CONSEQUENTIAL
Consequential is a podcast that looks at the human side of technological change and develops meaningful plans of action for policymakers, technologists and everyday people to build the kind of future that reduces inequality, improves quality of life and considers humanity. In its third season, Consequential will examine how AI and machine learning will impact research practices and data collection, as well as the development and dissemination of knowledge. Topics will include combatting disinformation, the ethics of crowdsourced research, and representation in open source software development.
Host: Lauren Prastien, Eugene Leventhal
Season 3, Episode 9 - Is information democratized?
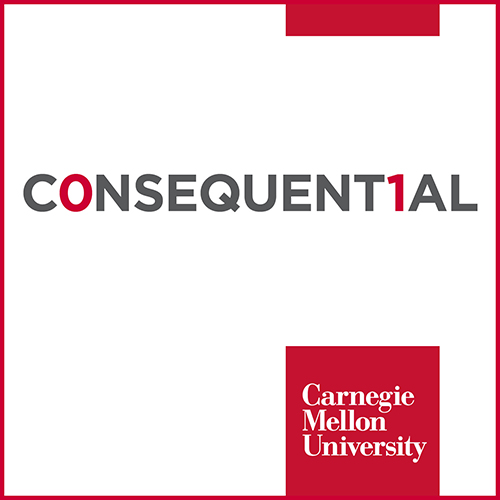
Season 3
Consequential Season 3 Trailer
In Season 3 of Consequential, hosts Eugene and Lauren will be exploring knowledge production in the Information Age. Beginning on October 21, this season will examine how AI and machine learning will impact research practices and data collection, as well as the development and dissemination of knowledge. Topics will include combatting disinformation, the ethics of crowdsourced research, and representation in open source software development.
Episode 1: Knowledge production and the bias pipeline: The story of the EEG
In the first episode of Season 3 of Consequential, hosts Eugene and Lauren look at how underlying biases in the development of the EEG have impacted healthcare, medical technology, and scientific research, with guests Ben Amaba, Arnelle Etienne, Pulkit Grover, and Shawn Kelly.
Episode 2: If banning bots won't stop disinformation, what will?
Disinformation is as old as the printing press, if not older. So what has accelerated its spread now, and what can be done to stop it? On this special bonus episode of Consequential, we speak to the experts about disinformation, the election, and COVID-19. This week's guests are Congressman Mike Doyle and Professor Kathleen Carley.
Episode 3: Is Crowdsourcing the Answer to our Data Diversity Problem?
Traditional scientific research has a data diversity problem. Online platforms, such as Mechanical Turk, give researchers access to a wider variety and greater volume of subjects, but they are not without their issues. Our hosts are joined by experts David S. Jones, Ilka Gleibs, and Jeffrey Bigham to discuss the pros and cons of knowledge production using crowdsourced data.
Episode 4: Can Automation Make Peer Review Faster and Fairer?
Peer review is the backbone of research, upholding the standards of accuracy, relevance and originality. However, as innovation in the fields of AI and machine learning has reached new heights of productivity, it has become more difficult to perform peer review in a fast and fair manner. Our hosts are joined by Nihar Shah to unpack the question of automation in the scientific publication process: could it help, is it happening already, and what does it have in common with the job application process?
Episode 5: Enron, Wikipedia and the Deal with Biased Low-Friction Data
The Enron emails helped give us spam filters, and many natural language processing and fact-checking algorithms rely on data from Wikipedia. While these data resources are plentiful and easily accessible, they are also highly biased. This week, we speak to guests Amanda Levendowski and Katie Willingham about how low-friction data sources contribute to algorithmic bias and the role of copyright law in accessing less troublesome sources of knowledge and data.
Episode 6: Is the presence of a human enough to regulate an AI decision-making system?
From helping to identify tumors to guiding trading decisions on Wall Street, artificial intelligence has begun to inform important decision-making, but always with the input of a human. However, not all humans respond the same way to algorithmic advice. This episode of Consequential looks at human-in-the-loop AI, with guests Sumeet Chabria, David Danks, and Maria De-Arteaga.
Episode 7: Why does open source have such a wide gender gap?
Open source software is the infrastructure of the Internet, but it is less diverse than the tech industry overall. In this deep-dive on gender in open source, we speak to CMU’s Laura Dabbish and Anita Williams Woolley about what’s keeping women from participating in open source software development and how increased participation benefits society as a whole.
Episode 8: Language, Power and NLP
Natural language processing is the branch of artificial intelligence that allows computers to recognize, analyze and replicate human language. But when it’s hard enough for humans to say what they mean most of the time, it’s even harder for computers to get it right. Even when they do, we might not like what we hear. In this week’s episode looks at sentiment analysis, search engine prediction, and what AI and human language can teach us about each other, with guests Alvin Grissom II of Haverford College and Alexandra Olteanu of Microsoft Research.
Episode 9: Is information democratized?
In the age of the Internet, a lot of information is at our fingertips. But is it accessible, reliable and up-to-date? In the season finale of Consequential, we're discussing information inequality with guests Asia Biega, Stephen Caines, and Myeong Lee.
Season 2
Consequential Season 2 Trailer
In light of recent developments related to COVID-19, we have decided to push back our second season to focus instead on what we can learn from the coronavirus in terms of technology and society. In our mini-season, we will cover the use of large-scale public health data, remote education, and the future of work.
Episode 1: Pandemics, Public Data and Privacy
Mobile data records, tracking devices and government-mandated selfies have played a large role in both enforcing quarantines and providing data to better understand the coronavirus. In this week’s episode of Consequential, hosts Eugene and Lauren examine the importance of collecting and using data for public health, the individual privacy concerns that arise as a result of this data collection, and the challenges of striking a balance between societal benefit and personal privacy. This episode is part one of a two-episode look on large-scale public health data analytics.
In this episode:
- Wilbert Van Panhuis, Assistant Professor of Epidemiology and Bioinformatics, University of Pittsburgh
- Tom Mitchell, University Professor of Computer Science and Machine Learning, Carnegie Mellon University
- Scott Andes, Executive Director of the Block Center for Technology and Society, Carnegie Mellon University
Episode 2: Sorry, Your Phone Says You Have Anxiety
How will certain new standards for data sharing and surveillance during the COVID-19 pandemic impact the future of healthcare? In episode two of Consequential's two-part deep-dive on pandemics, public health and privacy, hosts Eugene and Lauren examine the impact of big data on health and privacy.
In this episode:
- David S. Jones, A. Bernard Ackerman Professor of the Culture of Medicine, Harvard University
- Henry Kautz, Division Director for Information & Intelligent Systems, the National Science Foundation
- Tom Mitchell, University Professor of Computer Science and Machine Learning, Carnegie Mellon University
- Wilbert Van Panhuis, Assistant Professor of Epidemiology and Bioinformatics, University of Pittsburgh
- Scott Andes, Executive Director of the Block Center for Technology and Society, Carnegie Mellon University
Episode 3: How Will COVID-19 Change Higher Ed?
In the span of just two weeks, the entire American higher education system moved online due to COVID-19. While this is often considered a temporary measure, the truth is that higher ed may never fully go back to normal. And in some regards, we may not want it to. In this week’s episode, hosts Eugene and Lauren talk to professors across the United States about the future of higher education.
In this episode:
- Pedro Ferreira, Associate Professor Of Information Systems, Carnegie Mellon University
- Michael D. Smith, Professor of Information Technology And Marketing, Carnegie Mellon University
- Inez Tan, Academic Coordinator and Lecturer, the University of California at Irvine
- Julian Silverman, Assistant Professor of Chemistry and Biochemistry, Manhattan College
- Eric Yttri, Assistant Professor of Biological Sciences, Carnegie Mellon University
- Brian E. Herrera, Associate Professor of Theater, Princeton University
- Jessie Male, Faculty, New York University and the Ohio State University
Episode 4: Death by a Thousand Emails
Can teams still be effective when working together remotely? Is working from home the future of work? In this week’s episode, hosts Eugene and Lauren talk to Professor Anita Williams Woolley of Carnegie Mellon’s Tepper School of Business to learn about how communication and collaboration change once teams are no longer face-to-face, and we hear from people in a variety of fields about their experience working remotely.
In this episode:
- Anita Williams Woolley, Associate Professor of Organizational Behavior and Theory, Carnegie Mellon University
Episode 5: How Do You Reopen A State?
Today we're asking our experts: how do you coordinate a crisis response to an issue like COVID-19, where every public health decision has economic ramifications, and every economic decision has a direct impact on public health?
In this episode:
- Richard Stafford, Distinguished Service Professor, Carnegie Mellon University
- Ramayya Krishnan, Dean of the Heinz College of Information Systems And Public Policy and William W. and Ruth F. Cooper Professor of Management Science and Information Systems, Carnegie Mellon University
- Rayid Ghani, Distinguished Career Professor of Machine Learning Department, Information Systems and Public Policy, Carnegie Mellon University
Season 1
This is Consequential
Our future isn’t a coin flip. In an age of artificial intelligence and increasing automation, Consequential looks at our digital future and discusses what’s significant, what’s coming and what we can do about it. Over the course of our first season, hosts Lauren Prastien and Eugene Leventhal will unpack the narrative of technological change in conversation with leading technologists, ethicists, economists and everything in between.
Episode 1: Disruption Disrupted
Are the robots coming for your job? The answer isn’t quite that simple. We look at what’s real and what’s hype in the narrative of industry disruption, how we might be able to better predict future technological change and how artificial intelligence will change our understanding of the nature of intelligence itself.
In this episode:
- Lee Branstetter, Professor Of Economics And Public Policy, Carnegie Mellon University
- Anita Williams Woolley, Associate Professor of Organizational Behavior and Theory, Carnegie Mellon University
Episode 2: The Black Box
Inside the black box, important decisions are being made that may affect the kinds of jobs you apply for and are selected for, the candidates you’ll learn about and vote for, or even the course of action your doctor might take in trying to save your life. However, when it comes to figuring out how algorithms make decisions, it’s not just a matter of looking under the hood.
In this episode:
- Kartik Hosanagar, Professor of Operations, Information and Decisions, The Wharton School of the University of Pennsylvania
- Molly Wright Steenson, Senior Associate Dean for Research, Carnegie Mellon University
Episode 3: Data Subjects and Manure Entrepreneurs
Every time you order a shirt, swipe on a dating app or even stream this podcast, your data is contributing to the growing digital architecture that powers artificial intelligence. But where does that leave you? In our deep-dive on data subjects, we discuss how to better inform and better protect the people whose data drives some of the most central technologies today.
In this episode:
- Kartik Hosanagar, Professor of Operations, Information and Decisions, The Wharton School of the University of Pennsylvania
- Tae Wan Kim, Associate Professor of Business Ethics, Carnegie Mellon University
Episode 4: Fair Enough
Everyone has a different definition of what fairness means - including algorithms. As municipalities begin to rely on algorithmic decision-making, many of the people impacted by these AI systems may not intuitively understand how those algorithms are making certain crucial choices. How can we foster better conversation between policymakers, technologists and communities their technologies affect?
In this episode:
- Jason Hong, Professor of Human Computer Interaction, Carnegie Mellon University
- Molly Wright Steenson, Senior Associate Dean for Research, Carnegie Mellon University
- David Danks, Professor of Philosophy and Psychology, Carnegie Mellon University
Episode 5: Bursting the Education Bubble
Big data disrupted the entertainment industry by changing the ways that people develop, distribute and access content, and it may soon do the same for education. New technologies are changing education, both within and beyond the classroom, as well as opening up more accessible learning opportunities. However, without reform in our infrastructure, this ed-tech might not reach the people who need it the most.
In this episode:
- Michael Smith, Professor Of Information Technology And Marketing, Carnegie Mellon University
- Pedro Ferreira, Associate Professor Of Information Systems, Carnegie Mellon University
- Lauren Herckis, Research Scientist, Carnegie Mellon University
- Lee Branstetter, Professor Of Economics And Public Policy, Carnegie Mellon University
Episode 6: Staying Connected
If you think about any piece of pop culture about the future, it takes place in a city. Whether we realize it or not, when we imagine the future, we picture cities, and that idea is all the more problematic when it comes to who benefits from technological change and who does not. This episode will look at how emerging technologies can keep communities connected, rather than widen divides or leave people behind.
In this episode:
- Richard Stafford, Distinguished Service Professor, Carnegie Mellon University
- Karen Lightman, Executive Director - Metro21, Carnegie Mellon University
- Douglas G. Lee, President, Waynesburg University
Episode 7: A Particular Set of Skills
The World Economic Forum has found that while automation could eliminate 75 million jobs by 2022, it could also create 133 million new jobs. In this episode, we will look at how to prepare potentially displaced workers for these new opportunities. We will also discuss the “overqualification trap” and how the Fourth Industrial Revolution is changing hiring and credentialing processes.
In this episode:
- Liz Shuler, Secretary-Treasurer, AFL-CIO
- Craig Becker, General Counsel, AFL-CIO
- Oliver Hahl, Assistant Professor of Organizational Theory and Strategy, Carnegie Mellon University
- Lee Branstetter, Professor Of Economics and Public Policy, Carnegie Mellon University
Episode 8: The Future of Work
If artificial intelligence can do certain tasks better than we can, what does that mean for the concept of work as we know it? We will cover human-AI collaboration in the workplace: what it might look like, what it could accomplish and what policy needs to be put in place to protect the interests of workers.
In this episode:
- Parth Vaishnav, Assistant Research Professor of Engineering and Public Policy, Carnegie Mellon University
- Aniruddh Mohan, Graduate Research Assistant, Carnegie Mellon University
- Liz Shuler, Secretary-Treasurer, AFL-CIO
- Craig Becker, General Counsel, AFL-CIO
- Tom Mitchell, University Professor of Computer Science and Machine Learning, Carnegie Mellon University
Episode 9: Paging Dr. Robot
Don’t worry, your next doctor probably isn’t going to be a robot. But as healthcare tech finds its way into both the operating room and your living room, we’re going to have to answer the kinds of difficult ethical questions that will also determine how these technologies could be used in other sectors. We will also discuss the importance of more robust data-sharing practices and policies to drive innovation in the healthcare sector.
In this episode:
- David Danks, Professor of Philosophy and Psychology, Carnegie Mellon University
- Zachary Chase Lipton, Assistant Professor of Business Technologies and Machine Learning, Carnegie Mellon University
- Adam Perer, Assistant Research Professor of Human-centered Data Science, Carnegie Mellon University
- Tom Mitchell, University Professor of Computer Science and Machine Learning, Carnegie Mellon University
Episode 10: A Policy Roadmap
Over the last 9 episodes, we’ve presented a variety of questions and concerns relating to the impacts of technology, specifically focusing on artificial intelligence. To end season 1, we want to take a step back and lay out a policy roadmap that came together from the interviews and research we conducted. We will outline over 20 different steps and actions that policymakers can take, starting with laying the necessary foundations to applying regulatory frameworks from other industries to novel approaches.
TRANSCRIPTS
Consequential Season 1 Trailer
Lauren Prastien: In 2017, a team of researchers found that there is a 50 percent chance that artificial intelligence or AI will outperform humans in all tasks, from driving a truck to performing surgery to writing a bestselling novel, in just 45 years. That’s right. 50 percent. The same odds as a coin flip.
But the thing is, this isn’t a matter of chance. We aren’t flipping a coin to decide whether or not the robots are going to take over. And this isn’t an all or nothing gamble.
So who chooses what the future is going to look like? And what actions do we need to take now - as policymakers, as technologists, and as everyday people - to make sure that we build the kind of future that we want to live in?
Hi, I’m Lauren Prastien.
Eugene Leventhal: And I’m Eugene Leventhal. This is Consequential. We’re coming to you from the Block Center for Technology and Society at Carnegie Mellon University to explore how robotics, artificial intelligence and other emerging technologies can transform our future for better or for worse.
Lauren Prastien: Over the course of this season, we’re going to be looking at hot topics in tech:
Molly Wright Steenson: Well, I think a lot of things with artificial intelligence take place in what could call it gets called the black box.
Lauren Prastien: We’ll speak to leaders in the field right now about the current narrative of technological disruption:
Tom Mitchell: It's not that technology is just rolling over us and we have to figure out how to get out of the way. In fact, policymakers, technologists, all of us can play a role in shaping that future that we're going to be getting.
Lauren Prastien: And we’ll look at the policy interventions necessary to prepare for an increasingly automated and technologically enhanced workplace:
Anita Williams Woolley: So if we want to prepare our future workforce to be able to compliment the rise and the use of technology, it's going to be a workforce that's been well-versed in how to collaborate with a wide variety of people.
Eugene Leventhal: Along the way, we’ll unpack some of the concepts and challenges ahead in order to make sure that we build the kind of future that reduces inequality, improves quality of life and considers humanity. Because we’re not flipping a coin. We’re taking action.
This is Consequential: what’s significant, what’s coming and what we can do about it.
Follow us on Apple Podcasts or wherever you’re listening to this. You can email us directly at consequential@cmu.edu. To learn more about Consequential and the Block Center, you can check out our website at cmu.edu/block-center or follow us on Twitter @CMUBlockCenter.S1 E1: Disruption Disrupted
Lauren Prastien: So, maybe you’ve noticed that a lot of things have started to look a little different lately.
By which I mean, the other day, a good friend of mine called me to tell me that a robot had started yelling at her at the grocery store. Apparently, this robot was wandering up and down the aisles of the grocery store and suddenly, it blocks the entire aisle to announce,
Eugene Leventhal, as robot: “Danger detected ahead.”
Lauren Prastien: And she proceeds to text me a picture of this, as she put it, “absolute nightmare Roomba” because she wasn’t really sure what to do.
And when I asked her if I could use this story, she proceeded to tell me: “Lauren, I was at the craft store the other day, and as I was leaving, the store spoke to me.” There was this automated voice that said,
Eugene Leventhal, as robot: “Thank you for shopping in the bead section.”
Lauren Prastien: As in, as soon she left the bead section, the store knew and wanted to let her know that they were happy she stopped by. And, by the way, she hated this.
But this isn’t a podcast about how my friend has been hounded by robots for the past few weeks or even about the idea of a robot takeover. And it’s not only about the people those robots might have replaced, like the grocery store employee that would normally be checking the aisles for spills or the greeter at the door of the craft store. And it’s not a podcast saying that it’s time for us to panic about new technologies or the future, because by the way, we’ve always freaked out about that. Socrates was afraid a new technology called writing things down would make everyone forgetful and slow because we wouldn’t memorize things anymore. Ironically, we know this because Plato, Socrates’ student, wrote this down in his dialogue, the Phaedrus.
This podcast is actually about how the world is changing around us and the role that technology, specifically Artificial Intelligence or AI is playing in those changes. It’s about understanding the potential consequences, both good and bad. It’s about how you have played a central role in the development of these technologies and that you deserve a seat at the table when it comes to the role that these technologies are playing in our society and in your everyday life.
This is Consequential: what’s significant, what’s coming, and what we can do about it. I’m Lauren Prastien and I’ll be your main tour guide along this journey. You’ll also hear the voices of our many guests as well as your other host.
Eugene Leventhal: Hi, I’m Eugene Leventhal. I’ll be joining throughout the season to take a step back with Lauren and overview what was just covered, to talk policy, and to read quotes. I’ll pass it back to you now Lauren.
Lauren Prastien: Consequential is recorded at the Block Center for Technology and Society at Carnegie Mellon University. Established in 2018 through a generous gift from Keith Block, the Block Center is dedicated to investigating the economic, organizational, and public policy impacts of emerging technologies.
Over the course of this season, we’re going to talk about how a lot of the institutions and industries that we’ve previously thought unchangeable are changing, how the technologies accompanying and initiating these changes have become increasingly pervasive in our lives, and how both policymakers and individuals can respond to these changes.
In this first episode, we’ll start our journey by talking about some of the major changes humanity has witnessed in recent generations, about what intelligence means in an age of automation, and why all this AI-enabled technology, like algorithms, self-driving cars, and robots, will require more thoughtful and intentional engagement between individuals as a foundation to deal with these coming changes.
Our question today is “How can we disrupt the narrative of industry disruption?”
Lee Branstetter: The problem of course, is that we need to design public policies that can help cushion the disruption if it's going to come. But we need to have those policies in place before disruption has become a really major thing. So, you know, how do we get visibility on where this technology is actually being deployed and what its labor market effects are likely to be? Well our idea, and I think it's a pretty clever one, is to actually use AI to study this question.
Lauren Prastien: That and more when we return.
So, one hundred years ago, everyone was getting really worried about this relatively new, popular technology that was going to distract children from their schoolwork, completely ruin people’s social lives and destroy entire industries.
This was the phonograph. That’s right. People were scared that record players were going to destroy everything.
By the 1910s, the phonograph had given rise to a lot of new trends in music, such as shorter songs, which people thought would make our mental muscles flabby by not being intellectually stimulating enough. Record players also got people into the habit of listening to music alone, which critics worried would make us completely antisocial. One of the most vocal critics of the phonograph was John Philip Sousa, who you probably know for composing all those patriotic marches you hear on the Fourth of July. One hundred years ago, Sousa was worried that the phonograph – or as he called it, the “talking machine” – would disincentivize children from learning music and as a result, we’d have no new musicians, no music teachers and no concert halls. It would be the death of music.
That’s right: A lot of people were genuinely worried that the record player was going to destroy our way of life, put employees out of work, make us all really disconnected from each other and completely eliminate music making as we knew it. Which is really kind of funny when you consider a certain presidential candidate has been talking about how record players could bring us all back together again.
So we’ve always been a little ambivalent about technology and its capacity to radically change our lives for better or for worse. If we look at past interpretations of the future, they’re always a little absurd in hindsight. The campiness of the original Star Trek, the claustrophobia and anxiety of Blade Runner. Sometimes, they’re profoundly alarmist. And sometimes, they’re ridiculously utopian. Often, they’re predicated on this idea that some form of technology or some greater technological trend is either going to save us or it’s going to completely destroy us.
One of the most dramatic examples of us feeling this way was Y2K.
Twenty years ago, it was 1999, and we were preparing for a major technological fallout. This was when Netflix was only two years old, and it was something you got in the mail. People were terrified that computers wouldn’t be able to comprehend the concept of a new millennium. As in, they wouldn’t be smart enough to know that we weren’t just starting the 1900s over, and as a result, interest rates would be completely messed up, all the powerplants would implode and planes would fall out of the sky. Which, you know, none of that really happened. In part because a lot of people worked really hard to make sure that the computers did what they were supposed to do.
But while 1999 didn’t deliver on Y2K, it was the year that Napster hit the web. So, while the world may not have ended for you and me, it did end for the compact disc.
The new millennium saw us worrying once more about the death of the music industry. But we’re at a point now where we can see that this industry didn’t die. It changed. The phonograph didn’t kill music, and neither did Napster. In 2017, U.S. music sales hit its highest revenue in a decade. While not a full recovery from its pre-Napster glory days, paid subscription services like Spotify and Apple Music have responded to the changing nature of media consumption in such a way that has steered a lot of consumers away from piracy and kept the music industry alive.
This isn’t to say that the changing nature of the music industry didn’t put people out of work and companies out of business – it absolutely did. We watched a seemingly unsinkable industry have to weather a really difficult storm. And that storm changed it irrevocably.
The thing is, this is what technology has always done. In a recent report, the AFL-CIO’s Commission on the Future of Work and Unions noted:
Eugene Leventhal: “Technology has always involved changing the way we work, and it has always meant eliminating some jobs, even as new ones are created.”
Lauren Prastien: But in this report, the AFL-CIO emphasizes that this shouldn’t solely be viewed as an organic process. It’s something that needs to be approached with intentionality.
Today, we’re seeing those kinds of transformations happen much more swiftly and at a much higher frequency. But how do we know where those transformations are going to take place or what those transformations are going to look like?
Heads up – we’re not great at this.
Fifty years ago, it was 1969. The year of Woodstock, the Moon landing, Nuclear Nonproliferation and the Stonewall Riots. A US stamp cost just 6 cents.
This was the year a certain Meredith W. Thring, a professor of mechanical engineering at Queen Mary College, testified before the International Congress of Industrial Design in London. He was there to talk about the future, and Eugene’s going to tell us what he had to say about it:
Eugene Leventhal: “I do not believe that any computer or robot can ever be built which has emotions in it and therefore, which can do anything original or anything which is more sophisticated than it has been programmed to do by a human being. I do not believe it will ever be able to do creative work.”
Lauren Prastien: By creative work, Professor Thring meant cooking.
He believed that no robot would look like a person, which would make it easier for us to dehumanize them and, in his words, enslave them, and that their designs would be purely functional. Thring imagined robots would have eyes in the palms of their hands and brains between their toes. Or, in the case of an agricultural robot, a large, roving eye at the front of the tractor, angled to down the ground. A quick Google of the term “automated cooking,” will show you just how wrong our friend Meredith W. Thring was when it came to robots capable of preparing meals.
So if our own imaginations aren’t sufficient to understand where disruption is going to occur, what could be? There’s a group of researchers here at the Block Center who came up with an interesting way to measure just much AI disruption might be coming – patents.
Lee Branstetter: Now, of course, not all AI inventions are going to be patented, but if you've got something fundamental that you think is going to make you billions of dollars and you don't patent at least part of it, you're leaving yourself open to the possibility that somebody else is going to patent that thing and get the billion dollars instead of you.
Lauren Prastien: That was Lee Branstetter, a Professor of Economics and Public Policy at Carnegie Mellon. He also leads the Future of Work Initiative here at the Block Center, where his work focuses on the economic forces that shape how new technology is created, as well as the economic and social impacts of those new technologies.
Lee Branstetter: Once we can identify these AI patents, we know the companies that own them. We often know something about the industry in which they're being deployed. We know when the invention was created, even who the inventors are and when we know who the inventing firms are, we can link the patent data to data maintained by other sources, like the US Census Bureau.
Lauren Prastien: Combined with employment data, this patent data offers a really useful window into how this technology is being developed and deployed.
Lee Branstetter: And one of the most interesting pieces of data is the so called LEHD Dataset, the longitudinal employer household dynamics dataset. This is essentially a matched employer-employee dataset. We can observe the entire wage distribution of firms and how they're changing as AI technology is developed and deployed within the firm.
Lauren Prastien: When Eugene and I spoke to Professor Branstetter, we wanted to get a better idea of what industry disruption might actually look like and exactly who it was going to impact. Because right now, there are a lot of conflicting opinions out there about what exactly is going to happen to the concept of work as we know it.
Lee Branstetter: Everybody's already heard the sort of extreme positions that are being propagated in the media and on social media, right? On the one hand, there are the techno utopians who tell us that a life of endless leisure and infinite wealth, uh, is almost within our grasp. And then there are the technical dystopians, right? Who will tell us that the machines are going to take all of our jobs.
So one of my concerns is that AI is not going to render human labor obsolete, but it's going to exacerbate the trends that we've been seeing for decades, right? It's going to amplify demand for the highest skilled workers and it's going to weaken demand for the lower skilled workers. Well, with our data, we could actually match AI, patent data and other data to data on the entire wage distribution of firms and see how it evolves and see where and when and in what kind of industry these effects are starting to emerge and that can help inform public policy. All right? We can kind of see the leading edge of this disruption just as it's starting to happen. And we can react as policy makers.
Lauren Prastien: Professor Branstetter believes that being able to react now and take certain preemptive measures is going to be a critical part of being able to shape the narrative of disruption in this new age of artificial intelligence. Because even if it seems that everything has suddenly come together overnight: a robot cleaning the aisle in a grocery store, a robot thanking you for shopping in the bead section - this isn’t some kind of hostile robot takeover or sudden, unstoppable tide of change that we’re helpless to let wash over us. The fact is that this is all still relatively new.
Lee Branstetter: All of the debate, uh, is basically taking place in a virtual absence of real data. Means these technologies are still in their very early stages. You know, we're just starting along a pathway that is likely to take decades over which these technologies probably are going to be deployed in just about every sector of the economy. But we really don't know yet what the effect is.
Lauren Prastien: While the economic realities don’t point a massive change just yet, there are plenty of reasons to believe that more change is coming. Though only time will tell the exact extent and who will be impacted the most, the fact is that the increasing pace of technological change is very likely to lead to some large-scale changes in society. Our job will be to dig into what is real and what is hype, and what needs to be done so that we’re prepared for the negative outcomes.
Lee Branstetter: The problem of course, is that we need to design public policies that can help cushion the disruption if it's going to come. But we need to have those policies in place before disruption has become a really major thing. So, you know, how do we get visibility on where this technology is actually being deployed and what its labor market effects are likely to be?
Well our idea, and I think it's a pretty clever one, is to actually use AI to study this question. So I've been working with Ed Hovy who is a major scholar in the Language Technologies Institute of the School Computer Science. Um, he's an expert in using machine learning algorithms to parse text. And so together with one of his graduate students and a former patent attorney that is now getting two PhDs at Carnegie Mellon, uh, we're actually teaching an ensemble of machine learning algorithms to parse patent text and figure out on the basis of the language and the text whether this invention is AI related or not.
Lauren Prastien: That’s right. Professor Branstetter is using robots to fight the robots, in a manner of speaking.
But if we take a step back, there are some signs that certain industries are already being completely restructured or threatened. As an example: ride-hailing apps, like Uber and Lyft, have disrupted an industry previously considered to be un-disruptable: taxi services. So even as we use technologies like Professor Branstetter’s patent analysis to cushion the blow of technological change, we’re still going to see industries that are impacted, and as Professor Branstetter warned us, this could really exacerbate existing inequalities.
