2012) Trends in Neurosciences, Jun;35(6):345-55.
Wen, J.A. and Barth A.L. Synaptic lability after experience-dependent plasticity is not mediated by calcium-permeable AMPARs. (2012) Frontiers in Molecular Neuroscience 2012;5:15, epub 2012 Feb 29.
Shruti, S., Urban-Ciecko, J, Fitzpatrick, J., Brenner, R., Bruchez, M., and Barth, A.L. The brain-specific beta 4 subunit downregulates BK channel cell surface expression. (2012) PlosOne 2012;7(3):e33429, epub Mar 16.
Barth, A.L. AC-1 and synaptic development. (2012) Journal of Physiology 1;590(Pt 1):3.
Ly, C., Melman, T. Barth, A.L. and Ermentrout, G.B. Phase-resetting curve determines how BK currents affect neuronal firing. (2011) Journal of Computational Neuroscience Apr;30(2):211-23.
Wen, J.A. and Barth, A.L. Input-specific critical periods for experience-dependent plasticity in layer 2/3 pyramidal neurons. (2011) Journal of Neuroscience 23;31(12):4456-65.
Yassin, L., Benedetti, B.L., Jouhanneau, J.-S., Wen, J.A., Poulet, J.F.A. and Barth A.L. An embedded subnetwork of highly active neurons in the neocortex. (2010) Neuron 68(6):1043-50.