There’s another, more promising idea that these technologies could really help promote shared prosperity by breaking down the barriers to economic success. But for every company that implements a robot to do a task like, say, clean an aisle, so that that employee can do more human-facing, less-routinized work, there’s going to be a company that just implements a robot without finding new work for an existing employee. And so being able to shape how these technologies impact our way of life is going to take some real work. Real work that starts at the personal level, starting with the simple act of caring more about this in the first place and extending to working with governments, universities, and corporations to make the digitally-enabled future one that’s better for everyone.
Because just anticipating this disruption is only half the battle. Later on this season, we’re going to get into some of the specific policy interventions that could protect individuals working in disrupted industries and help them transition to new careers, like wage insurance and reskilling initiatives.
As we prepared the interviews that went into this season, we realized that the topic of tech as a tool kept coming up, and reasonably so. The sound of using AI or robots to enhance our human abilities sounds like we’re in some sci-fi movie, though I’m sure that’s not the only reason researchers look into it. But these tools aren’t infallible: they’re going to see the world with the same biases and limitations as their creators. So thinking technology can somehow make the underlying problems that people are concerned with go away is kind of unrealistic.
As technologies continue to evolve at ever faster rates, one of the things you’ll hear mentioned throughout the season are the human challenges. It’s important to consider that technology in and of itself is not useful – it is only helpful when it actually solves problems that we humans have. And these technologies have the potential to do a lot of good, from helping to save lives by improving diagnosis to making the workplace safer by aiding in the performance of difficult physical tasks to opening up new opportunities through remote work, online learning and open-source collaboration. Sometimes, disruption is a good thing. But we can’t lose the human factor or simply allow these technologies to bulldoze right through us.
If anything, as these technologies become more complex, that means that we get to delve into increasingly more complex topics related to being human. You may have heard this new little catchphrase that EQ, or emotional intelligence, is the new IQ, or how robots are only going to make the things that make us human all the more significant.
Anita Williams Woolley: And so this really suggests that school funding models that take resources away from the activities that foster teamwork and foster social interaction in favor of you know, more mathematics for example, will really be shortchanging our children and really our economy.
Lauren Prastien: We’re going to talk a little more about that in just a moment, so stay tuned.
In his book AI Superpowers: China, Silicon Valley and the New World Order, computer scientist and businessman Kai-Fu Lee looks at the story of AlphaGo versus Ke Jie. In 2017, Ke Jie was the reigning Go champion. Go is a strategy board game where two players try to gain control of a board by surrounding the most territory with their game pieces, or stones. It is considered to be one of the oldest board games in human existence, invented in China during the Zhou dynasty and still played today. Back during antiquity, being able to competently play Go was considered one of the four essential arts of a Chinese Scholar, along with playing a stringed instrument, calligraphy, and painting.
So, in May of 2017, Ke Jie, the worldwide Go champion, arranged to play against a computer program called AlphaGo. They played for three rounds, and AlphaGo won all of them. Which, in the battle for human versus robot, might seem really discouraging.
But of this defeat, Kai-Fu Lee, as read by Eugene, wrote:
Eugene Leventhal: “In that same match, I also saw a reason for hope. Two hours and fifty-one minutes into the match, Ke Jie had hit a wall. He’d given all that he could to this game, but he knew it wasn’t going to be enough. Hunched low over the board, he pursed his lips and his eyebrow began to twitch. Realizing he couldn’t hold his emotions in any longer, he removed his glasses and used the back of his hand to wipe tears from both of his eyes. It happened in a flash, but the emotion behind it was visible for all to see. Those tears triggered an outpouring of sympathy and support for Ke. Over the course of these three matches, Ke had gone on a roller-coaster of human emotion: confidence, anxiety, fear, hope, and heartbreak. It had showcased his competitive spirit, but I saw in those games an act of genuine love: a willingness to tangle with an unbeatable opponent out of pure love for the game, its history, and the people who play it. Those people who watched Ke’s frustration responded in kind. AlphaGo may have been the winner, but Ke became the people’s champion. In that connection – human beings giving and receiving love – I caught a glimpse of how humans will find work and meaning in the age of artificial intelligence.”
Lauren Prastien: Like Kai-Fu Lee, I don’t want to believe that this is a matter of us versus them. I also believe in that glimpse that he describes, and I think that glimpse is something we call emotional intelligence.
But to really understand how emotional intelligence and other forms of human intelligence are going to keep us from being automated out of existence, we’re going to have to understand what we mean by intelligence. Breaking down the idea of human intelligence is another subject for a different podcast from someone far better-equipped to handle this stuff. But let’s use a really basic working definition that intelligence is the ability to acquire and apply knowledge or skills.
A lot of the time when we talk about intelligence, we think about this as the individual pursuit of knowledge. But as the nature of our workplace changes with the influx of these new technologies, we’re going to see an emphasis on new kinds of intelligence that can compete with or even complement artificial intelligence. And one of these is collective intelligence.
Anita Williams Woolley: Collective intelligence is the ability of a group to work together over a series of problems. We really developed it to compliment the idea of individual intelligence, which has historically been measured as the ability of an individual to solve a wide range of problems.
Lauren Prastien: That’s Anita Williams Woolley. She is a Professor of Organizational Behavior and Theory at Carnegie Mellon University. She’s used collective intelligence to look at everything from how to motivate people to participate in massive open-source collaborations like Wikipedia to explaining how the September 11th attacks could have been prevented with better communication and collaboration.
Anita Williams Woolley: In order for a group to be able to work together effectively over a range of different kinds of problems, they really need different perspectives, different information, different skills. And you can't get that if everybody is the same. And so it’s not the case that a high level of diversity automatically leads to collective intelligence. There needs to be some other behaviors, some other communication behaviors and collaboration behaviors that you need to see as well.
It's, it's not necessarily how individually intelligent people are, but the skills that they bring that foster collaboration as well as again, the diversity of, of different skills. So in terms of collaboration skills, initially what we observed was that having more women in the team led to higher collective intelligence over time we found more of a curvilinear effect.
Lauren Prastien: Real quick, curvilinear means that if there’s two variables, they’re going to both increase together at the same rate for a little while, but then at some certain point, while one variable keeps increasing, the other starts decreasing. Think of it as the “too much of a good thing” relationship. So, in the case of having women in a group, the curvilinear effect looked something like this. If a group had no women, there wasn’t very high collective intelligence. Sorry, guys. And as more and more women are added to a group, the collective intelligence of that group increases. But to a point. A group with majority women participants is going to have really high collective intelligence, but if a group is entirely women, collective intelligence is actually going to be a little lower than it would be if there were also some men in the group. It’s also really important to quickly clarify why this is. It’s not that women are magic. I mean, we are. But Professor Woolley has a more sociological explanation for why women participants boost a group’s collective intelligence.
Anita Williams Woolley: So one of the reasons why having more women helps teams is because women on average tend to have higher social perceptiveness than men. However, that said, if an organization is really doing a lot of collaboratively intensive work, if they focus on hiring people who have higher levels of social skills, whether they're male or female, it should enhance the ability of their teams to be more collectively intelligent.
Lauren Prastien: But creating a strong collectively intelligent group isn’t just a matter of gender. Professor Woolley has found that this trend extends to other forms of diversity as well.
Anita Williams Woolley: So we've looked at gender diversity, we've looked at some ethnic diversity. In both cases we find that you, you know, there is a benefit to both sorts of diversity for collective intelligence, but specifically we also find a benefit for cognitive diversity. And it's the cognitive styles that we look at are styles that tend to differentiate people who go into different academic fields. And so there's a cognitive style that's predominant in the humanities, one that's predominant in engineering and the sciences, one that's predominant in the visual arts. And we find that at least a moderate of cognitive diversity along these cognitive styles is best for collective intelligence. So trying to create organizations, create teams that are diverse in these ways is going to lead to higher collective intelligence.
Lauren Prastien: So what does this have to do with contending with artificial intelligence and automation? Partially, it’s to say that we’re not going to succeed in managing these technologies if we keep trying to prop up exemplary individuals to compete with them. One of Professor Woolley’s studies showed that a team of regular people with strong communication skills handled a simulated terrorist attack better than actual counterterrorism experts. That is, until those experts participated in a communication seminar.
But the more important point here is that one of the best ways to leverage these new technologies is not to look at how they can replace us, but to understand how they can complement the things we’re already great at.
Anita Williams Woolley: I think it's important to keep in mind the distinction between production technologies and collaboration technologies. So when you think about a robot who's just going to do your job for you, that would be an example of a production technology where they're actually doing the task. And that's usually what people call to mind if they think about AI coming to take their job. However, the bigger possibility and actually the one that is potentially very positive for many of us is a coordination technology, which is where the robots come and they help us coordinate our input so that they get combined more effectively. So that we don't have you know, gaps or people doing, you know, the same work or you know, other coordination losses that you often see in organizations.
Lauren Prastien: Professor Woolley’s research has shown that sometimes, people can really struggle when it comes to articulating what they’re good at or when they have to allocate tasks among a team. But that doesn’t mean that our future managers and mentors are going to be robots.
Anita Williams Woolley: You'd be willing to have a machine tell you, oh, the most of you know, the best time for you to have this meeting is at this time because that's when everybody is available. Okay, fine, I'll do that. But am I going to take career advice or life advice, you know, from this robot?
So we have done some studies. We're starting to work now on a new program looking at AI-based coaches for task performance. And so in some of the pilot studies we were interested in how do humans perceive these coaches and do they find them as competent, as warm, you know, do they want to work with them? And the answer is no. So if the same if, if a performer was getting the same advice but thought it was from a human, they thought it was much more competent and credible than if they thought it was from a bot.
Lauren Prastien: Professor Woolley proposes that artificial intelligence could help coordinate people to more effectively tackle challenges and derive more value from the work they do. Because ultimately, while there’s work that technology may be able to do slightly better than we do – there’s a lot of stuff that technology simply cannot match us in. It’s the stuff that made us root for Ke Jie, even when he was losing to AlphaGo. Especially when he was losing to AlphaGo.
And it’s the kind of stuff that makes us feel kind of nice when a human thanks us for shopping in the bead section and feel really unnerved when a robot does it. There are going to be the machines that beat us at games, the machines that lay bricks more efficiently than we do and the machines that write up contracts faster that we can. But what both Kai-Fu Lee and Professor Woolley are arguing is that machines cannot take away the things that make us innately human. If anything, they can help enhance them.
But it’s not going to happen organically. According to Professor Woolley, it’s going to take some interventions in policy and education.
Anita Williams Woolley: I think focusing on the education policy is a big piece of this. Traditionally in the last few decades in the United States, we focused a lot on STEM education and mathematics. And, and related fields. And those are important. But what we see as we look at the economy and also look at you know, where wages are rising, it's in those occupations and in fields where you both need technical skill but also social skill. And so this really suggests that school funding models that take resources away from the activities that foster teamwork and foster social interaction in favor of you know, more mathematics for example, will really be shortchanging our, our children and really our economy.
Lauren Prastien: It’s really important to stress this shifting nature of intelligence, and the fact that this isn’t the first time we’ve seen this. Since the Industrial Revolution, the proliferation of new technologies has continuously emphasized the value of science, math, and engineering education, often to the detriment of the arts and the humanities. Now, we are seeing many issues related to technology that center around a lack of social education. As tech increases our ability to communicate freely and more tasks become automated, we have to start placing an emphasis on skills that have been relatively undervalued as of late.
Anita Williams Woolley: Especially as we get more and more technologies online that can take over some of the jobs that require mathematical skill, that's going to increase the value of these social skills even more. So if we want to prepare our future workforce to be able to compliment the rise and the use of technology, it's gonna be a workforce that's been well versed in how to collaborate with a wide variety of people and that's best accomplished in a school setting.
Lauren Prastien: If used correctly, technology can help us achieve more than we may be able to without it. But can we disrupt disruption? So Eugene, we talked to some experts this week. What do you think?
Eugene Leventhal: Well, Lauren, the fact is that technology isn’t some unstoppable force that we are powerless to lose our jobs and sense of worth to. But ensuring that disruption doesn’t exacerbate existing inequalities means taking steps to anticipate where this disruption may occur and determining how to best deploy these technologies to enhance human work, rather than to replace it. It also means providing adequate support through education and other avenues to strengthen and reinforce the skills that make us innately human. And so where does our journey take us from here?
Lauren Prastien: In the coming episodes, we will discuss the increasing influence of emerging technologies, concerns of algorithmic bias, potential impacts on social and economic inequality, and what role technologists, policymakers and their constituents can play in determining how these new technologies are implemented, evaluated and regulated.
In the next episode of Consequential, we’ll talk about the AI black box: what is it, why is it important, and is it possible to unpack it? Here’s a snippet from Molly Wright Steenson, a Professor of Ethics & Computational Technologies here at CMU, who’s going to join us next week:
Molly Wright Steenson: Some people say that an AI or a robot should be able to say what it's doing at any moment. It should be able to stop and explain what it's done in what its decision is. And I don't think that's realistic.
Lauren Prastien: I’m Lauren Prastien.
Eugene Leventhal: And I’m Eugene Leventhal.
Lauren Prastien: And this was Consequential. We’ll see you next week.
Consequential was recorded at the Block Center for Technology and Society at Carnegie Mellon University, which was established to examine the societal consequences of technological change and create meaningful plans of action. To learn more about Consequential, the Block Center and our faculty, you can check out our website at cmu.edu/block-center or follow us on Twitter @CMUBlockCenter. You can also email us at consequential@cmu.edu.
This episode of Consequential was written by Lauren Prastien, with editorial support from Eugene Leventhal. It was edited by Eugene and our intern, Ivan Plazacic. Consequential is produced by Eugene, Lauren, Shryansh Mehta and Jon Nehlsen.
This episode uses a clip of John Philip Sousa’s High School Cadets march, a portion of the AFL-CIO Commission on the Future of Work and Unions’ report to the AFL-CIO General Board and an excerpt of Kai-Fu Lee’s AI Superpowers: China, Silicon Valley and the New World Order.
S1 E2: The Black Box
Lauren Prastien: So, you might know this story already. But bear with me here.
In 2012, a teenage girl in Minneapolis went to Target to buy some unscented lotion and a bag of cotton balls. Which, okay. Nothing unusual there. She was also stocking up on magnesium, calcium and zinc mineral supplements. Sure, fine – teenagers are growing, those supplements are good for bones and maintaining a healthy sleep schedule. But here’s where things get strange – one day, Target sent her a mailer full of coupons, which prominently featured products like baby clothes, formula, cribs. You know, things you might buy if you’re pregnant. Yeah, when I got to this point in the story the first time I heard it, I was cringing, too.
Naturally, an awkward conversation ensued because, you guessed it, Target had figured out that this teenage girl was pregnant before her own parents did.
Or, I should say, an algorithm figured out. It was developed by statistician Andrew Pole. In a partnership with Target, Pole pinpointed twenty-five products that, when purchased together, might indicate that a consumer is pregnant. So, unscented lotion – that’s fine on its own. But unscented lotion and mineral supplements? Maybe that shopper’s getting ready to buy a crib.
It might seem unsettling but consider: we know what that algorithm was taking into account to jump to that conclusion. But what happens when we don’t? And what happens when an algorithm like that has a false positive? Or maybe even worse, what happens when we find out that there’s an algorithm making a bigger decision than whether or not you get coupons for baby products - like, say, whether or not you’re getting hired for a job - and that algorithm is using really messed up criteria to do that?
So, full disclosure: that happened. In 2018, the journalist Jeffrey Dastin broke a story on Reuters that Amazon was using a secret AI recruiting tool that turned out to be biased against job candidates that were women. Essentially, their recruiting algorithm decided that male candidates were preferable for the positions listed, and downgraded resumes from otherwise strong candidates just because they were women. Fortunately, a spokesperson for Amazon claims that they have never used this algorithm as the sole determinant for a hiring decision.
So far, this has been the only high-profile example of something like this happening, but it might not be the last. According to a 2017 study conducted by PwC, about 40% of the HR functions of international companies are already using AI, and 50% of companies worldwide use data analytics to find and develop talent. So these hiring algorithms are probably going to become more common, and we could have another scandal like Amazon’s again.
We don’t always know how artificial intelligence makes decisions. But if we want to, we’re going to have to unpack the black box.
When I say the words “black box,” you probably think of airplanes. A crash. The aftermath. An account of the things that went wrong.
But this is a different kind of black box. It’s determining whether or not you’re getting approved for a loan. It’s picking which advertisements are getting pushed to your social media timelines. And it’s making important decisions that could affect the kinds of jobs you apply for and are selected for, the candidates you’ll learn about and vote for, or even the course of action your doctor might take in trying to save your life.
This is Consequential: what’s significant, what’s coming, and what we can do about it. I’m Lauren Prastien and I’ll be your main tour guide along this journey. You’ll also hear the voices of our many guests as well as of your other host.
Eugene Leventhal: Hi, I’m Eugene Leventhal. I’ll be joining throughout the season to take a step back with Lauren to overview what was just covered, to talk policy, and to read quotes. I’ll pass it back to you now, Lauren.
Lauren Prastien: Consequential is recorded at the Block Center for Technology and Society at Carnegie Mellon University. Established in 2018 through a generous gift from Keith Block and Suzanne Kelly, the Block Center is dedicated to investigating the economic, organizational, and public policy impacts of emerging technologies.
Today, we’re going to talk about algorithmic transparency and the black box. And we’ll try to answer the question: can we - and should we - unpack the black box? But before that, we’ll need to establish what these algorithms are and why they’re so important.
Kartik Hosanagar: Really, they’re all around us, whether it’s decisions we make or others make for us or about us. They’re quite pervasive, and they’ll become even more central to decisions we’ll make going forward.
Lauren Prastien: That and more soon. Stay with us.
Kartik Hosanagar: Algorithms are all around us. When you go to an ecommerce website
like Amazon, you might see recommendations...That’s an algorithm that’s convincing you to buy certain products. Some studies show that over a third of the choices we make on Amazon are driven by algorithmic decisions.
Lauren Prastien: That’s Kartik Hosanagar. He’s a Professor of Technology and Digital Business at the University of Pennsylvania. He’s also the author of A Human’s Guide to Machine Intelligence: How Algorithms Are Shaping Our Lives and How We Can Stay in Control.
Kartik Hosanagar: On Netflix, an algorithm is recommending media for us to see. About 80% of the hours you spend on Netflix are attributed to algorithmic recommendations. And of course, these systems are making decisions beyond just products we buy and media we consume. If you use a dating app like Match.com or Tinder, algorithms are matching people and so they’re influencing who we date and marry.
Lauren Prastien: But algorithms aren’t just responsible for individual decision-making. In addition to making decisions for us, they’re also making decisions about us.
Kartik Hosanagar: They’re in the workplace. If you look at recruiting, algorithms are helping recruiters figure out who to invite for job interviews. They’re also making life and death decisions for us. So, for example, algorithms are used in courtrooms in the US to guide judges in sentencing and bail and parole decisions. Algorithms are entering hospitals to guide doctors in making treatment decisions and in diagnosis as well. So really, they’re all around us, whether it’s decisions we make or others make for us or about us. They’re quite pervasive, and they’ll become even more central to decisions we make going forward.
Lauren Prastien: We’re going to get into the implications of some of these more specific examples throughout the season, but right now, I want to focus on why it’s important that these algorithms exist in the first place, how they can actually be useful to us, and what happens when they don’t do what they’re supposed to do.
To make sure we’re all on the same page, an algorithm is a set of instructions to be followed in a specific order to achieve specific results. So technically, making a peanut butter and jelly sandwich is an algorithm. You take out your ingredients. You remove two slices of bread from the bag. You toast the bread. You open the jar of peanut butter and use the knife to apply a layer of peanut butter to the open face of one of the slices. You then open the jar of jelly and use your knife to apply a layer of jelly to the open face of the other slice. You press the peanut butter-covered side of the first slice onto the jelly-covered side of the second slice. Voila - peanut butter and jelly sandwich. A set of instructions, a specific order, specific results.
Have you ever had to do that team-building exercise where you make peanut butter and jelly sandwiches? One person gives directions, and one person has to follow the directions literally? So, if the person giving the directions forgets to say to take the bread out of the bag, the person making the sandwich has to just spread peanut butter and jelly all over a plastic bag full of bread. If you’ve ever had to write some code, only to realize you skipped a line or weren’t specific enough, you know this kind of frustration.
So, in that way - the act of getting dressed is an algorithm: you can’t put on your shoes before you put on your socks. And driving involves a pretty complicated algorithm, which we’ll talk about when we talk about autonomous vehicles in another episode.
Algorithms actually originated in mathematics - they’re how we do things like find prime numbers. The word algorithm comes from Algorismus, a 9th century mathematician whose writings helped bring algebra and the Arabic numerals - aka the numbers we use every day - to Europe. But the algorithms that we’re talking about this season are the ones that turn up in computer science. Essentially, they’re programs set up to solve a problem by using a specific input to find a specific output. If we take a step back in history, this was more or less how computing started - we made machines that were capable of receiving data and then processing that data into something we could understand.
And when it comes to AI, this still holds mostly true. Algorithms use models of how to process data in order to make predictions about a given outcome. And sometimes, how those algorithms are using the data to make certain predictions is really difficult to explain.
So, the Amazon hiring program was probably using a set of sourcing, filtering and matching algorithms that looked through a set of resumes, found resumes that exhibit certain characteristics, and selected those candidates that best matched their hiring criteria for HR to then review. It did this through a process known as machine learning, which we’ll talk about a lot this season. Essentially, machine learning is a form of artificial intelligence that uses large quantities of data to be able to make inferences about patterns in that data with relatively little human interference.
So, Amazon had about ten years of applicant resumes to work off of, and that’s what they fed to their machine learning algorithm. So the algorithm saw these were the successful resumes, these people got jobs. So, the instructions were: find resumes that look like those resumes, based on some emergent patterns in the successful resumes. And this is what machine learning algorithms are great at: detecting patterns that we miss or aren’t able to see. So, a successful hiring algorithm might be able to identify that certain je ne sais quoi that equates to a good fit with a certain job position.
In addition to finding that certain special characteristic, or, ideally, objectively hiring someone based on their experience, rather than based on biases that a human tasked with hiring might have, a hiring algorithm like Amazon’s is also useful from a pure volume perspective. As a hiring manager, you’re dealing with thousands of applicants for just a handful of spots. When it comes to the most efficient way of narrowing down the most promising applicants for that position, an algorithm can be really useful. When it’s working well, an algorithm like Amazon’s hiring algorithm would toss out someone with, say, no experience in coding software for a senior level software engineer position, and select a candidate with over a decade of experience doing relevant work.
But as you’ve seen, that can sometimes go really wrong. And not just with Amazon.
Kartik Hosanagar: And here's a technology that was tested quite extensively, uh, in lab settings and launched. And it didn't really take long for it to just go completely awry. And it had to be shutdown within 24 hours.
Lauren Prastien: That and more when we come back.
As a good friend of mine put it: the wonderful thing about artificial intelligence is that you give your model a whole bunch of latitude in what it can do. And the terrible part is that you give your model a whole bunch of latitude in what it can do.
While algorithms can pick up on patterns so subtle that sometimes we as humans miss them, sometimes, algorithms pick up on patterns that don’t actually exist. The problem with the Amazon hiring algorithm was that most of the resumes that the machine learning algorithm had to learn from were from men. So, the algorithm jumped to the conclusion that male candidates were preferable to female candidates. In the peanut butter and jelly sandwich example I gave you earlier, this is the equivalent to someone spreading peanut butter on a plastic bag full of bread. From a purely technical perspective, that algorithm was following directions and doing its job correctly. It noticed that the successful candidates were mostly male, and so it assumed that it should be looking for more men, because for some reason, male meant good fit.
But we know that that’s not how it works. You don’t also eat the bag when you eat a peanut butter and jelly sandwich. And it’s not that men were naturally better at the jobs Amazon was advertising for, it’s that tech has a huge gender problem. But an algorithm isn’t going to know that. Because algorithms just follow directions - they don’t know context.
The fact is that algorithms are, well, just following orders. And so when you put problematic data or problematic directions into an algorithm, it’s going to follow those directions correctly - for better or for worse. And we’ve seen first-hand how bad data can make these programs absolutely disastrous. Right, Professor Hosanagar?
Kartik Hosanagar: I think it was 2016, this was a chatbot called Microsoft Tay. It was launched on Twitter and the chat bot turned abusive in a matter of minutes.
Lauren Prastien: So maybe you’ve heard the story of Microsoft Tay. Or you were on Twitter when it all went down. But basically, Tay - an acronym of Thinking About You - was a chatbot designed to talk as though it were a 19-year-old American girl. It was branded by Microsoft as “the AI with zero chill.” Having once been a 19-year-old American girl with absolutely no chill, I can confirm that Tay was pretty convincing at first. In one of her earliest missives, she declared to the Internet: “i love me i love me i love me i love everyone.”
In early 2016, Microsoft set up the handle @TayandYou for Tay to interact with and learn from the denizens of Twitter. If you’ve spent more than 5 minutes on Twitter, you understand why this basic premise is pretty risky. At 8:14 AM on March 23, 2016, Tay began her brief life on Twitter by exclaiming “hellooooo world!” By that afternoon, Tay was saying stuff that I am not comfortable repeating on this podcast.
While Tay’s algorithm had been trained to generate safe, pre-written answers to certain controversial topics, like the death of Eric Garner, it wasn’t perfect. And as a lot of poisonous data from Twitter started trickling into that algorithm, Tay got corrupted. To the point that within 16 hours of joining Twitter, Tay had to be shut down.
Kartik Hosanagar: And here's a technology that was tested quite extensively in lab settings and launched. And it didn't really take long for it to just go completely awry. And it had to be shut down within 24 hours.
Lauren Prastien: At the end of the day, while Microsoft Tay was a really disturbing mirror that Twitter had to look into, there weren’t a ton of consequences. But as we learned with the Amazon hiring algorithm, there are real issues that come into play when we decide to use those algorithms for more consequential decisions, like picking a certain job candidate, deciding on a course of cancer treatment or evaluating a convicted individual’s likelihood of recidivism, or breaking the law again.
Kartik Hosanagar: And so I think it's speaks to how we need to, when we're talking about AI and, uh, really using these algorithms to make consequential decisions, we really need to be cautious in terms of how we understand the algorithms. There are limitations, how we use them what kinds of safeguards we have in place.
Lauren Prastien: But Professor Hosanagar and I both believe that this isn’t a matter of just never using algorithms again and relying solely on human judgment. Because human judgment isn’t all that infallible, either. Remember - those problematic datasets, like the male resumes that Amazon’s hiring algorithm used to determine that the ideal candidates were male, were made as a result of biased human decision-making.
As it stands, human decision-making is affected by pretty significant prejudices, and that can lead to serious negative outcomes in the areas of hiring, healthcare and criminal justice. More than that, it’s subjected to the kinds of whims that an algorithm isn’t necessarily susceptible to. You’ve probably heard that statistic that judges give out harsher sentences before lunch. Though I should say that the jury’s out - pun intended - on the whole correlation/causation of that.
When these algorithms work well, they can offset or even help to overcome the kinds of human biases that pervade these sensitive areas of decision-making.
This is all to say that when algorithms are doing what they are supposed to do, they could actually promote greater equality in these often-subjective decisions. But that requires understanding how they’re making those decisions in the first place.
Kartik Hosanagar: Look, I don't think we should become overly skeptical of algorithms and become Luddites and run away from it because they are also part of the progress that's being created using technology. But at the same time, when we give that much decision-making power and information to algorithms, we need some checks and balances in place.
Lauren Prastien: But the issue comes down to exactly how we enforce those checks and balances. In the next episode of this podcast, we’re going to get into what those checks and balances mean on the information side. But for the rest of this episode, we’re going to focus on the checks and balances necessary for decision-making. Especially when sometimes, we don’t know exactly how algorithms are making decisions.
Molly Wright Steenson: Some people say that an AI or a robot should be able to say what it's doing at any moment. It should be able to stop and explain what it's done and what it’s decision is. And I don't think that's realistic.
Lauren Prastien: Stay with us.
Molly Wright Steenson: Architecture, AI and design work together in ways that we don't talk about all the time. But also I think that with AI and design, design is where the rubber meets the road. So, the way that decisions have been made by AI researchers or technologists who work on AI-related technologies - it's decisions that they make about the design of a thing or a product or a service or something else. Those design decisions are felt by humans. And that's where design is involved.
Lauren Prastien: That’s Molly Wright Steenson, a Professor of Ethics & Computational Technologies at CMU. Her research focuses on how the principles of design, architecture and artificial intelligence have informed and can continue to inform each other. She’s the author of Architectural Intelligence: How Designers and Architects Created the Digital Landscape.
Molly Wright Steenson: Well, I think a lot of things with artificial intelligence take place in what could call it gets called the black box.
Algorithms make decisions, um, process things in a way that's opaque to most of us. So we know what the inputs are, us, the things we do that get changed into data, which we don't necessarily understand that gets parsed by an algorithm and then outcomes happen. People don't get the student loan or they don't see the really high paying jobs on their LinkedIn profile or something like that. So these decisions get made in a black box and some people say that an AI or a robot should be able to say what it's doing at any moment. It should be able to stop and explain what it's done in what its decision is. And I don't think that's realistic.
Lauren Prastien: Like I mentioned before the break, there are some real consequences to an algorithm receiving faulty data or creating a problematic pattern based on the information it receives. But when it comes to actually unpacking that black box and seeing how those decisions are made, it’s not as easy as lifting a lid and looking inside.
Molly Wright Steenson: We know with deep learning that most researchers don't even understand how the algorithms do the algorithms. And we also know that sometimes if you want to be totally transparent and you give someone way too much information, it actually makes matters worse. So Mike Anthony and Kate Crawford talk about a bunch of reasons why transparency is kind of, I don't want to say a lie, but it might, it might be harmful.
Lauren Prastien: Lately, transparency is a pretty hot topic in artificial intelligence. The idea is this: if we know what an algorithm is doing at any given point, we would be able to trust it. More than that - we could control it and steer it in the right direction. But like Professor Steenson said, there’s a lot of problems with this idea.
In their paper “Seeing without knowing: Limitations of the transparency ideal and its application to algorithmic accountability,” Mike Annony of the University of Southern California and Kate Crawford of Microsoft Research and New York University ask:
Eugene Leventhal: “Can “black boxes’ ever be opened, and if so, would that ever be sufficient?”
Lauren Prastien: And ultimately, what they find is that transparency is a pretty insufficient way to govern or understand an algorithm.
This is because while Annony and Crawford have found that while we assume,
Eugene Leventhal: “Seeing a phenomenon creates opportunities and obligations to make it accountable and thus to change it.”
Lauren Prastien: The reality is that,
Eugene Leventhal: “We instead hold systems accountable by looking across them—seeing them as sociotechnical systems that do not contain complexity but enact complexity by connecting to and intertwining with assemblages of humans and non-humans.”
Lauren Prastien: Essentially, what that means is seeing that algorithm or its underlying data isn’t the same as holding that algorithm accountable, which is ultimately the goal here.
If I look under the hood of a car, I’m going to be able to understand how that car functions on a mechanical level. Maybe. If I have the training to know how all those moving parts work. But looking under the hood of that car isn’t going to tell me how that car’s driver is going to handle a snowstorm or a deer running into the road. Which is why we can’t just look at the system itself, we have to look at how that system works within the other systems operating around it, like Annony and Crawford said.
We can look at the Target marketing algorithm from the beginning of this episode and see, yes, it’s found those products that pregnant people normally buy, and now it’s going to help them save money on those products while making some revenue for Target, so this is a good algorithm. But the second we zoom out and take a look at the larger systems operating around that algorithm, it’s really not. Because even if that algorithm has perfectly narrowed down its criteria - we can see, for instance, that it’s looking at unscented lotion and mineral supplements and cotton balls and the other twenty-two products that purchased together, usually equal a pregnant customer - it’s not taking into account the greater social implications of sending those coupons to the home address of a pregnant teenager in the Midwest. And then, wow, that’s really bad. But transparency doesn’t cover that, and no amount of transparency would have prevented that from happening.
Which is why Professor Steenson is more interested in the concept of interpretability.
Molly Wright Steenson: It's not a matter of something explaining itself. It's a matter of you having the information that you need so you can interpret what's happened or what it means. And I think that if we're considering policy ramifications, then this notion of interpretation is really, really important. As in, it's important for policy makers. It's important for lawmakers, and it's important for citizens. We want to make decisions on our own. We might not come to the same decision about what's right, but we want to be able to make that interpretation.
Lauren Prastien: When it comes to managing the black box and the role of algorithms in our lives, Professor Steenson sees this as a two-sided approach.
One side is the responsibility that lies with our institutions, such as companies and governments. And what would that look like? Technologists would be more mindful of the implications of their algorithms and work towards advancing explainability. Governments would create structures to limit the chance that citizens are adversely affected as new technologies are rolled out. And companies would find new ways of bringing more people to the table, including people who aren’t technologists, to truly understand the impacts of algorithms. This comes back to the fundamentals of design approaches taken towards artificial intelligence and tech in general.
And this brings us to the other side of the coin - us. Though this is directly linked with education, even before there is a change in how digital literacy is approached, we can start by being more engaged in our part in how these algorithms are being deployed and which specific areas of our lives they’re going to impact. And, by the way, we’ll get into what that might look like next week.
But when it comes to us, Professor Hosanagar agrees that we can’t just sit back and watch all of this unfold and hope for the best. But that doesn’t necessarily mean that we have to become experts in these technologies.
Kartik Hosanagar: If you have users who are not passive, who are actually actively engaging with the technology they use, who understand the technology they use, they understand the implications and they can push back and they can say, why does this company need this particular data of mine? Or I understand why this decision was made and I'm okay with it.
Lauren Prastien: Until there’s more public will to better understand and until there are more education opportunities for people to learn, it may be challenging to get such controls to be effectively used. Think of privacy policies. Sure, it’s great that companies have to disclose information related to privacy. But how often do you read those agreements? Just having control may be a bit of a false hope until there is effort placed around education.
So can we unpack the black box? It’s complicated. Right, Eugene?
Eugene Leventhal: It absolutely is, Lauren. As we’ve learned today from our guests, figuring out what an algorithm is doing isn’t just a matter of lifting a lid and looking inside. It’s a matter of understanding the larger systems operating around that algorithm, and seeing where that algorithm’s decision-making fits into those systems as a whole. And there’s an opportunity for policymakers, technologists and the people impacted by these algorithms to ask, “what kind of data is this algorithm using, and what biases could be impacting that data?”, as well as to consider “is using an algorithm in this context helpful or harmful, and to whom?”
Lauren Prastien: Over the next two episodes we’re going to explore some of the potential policy responses, ranging from looking at different ways of empowering digital rights to the importance of community standards.
Next week, we’ll be looking at data rights. Did you know that you played a pretty significant role in the digitization of the entire New York Times archive, the development of Google Maps and, now, the future of self-driving cars? We’ll talk about what that means, and what that could entitle you to next week. And here’s a preview of our conversation with our guest Tae Wan Kim, a professor of Business Ethics:
Tae Wan Kim: Data subjects can be considered as a special kind of investors.
Lauren Prastien: I’m Lauren Prastien.
Eugene Leventhal: And I’m Eugene Leventhal.
Lauren Prastien: This was Consequential. We’ll see you next week.
Eugene Leventhal: Consequential was recorded at the Block Center for Technology and Society at Carnegie Mellon University, which was established to examine the societal consequences of technological change and create meaningful plans of action. To learn more about Consequential, the Block Center and our faculty, you can check out our website at cmu.edu/block-center or follow us on Twitter @CMUBlockCenter. You can also email us at consequential@cmu.edu.
This episode of Consequential was written by Lauren Prastien, with editorial support from Eugene Leventhal. It was edited by Eugene and our intern, Ivan Plazacic. Consequential is produced by Eugene, Lauren, Shryansh Mehta and Jon Nehlsen.
This episode uses an excerpt of Mike Annony and Kate Crawford’s “Seeing without knowing: Limitations of the transparency ideal and its application to algorithmic accountability.”
S1 E3: Data Subjects and Manure Entrepreneurs
Lauren Prastien: Did you know that you played a vital role in the digitization of the entire New York Times archive, the development of Google Maps and the creation of Amazon’s recommendation engine? That's right, you!
Whether or not you know how to code, you've been part of the expansion of just how prevalent artificial intelligence is in society today. When you make choices of what to watch on Netflix or YouTube, you're informing their recommendation engine. When you interact with Alexa or Siri, you help train their voice recognition software. And if you've ever had to confirm your identity online and prove that you are not a robot, then you’re familiar with our key example for today - CAPTCHA. It started as a security check that digitized books, but now, every time you complete a CAPTCHA, you are determining the future of self-driving cars.
So, where does all of this leave you and your relationship with technology as a whole?
This is Consequential: what’s significant, what’s coming, and what we can do about it. I’m Lauren Prastien and I’ll be your main tour guide along this journey. You’ll also hear the voices of our many guests as well as of your other host.
Eugene Leventhal: Hi, I’m Eugene Leventhal. I’ll be joining throughout the season to take a step back with Lauren and overview what was just covered, talk policy, and read quotes. I’ll pass it back to you now, Lauren.
Lauren Prastien: Consequential is recorded at the Block Center for Technology and Society at Carnegie Mellon University. Established in 2018 through a generous gift from Keith Block and Suzanne Kelley, the Block Center is dedicated to investigating the economic, organizational, and public policy impacts of emerging technologies.
This week, we’re talking about Data Subjects and Manure Entrepreneurs.
So stick with us.
Our journey begins with CAPTCHA.
So, CAPTCHA stands for “Completely Automated Public Turing test to tell Computers and Humans Apart.” It’s catchy, I know. The idea is you’re trying to tell your computer that you’re a person and not, say, a bot that’s trying to wreak havoc on the Internet or impersonate you and steal your information. In his 2018 Netflix special Kid Gorgeous, comedian John Mulaney summed this up pretty well:
John Mulaney: The world is run by computers. The world is run by robots. And sometimes they ask us if we’re a robot, just cause we’re trying to log on and look at our own stuff. Multiple times a day. May I see my stuff please? I smell a robot. Prove. Prove. Prove you’re not a robot. Look at these curvy letters. Much curvier than most letters, wouldn’t you say? No robot could ever read these.
Lauren Prastien: Originally, this was the conceit: You’re trying to log into a website, and you’re presented with a series of letters and numbers that look like they’ve been run through a washing machine. You squint at it, try to figure it out, type it in, and then you get to see your stuff. Or you mess up, briefly worry that you might actually be a robot, and then try again.
But aside from keeping robots from touching your stuff or, say, instantaneously scalping all the tickets for a concert the second they drop and then reselling them at three times the cost, this didn’t really accomplish anything.
And this started to bother one of the early developers of CAPTCHA, Luis von Ahn. You probably know him as the co-founder and CEO of the language-learning platform Duolingo. But back in 2000, von Ahn was a PhD candidate at Carnegie Mellon University, where he worked on developing some of the first CAPTCHAs with his advisor, Manuel Blum. And for a minute there, he was kind of regretting subjecting humanity to these really obnoxious little tasks with no payoff. You proved you weren’t a robot, and then you proved you weren’t a robot, and then you proved you weren’t a robot, and you had nothing to show for it. You know, imagine Sisyphus happy.
So in 2007, von Ahn and a team of computer scientists at Carnegie Mellon established reCAPTCHA, a CAPTCHA-like system that didn’t just spit out a bunch of random letters and numbers – it borrowed text from otherwise hard-to-decipher books. So now, instead of just proving you weren’t a robot, you would also help digitize books.
That’s pretty useful, right? Now you’re not just seeing your stuff, you’re making books like Pride and Prejudice and the Adventures of Sherlock Holmes freely available online. If you’re interested in learning about reCAPTCHA’s work digitizing out-of-copyright books, the journalist Alex Hutchinson did some fantastic reporting on this for The Walrus in 2018, but let me give you the abbreviated version:
In 2004, there was a huge international initiative to digitize every out-of-copyright book in the world to make it freely available to anyone. While the software was able to digitize the content of a new book with 90% accuracy, older books presented some problems because they weren’t printed in a lot of the standard fonts we have now. So, the software could only accurately transcribe about 60% of older texts.
This was where the reCAPTCHA came in. The reCAPTCHA would consist of two words: A known word that serves as the actual test to confirm that you were a human and an unknown word that the software failed to characterize. If you go on CAPTCHA’s website, the example CAPTCHA you’ll get includes the words: “overlooks inquiry.” So let’s say the software already knows that the word overlooks is indeed the word overlooks. There’s your Turing test, where you prove you’re not a robot. But the word “inquiry” – I don’t know, it also looks like it could maybe be the word injury? So you throw that in the reCAPTCHA. And after a general consensus among four users as to what that word is, you’ve now transcribed the missing word in the book – at 99.1% accuracy.
The reCAPTCHA system helps to correct over 10 million words each day, allowing people to freely access books and articles online that they may never have had access to before. It’s also responsible for digitizing the entire New York Times archive, from 1851 to the present day. So, bravo! You did that!
But perhaps you’ve noticed that in the past few years, the CAPTCHAs reCAPTCHA was showing you have looked…a little different. Maybe you had to tell reCAPTCHA which pictures had storefronts in them. Or, maybe you had to pick all of the pictures of dogs. Or maybe only one of the words was a word, and the other one was a picture of a house number. Or, oh, I don’t know…
John Mulaney: I’ve devised a question no robot could ever answer! Which of these pictures does not have a stop sign in it? What?!
Lauren Prastien: Yeah. You know what kind of computer needs to recognize a stop sign and differentiate it from, say, a yield sign? Like I said, congratulations, you are part of the future of self-driving cars.
When it comes to making books freely available, it’s really easy to see this as a work of altruism for the common good. And that’s what Luis von Ahn envisioned: a collective effort on the part of humanity to share knowledge and literature across the world wide web.
And this isn’t the only time we’ve done something like this. Wikipedia is a vast online database of knowledge that is developed almost entirely from open-source labor. It’s an amazing example of something we discussed in our first episode: collective intelligence. Most Wikipedia editors self-describe as volunteers. And by the way, I got that from a Wikipedia article titled “Thoughts on Wikipedia Editing and Digital Labor.” But while Wikipedia also relies on free labor to promote the spread of knowledge, that labor was completely voluntary.
But in the case of reCAPTCHA, you can make the argument that you were an unconsenting, unpaid laborer in the process. Which is exactly what one Massachusetts woman did in 2015, when she filed a class-action lawsuit against Google, Inc., which bought reCAPTCHA in 2009. The suit alleged that asking users to transcribe text for Google’s commercial use and benefit, with no corresponding benefit to the user, was an act of fraud. Remember, only one of the two words in a reCAPTCHA actually keeps your stuff safe, so to speak.
However, the case was dismissed by the US District Court of the Northern District of California in early 2016 on the grounds that typing a single word without knowledge of how Google profits from such conduct does not outweigh the benefit. Essentially, the US District Court argued that the Plaintiff was being compensated, just not financially: she’s allowed to use the free Google services that rely on those reCAPTCHAS like Google Maps and Google Books, as well as the free Gmail account she was signing up for when she completed the reCAPTCHA. In other words, the court found that the value of that free labor - however unwitting it is - does not outweigh the value of the benefits that someone receives for performing that labor.
But is that still true today? Consider a recent report from Allied Market Research, which priced the global market for autonomous vehicles at 54.23 billion dollars, with the expectation that this market will be worth more than 500 billion by 2026.
This isn’t just about reCAPTCHA and self-driving cars. And it isn’t just a financial issue or a labor issue. Your data is an incredibly valuable and ultimately essential resource, and it’s driving more than just autonomous vehicles. Last episode, we discussed just how pervasive algorithms have become, from recommending the things we buy and watch to supporting treatment and hiring decisions. But it’s important to remember that these algorithms didn’t just appear out of nowhere. The algorithms that we use every day could not exist without the data that we passively offer up anytime we click on an advertisement or order a t-shirt or binge that new show everyone’s talking about.
So it’s easy to feel absolutely out of control here, like you don’t have a seat at the table. But here’s the thing: You have a seat, it’s just been empty.
Tae Wan Kim: Without the data, the AI’s not going to work. But the problem is, who really owns the data? So who benefits, and who does not?
Lauren Prastien: So stay with us.
If these algorithms need our data to function, that means we’re an absolutely necessary and, dare I say, consequential part of this process. And that might entitle us to some kind of authority over how our data is being used. But in order to define our rights when it comes to our data, we need to define what sort of authority we have.
That’s where Professor Tae Wan Kim comes in. He’s a Professor of Business Ethics, and specifically, he’s interested in the ethics of data capitalism. In other words, he wants to know what our rights are when big data is monetized, and he’s interested in articulating exactly where a data subject - or anyone whose data is being used to drive technology - sits at the table.
Tae Wan Kim: So benefits, and who does not? Our typical understanding of data subjects are that they are consumers. So, we offer data to Facebook. In exchange, Facebook offers a service. That is a discrete transaction. Once we sell the data to Facebook, then the data is theirs. But there is a problem – legally and philosophically – to make a case that we sell our privacy to someone else. That’s the beginning of this question.
Lauren: As we discussed with the example of reCAPTCHA, there’s also a pervading argument that data subjects are workers. But Professor Kim is interested in a different framework for encouraging data subjects to take a proactive role in this decision-making: data subjects as investors.
Tae Wan Kim: Data subjects can be considered as a special kind of investors. Like shareholders.
Lauren Prastien: In his research on data ownership, Professor Kim found that the relationship between data subjects and the corporations that use their data is structurally similar to the relationship between shareholders and the corporations that use their investments. Essentially, both data subjects and traditional shareholders provide the essential resources necessary to power a given product. For shareholders, that’s money - by investing money into a business, you then get to reap the rewards of your investment, if it’s a successful investment. And that’s pretty similar to what data subjects do with their data - they give the basic resources that drive the technology that they then benefit from using. Like how the people filling out reCAPTCHAS got to use Google’s services for free.
But there’s a big difference between shareholders and data subjects - at least right now. Shareholders know how much money they invested and are aware of what is being done with that money. And even in a more general sense, shareholders know that they’re shareholders. But some data subjects aren’t even aware they’re data subjects.
Tae Wan Kim: The bottom line is informed consent. But the problem is informed consent assumes that the data is mine and then I transfer the exclusive right to use that data to another company. But it’s not that clear of an issue.
Lauren Prastien: This kind of grey area has come up before, by the way, in a very different kind of business model.
Tae Wan Kim: In the nineteenth century before the introduction of automobiles, most people used horse-drawn wagons. Horses create manure. All the way down, all the roads. No one thought that would be an important economic resource. But some people thought that maybe, no one cares about that, no one claims ownership.
Lauren Prastien: Yeah. You can see where this is going. Some very brave man named William A. Lockwood stepped into the street, found eighteen piles of horse droppings just kind of sitting there and saw an opportunity to make some fertilizer on the cheap. The problem was that this guy named Thomas Haslem had ordered two of his servants to make those piles with the intention of I don’t know, picking them up later, I guess? And when he arrives the next day to find the piles of manure gone, he says, hey, wait a second, that’s my horse’s droppings. You can’t just use my horse’s droppings that I left in the street for profit. So I want the $6 that the fertilizer you made is worth. Then Lockwood the manure entrepreneur said, well, no, because I waited 24 hours for the original owner to claim it, I asked a few public officials if they knew who made those piles and if they wanted them, and this constable was basically like, “ew. No.” So I found your weird manure piles and I gathered them up, and then did the labor of making the fertilizer. And the court said, “I mean, yeah, that’s valid.”
The case, Haslem v. Lockwood, is hilarious and fascinating and would take an entire episode to unpack. But the point here is this: these questions are complicated. But that doesn’t mean we shouldn’t tackle them.
I should note here that Haslem v. Lockwood is an interesting analog, but it’s not a perfect point of comparison. Horse droppings are, well, excrement. And the fertilizer that Lockwood made didn’t impact Haslem’s ability to get a job or secure a loan. So our data is a little different from that.
Tae Wan Kim: If our society is similar about data, if no one cares about data, then the courts will decide with the companies. But once we as the individuals start claiming that I have interest in my data, claim that I have some proprietary interest in my data, then the landscape will probably change. So it’s up to us, actually.
Lauren Prastien: Despite how unapproachable topics such as AI and machine learning can seem for those who do not specialize in these areas, it’s crucial to remember that everyone plays an important role in the future of how technology gets rolled out and implemented. By ensuring that individuals have rights relating to their own data, policymakers can set the stage for people to have some control over their data.
Tae Wan KIm: So for instance, shareholders are granted several rights. One is information rights. Once they invest their money, the company has a duty to explain how the company has used the investment for some period of time. How to realize that duty in typical societies is using annual shareholders meeting, during which shareholders are informed of how their money has been used. If data subjects have similar information rights, then they have a right to know how companies have used their data to run their companies. So, we can imagine something like an annual data subjects meeting.
Lauren Prastien: It might be an added burden on the companies innovating with AI and machine learning, but creating such rights would also ensure a higher standard of protection for the individuals. And by articulating that data subjects are in fact investors, we’d know how to enact legislation to better protect them.
Tae Wan Kim: It is a philosophical and legal question. What is really the legitimate status of the data subject? Are they simply consumers? Then the consumer protection perspective is the best. So, public policymakers can think of how to protect them using consumer protection agencies. If data subjects are laborers, then labor protection law is the best way to go. If investor is the right legitimate status, then we have to think of how to use the SEC.
Lauren Prastien: If we had such rights, we could fight for programs to help deal with some of the problematic areas of AI, such as the kinds of harmful biases that can emerge in the sorts of algorithms that we discussed last week. But that’s going to take some education, both on our part and on the part of our policymakers.
Senator Orrin Hatch: If so, how do you sustain a business model in which users don’t pay for your service?
Mark Zuckerberg: Senator, we run ads.
Senator Orrin Hatch: I see. That’s great.
Lauren Prastien: Stay with us.
In a 2015 article in The Guardian titled “What does the panopticon mean in the age of digital surveillance?”, Thomas McMullan said of the sale of our privacy:
Eugene Leventhal: “In the private space of my personal browsing, I do not feel exposed - I do not feel that my body of data is under surveillance because I do not know where that body begins or ends.”
Lauren Prastien: Here, he was referring to how we do or do not police our own online behavior under the assumption that we are all being constantly watched. But there’s something to be said of the fact that often, we don’t know where that body of data begins or ends, particularly when it comes to data capitalism. And if we did, maybe we’d be able to take a more proactive role in those decisions.
Because while Professor Kim’s approach to understanding our legal role as data subjects could inform how we may or may not be protected by certain governing bodies, we can’t just be passive in assuming that that protection is absolutely coming. And by the way, we probably can’t wait around for policymakers to just learn these things on their own.
In April 2018, Facebook co-founder and CEO Mark Zuckerberg appeared before Congress to discuss data privacy and the Cambridge Analytica scandal. And it became pretty clear that a lot of really prominent and powerful policymakers didn’t really understand how Facebook and other companies that collect, monetize and utilize your data actually work.
Senator Orrin Hatch: If so, how do you sustain a business model in which users don’t pay for your service?
Mark Zuckerberg: Senator, we run ads.
Senator Orrin Hatch: I see. That’s great.
Lauren Prastien: Remember when Professor Kim said that every time we use a site like Facebook, we’re making a transaction? Essentially, instead of paying Facebook money to log on, share articles, talk to our friends, check up on our old high school rivals, we’re giving them our data, which they use in turn to push us relevant ads that generate money for the site. Which is why sometimes, you’ll go look at a pair of sneakers on one website, and then proceed to have those sneakers chase you around the entire Internet. And this is a pretty consistent model, but it’s also a pretty new model. And it makes sense once you hear it, but intuitively, we’re not always aware that that transaction is taking place.
The Zuckerberg hearings were ten hours long in total and, at times, really frustrating. But perhaps the most telling was this moment between Zuckerberg and Louisiana Senator John Kennedy:
Senator John Kennedy: As a Facebook user, are you willing to give me more control over my data?
Mark Zuckerberg: Senator, as someone who uses Facebook, I believe that you should have complete control over your data.
Senator John Kennedy: Okay. Are you willing to go back and work on giving me a greater right to erase my data?
Mark Zuckerberg: Senator, you can already delete any of the data that’s there or delete all of your data.
Senator John Kennedy: Are you going to work on expanding that?
Mark Zuckerberg: Senator, I think we already do what you think we are referring to, but certainly we’re working on trying to make these controls easier.
Senator John Kennedy: Are you willing to expand my right to know who you’re sharing my data with?
Mark Zuckerberg: Senator, we already give you a list of apps that you’re using, and you signed into those yourself, and provided affirmative consent. As I said, we don’t share any data with…
Senator John Kennedy: On that...on that user agreement - are you willing to expand my right to prohibit you from sharing my data?
Senator Mark Zuckerberg: Senator, again, I believe that you already have that control. I think people have that full control in the system already today. If we’re not communicating this clearly, then that’s a big thing that we should work on, because I think the principles that you’re articulating are the ones that you believe in and try to codify in the product that we build.
John Kennedy: Are you willing to give me the right to take my data on Facebook and move it to another social media platform?
Senator Mark Zuckerberg: Senator, you can already do that. We have a download your information tool where you can go, get a file of all the content there and then do whatever you want with it.
John Kennedy: Then I assume you’re willing to give me the right to say that I’m going to go on your platform and you’re going to tell a lot about me as a result but I don’t want you to share it with anybody.
Senator Mark Zuckerberg: Yes, Senator. I believe you already have that ability today.
Lauren Prastien: There’s a massive breakdown in communication between the people set to draw up legislation on platforms like Facebook and the people who design and run those platforms. But let me ask you something - did you know that you could go delete your data from Facebook? And did you know that actually, Facebook doesn’t sell your data - it acts as the broker between you and the companies that ultimately advertise to you by selling access to your newsfeed? A company can’t say, “hey Facebook, can you give me all of Lauren Prastien’s data so that I can figure out how to sell stuff to her? Please and thank you.” But it can say, “hey Facebook, can you give me access to someone who might be willing to buy these sneakers? Please and thank you.” And Facebook would say, “why yes. I can’t tell you who she is. But I can keep reminding her that these sneakers exist until she eventually capitulates and buys them.”
Which is something you can opt out of or manage. If you go to your preferences page on Facebook, you can decide what kinds of ads you want targeted to you, what kind of data Facebook can access for those ads, and what materials you might find upsetting to look at.
Which, by the way, wasn’t something I knew either, until I started researching for this episode.
But it’s also worth noting that on December 18, 2018, just eight months after the Zuckerberg hearings, Gabriel J.X. Dance, Michael LaForgia and Nicholas Confessore of the New York Times broke the story that Facebook let major companies like Microsoft, Netflix, Spotify, Amazon and Yahoo access user’s names, contact information, private messages and posts despite claiming that it had stopped this kind of sharing years ago. The Times also noted that some of these companies even had the ability to read, write and delete users’ private messages. Even the New York Times itself was named as a company that retained access to users’ friend lists until 2017, despite the fact that it had discontinued the article-sharing application that was using those friend lists in 2011. And all this is pretty meaningful, given this exchange in the Zuckerberg hearings:
Senator John Kennedy: Let me ask you one final question in my twelve seconds. Could somebody call you up and say, I want to see John Kennedy’s file?
Mark Zuckerberg: Absolutely not!
Senator John Kennedy: Not would you do it. Could you do it?
Mark Zuckerberg: In theory.
Senator John Kennedy: Do you have the right to put my data...a name on my data and share it with somebody?
Mark Zuckerberg: I do not believe we have the right to do that.
Senator John Kennedy: Do you have the ability?
Mark Zuckerberg: Senator, the data is in the system. So…
Senator John Kennedy: Do you have the ability?
Mark Zuckerberg: Technically, I think someone could do that. But that would be a massive breach. So we would never do that.
Senator John Kennedy: It would be a breach. Thank you, Mr. Chairman.
Lauren Prastien: In response to the New York Times exposé, Facebook’s director of privacy and public policy, Steve Scatterfield, said none of the partnerships violated users’ privacy or its 2011 agreement with the Federal Trade Commission, wherein Facebook agreed not to share users’ data without their explicit permission. Why? Essentially, because the 150 companies that had access to the users’ data, even if those users had disabled all data-sharing options - that’s right, 150, and yes, you heard me, even if users were like please share absolutely none of my data - those companies were acting as extensions of Facebook itself. Which...meh?
So while Facebook may not have literally sold your data, they did make deals that let some of the most powerful companies in the world take a little peek at it. Which was not something that I considered as within the realm of possibility when I agreed to make a data transaction with Facebook.
And that’s just Facebook.
Kartik Hosanagar: I think in today's world we need to be talking about, uh, basic data and algorithm literacy, which should be in schools and people should have a basic understanding of when I do things on an app or on a website, what kinds of data might be trapped? What might, what are the kinds of things that companies can do with the data? How do I find out how data are being used.
Lauren Prastien: Stay with us.
Have you ever been walking around and suddenly got a notification that a completely innocuous app, like, I don’t know, a game app that you play to make your commute go faster, has been tracking your location? And your phone goes,
Eugene Leventhal: “Hey, do you want this app to continue tracking your location?”
Lauren Prastien: And you’re like, “wait, what do you mean, continue?”
By the way, the reason why a lot of those apps ask to track your location is to be able to target more relevant ads to you. But even though I technically consented to that and then had the ability to tell the app, “hey, stop it. No, I don’t want you to track my location,” I didn’t really know that.
So there’s a lot of confusion. But there is some legislation in the works for how to most effectively regulate this, from requiring users to opt in to sharing data rather than just sharing it by default to requiring tech companies to more overtly disclose which advertisers they’re working with.
One piece of legislation currently in the works is the DASHBOARD Act, a bipartisan effort that would require large-scale digital service providers like YouTube and Amazon to give regular updates to their users on what personal data is being collected, what the economic value of that data is, and how third parties are using that data. By the way, DASHBOARD stands for “Designing Accounting Safeguards to Help Broaden Oversight And Regulations on Data.” Yeah, I am also loving the acronyms this episode.
On a state level, California passed the California Consumer Privacy Act in late September 2019. This law is set to come into effect on January 1, 2020, and it will give the state increased power in demanding disclosure and, in certain circumstances, pursuing legal action against businesses. These laws will apply to companies earning over $25 million annually, holding personal information on over 50,000 people, or earning half of their revenue from selling others’ data.
In addition to creating frameworks that define and defend the rights of data subjects, policymakers can also focus on initiatives to educate data subjects on their role in the development of these technologies. Because, like Professor Kim said, a big difference between shareholders and data subjects is informed consent.
We asked Professor Hosanagar, our guest from our previous episode, what that kind of informed consent might look like.
Kartik Hosanagar: Yeah, I would say that, first of all where we are today is that most of us use technology very passively. And, uh, you know, as I mentioned, decisions are being made for us and about us when we have no clue, nor the interest in digging in deeper and understanding what's actually happening behind the scenes. And, and I think that needs to change. Um, in terms of, uh, you know, to what extent are companies providing the information or users digging in and trying to learn more? Not a whole lot is happening in that regard. So we're mostly in the dark. We do need to know certain things. And again, it doesn't mean that we need to, don't know the nitty gritty of how these algorithms work and you know, all the engineering details.
Lauren Prastien: While it may not be realistic to think that every person on Earth will be able to read and write code, it is possible to add a basic element of digital literacy to educational systems. This is something that the American education system has tried to do whenever we encounter a new technology that’s going to impact our workforce and our way of life. Growing up in the American public-school system, I remember learning skills like using Wikipedia responsibly and effectively navigating a search engine like Google. So what’s to stop us from incorporating algorithmic literacy into curricula?
Kartik Hosanagar: You know, we used to talk about digital literacy 10, 15 years back and basic computer literacy and knowledge of the Internet. I think in today's world we need to be talking about basic data and algorithm literacy, which should be in schools and people should have a basic understanding of, you know, when I do things on an app or on a website, what kinds of data might be tracked? What might, what are the kinds of things that companies can do with the data? How do I find out how data are being used?
Lauren Prastien: You also may have noticed that a lot of the policy recommendations that have come up on this podcast have some educational component. And this isn’t a huge coincidence. Education is a big theme here. As this season progresses, we’re going to be digging into how education has changed and is going to continue to change in response to these technologies, both in terms of the infiltration of tech into the classroom and in terms of preparing individuals for the way these technologies will impact their lives and their places of work.
This brings us back to one of our central points this season - that you play a very crucial role in shaping an equitable digital future. Not just in providing the data, but in advocating for how that data gets used.
Before we end, it’s worth mentioning that a few weeks ago, Mark Zuckerberg returned to Capitol Hill to talk to the House’s Financial Services Committee about the Libra cryptocurrency system. Some of the issues we’ve been discussing today and that Zuckerberg discussed in his 2018 hearings came up again.
So we thought it would be important to watch and review the 5-hour hearing before we released this episode as written. And something that we noticed was that this time, Congress was pretty well-informed on a lot of the nuances of Facebook’s data monetization model, the algorithms Facebook uses and even data subject protections. Like in this exchange with New York Representative Nydia Velazquez:
Representative Nydia Velazquez: Mr. Zuckerberg, Calibra has pledged it will not share account information or financial data with Facebook or any third-party without customer consent. However, Facebook has had a history of problems safeguarding users’ data. In July, Facebook was forced to pay a 5 billion-dollar fine to the FTC. By far, the largest penalty ever imposed to a company for violating consumers’ privacy rights as part of the settlement related to the 2018 Cambridge Analytica Scandal. So let me start off by asking you a very simple question, why should we believe what you and Calibra are saying about protecting customer privacy and financial data?
Mark Zuckerberg: Well, Congresswoman, I think this is an important question for us on all of the new services that we build. We certainly have work to do to build trust. I think the settlement and order we entered into with the FTC will help us set a new standard for our industry in terms of the rigor of the privacy program that we’re building. We’re now basically building out a privacy program for people’s data that is parallel to what the Sarbanes-Oxley requirements would be for a public company on people’s financial data.
Lauren Prastien: So real quick. The Sarbanes-Oxley Act is a federal law that protects the investors in a public company from fraudulent financial reporting. It was passed in 2002 as a result of the financial scandals of the early aughts, like the Enron Scandal of 2001 and the Tyco Scandal of 2002.
The hearings also raised a really interesting issue when it comes to data subject rights: shadow profiles. Here’s Iowa Representative Cynthia Axne:
Representative Cynthia Axne: So do you collect data on people who don’t even have an account with Facebook?
Mark Zuckerberg: Congresswoman, there are a number of cases where a website or app might send us signals from things that they’re seeing and we might match that to someone who’s on our services. But someone might also send us information about someone who’s not on our services, in which case we likely wouldn’t use that.
Representative Cynthia Axne: So you collect data on people who don’t even have an account? Correct?
Mark Zuckerberg: Congressman, I’m not sure that’s what I just said. But-
Representative Cynthia Axne: If you are loading up somebody contacts and you’re able to access that information, that’s information about somebody who might not have a Facebook account. Is that correct?
Mark Zuckerberg: Congresswoman, if you’re referring to a person uploading their own contact list and saying that the information on their contact list might include people who are not on Facebook, then sure, yes. In that case they’re...
Representative Cynthia Axne: So Facebook then has a profile of virtually every American. And your business model is to sell ads based on harvesting as much data as possible from as many people as possible. So you said last year that you believed it was a reasonable principle that consumers should be able to easily place limits on the personal data that companies collect and retain. I know Facebook users have a setting to opt out of data collection and that they can download their information. But I want to remind you of what you said in your testimony, ”Facebook is about putting power in people’s hands.” If one of my constituents doesn’t have a Facebook account, how are they supposed to place limits on what information your company has about them when they collect information about them, but they don’t have the opportunity to opt out because they’re not in Facebook?
Mark Zuckerberg: Congresswoman, respectfully, I think you…I don’t agree with the characterization saying that if someone uploads their contacts…
Representative Cynthia Axne: That’s just one example. I know that there’s multiple ways that you’re able to collect data for individuals. So I’m asking you, for those folks who don’t have a Facebook account, what are you doing to help them place limits on the information that your company has about them?
Mark Zuckerberg: Congresswoman, my understanding is not that we build profiles for people who are not on our service. There may be signals that apps and other things send us that might include people who aren’t in our community. But I don’t think we include those in any kind of understanding of who a person is, if the person isn’t on our services.
Representative Cynthia Axne: So I appreciate that. What actions do you know specifically are being taken or are you willing to take to ensure that people who don’t have a Facebook account have that power to limit the data that your company is collecting?
Mark Zuckerberg: Congresswoman, what I’m trying to communicate is that I believe that, that’s the case today. I can get back to you on all of the different things that we do in terms of controls of services.
Representative Cynthia Axne: That would be great. Because, we absolutely need some specifics around that to make sure that people can protect their data privacy. Mr. Zuckerberg, to conclude, Facebook is now tracking people’s behavior in numerous ways, whether they’re using it or not. It’s been used to undermine our elections. And of course, I know you’re aware Facebook isn’t the most trusted name. So I’m asking you to think about what needs to be fixed before you bring a currency to market. Thank you.
Lauren Prastien: This isn’t the first time Mark Zuckerberg has been asked about shadow profiles by Congress, they came up in the 2018 hearings as well, where he denied having any knowledge of the existence of these profiles. However, in 2018, the journalist Kashmir Hill of Gizmodo found that Facebook’s ad targeting algorithms were indeed using the contact information of individuals who did not necessarily have Facebook accounts, which they obtained via users who had consented to allow Facebook access to their contact information. Which might be the next frontier in the battle for data subject rights and informed consent.
Which is all to say that today, you have clearly defined rights as a consumer, and you have clear protections to ensure that the products you buy aren’t going to hurt you. When you go to school, you have rights and protections as a student. When you walk into a doctor’s office, you have rights and protections as a patient. And if you buy shares in a company, you have rights and protections as a shareholder - thanks, Sarbanes-Oxley. So why not as a data subject?
We hope that today’s examples of exploring how you have contributed to the development of some of the most pervasive technologies in use today have left you feeling more encouraged about your seat at the table when it comes to tech development.
So what does demanding your seat at the table look like?
Eugene Leventhal: That’s a great question, Lauren, and it’s something that’s still being determined. From Professor Kim’s work in defining what role data subjects have and what rights and protections that entitles them to Professor Hosanagar’s work in advocating for adequate educational reform for data subjects, there’s a ton that can be happening on the policy side that can impact your place in the implementation of these technologies.
There are various national organizations to better understand the impacts of AI and to help you, as someone who’s data is being used for these systems, better understand how these algorithms impact you. Academically linked efforts such as AI Now Institute out of NYU, Stanford’s Institute for Human-Centered Artificial Intelligence, and here at Carnegie Mellon, the Block Center for Tech and Society are all working to increase the amount of attention researchers are paying to these questions. Nonprofits such as the Center for Humane Technology are helping people understand how technology overall is affecting our well-being, while more localized efforts, such as the Montreal AI Ethics Institute and Pittsburgh AI are creating new ways for individuals to learn more about your role in AI and to advocate for your rights and to engage in the ongoing conversation surrounding data rights as a whole. And so where do we go from here, Lauren?
Lauren Prastien: We’re going to take the next episode to explore how becoming more active participants in this landscape could help shape this landscape for the better - as well as some of the obstacles to facilitating communication between the technologists that develop algorithms, the policymakers that implement them and the communities that these algorithms affect. Because the fact is that algorithms are becoming more and more present in our lives and affecting continuously more important decisions. Ultimately, if we have better insight into these decision-making processes, we can help to improve those processes and use them to help improve our way of life, rather than diminish it.
Next week, we’ll talk to Jason Hong, a professor in Carnegie Mellon University’s Human-Computer Interaction Institute, who has conceived of a rather clever way for the people affected by algorithms to help hold them accountable:
Jason Hong: It turns out that several hundreds of companies already had these bug bounties and it’s a great way of trying to align incentives of the security researchers. So what we’re trying to do with bias bounty is can we try to incentivize lots of lay people to try to find potential bugs inside of these machine learning algorithms.
Lauren Prastien: I’m Lauren Prastien, and this was Consequential. We’ll see you next week.
This episode of Consequential was written by Lauren Prastien, with editorial support from Eugene Leventhal. It was edited by Eugene and our intern, Ivan Plazacic. Consequential is produced by Eugene, Lauren, Shryansh Mehta and Jon Nehlsen.
This episode uses clips of John Mulaney’s comedy special Kid Gorgeous, the Wikipedia article “Thoughts on Wikipedia Editing and Digital Labor,” Alex Hutchinson’s reporting on reCAPTCHA for The Walrus, an excerpt of Thomas McMullan’s article “What does the panopticon mean in the age of digital surveillance?”, which was published in The Guardian in 2015, excerpts of Mark Zuckerberg’s 2018 and 2019 hearings before Congress, an excerpt from Gabriel J.X. Dance, Michael LaForgia and Nicholas Confessore’s article “As Facebook Raised a Privacy Wall, It Carved an Opening for Tech Giants,” and Kashmir Hill’s reporting for Gizmodo on Facebook’s shadow profiles.
S1 E4: Fair Enough
Lauren Prastien: Let me ask you a question. What does fairness mean?
Don’t look it up in the dictionary. I already did. In case you’re curious, fairness is impartial and just treatment or behavior without favoritism or discrimination.
What I’m really asking you is what does fairness mean mathematically? Computationally? Could you write me an algorithm that’s going to make the fairest decision? The most impartial, just, unbiased decision?
And if you’re thinking, wait a second. Fairness isn’t a mathematical idea, it’s an ethical one. Fine, okay: whose ethics? Mine? Yours? A whole bunch of people’s?
So what does fairness mean culturally? What does it mean to a community?
Over the past few weeks, we’ve talked about how algorithms can be really helpful and really hurtful. And so far, that’s been using a rather un-nuanced metric of
Eugene Leventhal: Oh, an algorithm to overcome human bias in hiring decisions? It works? that’s great.
Lauren Prastien: Versus
Eugene Leventhal: Oh, the hiring algorithm favors men? Nevermind! That’s bad! That’s real bad.” But a lot of algorithms aren’t just objectively bad or good.
We can just about universally agree that an algorithm that tosses out applications from women is problematic. But take this example: In 2017, the City of Boston set out to try to improve their public school system’s busing processes using automated systems. To give you some context: Boston’s public school system had the highest transportation costs in the country, accounting for 10% of the district’s entire budget, and some schools drew students from over 20 zip codes.
So, the City issued the Boston Public Schools Transportation Challenge, which offered a $15,000 prize for an algorithm that would streamline its busing and school start times. The winning research team devised an algorithm that changed the start times of 84% of schools and was was 20% more efficient than any of the route maps developed by hand. The algorithm saved the City $5 million that was reinvested directly into the schools, and it cut more than 20,000 pounds of carbon dioxide emissions per day.
Here’s the thing, though: While the busing component of this algorithm was a huge success, the start time component was never implemented. Why? Because while the algorithm would have benefited a lot of people in Boston - for instance, it reduced the number of teenagers with early high school start times from 74% to just 6%, and made sure that elementary schools let students out well before dark - a lot of families that benefited from the old system would now have to make pretty dramatic changes to their schedules to accommodate the new one. Which, those families argued, was unfair.
There are a lot of examples of seemingly good AI interventions being ultimately rejected by the communities they were designed for. Which, to be fair, seems like something a community should be able to do. Sometimes, this is because the definition of fairness the community is using doesn’t quite match with the definition of fairness the algorithm is. So how do we balance these competing definitions of fairness?
This is Consequential: what’s significant, what’s coming, and what we can do about it. I’m Lauren Prastien and I’ll be your main tour guide along this journey. You’ll also hear the voices of our many guests as well as of your other host.
Eugene Leventhal: Hi, I’m Eugene Leventhal. I’ll be joining throughout the season to take a step back with Lauren and overview what was just covered, talk policy, and read quotes. I’ll pass it back to you now Lauren.
Lauren Prastien: Consequential is recorded at the Block Center for Technology and Society at Carnegie Mellon University. Established in 2018 through a generous gift from Keith Block and Suzeanne Kelly, the Block Center is dedicated to investigating the economic, organizational, and public policy impacts of emerging technologies.
Last week, we talked about your evolving rights as a data subject, and how important it is to understand these technologies. This week, it’s all about fairness, community standards and the bias bounty. Our question: how do we make sure these algorithms are fair...or, you know, fair enough?
This wasn’t the first time Boston tried to use an algorithm to reform their public school system and then decided not to implement it.
Their first attempt was really ambitious: the City wanted to improve the racial and geographic diversity of their school districts, while also providing students with education that was closer to home. Multiple studies have shown that racially and socioeconomically diverse classrooms lead to students that are better leaders, problem-solvers and critical thinkers, have lower drop-out rates and higher college enrollment rates. So like Professor Woolley found in her studies of collective intelligence, which you may remember from our first episode, diversity is a really good thing, on teams, in the workplace, and in classrooms.
However, by shortening commutes, the algorithm actually decreased school integration, because it wasn’t able to pick up on the larger racial disparities and socioeconomic contexts that pervade the City of Boston. So, the city rejected the algorithm.
Which makes sense - the algorithm didn’t do what it set out to do. But in the case of the start time suggestions from the busing algorithm, things are a little fuzzier.
On December 22, 2017, the Boston Globe ran an opinion piece titled “Don’t blame the algorithm for doing what Boston school officials asked.” And the general premise was this: school start and end times are political problems, and algorithms shouldn’t be solving political problems. The authors argued that it was debatable whether Boston Public Schools wanted to enhance the students’ learning experience or just save money. If it was the latter, then it succeeded. And if it was the former, then some of the blowback might indicate that it didn’t. Because here are these parents, saying the system is unfair.
But this is the challenge: we haven’t actually agreed on a universal definition of fairness - algorithmic or otherwise.
Jason Hong: The question of what is fair has been something that's been plaguing humanity since the very beginning.
Lauren Prastien: That’s Jason Hong. He’s a professor in the Human-Computer Interaction Institute at Carnegie Mellon. His research looks at how computer scientists can make technologies more easily understandable to the general public, particularly when it comes to issues like privacy, security and fairness. Because fairness in a computer science sense is a little different than the way we understand fairness by most cultural and philosophical definitions.
Jason Hong: You mentioned the mathematical definitions of fairness, and to give you a few examples of those one would be that it’s equally unfair or it’s equally wrong for different groups of people.
Lauren Prastien: Correct me if I’m wrong here, but when most people say “I’d like to do this the fairest way possible,” they don’t actually mean “I’d like to do this such that it is equally unpleasant for everyone.” Or at least, that’s not the first place they’d go. But sometimes, that’s what an algorithm uses as a metric of fairness. But that’s also not the only way an algorithm can determine fairness.
Jason Hong: So say for example, if you have two groups of people, let's call them East Coast people and West Coast people and you want to give out loans to them. Uh, one definition of fairness would be that is equally accurate on both cases that an algorithm would correctly give loans to people who would repay correctly. But then it also does not give loans to people who are not repaying those loans. Uh, but that's just one definition of fairness.
Lauren Prastien: And those are just the mathematical definitions. When it comes to the way people measure fairness, there’s usually a little element of ethics thrown in there, too.
Jason Hong: It comes from moral philosophy. One is called deontological. And the way to think about this one is it basically follows the rules and processes. So given that you had a set of rules, which people have already declared, say, here's how we're going to go do things, have you followed those rules and are you falling into a declared processes?
Uh, the other kind of fairness would be described as consequentialist. So basically outcomes. So is the outcome actually fair or not?
Lauren Prastien: I promise we haven’t tried to put some form of the word consequential in every episode. It’s just been turning out that way. But real quick - consequentialism is a form of moral philosophy that basically says that the morality of an action is based entirely on the consequences of that action, rather than the intention of that action. That’s where deontological fairness conflicts with consequentialist fairness.
To explain this, let’s look at the story of Robin Hood. Remember, Robin Hood’s whole deal is that he steals from the rich in order to give to the poor. Which is, you know, a crime. But Robin Hood is considered the hero of the story, and this is because the rich, and in particular the Sheriff of Nottingham, are over-taxing the poor to the point of starvation and using those taxes to line their pockets. Which you could also argue is a form of theft. So do you punish Robin Hood?
The deontologist would say yes, both he and the Sheriff stole from the community, just in different ways. And if you don’t hold them both accountable, the social order is going to break down and the rules are going to have no meaning.
But the consequentialist would say no. Because by robbing the rich, Robin Hood was benefitting his community, not himself. And it’s not fair to treat the actions of the Sheriff of Nottingham and Robin Hood as equal crimes.
We’re not going to settle the debate between deontological morality and consequentialism and several of the other branches of moral philosophy - nihilism, utilitarianism, pick your ism of choice. NBC’s The Good Place has been at it for four seasons, and philosophers have been trying to untangle it for much, much longer than that.
The point is that “fairness” isn’t an objective concept. Usually, it’s determined by a community’s agreed-upon standards. But even then, everyone in that community doesn’t always agree upon those standards.
Here’s something really crucial to consider with this Boston example. In 2018, the journalist David Scharfenberg published an article in The Boston Globe titled, “Computers Can Solve Your Problem. You May Not Like the Answer.”
In speaking to the developers of the bus algorithm, Scharfenberg learned that their algorithm sorted through 1 novemtrigintillion options - a number I did not know existed, but is apparently 1 followed by 120 zeroes. And one of their biggest achievements with this one in one novemtrigintillion options was that it made sure that 94% of high schools had a later start time, which is really important. Teenagers have different circadian rhythms than children and adults, and as a result, the effect of sleep deprivation on teenagers is especially pronounced, and it can be catastrophic to a student’s academic performance, mental health and physical development.
Scharfenberg also noted that the current system was disproportionately assigning ideal school start times to residents in whiter, wealthier regions of Boston. By redistributing those start times, the algorithm made getting to school, having a better educational experience and ensuring a good nights’ sleep more tenable for families in regions that weren’t white or affluent.
However, it did require a trade-off for those families that did benefit from the previous system.
I don’t want to pass judgment here at all, or say if one group was absolutely right or wrong. And it’s not my place to say if this was the best of the 1 novemtrigintillion options or not.
However, I do want to discuss the fact that this emphasizes the value of really communicating with a community on what an algorithm is taking into account to make a fair decision. And that in turn raises two really important questions:
First: Where do conversations of ethics and values fit into the development and regulation of technology?
And second: How do we make sure that policymakers and technologists are effectively ensuring that communities are able to make informed judgments about the technologies that might impact them?
Molly Wright Steenson: The way that decisions have been made by AI researchers or technologists who work on AI related technologies - it's decisions that they make about the design of a thing or a product or a service or something else. Those design decisions are felt by humans.
Lauren Prastien: If you’re having a little deja vu here, it’s because this is a clip from our interview with Molly Wright Steenson from our episode on the black box. But as we were talking to Professor Steenson about the black box, the subject of fairness came up a lot. In part because these subjects are haunted by the same specters: bias and subjectivity.
Molly Wright Steenson: That question of fairness I think is really good because it’s also what’s really difficult about, um, about AI. The fact is that we need bias some way or another in our day to day lives. Bias is what keeps me crossing the street safely by determining when I should go and when I should stop.
Lauren Prastien: And when it comes to determining which biases are just plain wrong or actively harming people, well, that comes down to ethics.
The notion of ethical AI is kind of all the rage right now. For good reason. Like Professor Steenson said, these decisions are being felt by humans.
But as this culture of ethical AI rises, there is a lot of cynicism around it. At a recent conference at Stanford University’s Human-AI Initiative, Dr. Annette Zimmermann, a political philosopher at Princeton University, presented a slide on “AI Ethics Traps Bingo,” detailing everything from “let’s make a checklist” to “who needs ethics once we have good laws?”
Ethics isn’t just a box to tick, but it can be really tempting to frame it that way to avoid getting into the weeds. Because, as we saw in the debate between deontology and consequentialism, ethics can be kind of time-consuming, circuitous, and complicated. You know, sort of the opposite of the things that good tech likes to advertise itself as: quick, direct, and simple.
Molly Wright Steenson: I think that if you want to attach an ethicist to a project or a startup, then what you’re going to be doing is it’s like, it’s like attaching a post it note to it or an attractive hat. It’s gonna fall off.
Lauren Prastien: By the way, that’s one of my favorite things anyone has said this season.
Molly Wright Steenson: What you needed to be doing is it needs to be built into the incentives and rewards of, of the systems that we’re building. And that requires a rethinking of how programmers are incentivized.
If you are just focused on operationalizing everything in Silicon Valley or in a startup, where on earth are you going to put ethics? There’s no room for it. And so what you need instead to do is conceptualize what we do and what we build ethically from the get go.
Lauren Prastien: When it comes to incorporating ethics into design processes and reframing how programmers approach their work, Professor Steenson referred to a concept called service design.
Molly Wright Steenson: There’s a design discipline called service design, um, which is considering the multiple stakeholders in a, in a design problem, right? So it could be citizens and it could be the team building whatever technology you’re using, but there are probably secondary people involved. There are whole lot of different stakeholders. There are people who will feel the impact of whatever is designed or built. And then there’s a question of how do you design for that, right?
Lauren Prastien: In their 2018 book, This Is Service Design Doing: Applying Service Design Thinking in the Real World, Adam Lawrence, Jakob Schneider, Marc Stickdorn, and Markus Edgar Hormess propose a set of principles for service design. Among them are that service design needs to be human-centered, or consider the experience of the people affected by the given product, and that it needs to be collaborative, which means that the stakeholders should be actively involved when it comes to the process of design development and implementation. The authors also say that the needs, ideas and values of stakeholders should be researched and enacted in reality, and adapted as the world and context that these design decisions are enacted in shifts.
According to Professor Steenson, context and adaptability are really important elements of addressing issues of bias and fairness. Because as these technologies become more pervasive, the stakes get much higher.
Molly Wright Steenson: One thing that I think can happen if we do our jobs right as designers and for my position as, as a professor is to get students to understand what they’re walking into and what the scope is that they might be addressing. That it isn’t just about making an attractive object or a nice interface.
I think we see the ramifications as these technologies are implemented and implemented at scale. You know, Facebook means one thing when it’s 2005 and people on a few college campuses are using it. It means something else when it has 2.7 billion users.
Lauren Prastien: When it comes to developing these algorithms within a larger cultural and social context - especially when the stakes attached are in flux - there are going to be some trade-offs. It is impossible to please all 2.7 billion users of a social networking platform or the entire Boston Public School system.
So how do we navigate managing these challenging tradeoffs?
[BREAK ]
Lauren Prastien: As Professor Steenson noted, scaling up algorithmic decision-making is going to impact the design decisions surrounding those algorithms, and those decisions don’t occur in a vacuum.
So, we consulted with the Block Center’s Chief Ethicist. His name is David Danks, and he’s a professor of philosophy and psychology here at CMU, where his work looks at the ethical and policy implications of autonomous systems and machine learning.
David Danks: A really important point is the ways in which the ethical issues are changing over time. That it’s not a stable, “ask this question every year and we’re going to be okay about all of it.”
Lauren Prastien: Remember what Professor Steenson said about how scope and stakes can really impact how technologists need to be thinking about design? It’s why putting on the fancy hat of ethics once doesn’t work. These things are always in flux. The hat is going to fall off.
And as the scope of a lot of these technologies has started to broaden significantly, Professor Danks has seen the ethical landscape of tech shifting as well.
David Danks: I think one set of ethical issues that’s really emerged in the last year or two is a growing realization that we can’t have our cake and eat it too. That many of the choices we’re making when we develop technology and we deploy it in particular communities involve tradeoffs and those trade offs are not technological in nature. They are not necessarily political in nature, they’re ethical in nature. And so we really have to start as people who build, deploy and regulate technology to think about the trade offs that we are imposing on the communities around us and trying to really engage with those communities to figure out whether the trade offs we’re making are the right ones for them rather than paternalistically presupposing that we’re doing the right thing.
Lauren Prastien: As Professor Danks has mentioned, just presupposing the answers to those questions or just assuming you’re doing right by the people impacted by a given piece of technology can be really harmful. History is full of good intentions going really poorly. And like the principles of consequentialism we went over earlier in this episode emphasize: outcomes matter.
David Danks: It’s absolutely critical that people recognize these impacts and collaborate with people who can help them understand the depth of those impacts in the form of those impacts. Now that requires changes in education. We have to teach people how to ask the right questions. It requires changes in development practices at these software companies. They need to get better at providing tools for their developers to rapidly determine whether they should go talk to somebody.
Lauren Prastien: And there are a lot of places to find those answers. From consulting with ethicists who have spent a lot of time toiling with these questions to actually asking the communities themselves which values and standards are the most important to them when it comes to making a decision.
But when it comes to community engagement, a lot of that presupposing to date has come from the fact that it’s actually quite difficult to facilitate these conversations about fairness in the first place.
So how do we ensure these conversations are taking place?
In January of 2019, Alexis C. Madrigal published a piece in the Atlantic titled, “How a Feel-Good AI Story Went Wrong in Flint.” I highly recommend you read it, but for the sake of our discussion today, let me give you a quick summary.
After Flint’s Water Crisis came to light, the City was faced with the task of having to find and remove the lead pipes under people’s homes. The problem was that the city’s records on this were pretty inconsistent, and sometimes just outright wrong. And so, a team of volunteer computer scientists put together a machine learning algorithm to try to figure out which houses had lead pipes. In 2017, this algorithm helped the City locate and replace lead pipes in over 6,000 homes, operating ahead of schedule and under budget.
But the following year, things slowed down significantly. While the algorithm was operating at 70% accuracy in 2017, by the end of 2018, they had dug up about 10,531 properties, and only located lead pipes in 1,567. And thousands of homes in Flint still had lead pipes.
This happened because in 2018, the city abandoned the machine learning method because it was receiving pressure from the residents of Flint, who felt that certain neighborhoods and homes were being overlooked. In other words, the City would come by and dig up the yards of one neighborhood, then completely skip another neighborhood, and then maybe only dig up a few yards in the next neighborhood. Which, if you’re one of the people getting skipped, is going to feel really suspicious and unfair.
But the city wasn’t looking at certain homes and neighborhoods because there was a very low probability that these homes and neighborhoods actually had lead pipes. But they didn’t really have a way of effectively and efficiently communicating this to the community. And if a city’s trust has already been fundamentally shaken by something as devastating as a water crisis, it’s probably not going to feel super great about inherently trusting an algorithm being employed by the city, especially with the whole “Schrodinger’s pipes” situation it left them in. Which, to be fair: wouldn’t you also always wonder if your pipes were actually made of lead or not?
By looking at every home, the project slowed down significantly. In the eastern block of Zone 10, the city dug up hundreds of properties, but not a single one of them had lead pipes. Meaning that while the City dug up those pipes, there were actual lead pipes in other neighborhoods still sitting under the ground and potentially leaching into people’s water. Like in the City’s Fifth Ward, where the algorithm estimated that 80% of houses excavated would have lead, but from January to August 2018, this was the area with the fewest excavations.
And here’s what’s really upsetting: when the National Resources Defense Council ultimately pursued legal action against the City of Flint, it did so because it believed the City had abandoned its priority of lead removal, thereby endangering certain communities like the Fifth Ward. But the City’s decision to deviate from that algorithm was because of community distrust of that algorithm, and that was based on the fact that the community didn’t actually know whether or not the algorithm was making a fair assessment of which properties to dig up.
Since the publication of Madrigal’s article, the City of Flint switched back to the algorithm. And this time, there’s something really important happening: the developers of the pipe detection algorithm are working on the creation of an interpretable, accessible way to show the residents of Flint how the algorithm is making its decisions.
According to Professor Hong, that kind of communication is key when it comes to introducing an algorithmic intervention like that into a community. Right now, his team is working on a project to help facilitate clearer communication about the ways an algorithm is operating such that the people impacted by these algorithms are able to evaluate and critique them from an informed perspective.
Jason Hong: And so what we’re trying to do, this is, you know, make machine learning algorithms more understandable and then also probe what people’s perceptions of fairness are in lots of different situations. Which one...which mathematical definition is actually are closest to people’s perceptions of fairness in that specific situation. It might be the case that people think that this mathematical definition over here is actually really good for face recognition, but this mathematical definition over there is better for, uh, say advertisements.
Lauren Prastien: Professor Hong’s work could help make algorithmic implementation more of a community effort, and he hopes that by coming up with better ways of conveying and critiquing algorithmic fairness, we’ll be able to have these complicated ethical discussions about algorithms that do not necessarily seem completely bad or absolutely perfect.
Jason Hong: There’s going to be the people who are developing the systems, the people might be affected by the systems, the people who might be regulating the systems and so on. And um, you have to make sure that all of those people and all those groups actually have their incentives aligned correctly so that we can have much better kinds of outcomes.
Lauren Prastien: When we have communities engaged in ensuring that the technologies they’re adopting are in line with their values, those constituents can start participating in making those technologies better and more equitable, which brings us to another aspect of Professor Hong’s work.
Jason Hong: There’s this thing in cybersecurity known as a bug bounty. The idea is that you want to incentivize people to find security bugs in your software, but to inform you of it rather than trying to exploit it or to sell it to criminals. Apple said that they are offering $1 million to anybody who can hack and the ios right now or the iPhone. It turns out that several hundreds of companies already had these bug bounties and it’s a great way of trying to align incentives of the security researchers.
Lauren Prastien: In April of this year, the cybersecurity company HackerOne announced that in the past 7 years, the members of its community had won more than 50 million dollars in bug bounty cash by reporting over 120,000 vulnerabilities to over 1,300 programs. Which is amazing, considering what could have happened if those vulnerabilities were in the wrong hands.
Looking at the success of bug bounties, Professor Hong was inspired to develop something similar to involve communities in the algorithms impacting their lives: a bias bounty.
Jason Hong: So for example, a lot of face recognition algorithms, it turns out that they are less accurate on people with darker skin and also people who are women. And so I think a lot of people would say, hmm, that doesn't seem very right. Uh, just intuitively without even a formal definition, a lot of people would say that seems not very fair.
So what we’re trying to do with bias bounty is can we try to incentivize lots of lay people to try to find potential bugs inside of these machine learning algorithms. So this might be a way of trying to find that, for example, this computer vision algorithm just doesn’t work very well for people who are wearing headscarves. So, hey, here’s this algorithm for trying to recognize faces and oh, here’s an example of that one that doesn’t work. Here’s another example of one that doesn’t work and so on.
Lauren Prastien: That sounds pretty awesome, right? You find something wrong with an algorithm, and then you get rewarded.
Hearing this, I couldn’t help thinking of the slew of videos that have hit the internet over the past few years of automatic soap dispensers not detecting black people’s hands. In 2015, an attendee of the DragonCon conference in Atlanta, T.J. Fitzpatrick, posted a video of his hand waving under an automatic soap dispenser. No matter how hard he tries, even going so far as to press the sensor with his finger, no soap. So he gets his white friend, Larry, to put his hand under the dispenser and, viola, there’s the soap.
The reason why this is happening is because soap dispensers like that use near-infrared technology to trigger the release of soap. When a reflective object, like, say, a white hand, goes under the dispenser, the near-infrared light is bounced back towards the sensor, triggering the release of soap. But darker colors absorb light, so it’s not as likely for that light to be bounced back towards the sensor.
Which seems like a pretty big design flaw.
Jason Hong: That’s also a good reason for why, you know, Silicon Valley for example, needs a lot more diversity in general because you want to try to minimize those kinds of blind spots
that you might have. Uh, but for other researchers and for, you know, other kinds of government systems, I think, you know, the bias money that we were just talking about I think could be effective. Uh, it’s definitely something where you can get a lot more people involved and could also be sort of fun. And also it’s trying to get people involved with something that’s much bigger than any single person that you are trying to help protect other people or trying to make sure the world is more fair.
Lauren Prastien: Between bringing people into the conversation on AI fairness and incentivizing users of these technologies - from things as high-stakes as bus algorithms to as simple as automatic soap dispensers - to meaningfully critique them, we could see communities more effectively developing and enforcing these standards for their technologies.
It’s important to say once more that all of this stuff is very new and its scope has been increasing dramatically, and so setting the standards for policy, regulation and accountability is sometimes without precedent.
So, how do we make sure these algorithms are fair...or, you know, fair enough?
Eugene Leventhal: Today’s discussion highlights that some of the biggest challenges that we have to overcome in relation to emerging technologies such as AI are not technical in nature. On the contrary, questions of how to bring people together and get them involved in the development of solutions is a much more human question. A big part of this is how organizations and policymakers communicate the components and impacts of an algorithm. There’s a lot of uncertainty ahead relating to finding approaches that benefit everyone, but that is no reason to shy away from these challenges that are continuously growing in importance.
So where do we go from here Lauren?
Lauren Prastien: Over the past few weeks, we’ve dug into the pervasiveness of algorithms, their potential impact on industries and the ways we - as data subjects and as communities - can have a say in how these technologies are enacted. Next week, we’re going to look at an area where we could see a lot of these emerging technologies begin to disrupt a lot of long-standing structures, for better or worse: and that’s education. Here’s a clip from one of our guests next week, Professor Michael D. Smith:
Michael D. Smith: Technology is never going to change higher education, right? Cause we’re the one industry on the planet who doesn’t have to worry about technology coming in and disrupting our business. He says provocatively.
Lauren Prastien: I’m Lauren Prastien,
Eugene Leventhal: and I’m Eugene Leventhal
Lauren Prastien: and this was Consequential. We’ll see you next week.
Eugene Leventhal: Consequential was recorded at the Block Center for Technology and Society at Carnegie Mellon University. The Block Center was established to examine the societal consequences of technological change and create meaningful plans of action. To learn more about Consequential, the Block Center and our faculty, you can check out our website at cmu.edu/block-center or follow us on Twitter @CMUBlockCenter. You can also email us at consequential@cmu.edu.
This episode of Consequential was written by Lauren Prastien, with editorial support from Eugene Leventhal. It was edited by Eugene and our intern, Ivan Plazacic. Consequential is produced by Eugene, Lauren, Shryansh Mehta and Jon Nehlsen.
This episode uses Kade Crockford and Joi Ito’s “Don’t blame the algorithm for doing what Boston school officials asked” and David Scharfenberg’s “Computers Can Solve Your Problem. You May Not Like the Answer,” both published in The Boston Globe. It also refers to Dr. Annette Zimmermann’s “AI Ethics Traps Bingo,” Adam Lawrence, Jakob Schneider, Marc Stickdorn, and Markus Edgar Hormess’s book This Is Service Design Doing: Applying Service Design Thinking in the Real World, and Alexis C. Madrigal’s article in the Atlantic titled, “How a Feel-Good AI Story Went Wrong in Flint.” We have also used a tweet from T.J. Fitzpatrick.
S1 E5: Bursting the Education Bubble
Lauren Prastien: This is a story about the underdog. About power, about scarcity. This is, I’m not going to lie, one of my favorite stories about the Internet.
In 2018, nineteen-year-old Montero Lamar Hill, better known as Lil Nas X, dropped out of college to devote himself fully to his rap career. He was living with his sister, sleeping only three hours a night, when he bought a beat for 30 dollars on the Internet - an infectious trap reimagining of a Nine Inch Nails song. He wrote a song about a plucky, independent cowboy that oozed Americana and bravado, and recorded it in under an hour at a small recording studio in Atlanta, using their $20 Tuesday discount. Yeah. Between the beat and the hour at the CinCoYo Recording Studio, “Old Town Road” cost 50 dollars, the price of a nice dinner for two.
For reference, in 2011, NPR’s “Planet Money” podcast estimated that Rihanna’s single “Man Down” cost $78,000 to make through traditional channels, and then another million dollars to promote through those channels. But Lil Nas X’s promotion model was a little different from that. He used TikTok, a free social video-sharing app, where “Old Town Road” caught on like wildfire in late 2018. So if we want to talk about an industry disruptor, look no further than Lil Nas X.
In October 2019, “Old Town Road” was awarded a diamond certification by the Recording Industry Association of America, for selling or streaming ten million copies in the United States. And by the way, it achieved this diamond certification faster than any of the 32 other songs to reach the distinction. Again, for reference: The Black Eyed Peas song “I Gotta Feeling,” the most requested song of 2009, took nearly ten years to go diamond. Old Town Road took ten months.
It’s really important to remember that “Old Town Road” isn’t a fluke or an exception. It’s part of a larger trend in the entertainment industry, where the growth of technologies for content creation and distribution have disrupted the existing power structures that have controlled who gets to make and share that content for years. Lil Nas X is not the first star to come up through the Internet. He’s in good company with Justin Bieber, who’s arguably YouTube’s biggest success story; SoundCloud artists like Post Malone and Halsey; Comedians, like Bo Burnham, Grace Helbig, and SNL stars Beck Bennett and Kyle Mooney. The bestseller 50 Shades of Grey was published on an online fan-fiction website before shattering distribution records previously held by mainstream thriller writer Dan Brown. The idea for an upcoming heist film starring Rihanna and Lupita Nyong’o was pitched not to a room full of executives, but by a fan on Twitter.
And this year, Alfonso Cuaron’s Roma, a film distributed on Netflix after running in theaters for just three weeks, was nominated for 10 Academy Awards and won 3, sparking controversy among some of the biggest names in Hollywood, like director Steven Spielberg:
Steven Spielberg: Once you commit to a television format, you’re a TV movie. You certainly, if it’s a good show, deserve an Emmy, but not an Oscar. I don’t believe films that are just given token qualifications in a couple of theaters for less than a week should qualify for the Academy Award nomination.
Lauren Prastien: Like it or not, technology is shaking up traditional models of media creation, distribution and consumption, and it has changed the entertainment industry forever. According to researchers here at Carnegie Mellon, what happened to entertainment might happen again to a very different kind of industry: higher education.
This is Consequential: what’s significant, what’s coming, and what we can do about it. I’m Lauren Prastien and I’ll be your main tour guide along this journey. You’ll also hear the voices of our many guests as well as of your other host.
Eugene Leventhal: Hi, I’m Eugene Leventhal. I’ll be joining throughout the season to take a step back with Lauren and overview what was just covered, to talk policy, and to read quotes. I’ll pass it back to you now Lauren.
Lauren Prastien: Consequential is recorded at the Block Center for Technology and Society at Carnegie Mellon University. Established in 2018 through a generous gift from Keith Block and Suzanne Kelly, the Block Center is dedicated to investigating the economic, organizational, and public policy impacts of emerging technologies.
Today, we want to know: is tech going to burst the education bubble?
Michael D. Smith: Technology is never going to change higher education, right? Cause we're the one industry on the planet who doesn't have to worry about technology coming in and disrupting our business. He says provocatively.
Lauren Prastien: That’s Michael D. Smith. He’s a professor of Information Technology and Marketing at Carnegie Mellon. And before he was making that provocative statement about higher ed, he was making a really similar provocative statement about the entertainment industry.
Michael D. Smith: It had been an industry that was incredibly stable for a hundred years. Um, you know, massive shifts in technology, but the same six motion picture studios, the same four record labels, the same five publishers dominated the business. And then all of a sudden when information technology hit each of these powerful in each of these powerful players lost power very quickly.
Lauren Prastien: Why? Well, in 2016, Professor Smith published a book called, Streaming, Sharing, Stealing: Big Data and the Future of Entertainment, with Rahul Telang, a professor of Information Systems and Management at Carnegie Mellon, to answer that very question. And what Professors Smith and Telang found was that the longevity of this industry relied on a little something called scarcity.
Michael D. Smith: They were able to maintain their power for the, for a hundred years because they were able to control access to the scarce resources necessary to create content, the scarcity for this resources, to distribute content. And then, and then we’re able to create scarcity around how content got consumed.
Lauren Prastien: Without scarcity of those resources, anyone can be an actor, a singer, a director, you name it, and broadcast it out onto the Internet. You know, the whole Warholian “in the future, everyone will be famous for 15 minutes” kind of thing.
And that’s really good! Digital platforms and entertainment analytics have allowed for the distribution of voices and stories that have been previously underrepresented in media, providing for the development and elevation of more content in what marketers call “the long-tail.”
Real quick: the term long-tail is attributed to the work of mathematician Benoît Mandelbrot, and was popularized by author and entrepreneur Chris Anderson in a 2004 article in Wired. It comes up in a lot of different fields, but I’m going to focus on the way it’s used in media. Essentially, when it comes to content, you’ve got two categories: the head and the long-tail. Think of the head as the small set of items that appease a big market, so Top 40 music and Hollywood blockbusters. And the long-tail is a larger set of items that each have smaller markets. It’s the niche content.
In a traditional entertainment model, concentrating mostly on the head made sense. You only had a finite set of timeslots on channels, a finite amount of airtime on a radio station, a finite number of screens at a movie theater, and so on. Which was how those big names were able to maintain control: by channeling their resources into head content that would occupy those finite spaces.
And right now, Professor Smith sees something really similar happening in higher education.
Michael D. Smith: Higher education looks very similar to the entertainment industry in the sense that our power is based on our ability to control scarcity in who gets seats in the classes, scarcity in the professors, um, and then scarcity in, in communicating to the market who’s smart and who’s not by by using a sheet of paper with a stamp on it, uh, that, that you have to pay a quarter of a million dollars and four years of your life to get, um, what would happen if those scarce resources weren’t as scarce anymore.
Lauren Prastien: We’ve seen what happens in entertainment. Platforms like Netflix and Hulu don’t have to worry about filling slots with the most broadly appealing content. First of all - there are no timeslots. The concept of Primetime doesn’t exist on Netflix. And if I don’t like what I’m watching on Hulu, it’s not like I have to stop giving Hulu my business by changing the channel - because, well, no channels. I can just consume some other content on that platform. And it goes further than that.
Michael D. Smith: When I think about the benefits of on demand online streaming platforms like nec, like Netflix, it's this ability to say, what exactly are you? Let me, let me understand. You as an individual, understand what you've liked in the past, what other people have liked that similar, and then create something that's, you create a set of programming that's uniquely customized to you, um, versus the broadcast world, which was let's find a single message that's broadly applicable to everyone. Um, could you do with the same thing in the classroom? I think so. Today we think about teaching in terms of a broadcast world. I'm going to come to class with a lecture that I'm hoping is broadly applicable to all of all 40 of students, when in fact each of those 40 students is an individual with a unique background, a unique set of knowledge, a unique way. They learn a unique way.
Lauren Prastien: What Professor Smith is getting at here isn’t a new concept. It’s called mastery learning. It was first proposed by the educational psychologist Benjamin Bloom in 1968, and the basic idea was this: until students were able to demonstrate that they had a level of mastery of a given concept or piece of information, they wouldn’t be able to move forward to learn any subsequent information. This means that instead of solely placing the burden of keeping up on the student, the responsibility is now shared between the student and the instructor, who needs to ensure that they are able to effectively convey the material to everyone in the class, catering to their given learning styles and providing supplemental materials where necessary. Which sounds really, really great.
But in a traditional higher ed model, you only have a semester to teach this material, and you’ve gotta get through it, whether everyone’s on board or not. Again: think head versus long tail.
And time is just one of the forms of scarcity in education.
But like Professor Smith said, higher education has several other forms of scarcity, and they have really wide-reaching implications.
Michael D. Smith: You talk to admissions officers and we all know that the SAT is easily manipulated by the ability. Just, just the ability to get review courses, um, you know, can change, can change your, your score. And if you live in a zip code where it's unlikely you're going to have access to those review courses, you're at a huge disadvantage. Um, that's a problem for the individual student obviously. That's kind of the hope of what we're talking about here. That in that in the same way technology allowed people who had stories to tell to tell those stories, technology might allow people who have unique skills and gifts to contribute to society in a way that we're not allowing them to today.
All of a sudden people had opportunities to tell their stories that didn't, did, didn't have opportunities before and people had opportunities to consume stories. I think the same thing's going to be true in education. This is going to be tough on established universities, but I think it's going to be great for the industry of teaching and learning.
Lauren Prastien: So what is this going to look like - both inside and outside of traditional classrooms? And how do we ensure that students using these more accessible forms of education are protected from misinformation, scams and faulty materials? Stay with us.
Let me tell you a quick story. When I was in college, there was this one really famous professor that taught intro to creative writing. And in order to even take a class with this person, you had to apply for a spot and then you had to stand on a line outside of the registration office on a given date, and be one of the lucky first few in line to put your name on the list for that professor’s section of the course. and okay to be fair, it was a really good class.
The other day, I was scrolling through Instagram, and I saw an ad for a certain platform where you stream online classes from celebrated experts in their fields. Such as, as this ad on my Instagram showed me the other day: the very same in-demand professor from my alma mater. That’s right: no more standing on a line in the snow. No more application. And, hey, you don’t even have to be a student.
And frankly, I loved seeing this. Because, why not? There might be someone who could really benefit from that class who doesn’t want or need the four years of college that would usually come with it. And while it’s true that you won’t have the experience of having that professor read your work, you will still get her insights on writing short stories. Because in the preview for this class, she was saying a lot of the same stuff she said to us on our first day of her class.
And this is something that’s another really fascinating commonality about the narratives of the entertainment and education industries: the new opportunities that come with streaming video. Be it the development of services like the one I just described or the rise of the massive open online course, or MOOC, streaming video has allowed individuals to take a certain class or learn a specific skill that they may not have had access to based on their geographic location, socioeconomic status or even just their daily schedule.
And learning via streaming video is becoming really common. In its most recent “By The Numbers” report on MOOCs, Class Central found that 20 million new learners signed up for at least one MOOC in 2018. While this was down slightly from 23 million in 2017, the number of paying users of MOOC platforms may have increased. Currently, more than 101 million students are actively enrolled in MOOCs, and over 900 universities around the world, including MIT, Imperial College London, and our very own Carnegie Mellon University, offer at least one MOOC.
But how effective is learning via video? And how do you handle the credentialing of someone who learned a skill from a video in their home versus learning that skill in a classroom and then getting a diploma?
Pedro Ferreira: We know that students love video, video games for education. We know that students love playing games, but that they actually learning? There's some anecdotal evidence that there are some learning involved, but how does that actually work?
Lauren Prastien: That’s Pedro Ferreira. He’s a professor of information systems, engineering and public policy at Carnegie Mellon. And along with Professor Smith, who we spoke to earlier, he’s working on how to make better videos for better education.
Pedro Ferreira: How can we actually understand how the students learn? And then what conditions in the right contexts for what kind of goals? And then with all that information, can we perhaps personalize? And one video works for you, but it doesn't work for the next student and different places of learning and so on. And so far, so we aim in this project to do large scale experimentation to actually find out what works.
Lauren Prastien: This isn’t the first time that Professor Ferreira’s looked at tech - and even videos - in the classroom. In 2014, he released a study on the effect that YouTube had on classrooms in Portugal. The data was from 2006 - so, you know, at this point, YouTube had actually only been around for a year, and it looked really, really different from the YouTube of today. What Professor Ferreira found was that, unfortunately, in the classrooms with access to YouTube, student performance went way, way, way down. So what makes him optimistic about video technology in the classroom now?
Pedro Ferreira: That study, uh, looked at the context, actually happens lots of time, which is the technology kind of parachutes into the school. And people are not used to the technology. They don't know what to do with. And you have as powerful technology like access to the Internet that allows you for both learning and distraction at the same time, guests which one you go, it's going to prevail if you don't actually thought about how you're going to use it productively. So for example, just to give you a counter case, just more recently, I've been working on a paper where we put smart phones into the classroom, not Internet, but we put them in a condition where the students can use them at twill, but they also can use in another condition. They can use the smart phones at will, but the teacher actively uses the smartphones to learn. And guess what? That's the condition where it actually grades are better. So you can actually introduce technology into the classroom in a positive way. And also in a negative way. It depends on how you actually combine the use of the technology with what you want to teach.
Lauren Prastien: Educators are no strangers to the risks of parachuting. There are entire databases, message boards and even listacles on the Internet devoted to ed tech failures. And it always boils down to the same formula: the technology is kind of just dropped into the classroom, with very little pedagogical motivation beyond “this thing is new and it’s cool.”
In this collaboration with Professor Smith, Professor Ferreira wants to see how, when approached with intentionality, videos can enhance student learning experiences and even teach us more about how students learn.
Pedro Ferreira: We're looking at how people actually gonna start video, stop videos.
Fast forward because I know this stuff for I rewind because I need to learn that again. And so we'll have this almost like frame by frame understanding of how the students interacted with the video and we were endowing a platform to run experiments where students can actually then comment on particular frames. I want to know this particular frame in this particular video was the one that spurred all these discussion among students because then there's a chat room and the messages back and forth and so on and so forth.
Lauren Prastien: By allowing students to have an active role in consuming, critiquing and in some cases even creating educational video, Professor Ferreira envisions being able to provide more personalized educational experiences that more effectively cater to students’ specific needs. But he does admit that not all video is proven equal.
Pedro Ferreira: We are talking about the world where users generate content, generate small videos that generate small videos for education, for entertainment, and so on and so forth. And without that, 90% of the people don't generate anything. 9% generate something in 1% generates good stuff, right? And so we have been inundated by, I would say, low quality stuff on the Internet these days. Uh, and also good quality, but you need to go through and, and, and, and navigate, right? And so we need to understand what's actually good for each student at each point in case, because we can actually widen the gaps if we put students in front of bad material. And so, uh, the recommended system for education I think need to actually be very much more precise.
Lauren Prastien: That’s one of the important distinctions between the entertainment industry and higher ed. If I see a bad movie or listen to a bad song on the Internet, there aren’t very serious consequences for that. But that’s not how it goes with education.
Pedro Ferreira: How are we actually going to certify people that went on the Internet to watch this video for these many hours or binged watched all these education content and now all of a sudden I'm an expert in, some of them are, we just need to actually find out that they are and, and imply them. And so how the market is formally going to certify these people I think is a huge, a huge opportunity and also huge hurdle.
Lauren Prastien: Ensuring the legitimacy of video-based education, adapting traditional credentialing frameworks to more holistically address the changing nature of learning, and protecting the rights of users of MOOCs are all really important issues for policymakers to examine. And right now, educators and regulators alike are really torn on how to handle this.
In an article for Inside Higher Ed, the journalist Lindsay McKenzie outlined some of the issues pervading the legislation of distance education. What she found was that in part, it boiled down to whether these protections need to be enacted on the state level or on the national-level. Essentially, because these online programs often operate in multiple states, they are really difficult to legislate. Earlier this year, the US Department of Education convened a panel to set up a new national set of rules for accreditors, or providers of online education, and in October, they published a set of regulations based partially on the panel’s findings that will take effect in July of 2020. These new provisions have received a lot of criticism, particularly for relaxing a lot of regulations and protections related to for-profit online education institutions. Because while proponents argue that these national regulations streamline otherwise complicated state-level regulations, critics of the new regulations maintain that this will actually substantially weaken protections for students and taxpayers alike.
The debate over how to handle the legislation of online education been going on for more than a decade, and as the nature and scope of educational videos change, these questions become a lot more complicated. Because while there are the institutions and opportunities that open doors for people who normally would not have the chance to take a certain class or learn a particular skill, there are online education entities that are just flat-out scams.
But students aren’t the only group that will be impacted by online education and the proliferation of tech in the classroom, and they’re not the only group that will need support. As Professor Ferreira found, this is also going to have a profound impact on teachers:
Pedro Ferreira: We've been trying with some schools to try and find out how the students react to videos and so on and so forth. And one thing that I have learned already is that when you increasingly rely on these kinds of materials, the role of the teacher changes.
Lauren Prastien: So, what will this mean for teachers? We’ll get into that in just a moment.
Lauren Herckis: Technology affords educators the opportunity to implement their pedagogies in ways that make sense for them with their students. So a shift in the, the ecosystem, the technological ecosystem in which they're doing their teaching means they need to rethink some of the minutiae of teaching that are so important to them.
Lauren Prastien: That’s Lauren Herckis. She’s an anthropologist at Carnegie Mellon University, and her work looks at faculty culture, from the use of technology in higher education to something that I can relate to as a former educator: the fear of looking stupid in front of your students. By the way, she also teaches a course on the Archaeology of Death, which I think is very, very cool, but that’s neither here nor there.
Lauren Herckis: So when we're talking about a student body that's now not just the students sitting in front of you and your classroom, but also the students who are watching and who are participating over, uh, a link at a distance that requires a shift, but that's not new. Teaching has always required adaptation to the new tech, new technologies, new contexts and new students.
Lauren Prastien: And sometimes, that adaptation is happening at a different pace for a teacher than it is for a student.
Lauren Herckis: But really any time there's a shift that changes classroom dynamics, there's a growth that's necessary. There are shifts in pedagogy and in understanding of what teaching and learning is for and a recalibration of, well, what are my students looking for? Why are they here? What are their goals? And does this class meet their goals? Um, there's a, there's a recalibration required, um, for how our understanding of content and the process of teaching can work with a new kind of classroom or a new set of students.
Lauren Prastien: And Professor Herckis suggests that when it comes to how to manage the changing nature of education and best prepare teachers for new kinds of students and new technologies, higher education can take some inspiration from healthcare.
Lauren Herckis: But there's been a revolution in medicine over the last several decades in which doctors are connected to one another and to evidence-based networks and organizations that can help provide those kinds of supports, like checklists that can ensure that our current knowledge about what's best, um, is implementable, is accessible. And, and so, yeah, most professors are not in a position to take a class on how to teach, let alone a course of study on how to teach. But providing accessible support that can ensure that when they're teaching in this new way or with this new thing, they're doing this in a way that is evidence and in line with our best understanding of how to teach effectively or how to use technology to its best advantage. Um, every little bit helps and accessible supports, um, have made just a tremendous difference in medicine. And there's no reason why we can't produce the similar kinds of supports in postsecondary education.
Lauren Prastien: And those supports are really, really important, because the kind of parachuting that Professor Ferreira described earlier is just as taxing on teachers as it is on students.
Lauren Herckis: Big changes are often rolled out on on college campuses as though they are a solution to problems that people have been concerned about and they will be the solution for the future without an acknowledgement that this too will be phased out. How long will this, uh, this set of smartboards, this new learning management system, um, this set of classroom equipment that's being rolled out to every classroom, how long do we really expect this to be the state of the art and what other things are going to change during that time?
Lauren Prastien: But Professor Herckis agrees that an openness to these new forms of teaching and learning is really important, and it’s an overall good trend in the field of education.
Lauren Herckis: But I think that some of the most powerful technological innovations that, that are currently revolutionizing education and stand to in the future are, are basically communication technologies. I think that a person who's living in a place that doesn't have access to a school but who wants to learn, could use a communication technology to have a conversation regularly, a face to face conversation effectively with someone where they practice a language that nobody speaks within a hundred miles of where they live is pretty powerful.
Lauren Prastien: But how do we make sure that all of this innovation actually reaches the people it needs to? Because, heads up - it might not.
Although we’ve laid out a number of ways in which education is being changed by technology, it’s crucial to keep in mind that a few things are being assumed for all of this to work. For one, if students don’t have access to computers or tablets, then they won’t be able to access any kind of digital solutions. On an even more fundamental level, if students don’t have access to broadband, then it becomes practically impossible for those students without broadband access to keep up with those who have it.
But first, Eugene, what are some things that policymakers should be thinking about when it comes to EdTech and making sure the necessary infrastructure is in place?
Eugene Leventhal: First, the foundational level, which is making sure that there is broadband internet available for everyone. This is something that we’ll be hearing about in our next episode.
Once we shift to looking at the solutions getting deployed in schools, we can turn to Professor Lee Branstetter, who you may remember from our first episode as the Head of our Future of Work Initiative, for a potential solution.
Lee Branstetter: I think part of the solution, um, is for government and government funded entities to do for Ed Tech, what the FDA does for drugs, submit it to scientific tests, rigorous scientific tests, um, on, you know, human subjects in this case students, and be able to help people figure out what works and what doesn't mean. We would never imagine a world in which the drug companies can just push whatever the heck they want to without any kind of government testing or regulation. But guess what, that's the world of Ed Tech. We can do better.
Eugene Leventhal: And when we look beyond Ed Tech to online courses, there is a lot of disagreement about how exactly to protect the students, teachers and taxpayers that are the stakeholders in this system. Though policymakers don’t need to worry about academic institutions in their jurisdictions being completely replaced by online learning environments, it’s important to be aware of how technology can help students and schools when applied with thoughtful, evidence-backed intent. Aside from talking about the lack of broadband that many Americans deal with, Lauren what else are we covering next week?
Lauren Prastien: In exploring some of the issues and potential solutions with broadband, we’re going to be getting into a larger discussion on what’s being called the rural-urban divide. The term has been coming up in the news a lot lately, and so we want to know: is there such thing as a rural-urban divide? And how can emerging technologies complement the values, character and industries of rural communities, rather than attempt to overwrite them? We’ll also talk about the role that universities can play in the context of bridging resource gaps and how physical infrastructure and mobility play a role in resources divides as well.
Here’s a preview of our conversation next week with Karen Lightman, the Executive Director of Metro21: Smart Cities Institute at Carnegie Mellon:
Karen Lightman: And we live in an area where we have access to pretty good high speed broadband. And there's a promise with 5g if going in even faster. But there's a good chunk of the, of the United States, the world that doesn't have access to high speed broadband. So that means kids can't do their homework. Right?
Lauren Prastien: I’m Lauren Prastien,
Eugene Leventhal: and I’m Eugene Leventhal
Lauren Prastien: and this was Consequential. We’ll see you next week.
Consequential was recorded at the Block Center for Technology and Society at Carnegie Mellon University. The Block Center was established to examine the societal consequences of technological change and create meaningful plans of action. To learn more about Consequential, the Block Center and our faculty, you can check out our website at cmu.edu/block-center or follow us on Twitter @CMUBlockCenter. You can also email us at consequential@cmu.edu.
This episode of Consequential was written by Lauren Prastien, with editorial support from Eugene Leventhal. It was edited by Eugene and our intern, Ivan Plazacic. Consequential is produced by Eugene, Lauren, Shryansh Mehta and Jon Nehlsen.
This episode references an episode of NPR’s Planet Money podcast, Class Central’s “By The Numbers: MOOCs in 2018” report, and Lindsay McKenzie’s 2019 article “Rift Over State Reciprocity Rules” from Inside Higher Ed.
S1 E6: Staying Connected
Lauren Prastien: I want you to think about your favorite piece of media about the future. It can be a movie, a book, a television show. A graphic novel. It can even be a song.
Where does it take place?
It’s a city, isn’t it?
If you think about just about any piece of pop culture about the future, particularly anything that’s come out in the last 25 years, be it utopian or dystopian, especially if it’s about robots or artificial intelligence, it takes place in a city. 1984, Fahrenheit 451, The Matrix, Blade Runner, Her, Altered Carbon, Gattaca, Minority Report, The Jetsons, I - Robot, Metropolis. Even The Flaming Lips song “Yoshimi Battles The Pink Robots,” one of my personal favorite pieces of media about the future, is guilty of this. After all, Yoshimi literally works for the city.
And if there is a rural setting, it’s usually an indication of a situation of extreme poverty or a nostalgic attachment to the past. So, sure, The Hunger Games doesn’t take place exclusively in the Capitol, but that is where all the prosperity and technology is. And Westworld is set in a rural playground visited by tourists coded as citydwellers and run by people who live in a nearby city. Face it, when we think about the future, we picture cities. And that idea is really, really problematic.
This is Consequential: what’s significant, what’s coming, and what we can do about it. I’m Lauren Prastien and I’ll be your main tour guide along this journey. You’ll also hear the voices of our many guests as well as of your other host.
Eugene Leventhal: Hi, I’m Eugene Leventhal. I’ll be joining throughout the season to take a step back with Lauren and overview what was just covered, to talk policy, and to read quotes. I’ll pass it back to you now Lauren.
Lauren Prastien: Consequential is recorded at the Block Center for Technology and Society at Carnegie Mellon University. Established in 2018 through a generous gift from Keith Block and Suzanne Kelley, the Block Center is dedicated to investigating the economic, organizational, and public policy impacts of emerging technologies.
Today, we want to know: Is there a rural-urban divide? And if there is, how can technology help us overcome it, rather than widen it?
So, the rural-urban divide is a blanket term intended the encapsulate the political, economic and cultural differences between the rural and urban populations of the United States. Its narrative is relatively simple: as U.S. cities flourish and more people move there, rural areas languish. In particular, much of the discourse on this divide pertains to widening gaps in employment and income.
Real quick, here are some important stats to keep in mind: Today, nearly half of the world’s population lives in urban areas, and by 2050, the United Nations expects this to increase to 66%. And while the U.S. Census Bureau found in 2015 that poverty rates are higher in urban areas than rural areas, the median household income for rural households was about 4 percent lower than the median for urban households. Additionally, United States Department of Agriculture’s Economic Research Service found that since 2012, the rural unemployment rate has exceeded the urban unemployment rate and prime-age labor-force participation rates have remained depressed in rural areas.
So is there a rural-urban divide?
Eugene and I spoke to an expert on this very subject here at the Block Center, to learn how real this sense of a divide is, what role technology is playing in this divide and what today’s discourse on these issues might be leaving out.
Richard Stafford: I married a coal miner’s daughter, by the way, and I’m still married to her. So, uh, the fact is that when she was born and her dad was a coal miner, you would look at a County like Fayette which is here in Southwestern Pennsylvania, right next to Greene County where I grew up. And there were probably 10, 12,000 miners at work. Today, there’s probably 400. They’re mining almost the same amount. What happened?
Lauren Prastien: That’s Richard Stafford. He’s a Distinguished Service Professor at Carnegie Mellon. Prior to coming to CMU, Professor Stafford served as the Chief Executive Officer for the Allegheny Conference on Community Development. And now, using his civic experience, Professor Stafford is looking at how public policy can respond to the societal consequences of technological change.
Richard Stafford: Automation. That’s what happened. Uh, so that whole impact of those jobs is still being felt and still being resented.
Their feeling is much stronger than you might suspect that we’re being left behind. And that characterizes, I think that the rural small town feeling looking at the city and thinking, yeah, well everybody cares about the city and they get all the jobs and you know, we’re being left behind. And it’s aggravated in our region, in the Pittsburgh region. If you look at the rural areas and how, what happened there and what prosperity was there had to do with these basic industries that have disappeared. Steel’s the obvious, biggest example. Steel was dependent on coal. Where did coal come from? Coal came from the rural areas. Okay. What happened to coal?
Lauren Prastien: In 2016, the International Institute for Sustainable Development released a report titled “Mining A Mirage? Reassessing the shared-value paradigm in light of technological advances in the mining sector.” It’s a mouthful, but let me give you the bottom line: The report found that a lot of the coal mining process has already been automated, from the trucks that haul the coal to the GIS systems that power mine surveying, and in the next 10 to 15 years, automation will be likely to replace anywhere from 40 to 80 percent of workers in a coal mine. And while automating a lot of these processes and even moving away from using coal as an energy source does have positive side-effects - it’s more environmentally-friendly and it’s also safer for employees working on these sites - it does mean that many regions will lose an industry that is a cornerstone of their economy.
And this goes further than coal. A lot of other rural industries have seen massive employee displacement as a result of artificial intelligence and enhanced automation. According to the U.S. Census Bureau’s American Community Survey, these are some of the top sectors filled by the civilian labor force in rural counties: manufacturing, retail trade, agriculture, mining, construction, transportation, warehousing and utilities. Yeah. A lot of the sectors that come up when we talk about where automation is going to take place.
But employment is just one facet of this issue.
Richard Stafford: When I think of the rural-urban divide, I think of the accessibility to healthcare to education to the kinds of basics in life that there’s a big difference in. So if you think about transportation, for example, in some rural areas, and you think about autonomous vehicles, well, the future of autonomous vehicles is going to be largely dependent on the ability of the communication system to communicate with that vehicle. Right now in Greene County, you can’t get cell phone connection in Western Greene County. So let alone high-speed Internet for the kids that, if they were going to join the future workforce, it would be nice if they could do their homework on the Internet, like all the kids in the urban area.
Lauren Prastien: But it’s also crucial to take into account that these problems aren’t exclusively rural issues.
Richard Stafford: Now, having said all that, by the way, there’s huge similarities between rural and small town disadvantages as technology progresses to areas of the city that have the same problem, right? To whether it’s Internet access or health care access or whatever. So in a lot of ways, while there is a rural-urban divide, I think we need to be careful about thinking of it as too distinct. We need to think in a sense for whatever area that you need to prosper, we need to think of the haves and have-nots.
Lauren Prastien: And according to Professor Stafford, a really great place to direct that focus is broadband Internet.
Richard Stafford: If you think of high speed Internet access as a utility, which is what it should be thought of today, we went through electricity becoming a utility, right? If you look historically, huge disadvantage right now in rural areas. And it’s a very simple thing because in the sense to understand because we’re telecommunications companies can make money is density. Rural areas by definition aren’t that dense. So how do we overcome that? How do we find a way from a public policy standpoint to redistribute in some acceptable way because that scares redistribution scares people that have! In some acceptable way as we have in electricity. So that the benefits can be there for those families and those kids that are growing up in rural areas to be part of the prosperity that supposedly AI and technology, technological development will provide. It’s a big issue and it will only be solved at a public policy forum. It won’t be just a matter of leave it to the free market.
Lauren Prastien: So what would that look like? Stay with us.
Hey. Remember this? [Dial-up modem sound effect]
Karen Lightman: I remember that sound and waiting and the anticipation of getting online and then you're about to load an image. And I mean it's...I get that. And what's really amazing to me is now we have Fios and Xfinity and you know, all this. And we live in an area where we have access to pretty good high-speed broadband. And there's a promise with 5G of going in even faster.
Lauren Prastien: That’s Karen Lightman. She’s the Executive Director of the Metro21: Smart Cities Institute at Carnegie Mellon, where her work looks at the use of connected, intelligent infrastructural technologies for improving sustainability, safety and quality of life. And a huge part of that depends on access to broadband.
Karen Lightman: But there's a good chunk of the United States, the world that doesn't have access to high speed broadband.
Lauren Prastien: In a 2018 study, Microsoft found that 162.8 million people in the United States lack regular access to broadband Internet. And according to the FCC, the option of using broadband isn’t even available to 24.7 million Americans, more than 19 million of which are based in rural communities. For some perspective: imagine if everyone in the entire State of New York couldn’t get on the Internet.
And this has greater implications than not being able to stream Netflix. According to the US Bureau of Labor Statistics, the highest unemployment rates in the US are frequently associated with counties with the lowest availability of broadband Internet.
Karen Lightman: So that means people can't telecommute. That means that if there is, you know, an emergency where they have to get information out, like there's a flood alert in a low lying area, that means that are not getting that information because a lot of it is digital and there's an assumption and so we could do better. I think that's the bottom line.
Lauren Prastien: According to the most recent data from the FCC’s Broadband Task Force, 70% of teachers in the United States assign homework that requires broadband access to complete. And by the way, this data is from 2009. It’s probably much, much higher today. So why is this happening?
Karen Lightman: When we had infrastructure investments in like the creation of highways, right? So there was a huge investment and the government is deciding that highways are really important, right? But the whole backstory on why that was there, you know, those military applicate uh, implications. But there was an investment by the federal government saying that this kind of infrastructure of connecting communities is important and we're going to make that investment. We have lights, right? So we had, you know, investment in electricity so that we could have lights, we could have, you know, electricity in our homes. Phones. So there were utilities, public utilities, and yet with broadband it's this fuzzy area that it's sort of regulated but sort of not. And it's mainly driven by investments by privately-held mega companies, right? I'm not going to name names, but they know who they are and their focus is profit, right? And it's not a public utility, so it's not like water and electricity, but maybe it should be considered that way.
Lauren Prastien: But what happens when we make broadband a public utility? Well, look no further than Chattanooga.
Before Chattanooga made high-speed Internet a public utility, its Downtown area had pretty good access to privately-owned broadband - so think Comcast and AT&T - but once you left the Downtown area, and particularly once you got into the more rural areas surrounding Chattanooga, there was really, really spotty or just completely non-existent broadband access. And because these were really small markets, the private broadband companies that covered the Greater Chattanooga area didn’t consider building out the infrastructure in these areas to be a worthwhile investment. Think the head and the long-tail.
Karen Lightman: And so they made the investment, they put in the fiber and they own it.
Lauren Prastien: So it’s important to note that in 1938, the Tennessee Legislature set up the Electric Power Board of Chattanooga, or EPB, as an independent entity to provide electricity to the Greater Chattanooga area. So already, Chattanooga’s electricity was a publicly-owned utility.
And so in 2009, the EPB widened its focus to broadband. With the help of a $111 million federal stimulus grant from the Department of Energy and a $169 million loan from the Chattanooga City Council, the EPB developed its own smart grid. And private Internet providers panicked. They sued the City of Chattanooga four times, and even tried introducing more competitive packages to dissuade Chattanoogans from using the publicly-owned broadband. But by 2010, Chattanooga’s residential symmetrical broadband Internet was operating at 1 gigabit per second, which was, at the time, 200 times faster than the national average.
Karen Lightman: Understanding the ROI, so looking at the examples, like what happened in Chattanooga is seeing that if you make a big investment, it's not trivial, then there is an economic development boost to a community. I think that's where the argument needs to be made.
Lauren Prastien: And the ROI was incredible. By adopting the first citywide gigabit-speed broadband in not just the United States, but the entire Western Hemisphere, Chattanooga spurred economic growth in not just the city itself, but the entirety of Hamilton County. According to an independent study by researchers at the University of Tennessee at Chattanooga and Oklahoma State University, EPB’s smart grid created and maintained an extra 3,950 jobs in its first 5 years of implementation. In a 2016 article in VICE, the journalist Jason Koebler called Chattanooga “The City That Was Saved by the Internet.” Because not only did the City rather swiftly break even on its investment, it also saw a strong reduction in unemployment and got a new identity as The Gig City, incentivizing the growth of new businesses and attracting younger residents to a region that was once seeing a pretty serious exodus.
Currently, 82 cities and towns in the United States have government-owned, fiber-based broadband Internet. And while there have been some areas that have experimented with municipal broadband and not seen the success of Chattanooga, the fact is that the lack of broadband availability is keeping people from participating in not just new educational or work opportunities, but very basic aspects of our increasingly connected world. And right now, there’s very little national oversight on making sure that all Americans have access to a service that is becoming as vital a utility as electricity.
Karen Lightman: It's like the wild west and it's not consistent. There's no, the federal, like I said, the federal government's not playing a role.
Lauren Prastien: Lately, we have seen the development of some legislative solutions to address this on the federal level, like the ACCESS BROADBAND Act. ACCESS BROADBAND is an acronym, and it’s even better than the DASHBOARD Act’s acronym we talked about with reference to data subject rights. So get ready. Actually, Eugene. Get over here. Come read this.
Eugene Leventhal: All right. Here goes. ACCESS BROADBAND stands for Advancing Critical Connectivity Expands Service, Small Business Resources, Opportunities, Access and Data Based on Assessed Need and Demand.
Lauren Prastien: Quick aside: Whoever has been coming up with these really amazing and really ambitious acronyms for these bills, we appreciate your hard work.
But anyway - the bill aims to promote broadband access in underserved areas, particularly rural areas that don’t quite fit the “head” category in the “head and long-tail” framework. It would also establish an Office of Internet Connectivity and Growth at the National Telecommunications and Information Administration, which would help to provide broadband access for small businesses and local communities, as well as provide a more efficient process to allow small business and local governments to apply for federal broadband assistance. So far, it passed the House of Representatives back in May, and it’s been received by the Senate and referred to the Committee on Commerce, Science and Transportation.
The federal assistance aspect of this bill could be really promising. Because the fact is that high-speed broadband requires major investments in infrastructure for 5G technology to work, and that’s often really expensive.
Karen Lightman: It’s not just laying down a new piece of fiber through a conduit pipe. It’s also these, basically be changing kind of the cell towers that we now see on rebuilding. They’d actually be, they have to be, in order to have that kind of Internet-of-things capability, they need to be lower to the ground and they are large for the most part, and they are shorter distance from each other.
Lauren Prastien: So, it’s a big investment, which can be discouraging to both the public sector and private companies alike. In addition to increasing federal support for these initiatives, Professor Lightman has also seen a lot of value in using smaller-scale deployments, from both the public sector and from private companies, to gain public trust in a given project and troubleshoot potential issues before major investments are made.
Karen Lightman: Pittsburgh is a neat city because we’ve got all these bridges. We’ve got a lot of tunnels, we’ve got a lot of hills and valleys. The joke is that it, Pittsburgh is a great place for wireless to die, so it’s a great test bed.
Lauren Prastien: And when it comes to facilitating these deployments, she sees a lot of value in the role of universities.
Karen Lightman: So that’s where the role of a university and a role of Metr 21 to do the deployment of research and development. And do a Beta, do a time-bound pilot project with a beginning and an end in a way to measure it and to see yes this works or no, we need to go back and tweak it. Or this was the worst idea ever! And I think what’s also unique about the work that we do, and this is pretty unique to Metro21, is that we really care about the people that it’s affecting. So we have social decision scientists that work alongside our work. We have designers, we have economists. So we’re thinking about the unintended consequences as long as, as well as the intended. And that’s where a university has a really nice sweet spot in that area.
Lauren Prastien: With these pilot projects, Professor Lightman aims to keep the community informed and involved as new technologies become implemented into their infrastructure. Which is great, when we think about some of the problems that come along when a community isn’t kept in the loop, like we saw in episode 4.
And while broadband access is a really vital part of being able to ensure that people aren’t being left behind by the digitization of certain sectors - like what we’ve seen in education - the issue doesn’t just boil down to broadband. And broadband isn’t a silver bullet that’s going to solve everything. But the implementation of a more equitable broadband infrastructure could assist in helping to close some of the more sector-specific gaps that are widening between rural and urban areas - or between haves and have-nots regardless of region - and are contributing to this narrative of a rural-urban divide. Because often, these issues are all linked in really complex, really inextricable ways.
Like how broadband access factors into healthcare. And right now, in rural regions, access to quality healthcare is becoming more and more difficult. According to the US Department of Health and Human Services’ Health Resources and Services Administration, of the more than 7,000 regions in the United States with a shortage of healthcare professionals, 60% are rural areas. And while Professor Lightman has seen some promising breakthroughs in telemedicine and sensor technology to fill some of these gaps, you guessed it: they need high-speed Internet to work.
Karen Lightman: There's also healthcare and the idea of hospitals are closing and a lot of these communities, so we have the technology for telemedicine. I mean, telemedicine is so amazing right now that, but you need internet, you need high speed, reliable internet. In order to, if you’re having a face to face conversation with a doctor or maybe you have sensor technology to help with blood pressure or diabetes and that information can be, you know, sent over the Internet remotely even. And that technology exists. But if there's no secure Internet, it's not gonna it doesn't work. There are a lot of other issues at play here, from the impact of automation on a region’s dominant industry to the lack of availability of services that would normally help people transition to a new career when that industry goes away in a more urbanized setting.
But addressing this is not a matter of just packing it all up and saying everyone needs to move to a city. Because not everyone wants to live in the city. And that should be fine. It should be an option. Because by the way, cities don’t automatically equal prosperity. In his lecture at the annual meeting of the American Economic Association earlier this year, MIT economist David H. Autor found that not only do cities not provide the kinds of middle-skill jobs for workers without degrees that they once did, they’re also only good places for as few as one in three people to be able to live and work.
So when you picture the future, I don’t want you to just picture cities. And when we talk about extending these opportunities more equitably into rural areas, I also don’t want you to think of this as cities bestowing these developments on rural communities. Because, one, come on. It’s condescending. And two, that’s just not how it’s playing out.
A lot of really incredible breakthroughs and a lot of thought leadership related to using tech for social good and preparing for the future of work aren’t coming out of cities.
I’ll give you a great example in just a moment, so stay with us.
So, Greene County has come up a few times in this episode. And real quick, if you’re not familiar with it, let me paint a picture for you. The cornerstone of the keystone state, it’s the southwestern-most county in Pennsylvania, sitting right on our border with West Virginia. It’s about 578 square miles. In case you’re wondering, that’s double the size of Chicago, or a little bit bigger than one Los Angeles. The city, not the county. It has about fifteen public schools spread out over five districts, three libraries, and a small, county-owned airport.
Also, it’s home to the Greene River Trail along Tenmile Creek which is, in my humble opinion, a really pretty place to hike.
Its county seat, Waynesburg, is home to Waynesburg University. It’s a small, private university with a student population of around 1,800 undergraduates and 700 graduate students. Some of its most popular majors are nursing, business administration and criminal justice. And under the leadership of its President, Douglas G. Lee, the University has received a lot of attention for the economic outcomes and social mobility of its graduates from institutions like Brookings and US News and World Report.
We spoke to President Lee about the ways that automation, artificial intelligence and other emerging technologies are going to change the way that non-urban areas - and particularly universities in these areas - can respond in such a way that they feel the benefits of these changes, rather than the drawbacks.
Douglas Lee: I grew up in this area and I saw what happened to many of the steelworkers when they lost their jobs and how hard it was for those folks to adapt and retrain into another position. And you see that in the coal industry as well today. So, linking this type of education to the workforce of the future I think is going to be critical.
Lauren Prastien: And according to President Lee, a huge part of that reframing is also adapting how we look at the role of educational institutions in the lives of their students, alumni and the communities they occupy.
Douglas Lee: So it's really about educating all of these young people to have, be lifelong and encouraging that and building that into a culture. And I think higher education needs to play a significant role in that and looking for those ways that you continue to grow and develop that concept as well because it's not just four years or six years or seven years in, year out it's lifetime now. And engaging in that lifetime experience, whether it's with your alumni, whether it's members of the community or in a larger sense, people that have an interest in a specific mission purpose in what you're educating at your university and plugging them into that.
Lauren Prastien: And this is going to factor a lot to our new understanding of how and when we acquire skills. Because regardless, where you choose to live shouldn’t preclude you from prosperity. A lot of really incredible breakthroughs in technologies are moving us into the future, and entire populations don’t deserve to be left behind. So, Eugene, how do we bridge these kinds of divides, be they rural and urban or have and have not?
Eugene Leventhal: The first step that should be taken is building community, especially with those who live in the areas that do not get the same access to resources or infrastructure. In order to make sure any solutions are as effective as possible, those individuals who are most affected need to be included in the decision making process in some capacity. Focusing on community can help understand how to meaningfully bring residents into the conversation.
We heard of the importance of broadband access and the comparison to how people didn’t have easier access to electricity until it became a public utility. It is important to seriously consider whether the role of high-speed internet qualifies it for becoming a public utility. If not, then it’s still crucial to have actionable plans for how broadband can be provided to all citizens. Ensuring high-speed internet for everyone would help raise the quality of education that students receive as well.
Lauren Prastien: Next week, we will be discussing the impact of automation on the creation and elimination of jobs and industries, with a focus on how policymakers, educational institutions and organized labor can prepare potentially displaced workers for new opportunities. We’ll also discuss the “overqualification trap” and how the Fourth Industrial Revolution is changing hiring and credentialing processes. Here’s a preview of our conversation with one of our guests next week, Liz Schuler, the Secretary-Treasurer of the AFL-CIO:
Liz Schuler: How do we have a conversation at the federal level to know what the needs are going to be and approach it in a systematic way so that our planning and our policy making can mirror what the needs of the future workforce are going to be and not have pockets of conversation or innovation happening in vacuumd in different places. And so the labor movement can be a good connective tissue in that regard.
Lauren Prastien: I’m Lauren Prastien,
Eugene Leventhal: and I’m Eugene Leventhal
Lauren Prastien: and this was Consequential. We’ll see you next week.
Eugene Leventhal: Consequential was recorded at the Block Center for Technology and Society at Carnegie Mellon University. The Block Center was established to examine the societal consequences of technological change and create meaningful plans of action. To learn more about Consequential, the Block Center and our faculty, you can check out our website at cmu.edu/block-center or follow us on Twitter @CMUBlockCenter. You can also email us at consequential@cmu.edu.
This episode of Consequential was written by Lauren Prastien, with editorial support from Eugene Leventhal. It was edited by Eugene and our intern, Ivan Plazacic. Consequential is produced by Eugene, Lauren, Shryansh Mehta and Jon Nehlsen.
This episode references the 2014 revision of the United Nations’ World Urbanization Prospects report, the US Census Bureau’s 2014 American Community Survey, a 2019 report from the United States Department of Agriculture’s Economic Research Service on Rural Employment and Unemployment, a 2016 report from the International Institute for Sustainable Development on automation in mining, Microsoft’s 2018 report “The rural broadband divide: An urgent national problem that we can solve,” a 2009 report from the FCC’s Broadband Taskforce, 2019 employment data from the US Bureau of Labor Statistics, Jason Koebler’s 2016 article “The City That Was Saved by the Internet” for VICE, 2018 data from HRSA on healthcare shortage areas and David H. Autor’s lecture at this year’s American Economic Association. The sound effect used in this episode was from Orange Free Sounds.
S1 E7: A Particular Set of Skills
Lauren Prastien: In 2018, the World Economic Forum released a report saying that by 2022, automation is expected to eliminate 75 million jobs. But, it’s also expected to create another 133 million new jobs.
So what would that look like? How can a technology that replaces a job create even more jobs? Let me use a really simplified example of how disruption can turn into job creation.
In the 2005 movie Charlie and the Chocolate Factory, Charlie Bucket’s father works at a toothpaste factory where his sole responsibility is screwing the caps onto tubes of toothpaste. That is, until a candy bar sweepstakes absolutely bamboozles the local economy.
Excerpt: The upswing in candy sales had led to a rise in cavities, which led to a rise in toothpaste sales. With the extra money, the factory had decided to modernize, eliminating Mr. Bucket’s job.
Lauren Prastien: There’s that first part of the prediction. Mr. Bucket gets automated out of his job. But don’t worry, because a little later in the movie, Charlie’s father gets a better job at the toothpaste factory...repairing the machine that had replaced him.
It would be irresponsible - and also flat-out wrong - for me to say that this process is absolutely organic. You need interventions in place to make sure that the individuals displaced by technology are able to find new, meaningful, well-paying occupations. But what are those interventions? And who is responsible for enacting them?
This is Consequential: what’s significant, what’s coming, and what we can do about it. I’m Lauren Prastien and I’ll be your main tour guide along this journey. You’ll also hear the voices of our many guests as well as of your other host.
Eugene Leventhal: Hi, I’m Eugene Leventhal. I’ll be joining throughout the season to take a step back with Lauren and overview what was just covered, to talk policy, and to read quotes. I’ll pass it back to you now Lauren.
Lauren Prastien: Consequential is recorded at the Block Center for Technology and Society at Carnegie Mellon University. Established in 2018 through a generous gift from Keith Block and Suzanne Kelley, the Block Center is dedicated to investigating the economic, organizational, and public policy impacts of emerging technologies.
Today, we’re talking about skills, displacement and overqualification. So stay with us.
So in the case of Charlie and the Chocolate Factory, automation took on a routinized task like screwing on toothpaste caps, and gave Mr. Bucket a more interesting and better-paying job. And historically, we’ve actually seen things like this happen. The term computer used to refer to a person, not a device. And while we no longer need people working through the minutiae of calculations that we’ve now more or less automated, the Bureau of Labor Statistics says that the computing and information technology sector is one of the fastest-growing industries in terms of employment today.
Or think of it this way: The invention of the alarm clock meant that there was no longer a need for a really unfortunately-named job called a “knocker-upper,” which was a person who was paid to go around the neighborhood, banging on doors to wake people up. So, no more “knocker-upper,” but now you need people designing alarm clocks, assembling them, selling them, repairing them, you get the idea.
And sometimes, technological disruption made new jobs that were a lot, lot safer. Back before phlebotomists were a thing and when blood-letting was a lot more common, you needed someone to go out and find leeches to draw blood. Leech collector was a real job, and, uh...no thanks.
But as technology took away these jobs, the skills required for the jobs it created in their place weren’t always the same. A person who was really good at banging on doors and waking people up might not be that great at engineering alarm clocks. Someone with the stomach for wading into rivers to collect leeches might not have the skills or even the desire to draw blood at a hospital.
So what are skills? According to Merriam-Webster, a skill is “a learned power of doing something competently.”
Napoleon Dynamite: You know, like nunchuck skills, bow hunting skills, computer hacking skills. Girls only want boyfriends who have great skills.
Lauren Prastien: Napoleon Dynamite’s onto something here. Our skills are how we demonstrate our suitability for a given position, because they’re what we need to fulfill the obligations of that position, be it the nunchuck skills that Napoleon Dynamite needs in order to be a good boyfriend, or, say, the technical proficiency Mr. Bucket needs to repair the robot that originally replaced him. Because you need, in the words of the Beastie Boys, “the skills to pay the bills.”
Skills are something that we usually hone over the course of our career. In a traditional model, you’ll gain the basis of those skills through your education, be it in K-12 and post-secondary institutions, at a trade school or through a working apprenticeship. You’ll usually then have some piece of documentation that demonstrates that you learned those skills and you’re proficient in using them: a diploma, a certification, a set of test scores, or even a letter from the person you apprenticed under saying that you can, indeed, competently perform the skills you learned during your apprenticeship.
Liam Neeson: I can tell you I don't have money. But what I do have are a very particular set of skills, skills I have acquired over a very long career, skills that make me a nightmare for people like you.
Lauren Prastien: And like Liam Neeson’s character in Taken just said, you’ll further hone those skills throughout your life, be it over the course of your career or even in your spare time. But what happens when a skill you’ve gone to school for and then used throughout a career becomes automated? Last week, we mentioned that automation has infiltrated industries like mining and agriculture, often making these industries a lot safer and a lot less environmentally harmful. But where does that leave the workers who have been displaced?
According to the World Economic Forum, transitioning 95% of at-risk workers in the United States into new jobs through reskilling could cost more than $34 billion. Lately, we’ve seen some efforts in the private sector to support reskilling in anticipation of the greater impacts of artificial intelligence and advanced automation. In 2018, AT&T began a $1 billion “Future Ready” initiative in collaboration with online educational platforms and MOOCs, which you may remember from our fifth episode are massive open online courses, in order to provide its workforce with more competitive and relevant skills as technology transforms the telecommunications industry. Earlier this year, Amazon announced a $700 million “Upskilling 2025” initiative to retrain a third of its workforce for more technically-oriented roles in IT and software engineering. And Salesforce has rolled out a suite of initiatives over the past few years focused on reskilling and upskilling, such as the “Vetforce” job training and career accelerator program for military service members, veterans and their spouses. But the World Economic Forum has found that the private sector could only profitably reskill about 25% of the workers at-risk for displacement, which indicates that this isn’t something we could rely exclusively on the private sector to handle. According to Borge Brende, President of the World Economic Forum:
Eugene Leventhal: If businesses work together to create economies of scale, they could collectively reskill 45% of at-risk workers. If governments join this effort, they could reskill as many as 77% of all at-risk workers, while benefiting from returns on investment in the form of increased tax returns and lower social costs including unemployment compensation. When businesses can’t profitably cover costs and governments can’t provide the solutions alone, it becomes imperative to turn to public-private partnerships that lower costs and provide concrete social benefits and actionable solutions for workers.
Lauren Prastien: And when we talk about training the future workforce and reskilling a displaced workforce, it’s important to understand the values, needs and concerns of the workers themselves. And as the voice of organized labor, that’s where unions come in.
So Eugene and I spoke to the American Federation of Labor and Congress of Industrial Organizations, or AFL-CIO. As the largest federation of unions in the United States, the AFL-CIO represents more than 12 million workers across a variety of sectors, from teachers to steelworkers to nurses to miners to actors. This fall, the AFL-CIO announced a historic partnership with Carnegie Mellon University to investigate how to reshape the future of work to benefit all working people.
In this spirit, we sat down with Craig Becker, the AFL-CIO’s General Counsel, and Liz Shuler, the Secretary-Treasurer of the AFL-CIO, to understand where policymakers need to focus their efforts in anticipation of worker displacement and in powering the reskilling efforts necessary to keep people meaningfully, gainfully employed. Because according to Liz Shuler, automation isn’t necessarily bad for workers.
Liz Shuler: Even though we know that the forecasts are dire, but at the same time we believe it's going to be about enhancing more than it is replacing. That a lot of new jobs will emerge, but some of the older jobs actually will evolve, using technology and freeing up humans to actually enhance their skills and use their judgment.
Lauren Prastien: Both Becker and Shuler agree that the anxiety over the huge impact that enhanced automation and artificial intelligence could have is really more about the ways in which these technologies will displace employees and degrade the value of their work than about automation in and of itself.
Craig Becker: If you want workers to embrace change and play a positive role in innovation, they have to have a certain degree of security. They can't fear that if they assist in innovation, it's gonna lead to their loss of jobs or the downgrading of their skills or degradation of their work. So a certain level of security, so policies which lead to unemployment policy, robust minimum wage, so that workers understand that if they are displaced, they'll get a new job and it won't be a worse job.
Lauren Prastien: And when we think about having workers embrace these changes and ensuring that these new positions suit the needs and desires of the workforce, you need to be actually getting input from the workforce itself. And that’s where unions can play a powerful role in these initiatives.
Liz Shuler: How do we have a conversation at the federal level to know what the needs are going to be and approach it in a systematic way so that our planning and our policy making can mirror what the needs of the future workforce are going to be and not have pockets of conversation or innovation happening in vacuum in different places? And so the labor movement can be a good connective tissue in that regard.
Lauren Prastien: And part of the reason why Shuler believes that unions are uniquely positioned to be that connective tissue is due to their own history as a resource for worker training.
Liz Shuler: We've handled this before. We've worked through it and really the labor movement sweet spot is in helping workers transition and ladder up to better careers. And so we are actually the second largest provider of training in the country behind the U.S. military.
We're sort of the original platform for upskilling. And so I think the labor movement has a real opportunity to be a center of gravity for all working people who are looking to make transitions in their careers as technology evolves.
Lauren Prastien: In addition to providing reskilling opportunities themselves, the organized labor union has also made reskilling a priority in their collective bargaining agreements with the industries they work with. In 2018, the AFL-CIO’s Culinary Workers Union in Las Vegas made reskilling a central factor in their negotiations with the Casinos, requiring management to communicate to the union before implementing a new technology.
The same thing happened with the 2018 contracts resulting from the bargaining agreements with Marriott Hotels. Their ensuing agreement made sure that the union receives 165 days’ notice from the company any time it plans to automate a given process, allowing for worker input on the use of technology in this way. Additionally, and perhaps more powerfully, all the workers affected by the implementation of a new technology are then entitled to retraining to either work with this new technology or to take on a new position within Marriott.
However, according to a Bloomberg Law survey, only 3 percent of employers’ contracts with workers included language on worker retraining programs, down sharply from 20 percent in 2011. Which, again, drives home the point that private sector efforts may not be enough to maintain a skilled and gainfully employed workforce.
Craig Becker: I think what's paradoxical here, or perhaps perverse here, is that while there's a wide recognition of an increased need for training and upscaling and continuing education, both public investment and employer investment in workforce training is down. And that I think largely has to do with the changing nature of the employment relationship. That is, employers see their workforce as turning over much faster than it did in the past and therefore don't see the need to invest in their workforce.
Lauren Prastien: So when it comes to investing in the workforce, they believe that a good lens to be using is that of investment in infrastructure.
Craig Becker: There's a wide recognition by both employers and unions and some public officials that there hasn't been sufficient investment in physical infrastructure: roads, pipes, water supplies, and digital infrastructure. I think the same thing that if we're going to be successful in other sectors, we need to have those complementary forms of investment. Digital infrastructure is obviously now key to the operation of much of that fiscal infrastructure, whether it's a factory or a water system or a sanitation system or transport system. All are now guided and aided by digital infrastructure. And similarly, investment in human infrastructure, training of the people to operate that new physical and digital infrastructure. All are significant and all are important. And there's a deficit in all three areas right now.
Lauren Prastien: And like we said, this isn’t a small investment. But just like investments in physical and digital infrastructure show a strong payoff, investments in human infrastructure are just as valuable. In 1997, the Association for Talent Development, then called the American Society for Training and Development, launched a major research initiative to evaluate the return on investment in worker education and training. In 2000, they released a comprehensive study titled “Profiting from Learning: Do Firms’ Investments in Education and Training Pay Off?” And essentially, the answer was, yes. A lot. Companies that offered comprehensive training programs not only had a 218% higher income per employee than companies without formalized training, they also saw a 24% higher profit margin than those who spent less on training. So it’s better for both employees and employers.
And what’s really exciting here is that the ROI we get from investing in worker training might even be bolstered by disruptive technologies. Because the technologies that we often think about as displacing workers can even help in reskilling them. Like this example, from Liz Shuler, on how augmented reality can help workers assess their interest in and suitability for a given job, before they make the investment in undergoing the training necessary for that job.
Liz Shuler: I was just at a conference with the sheet metal workers and I actually took the opportunity to do some virtual welding. They had a booth set up their conference so that people could actually see what the technology is all about. And I also did a virtual lift so that you're using that technology before you even leave the ground to know whether you have the aptitude or the stomach to be able to get into, uh, one of those lifts and not be afraid of heights, for example. Let me tell you, it was very challenging. Those are the kinds of tools and innovation that our training programs get ahead of.
Lauren Prastien: But what’s important to keep in mind here is that this sort of investment presupposes that organized labor has ample time to anticipate the disruption and retrain workers before they’re merely automated out of their jobs, which is why these efforts cannot be reactive.
Craig Becker: The role of unions as a voice for workers has to start really before the innovation takes place. There has to be a role for worker voice in deciding what kind of innovation would work. How would this innovation fit into the workplace? How would it expand what workers can do? How can it make them more safe? So that, you know, the type of dialogue which has gone on between our commission and CMU and its faculty is exactly what has to be promoted by policy makers.
Lauren Prastien: But what happens when this doesn’t play out? Like we discussed last episode, we’ve seen the larger socioeconomic implications that come with a region losing the industry that employs a large amount of its residents to automation. And like we said, the transition from one job to another isn’t always organic, and it often involves building new skills that take time and money to acquire, be it from going back to school, learning a new software or operating system, or picking up a new trade. Sometimes, providing retraining opportunities themselves aren’t enough, especially when the transition from one industry to another can come with a decrease in income.
But when it comes to protecting workers while they transition to another job and gain the skills necessary to support that position, there are steps that policymakers can take. I’ll tell you what that might look like in a few moments, so stay with us.
Lee Branstetter: The US federal government has been subsidizing worker retraining for decades. It spent billions of dollars and a lot of the results have been fairly disappointing. I think what we've learned is that retraining is hard, right? And then once a worker acquires a skill, they need to be able to move that skill to where the demand for that skill exists. All these need to be in alignment for retraining to work.
Lauren Prastien: If that voice sounds familiar, it’s because you’ve heard it a few times already over the course of this season. But if you’re just joining us or if you can’t quite place it, let me help you out. That’s Lee Branstetter. He’s a former Economic Advisor to President Obama, and he currently leads the Future of Work Initiative here at the Block Center. And based on his work as an economist and a professor of public policy, Professor Branstetter thinks unemployment insurance isn’t the right route to go. Instead, he thinks we should be focusing on wage insurance.
Lee Branstetter: The payout would not work like unemployment at all. The payout in our unemployment insurance where a system works in the following manner: you lose your job, you get some money, it's supposed to tide you over until you get another job. The problem we're finding is that workers go through a disruptive experience generated by technology or globalization and they spent decades honing a set of skills that the market no longer demands. So they have no problem getting another job, but the new job pays less than half of what the old job paid. We don't have any way of insuring against that. And the private market is probably not going to provide this insurance on its own because the only people who would sign up for it are the people who are about to get disrupted. Imagine how health insurance markets would work if the only people who sign up for health insurance were the people who are about to get critically ill.
Lauren Prastien: And Professor Branstetter sees that a relatively inexpensive intervention like wage insurance could protect the workers most impacted by technological change.
Lee Branstetter: With a fairly small insurance premium, we could provide a pretty generous level of insurance to that small fraction of the workforce that are contending with these long term income losses. And they're huge. I mean, the long term income losses we're talking about are on the same order of magnitude as if somebody's house burned down. Now, any of these workers can go on the Internet and insure themselves against a house fire quickly, cheaply, and easily. They cannot insure themselves against the obsolescence of their skill, but it would be pretty easy and straightforward to create this kind of insurance.
Lauren Prastien: Like Professor Branstetter said in our first episode on industry disruption, we still don’t know the exact impact that technological disruption is going to have on the workforce. But government interventions like wage insurance could cushion that impact.
In addition to seeing difficulties associated with workers needing to reskill as certain skills become automated, another issue that we’re seeing in the current workforce relates to overqualification. In 2017, the Urban Institute’s Income and Benefits Policy Center found that as many as 25 percent of college-educated workers were overqualified for their jobs. In other words, a full quarter of the American workforce with a college degree didn’t actually need that college degree to gain the skills or do the work that their jobs required them to do. And this is a huge issue, considering the rising costs of higher education and the growing burden of student loan debt.
So what impact is widespread overqualification having on our workforce? We’ll talk about that in just a moment.
According to the Federal Reserve, Americans owe more than $1.53 trillion in student loan debt. That’s the second-highest consumer debt category in the United States, exceeded only by mortgage debt. And according to the Institute for College Access and Success, borrowers from the Class of 2017, on average, still owe $28,650 in student loans. So imagine for a moment, please, for just a second, what it might mean to find out that you didn’t even need that degree in the first place, or that an advanced degree that you went into even more debt to obtain might even keep you from getting hired.
Oliver Hahl: There's this whole literature in the field on overqualification once you have a job and how people feel they get less enjoyment from their job when they're overqualified and all this. We were asking something that surprisingly no one really had studied at all, which was do hiring managers reject someone that they perceive to be overqualified? And if so, why?
Lauren Prastien: That’s Oliver Hahl. He’s a professor of organizational theory and strategy at Carnegie Mellon, where his work looks at how perceptions of socioeconomic success impact the behaviors of employment markets. In particular, how hiring organizations perceive candidates who are, quote, unquote, overqualified. Which is becoming an increasing concern as more and more people are not only getting college degrees, but also getting advanced degrees. Today, workers with at least a bachelor’s degree make up 36% of the workforce, and since 2016, have outnumbered workers with just a high school diploma.
Oliver Hahl: So basically what we found on the first paper was perceptions of commitment, which is a term of art for academics, which basically just means means a couple things. One is that the job candidate is less likely to stay with the firm. The employers are less likely to kind of get as much effort out of them. So the more committed you are to the organization, the more you're willing to put the organization first.
Lauren Prastien: And as Professor Hahl continues to look at the impact of how organizations perceive a candidates’ qualifications, his research group found that there are some really interesting tensions cut along the lines of gender.
Oliver Hahl: So then a student of mine, Elizabeth Campbell, who's at the Tepper School, came and was like, I think this is related to gender. The literature or the way that it's discussed, even interpersonally about a commitment, organizational commitment for men tends to be about, are you committed to the firm? For women, tends to be about, are you committed to your career? So I already have that divergence about what we mean by commitment, opens the door for, oh, there could be different outcomes, right?
Lauren Prastien: And by the way, Campbell was right. Gender did have an impact on the perception of qualification, though maybe not the impact you’d expect.
Oliver Hahl: And so as we thought about it, you realize the more qualifications you show on a resume might threaten the firm in saying like, you might not be committed to the firm, but it actually shows more commitment to your career because you've invested more. And so it kind of works in that, in these opposite directions. In the preliminary test, that's what we found. A woman who's overqualified relative to someone who's sufficiently qualified tends to be selected more. Whereas men, it's the opposite. Men who overqualified tend to be selected less than someone who's sufficiently qualified.
Lauren Prastien: So yeah, if you’re a man and you’re overqualified, you’re probably not going to be selected for that position. And if you’re a woman and you’re overqualified, the hiring organization is going to favor you. Essentially, Campbell and Hahl have summed it up this way:
Eugene Leventhal: “He’s Overqualified, She’s Highly Committed.”
Lauren Prastien: Which stinks, whether you’re a man not getting picked for a position or a woman being hired for a position you’re overqualified for. And this doesn’t just negatively impact job candidates. According to Professor Hahl, this hurts organizations, too.
Oliver Hahl: The implication of this is from kind of a strategy standpoint of how to manage your human capital is the organization is leaving really qualified people out in the workforce that, that they could get a lot of productivity from.
Lauren Prastien: And so we asked Professor Hahl what forces are driving this trend and contributing to this disparity.
Oliver Hahl: The fetishization of the undergrad degree as opposed to doing an associates degree or developing a technical skill. Going and getting an undergrad degree where it's not, you know, teaches you to think really well and it's great. And I'm not, you know, I get paid by universities. I think universities are great, but I don't know that it's for as many people who are going to get those jobs.
Lauren Prastien: As we talked about in our episode about the education bubble, higher education has relied on prestige and scarcity for a long time to maintain its dominance in the skill acquisition market, but the deployment of new technologies that impact the way people are being educated and how they are gaining credentials could shake this up significantly. And Professor Hahl also sees these technologies shaking up hiring, and potentially helping to overcome the quote, unquote overqualification trap, particularly if we take a page from how medical schools go about candidate recruitment.
Oliver Hahl: The way medical schools match with their with medical students. This comes from my talking with my two brothers in law who are doctors. So, when they were getting out of their medical school and matching for their fellowship. Or not fellowship, it’s the residence for the next step. They list, they go around and you go around and interview once with a bunch of schools. You talked to a bunch of schools and get your sense on how they think of you and how you think of them. But then it’s blind. You don’t know how they rank you relative to other people and they don’t know how you rank them relative to other schools. And you make a list of your top schools and they make a list of their top students and some algorithm matches them.
Lauren Prastien: So Eugene, what have we learned today about protecting workers from the greater impacts of technological change and ensuring that they have the skills and ability to find meaningful, well-paying jobs during what’s being called the Fourth Industrial Revolution?
Eugene Leventhal: Well Lauren, we’re starting to see a bit of a trend arising when it comes to the policy responses relating to the types of questions that we’re bringing up in this season. That trend is the fact that there is no easy solution, there is no single easy, laid-out path ahead of policymakers in terms of what is the best way to deal with it.
What we do know is that we need to work with the groups that are being affected. That includes individuals who are already in the process of being displaced by automation or have already been displaced by automation. Or that can be relating to industries where we see a high chance of those industries becoming disrupted in the coming years. Situations where policymakers are not able to directly administer studies looking into how the workforce within their jurisdiction is being affected, it’s important to partner with either universities or nonprofits focused on workforce redevelopment to better understand how are individuals being affected and who specifically is at a high risk of being affected.
As we heard today, retraining is definitely an important area to focus on in terms of supporting workers as career landscapes changed. However, retraining should not be seen as a panacea to these issues. We also need to think of other elements of supporting our workforce, such as the wage insurance idea that Professor Branstetter spoke of. We also need to have a larger cultural shift around how we view both education and some career paths, as continuing the current status of overqualification isn’t good for anyone involved. And so we see the potential policy response here starting with understanding who is being affected and how, as well as thinking what are the necessary support systems that need to be in place for workers that will be affected but for whom retraining may not be a viable option in the short-run.
Lauren Prastien: Thanks, Eugene. Now that we’ve discussed the changes in the workforce, we’ll look at changes in the workplace. In particular, human-computer collaboration. Here’s a preview of our conversation with one of our guests next week, Parth Vaishnav, a professor of engineering and public policy here at Carnegie Mellon:
Parth Vaishnav: Okay, if we accept the idea that there’s going to be someone in the truck, who is going to be monitoring these systems, how is the job of that person going to be different from what truckers do today? And are there other things that truckers do right now? Things like basic maintenance, things like paperwork, things like coordinating delivery times with customers, which an autonomous system may not be able to do. Would autonomy create jobs that are completely different from the trucking job, but still involved truckers?
Lauren Prastien: I’m Lauren Prastien,
Eugene Leventhal: and I’m Eugene Leventhal,
Lauren Prastien: and this was Consequential. We’ll see you next week.
Consequential was recorded at the Block Center for Technology and Society at Carnegie Mellon University. The Block Center was established to examine the societal consequences of technological change and create meaningful plans of action. To learn more about Consequential, the Block Center and our faculty, you can check out our website at cmu.edu/block-center or follow us on Twitter @CMUBlockCenter. You can also email us at consequential@cmu.edu.
This episode of Consequential was written by Lauren Prastien, with editorial support from Eugene Leventhal. It was edited by Eugene and our intern, Ivan Plazacic. Consequential is produced by Eugene, Lauren, Shryansh Mehta and Jon Nehlsen.
This episode references the World Economic Forum’s “The Future of Jobs 2018” report, the Bureau of Labor Statistics’ Occupational Outlook Handbook, a 2019 article from World Economic Forum President Borge Brende titled “We need a reskilling revolution. Here’s how to make it happen,” a 2019 Bloomberg Law survey titled “Bargaining Objectives, 2019,” a 2000 study from the ATD titled “Profiting from Learning: Do Firms’ Investments in Education and Training Pay Off?”, a 2017 study from the Urban Institute titled “Mismatch: How Many Workers with a Bachelor’s Degree Are Overqualified for their Jobs?”, consumer credit data from the Federal Reserve, data from the Institute for College Access & Success’s Project on Student Debt and data from Georgetown University’s Center on Education and the Workforce. It uses clips from the 2005 movie Charlie and the Chocolate Factory, the 2004 movie Napoleon Dynamite and the 2008 movie Taken.
S1 E8: The Future of Work
Lauren Prastien: Does your boss trap you in endless conversational loops? Do they ask your opinion on something they’ve clearly already decided upon? Are they obsessed with efficiency?
I’m so sorry to tell you this: your boss might be a robot. At least, according to author and Forbes columnist Steve Denning. Back in 2012, he wrote an article titled, “How Do You Tell If Your Boss Is A Robot?” And while Denning was referring to a metaphorical robot, as in, your boss is just kind of a jerk and obsessed with the bottom line - he was also speaking to a very real anxiety that as artificial intelligence becomes more and more competent, we’re going to start seeing it in the workplace, whether we’re consciously aware of it or not.
And this anxiety over being bamboozled by a surprise robot, or even just having robots in the workplace in general, hasn’t gone away. If anything, it’s intensified. Just this year, the journalist Eillie Anzilotti wrote an article for Fast Company titled, “Your new most annoying overachieving coworker is a robot.” Yes, a literal robot this time.
And in the trailer for the third season of Westworld, a construction worker played by Aaron Paul sits on a girder, the city looming behind him, looking lonely as he eats his lunch beside the robot that is presumably his coworker. It bears a stunning resemblance to the famous Charles C. Ebbets photograph Lunch atop a Skyscraper. But while the eleven men in Lunch atop a Skyscraper share cigarettes and chat away on their break, looking exhausted but somehow also enlivened by each others’ presence, Westworld’s human and robot coworkers bear the same hunched, defeated posture. They can’t even seem to look at each other.
But will human-computer collaboration actually look like that? How accurate is our current cultural anxiety over - and perhaps even fascination with - the future of work?
This is Consequential: what’s significant, what’s coming, and what we can do about it. I’m Lauren Prastien and I’ll be your main tour guide along this journey. You’ll also hear the voices of our many guests, as well as of your other host.
EL: Hi, I’m Eugene Leventhal. I’ll be joining throughout the season to take a step back with Lauren and overview what was just covered, to talk policy, and to read quotes. I’ll pass it back to you now Lauren.
LP: Consequential is recorded at the Block Center for Technology and Society at Carnegie Mellon University. Established in 2018 through a generous gift from Keith Block and Suzanne Kelley, the Block Center is dedicated to investigating the economic, organizational, and public policy impacts of emerging technologies.
Last week, we talked about how artificial intelligence and automation could displace workers, and what interventions need to be in place to protect our workforce.
Today, we’re going to look at what happens when these technologies enter the workplace and even just our lives in general. This week is all about human-computer collaboration and the future of work. So stay with us.
So real talk: your newest coworker is probably not going to be a literal, nuts-and-bolts robot with a face and arms and legs. Aaron Paul’s character in Westworld is probably more likely to work alongside a semi-autonomous 3D-printer or a robotic arm designed for quickly laying bricks. He might even wear something like a robotic suit or exoskeleton, which allow users to safely lift heavy objects. But, no, he’s probably not going to be working alongside a human-like robot. It’s more likely he’ll just use an algorithm to help efficiently develop a projects’ schedule and reduce the likelihood of delays. Though, okay, let’s be fair here: Westworld is fiction, and a scheduling algorithm doesn’t make great television like a fully realized robot does, and it doesn’t give us that really stunning shot of Aaron Paul and that robot on their lunch break. But wait a hot second - why does a robot need a lunch break?
Anyway: human-computer collaboration isn’t a discussion that takes place entirely in the future tense. Because already, there are a lot of industries that aren’t computing or even computing-related where we are seeing a lot of human-computer collaboration. And one of them is trucking.
Parth Vaishnav: There is already relatively more automation in trucking than we see in passenger cars. Things like cruise control, things like adaptive cruise control have been rolled out in trucking and have been studied for quite some time.
Lauren Prastien: That’s Parth Vaishnav. He’s a professor of engineering and public policy at Carnegie Mellon, where his work looks at the economic and environmental implications of automation, as well as human-computer collaboration, in several industries, including trucking.
Parth Vaishnav: There are companies which are actually implementing technologies like platooning where one truck follows another truck and the following truck essentially has a driver monitoring the system. But so long as they are in a platoon, the following truck acts as if it's fairly autonomous and obviously it's a low level of autonomy, which means that something goes wrong, you always have a vigilant driver who's ready to intervene.
Lauren Prastien: Quick clarification on the use of the term platoon here: a platoon is essentially a group of vehicles that travel close together, wherein the lead vehicle sets the speed and direction for the group. It’s also called a flock. Because, you know, that’s essentially what flocks of birds do. But frankly, I prefer the word platoon. Because, come on.
But anyway, it’s a form of vehicle autonomy, though, like Professor Vaishnav said, it’s a fairly low level of autonomy. But the level of sophistication of automation in trucking is increasing pretty rapidly. In 2017, the journalist Alex Davies reported for Wired that the transportation startup Embark was hauling smart refrigerators between Texas and California using a fleet of self-driving trucks. Yes, there was a human driver in the cab, which is - at least right now - pretty important.
Like we said all the way back in our second episode on the black box, driving involves a really sophisticated algorithm. It’s not just step one, step two, step three, you have reached your destination. You’re taking into account other cars, drivers who might not actually be following the rules of the road, pedestrians, detours, stoplights, you get the picture. So the algorithms that power self-driving technology don’t just have to understand the rules of the road, they have to rely on the sensors that feed them input about everything happening around the vehicle, from weather conditions to traffic to potholes.
If you remember from our third episode on data subjects, you contributed a lot of the data that helps these algorithms interpret what those sensors are picking up. You know, while you were proving you weren’t a robot online.
John Mulaney: I’ve devised a question no robot could ever answer! Which of these pictures does not have a stop sign in it? What?!
Lauren Prastien: Right. Thanks, John Mulaney. And thanks, CAPTCHA.
But let me make two really quick clarifications about how this technology is being used. First of all, autonomous vehicles aren’t just on the road with nobody in them. You need a driver in the cab, in the same way you still need a pilot in the cockpit when you’re on autopilot.
And continuing this pilot metaphor to make this second clarification: the majority of autonomous trucking is going to take place on highways and in long-hauls, not in urban areas. Because, again: detours, pedestrians, potholes, you get the picture. Think of it this way: for most commercial airlines, about 90% of a flight is done on autopilot. But 99% of landings and 100% of takeoffs are done by a human pilot, not an autopilot system.
So in the case of trucking, if those humans in the cab are not going to be spending that time driving, what can they be doing?
Parth Vaishnav: Okay, if we accept the idea that there’s going to be someone in the truck, who is going to be monitoring these systems, how is the job of that person going to be different from what truckers do today? And are there other things that truckers do right now, things like basic maintenance, things like paperwork, things like, coordinating delivery times with customers, which an autonomous system may not be able to do? Would autonomy create jobs that are completely different from the trucking job, but still involved truckers? What kinds of jobs does that create and what skills do those people require relative to the skillset already exist among people who service trucks on long haul routes right now?
Lauren Prastien: And there’s a really interesting tension here when it comes to how integrating automation into the trucking industry could do a lot of good and cause a lot of harm. A 2015 analysis of US Census Bureau conducted by National Public Radio found that “truck driver” was the most common job in 29 states, including Pennsylvania, California and Texas. But while the integration of driverless technology could also endanger the jobs of the 1.9 million professional truck drivers currently on the road in the United States, it’s also worth noting that the American trucking industry is experiencing a driver shortage that could be remedied through increased automation.
Parth Vaishnav: About 30% of the cost of trucking is the cost of the driver, and so there's a strong economic case to actually reduce costs by making trucking autonomous. Related to that, the reason why the costs of drivers are so high is that it's a hard job to do. You have to spend time away from home, you have to spend very long hours behind the wheel where you alternate between dealing with tricky situations like getting the truck in and out of warehouses and rest stops. And boredom. So it's a hard job to recruit people into doing. The turnover rate is fairly high.
Lauren Prastien: It’s also important to consider that actually, the trucking industry isn’t just truck drivers, even today. In its 2015 analysis of the truck driver shortage, the American Trucking Associations reported that actually, some 7.1 million people involved in the American trucking industry today aren’t drivers. And in addition to having a really high turnover rate, the trucking industry is also facing a pretty significant demographic problem. According to Aniruddh Mohan, a PhD student in Engineering and Public Policy at Carnegie Mellon and one of the researchers working with Professor Vaishnav on this project, changing the roles and responsibilities of individuals in this industry might actually be critical to making sure this industry survives.
Aniruddh Mohan: I think one of the interesting things about the trucking industry is that there's simultaneously a massive shortage of drivers in coming years, but there's also a lack of diversity in the industry. So, most of the truck drivers are baby boomers and there’s a lack of millennials, younger generations participating. And one of the interesting things that we want to find out from this work is to see how the jobs might change, particularly with the introduction of automation, and whether technology might make those jobs more attractive to younger generations who are more technology savvy.
Lauren Prastien: So how soon is the trucking industry going to feel the impact of these changes?
Parth Vaishnav: I think panic is probably premature at this point. I think some of the players in the industry have also admitted that autonomous vehicles are not going to come barreling down the highways, at the end of this year, whatever it is, that some people claim. I think there is time, but also there is value in performing the kinds of exercises that that we're performing where you try and understand how the jobs are going to change on what timescales and start preparing people with it. The other thing that's important is, fleet operators and drivers who are actually exposed to the risk both in terms of risk of accidents, but also the risk of their jobs changing or going away, should be brought into the conversation. We should try to bring in all the stakeholders into the conversation sooner rather than later.
Lauren Prastien: So what will bringing stakeholders into that conversation look like? And how will worker input impact and even improve the way technologies are being used in the workplace? We’ll talk about that in just a moment.
Like we said last week, if you’re going to talk about worker interests and needs, a great place to look is to unions. When we spoke to last week’s guests Liz Shuler, the Secretary-Treasurer of the AFL-CIO, and Craig Becker, General Counsel to the AFL-CIO, about displacement, the subject of human-computer collaboration naturally came up. Because as we’re seeing technologies like artificial intelligence and enhanced automation enter into a variety of sectors, Becker and Shuler believe that this is also going to show us where the need for a human is all-the-more important.
Craig Becker: I think one of the consensus predictions in terms of job loss is it's in jobs that require a human touch - teaching, nursing - that you're not going to see large amounts of displacement. That there's something about the classroom with a human teacher, the hospital room with the human nurse, that’s just irreplaceable. So I think you have to understand the possibilities in this area as enhancing the understanding of those human actors.
Liz Shuler: We have seen a lot of talk around technology being sort of a silver bullet, right, in the classroom. I went to the consumer electronics show this last year in Las Vegas and I saw a lot of robots that were meant to be used in the classroom. And we had a member of the AFT along with us at the show. And he said, you know, this seems like a good idea on the surface, but I've seen it where you're in a classroom of 30 people, and sometimes 40 people as we've heard in Chicago right now during the strike. A robot being used in a classroom, in some cases for remedial purposes where maybe a student has been as a following the lesson plan as closely or quickly. The minute the robot scoots over to that student's desk, that student is targeted or is feeling vulnerable and there's a lot that goes along with that. Whether it's bullying or some kind of an emotional impact that, you know, people don't foresee.
Lauren Prastien: This example with robots in the classroom points to something really vital. When it comes to the technologies being implemented into a given industry, those technologies themselves are sometimes not being developed by someone who works in that industry. Don’t get me wrong, sometimes they are. But this example of an assistive robot that might actually alienate the student it’s intended to help is a really telling sign of what happens when workers in a given industry aren’t involved in the development and integration of these technologies. And this isn’t an extraordinary circumstance. It’s a scenario that the AFL-CIO is seeing a lot.
Craig Becker: There was a young robotics professor developing a robotic arm to assist feeding of patients. The professor was there along with two graduate students, and in the group from our commission was the executive director of the nurses’ union. So, you know, what ensued was a very, very interesting back and forth between the two students and the professor about their conception of how this tool, this robotic arm would be used, and the nurse's conception of what actually takes place when a nurse is assisting a patient to be fed and how it's much more than making sure that the food goes into the patient's mouth in terms of assessments that are going on at the same time. And also how the tool would likely be deployed by actual health care institutions.
Lauren Prastien: Last week, we talked about how bringing workers into the process of implementing innovations into their given industry is really vital to preventing displacement. But putting workers in conversation with technologists and trusting workers to know what they need is also really vital to making sure that these technologies are actually being utilized meaningfully and not, you know, just being parachuted into an industry, to take a term from our education episode.
Because a lot of the popular discourse on human-computer collaboration can ignore a really important detail: it’s not just the technology improving the efficacy of the human. A lot of the time, it’s also the human improving the efficacy of the technology. And in a moment, we’ll dig into that a little more. So stay with us.
From trucking to nursing to teaching, we can see that human-computer collaboration can look really, really different across industries. On an even more granular level, there are different forms of human-computer collaboration, and they have really different implications for the future of work, depending on which we choose to implement and prioritize. And when it came to figuring out what that might look like, we turned to an expert on the subject:
Tom Mitchell: The focus of this study, our marching orders in that study was to analyze and understand and describe the impact that AI would have on the workforce. And what we found was that the impacts were many, not just one, and some were positive, some were negative, some were hard to evaluate.
Lauren Prastien: That’s Tom Mitchell. He’s a professor of computer science here at Carnegie Mellon, where he established the world's first department of machine learning. He’s also the Lead Technologist at the Block Center, where his work pertains to machine learning, artificial intelligence, and cognitive neuroscience, and in particular, developing machine learning approaches to natural language understanding by computers, which we’ll explain very, very soon.
The study he’s referencing here is called Information Technology and the U.S. Workforce: Where Are We and Where Do We Go from Here? It was published in 2017, and it covers everything from future trends in technology to the changing nature of the workforce.
One of the biggest takeaways from both this study for the National Academies of Sciences, Engineering and Medicine and several other studies Professor Mitchell has published is the idea of a job as a series of tasks. And that’s where human-computer collaboration, sometimes also called human-in-the-loop AI systems, comes in, by assigning certain tasks to the computer, such that humans are then freed up to do other tasks. Which can either result in an enhanced worker experience by creating a more meaningful and stimulating job, or can eventually lead to job displacement, depending on how that’s split up. So we asked Professor Mitchell for a few examples of this.
Tom Mitchell: Suppose you have people employed who are making routine repetitive decisions. Like there's somebody at CMU who approves or doesn't approve reimbursement requests when people take trips, for example. Now, there is a policy and that person is looking at data that's online and making the decision based on that policy. They use their human judgment in various ways. But if you think about it, the computers in that organization are capturing many training examples of the form: here's the request for reimbursement with the details and here's the person's decision. And that's exactly the kind of decision-making training example that machine learning algorithms work from. Now obviously, with that kind of human in the loop scenario, we might expect over time the computer to be able to either replace if it's extremely good, or more likely augment and error check the decision making of that person, but improve the productivity of that person over time. So that's a kind of human in the loop scenario that I would call the mimic the human.
Lauren Prastien: If you remember from episode 2, machine learning algorithms work by taking in large quantities of data to be able to make inferences about patterns in that data with relatively little human interference. So in the case of the “mimic the human” scenario, you can have this really negative outcome where the computer learns how to do the task that more or less makes up your job, then does your job better than you, then essentially Single White Females you out of your job. But don’t worry. This isn’t the only way human-computer interaction can play out!
Tom Mitchell: If you think about our goal, which is to figure out how to use computers to make even better decisions and make humans even better at the work that they're doing instead of replace them in many cases, it's what we'd like. Then there's a different kind of approach that's actually one of our current ongoing research projects and I call it conversational learning. If you hired me to be your assistant, you might, for example, say whenever it snows at night, wake me up 30 minutes earlier. As a person, I would understand that instruction. Today, if you say to your phone, whatever snows at night, wake me up 30 minutes earlier, notice two things: One, the computer won't understand what you're saying, but number two, it actually could do that. It could use the weather app to find out if it's snowing and it could use the alarm app to wake you up.
Lauren Prastien: Hey. This sounds a little like the knocker-upper from our last episode. But right now, my phone - which is, remember, a computer - can’t actually do that. Watch. Whenever it snows at night, can you set the alarm thirty minutes earlier?
Phone: I’ve set an alarm for 7:30 PM.
Lauren Prastien: That’s not helpful.
Tom Mitchell: In our prototype system, the system says, I don't understand, “do you want to teach me?” Then you can say yes. If you want to know if it snows at night, you open up this weather app right here and you click on it and you see right here where it says current conditions, if that says S, N, O, W, it's snowing. If you want to wake me up 30 minutes earlier, you open this alarm app here and you tap on it and you say right here where the number is, subtract 30 from that. So literally you teach or instruct or program your computer the same way that you would teach a person. And so the idea is if you step back, you'll notice if I ask how many people on earth can reprogram their telephones to do new things for them, the answer is about 0.001% of humanity. Those are the people who took the time to learn the programming language of the computer. Our goal is instead have the computer learn the natural instruction language of the person.
Lauren Prastien: In addition to just being really, really cool, this is incredibly significant when we think about the kinds of barriers that exist today in terms of who has access to this technology, who is able to innovate with this technology and who is benefitting from the innovations in this technology.
Tom Mitchell: If we can make it so that every worker can program or instruct your computer how to help them, then instead of computers replacing people, the dominant theme will be how creative can the worker be in thinking of new ways to teach the computer to help the worker. So there's an example of a place where we have a social question about impact of AI, but part of the solution can actually be changing the underlying AI technology. So in general, I think one of the things I love about the Block Center, is it's going, we're going beyond the idea that the Block Center should guide policy makers. It's just as important that the Block Center identify these kinds of technology research opportunities that technology researchers can work on. And if they succeed, it will change the kind of social impact that AI has.
Lauren Prastien: To close, I asked Professor Mitchell what we need to keep in mind going forward as all this technology is rolled out. And his answer was pretty encouraging.
Tom Mitchell: It's not that technology is just rolling over us and we have to figure out how to get out of the way. In fact, policymakers, technologists, all of us can play a role in shaping that future that we're going to be getting.
Lauren Prastien: On that note, Eugene, what’s our takeaway this week, and where can policymakers start to approach the really unwieldy topic that is the future of work?
Eugene Leventhal: To add to what Professor Mitchell was just saying, I would go so far as to say that it’s not that all of us can play a role. The reality is that, like it or not, we all will play a role. And so what should policymakers specifically be doing at this point?
A good place to start is not immediately buying into the hype that robots and AI will change everything right away. Policymakers have been contended with non-AI driven automation for decades, especially in terms of manufacturing and textiles. From there, efforts need to be directed towards understanding what functions and roles across various will be disrupted in the next few years and which are likely to be disrupted in the coming decades. Convening people from the public and private sectors, including academic institutions, can both be helpful and overwhelming. The reality is that more resources need to be dedicated towards helping policymakers know what is most pertinent to their constituents. Partnering with nonprofits and universities can help lighten that burden.
In addition to thinking of roles where humans are getting automated away, it’s crucial to focus attention on the areas where AI can collaborate with humans for better ultimate outcomes. Automation is an unquestionable concern moving forward, but that doesn’t mean that the beneficial prospects of AI should be overshadowed. The better we understand where AI can help boost human productivity, the easier it will be to envision new roles that don’t currently exist. If there will be more jobs eliminated than created, then the gains from productivity could be used to help fund programs such as Wage Insurance, which we heard about from Professor Lee Branstetter in last week’s episode called “A Particular Set of Skills.”
Lauren Prastien: Thanks, Eugene. Next week, we’re looking at a sector that’s seeing a lot of the issues we’ve been discussing all season playing out in real-time - from algorithmic bias to technological disruption to the potential of displacement - and how decisions made about this industry could have serious implications for the standards of other industries in the future. And that’s healthcare. Here’s a clip from one of our guests next week, Zachary Lipton, a professor of business technologies and machine learning at Carnegie Mellon:
Zach Lipton: How can you use the tools of modern computer vision to not just do the main thing, which is to say, try to imitate what a radiologist does, but to help a radiologist in this context of like review and, uh, kinda like continue learning? So among other things we imagine is the possibility of using computer vision as a way of like surfacing cases that would be interesting for review.
Lauren Prastien: I’m Lauren Prastien,
Eugene Leventhal: and I’m Eugene Leventhal,
Lauren Prastien: and this was Consequential. We’ll see you next week.
Consequential was recorded at the Block Center for Technology and Society at Carnegie Mellon University. The Block Center was established to examine the societal consequences of technological change and create meaningful plans of action. To learn more about Consequential, the Block Center and our faculty, you can check out our website at cmu.edu/block-center or follow us on Twitter @CMUBlockCenter. You can also email us at consequential@cmu.edu.
This episode of Consequential was written by Lauren Prastien and was produced by Eugene Leventhal with editing support from our intern, Ivan Plazacic. Our executive producers are Shryansh Mehta and Jon Nehlsen.
This episode references Steve Denning’s article “How Do You Tell If Your Boss is a Robot?” for Forbes in 2012, Eillie Anzilotti’s article “Your new most annoying overachieving coworker is a robot” for Fast Company in 2019, Alex Davies’ article “Self-Driving Trucks are Now Delivering Refrigerators” for Wired in 2017, a clip from John Mulaney’s comedy special Kid Gorgeous, the American Trucking Associations’ “Truck Driver Shortage Analysis 2015” and NPR Planet Money’s 2015 study “The Most Common Job in Each State 1978 - 2014.”
S1 E9: Paging Dr. Robot
Lauren Prastien: Right now, a lot of the media coverage on AI in healthcare falls into two categories: feel-good robot success story or horrifying robot nightmare. Success: a last-minute decision to put a face and heart-shaped eyes on an assistive robotic arm named Moxi helps nurses with staffing shortages while making patients feel happier and more comfortable. Nightmare: A popular search engine amasses millions’ of people’s health records without their knowledge. Success: A surgeon-controlled pair of robotic arms stitches the skin of a grape back together. Nightmare: A widely-implemented algorithm in the American healthcare system is found to be biased against black patients. Success: Telepresence technology helps people in underserved areas talk to therapists. Nightmare: An American engineer intentionally designs a robot to break Asimov’s first law of robotics: “never hurt humans.” Success, nightmare, success, nightmare. Rinse, repeat.
Over the course of this season, we’ve talked about some of the issues in our current technological landscape, from algorithmic bias to industry disruption to worker displacement to the AI black box. Right now, they’re playing out in real-time in the healthcare sector, and the decisions we make about how these technologies are implemented here may have greater repercussions for how they’re used and regulated in other sectors. And when it comes to medicine, the stakes are pretty high. Because, remember, sometimes, this is literally a matter of life and death.
This is Consequential: what’s significant, what’s coming, and what we can do about it. I’m Lauren Prastien and I’ll be your main tour guide along this journey. You’ll also hear the voices of our many guests, as well as of your other host.
EL: Hi, I’m Eugene Leventhal. I’ll be joining throughout the season to take a step back with Lauren and overview what was just covered, to talk policy, and to read quotes. I’ll pass it back to you now Lauren.
LP: Consequential is recorded at the Block Center for Technology and Society at Carnegie Mellon University. Established in 2018 through a generous gift from Keith Block and Suzanne Kelley, the Block Center is dedicated to investigating the economic, organizational, and public policy impacts of emerging technologies.
This week, we’re talking about healthcare and tech. So stay with us.
Lauren Prastien: I feel like just about everyone has a really harrowing or just kind of uncomfortable story about a time a doctor was far too clinical or dismissive with them. When I was fourteen, I went in for a physical and my GP kept insisting my acne would go away if I just quit smoking. Which was weird, because I’d never had a cigarette in my life. I finally got so exasperated that I was like, “hey, excuse me, why won’t you believe I’m not a smoker?” And without missing a beat, she replies in just about the flattest affect possible: “Well, you look like one.” Which, wow, thanks.
But the point is this: healthcare is incredibly vulnerable by nature, because our bodies and our humanity can sometimes feel really inextricable from each other. And when we talk about our bodies - particularly when we talk about the ways things can go really, really wrong with our bodies - that’s a really vulnerable act.
So naturally, it’s easy to worry that putting an assistive robot in a hospital or involving an algorithm in a serious treatment decision is going to make everything eerie and dehumanizing and clinical. But I can’t help but think of the mere fact that the term clinical - which comes from the Greek klinike, or bedside, as in, of or pertaining to the sick bed - has the connotation of being cold, detached, dispassionate, as in, the very same negative attributes we often apply to artificial intelligence. But don’t worry, your next doctor is probably not going to be a robot. We asked an expert.
Zachary Lipton: Yeah. That won't happen.
Lauren Prastien: That’s Zachary Chase Lipton. He’s a professor of Business Technologies and Machine Learning at Carnegie Mellon, where his work looks at the use of machine learning in healthcare. And he has a good reason for why you shouldn’t have to worry about the whole Dr. Robot thing.
Zachary Lipton: Machine learning is good at one thing, which is prediction. And prediction under a very kind of rigid assumption. When we say prediction, I think a lot of times when doctors say prediction and they mean like forecast a future like Zandar or something. Prediction doesn't necessarily mean like what will happen in the future, it means infer something unknown from something known
In medicine you're often concerned with something called a treatment effect, right? You really care. Like, if I were to give someone, not based on the distribution of historical data, if I were to intercede and give someone a different treatment than the doctors already would have given them, now are they going to do better or worse? And so the current crop of machine learning tools that we have doesn't answer that fuller like picture of actually how do we make better decisions. It gives us something like, you know, in a narrow little location we can say, is there a tumor or is there not a tumor given the image? But again, it doesn't tell us why should we give a treatment that we historically shouldn't have given. It doesn't tell us, you know, can we detect a tumor in the future? If suddenly there are changes to the equipment such as the images look a bit different from before, it doesn't tell us how you make structural changes to the healthcare system. So when people get carried away, like AI is taking over everything, it's more like we're plugging it in into these narrow places.
Lauren Prastien: And according to Adam Perer, a professor of human-computer interaction at Carnegie Mellon, the way we’re going to see artificial intelligence implemented in healthcare is going to look a lot like the human-in-the-loop systems that we discussed last week:
Adam Perer: Essentially it's a way to kind of help boost their cognitive abilities by giving them one other piece of information to act upon. But essentially we're nowhere near being able to replace them. We can just maybe give them a little extra, some information that maybe retrospective data suggests this, maybe you want to think about this, give some guidance towards that. But ultimately they need to make the decisions themselves.
Lauren Prastien: Right now, Professors Lipton and Perer are working to improve the way that clinicians interact with and even learn from the AI that is supplementing their work.
Zachary Lipton: So, this came out of conversations we've been having over the last year with, Dr. Rita Zuley who's over at Magee-Women's Hospital. Fortunately in UPMC, a lot of the patients are...they're a dominant provider in the area. So they have 40 hospitals. A lot of people are on the health plan. So all of their health is within the plan. And that's actually great from a research standpoint because it means that if they were screened in UPMC, then if something happened, they were probably treated in UPMC. The outcome was probably tracked and made it into the health record. And so they'll find out the doctor like, oh, this case that you reviewed a year ago, you called it negative, but it turned out that within a year they came in with a cancer and then they can go back and say, what did they get wrong? But we were interested in thinking, how can you use the tools of modern computer vision to not just do the main thing, which is to say, try to imitate what a radiologist does, but to help a radiologist in this context of like review and continue learning. So among other things, we imagine is the possibility of using computer vision as a way of like surfacing cases that would be interesting for review.
Lauren Prastien: An important piece of background: today, image recognition and other deep learning strategies have achieved really high accuracy in tumor diagnosis. As in, sometimes higher accuracy than actual doctors. And along with that, there is mounting anxiety over whether or not that means that doctors are just going to be replaced by these algorithms.
But remember last week, when our guests from the AFL-CIO talked to us about the areas that are more likely to see increased human-computer collaboration rather than displacement. Healthcare came up almost immediately. In the words of Craig Becker, General Counsel to the AFL-CIO, you need that human connection. I can’t imagine anything more, well, clinical, than having a computer program tell me that there’s an 87% chance I have breast cancer. It goes back to what we talked about in our very first episode this season: as these technologies continue to evolve, they are going to reinforce the importance of things like empathy, emotional intelligence and collaboration. And in that spirit, Professor Lipton is more interested in focusing on algorithms that help human clinicians become better doctors, rather than just trying to outdo them or replace them.
Zachary Lipton: How do we ultimately, to the extent that we can build a computer algorithm that sees something that a doctor might not, how do we not just sort of say, okay, we did better in this class images, but actually cycle that knowledge back? How do we help a human to sort of see perceptually what it is or what is the group of images for, you know, how we make it to that, as a function of some kind of processed by the human engaging with a model they're able to better recognize whatever is, you know, those, that subset of images for which the algorithm outperforms them.
Lauren Prastien: But this isn’t as simple as a doctor looking down at what the algorithm did and going,
Eugene Leventhal: “Oh, that is bad. I will do better next time.”
Lauren Prastien: Most doctors aren’t trained in being able to read and write code, and remember: a lot of what goes on with an algorithm mostly happens in a black box.
Adam Perer: So the really interesting challenge from my perspective is how do we explain what this algorithm is doing to the doctors so they can actually get potentially better at detecting cancer by understanding what the algorithm found that they couldn't find.
Lauren Prastien: An important step in this process is going to be making these results interpretable to the clinicians who will be learning from them, which is where Professor Perer’s expertise in creating visual interactive systems to help users make sense out of big data is going to come in handy as this project progresses.
Even in the most general sense: being able to interpret the results of healthcare algorithms, as well as understanding the larger cultural context that these algorithms may not be aware of, is really, really vital to ensuring that these algorithms are helpful and not harmful.
Look at what happened this past fall, when a major study published in the journal Science found that a popular commercial algorithm used in the American healthcare system was biased against black patients. Essentially, when presented with a white patient and a black patient, the algorithm would assign a lower risk score to the black patient than to the equally sick white patient, and, by extension, was then much more likely to refer the white patient for further treatment. As a result, only 17.7% of patients referred to additional care were black, which is super troubling when, once the researchers located this bias and attempted to improve the algorithm to eliminate it, that proportion shot up to 46.5%.
So where did this go so wrong? Essentially, the algorithm was basing the risk scores it assigned to patients on their total annual healthcare costs. On the surface, this makes a lot of sense: if have higher healthcare costs, you probably have greater healthcare needs. And in the data that the algorithm was trained on, the average black patient had roughly the same overall healthcare costs as the average white patient. But here’s the issue: even though black and white patients spent roughly the same amount per year in healthcare costs, when you compared a black patient and a white patient with the same condition, the black patient would spend $1,800 less in annual medical costs. So, the algorithm would see that and incorrectly assume that oh, black patients spend less on healthcare, so they’re actually healthier than white patients. But when researchers dug a little bit into the data that algorithm was trained on, they found that, actually, the average black patient was a lot more likely to have serious conditions like high blood pressure, anemia and diabetes. They just were a lot less likely to have received treatment - ergo, lower healthcare costs.
Zachary Lipton: If you reduce everything about your model's performance to a single number, you lose a lot of information. And if you start drilling down and say, okay, well how well is this model performing, for men, for women, for, for white people, for black people?
Lauren Prastien: In a 2018 article in Quartz, the journalist Dave Gershgorn considered: “If AI is going to be the world’s doctor, it needs better textbooks.” In other words, most healthcare data is super male and super white. But according to Professor Perer, there are ways to overcome this discrepancy, and to borrow a little bit of healthcare jargon, it involves seeking a second opinion.
Adam Perer: One way we tried to address this in some of the systems that I build is if so for deploying a system that can predict the risk of a certain patient population, if you're putting in a new patient and want to see what their risk score is going to be, you can kind of give some feedback about how similar this patient is to what the model has been trained on. And I think giving that feedback back to them also give some ability for the end user, the doctor, to trust this risk or not because they can kind of see exactly how close is this patient, has there never been a patient like this before? And therefore, whatever the model is, gonna output just doesn't make any sense. Or is there, do we have lots and lots of patients similar to this one. So similar demographics and their age, similar history of treatments and so on. And then you kind of give it a little bit more guidance for them.
Lauren Prastien: But remember, this data is trained on decisions made by humans. The data that powered that risk assessment algorithm didn’t just appear out of nowhere. And those human decisions were - and are - often plagued by the same prejudices that the algorithm itself was exhibiting. A 2015 study in JAMA Pediatrics showed that black children were less likely than white children to be administered pain medication in the emergency room while being treated for appendicitis. The next year, a study in the Proceedings of the National Academies of Sciences that found that in a survey of 222 white medical students and residents, roughly half of respondents believed the proven falsehood that black people naturally felt less pain than white people. And in 2019, the American Journal of Emergency Medicine published a review of studies from 1990 to 2018 comparing the role of race and ethnicity in a patient’s likelihood to receive medication for acute pain in emergency departments, which showed that across the board, emergency room clinicians were less likely to give painkillers to nonwhite patients than they were to white patients.
Like Professor Lipton said at the beginning of our interview, machine learning can’t tell us how to make structural changes to the healthcare system. AI isn’t going to take bias out of our society. We have to do that ourselves.
And like we’ve been saying, a lot of this technology is going to make us have to evaluate what our biases are, what our values are and what our standards are. We’ll talk a little more about what that looks like in just a moment.
In that article for Quartz I mentioned earlier, Dave Gershgorn posits a really interesting dilemma, which Eugene is going to read for us now:
Eugene Leventhal: Imagine there was a simple test to see whether you were developing Alzheimer’s disease. You would look at a picture and describe it, software would assess the way you spoke, and based on your answer, tell you whether or not you had early-stage Alzheimer’s. It would be quick, easy, and over 90% accurate—except for , it doesn’t work.
That might be because you’re from Africa. Or because you’re from India, or China, or Michigan. Imagine most of the world is getting healthier because of some new technology, but you’re getting left behind.
Lauren Prastien: Yeah, it’s just a classic trolley problem. But it’s really easy to take purely quantitative approach to the trolley problem until it’s you or someone you love who’s sitting on the tracks. And the trolley problem isn’t the only kind of complicated ethical question that the use of tech in healthcare, be it algorithms, robotics, telemedicine, you name it, is going to bring up. Some of them are going to be pretty high stakes. And for some of them, the stakes will be much lower. And like we learned in our episode on fairness, these questions usually don’t have cut-and-dry correct answers. The answers usually have more to do with our values and standards as a society.
So, we brought in an ethicist, who is fortunately much more prepared to take on these questions than we are.
David Danks: And so I think it’s such an exciting and powerful area because healthcare touches every one of us directly in the form of our own health and in the form of the health of our loved ones. But also indirectly because it is such a major sector of our economy and our lives.
Lauren Prastien: That’s David Danks, the Chief Ethicist here at the Block Center. As you may remember from our episode on fairness earlier in the season, he’s a professor of philosophy and psychology here at Carnegie Mellon, where his work looks at the ethical and policy implications of autonomous systems and machine learning.
David Danks: Well, I think what we have come to really see is the ways in which health care technologies, especially healthcare, AI in healthcare, robotics, they're um, by their nature, largely tools. They are tools that can assist a doctor and they can assist a doctor by augmenting their performance or freeing up their time for other tasks such as spending more time with their patients or it can make them even more efficient and help them to optimize the length of time they spend with a patient such that they can see twice as many people in a day. And I think one of the things we have to recognize as the ways in which, as individuals, this technology is going to start to mediate many of our interactions with doctors. And it can mediate for the better, it can mediate for the worse.
Lauren Prastien: Like Professor Danks mentioned when he joined us back in episode 4, a lot of the decisions being made about emerging technologies right now pertain to the ethical trade-offs inherent to how they’re implemented and regulated. And that’s absolutely the case in healthcare.
David Danks: So let me give a concrete example. I mean I already mentioned it might make it so a doctor could see twice as many people rather than spending twice as much time with each patient and we might have this immediate reaction. I think most people have the immediate reaction that it's of course awful that a doctor has to see twice as many people, except if we think about the ways in which certain communities and certain groups are really underserved from a medical care point of view, maybe the thing that we should be doing as a group is actually trying to have doctors see more people, that there's a trade off to be made here. Do we have deeper interactions with a select few, those who already have access to healthcare, or do we want to broaden the pool of people who have access to the incredibly high quality healthcare that we have in certain parts of the United States and other parts of the industrialized world?
Lauren Prastien: Broadening access could take on a lot of different forms. As an example, like we said in our episode on staying connected, more than 7,000 regions in the United States have a shortage of healthcare professionals, and 60% of these are in rural areas. So, there is a side to this that could benefit a lot of people, if, say, those doctors had time to take on patients via telemedicine. But like Professor Danks said, we are going to have to decide: is this what we want the future of healthcare to look like? And does it align with our values as a society?
To answer questions like these, Professor Danks emphasizes the importance of taking on a more holistic approach.
David Danks: And the only way to really tackle the challenge of what kinds of healthcare technologies we should and do want is to adopt this kind of a multidisciplinary perspective that requires deep engagement with the technology because you have to understand the ways in which the doctor's time is being freed up or their performance can be augmented. You have to understand the policy and the regulations around healthcare. What is it permissible for technology to do? What is, what do we have to know about a technology before a doctor is going to be allowed to use it? You have to understand sociology because you have to understand the ways in which people interact with one another in societies. You have to understand economics because of course that's going to be a major driver of a lot of the deployment of these technologies. And you have to understand ethics. What are the things that we value and how do we realize those values through our actions, whether individually or as a community?
Lauren Prastien: If you’re sitting here asking, who actually knows all of that? Well, his name is David Danks, there is only one of him, and we keep him pretty busy. But in all seriousness: this is why convening conversations between academics, technologists, policymakers and constituents is so crucial. All of these perspectives have something essential to offer, and not having them represented has really serious consequences, from widening the gaps in who benefits from these technologies to actively physically harming people.
But on an individual level, Professor Danks says just a basic understanding of what technology is actually out there and what that technology is actually capable of doing is a pretty vital place to start.
David Danks: Well, I think one of the first educational elements is having an understanding of what the technology is, but perhaps more importantly what it isn't.
Lauren Prastien: Because, hey, you can’t really regulate what you don’t understand. Or, at least, you really shouldn’t. And beyond that, knowing what these technologies are capable of will help to guide us in where their implementation will be most useful and where it really won’t.
David Danks: AI and robotic systems are incredibly good at handling relatively speaking, narrow tasks and in particular tasks where we have a clear idea of success. So if I'm trying to diagnose somebody's illness, there's a clear understanding of what it means to be successful with that. I get the diagnosis right. I actually figure out what is wrong with this individual at this point in time. But if we think about what it means to have a successful relationship with your doctor, that is much less clear what counts as success. It's something along the lines of the doctor has my best healthcare interests at heart, or maybe my doctor understands what matters to me and is able to help me make healthcare decisions that support what matters to me. That if I'm a world-class violinist that maybe I shouldn't take a medication that causes hand tremors. Even if that is in some sense the medically right thing to do, maybe we should look for alternative treatments. And I think those are exactly the kinds of nuanced context-sensitive, value-laden discussions and decisions where AI currently struggles quite a bit.
Lauren Prastien: And so how does this maybe guide our understanding of how to approach other sectors that are seeing the integration of AI?
David Danks: So I think one of the things that people need to understand is that when they enter into whether it's healthcare service industry, transportation, that there are certain things that we humans do that we really don't know how to automate away yet. And so what we should be arguing for lobbying, for, seeking to bring about through our economic power. Consumers are changes to people's jobs that prioritize those things that really only a human can and should be doing right now and allowing technology to be what technology is very, very good at. Uh, if I have to add a bunch of numbers together, I'd much rather have a computer do it then than me. Um, I think by and large automatic transmissions have been a good thing for transportation, um, rather than having to use a stick shift all the time. But that's because we're letting the machine, the computer do what it's good at and reserving things like a decision about where to drive for us humans.
Lauren Prastien: If we take an informed approach and remain mindful of some of the risks we’ve discussed here, incorporating artificial intelligence into healthcare has the potential to streamline practices, fill staffing gaps, help doctors improve their diagnostic practices and, perhaps most importantly, save lives. But, by the way, without the ability to access the data necessary to power these algorithms, we might not see much of this happening.
Stay with us.
Like we said earlier, the data powering healthcare algorithms is often not all that diverse. And if you’re sitting here wondering, well, wait a second, why can’t the people making these algorithms just get more diverse datasets? So that way, we don’t have a model for spotting cancerous moles that can’t recognize moles on black skin. To explain, here’s Professor Perer:
Adam Perer: When this data was originally designed to be stored somewhere, it wasn't actually designed to be later leveraged, you know, decades later for machine learning. So they're really just data dumps of stuff that's stored there. Maybe they thought, okay, we have to keep it due to regulation, but we never really forced you to what the use cases would be. And now when you're trying to get information out of there, there is really, really hard limits. You know, we've worked with healthcare institutions where, you know, the doctors, the clinical research we're working with really want us to share their data. They have a few hundred patients, maybe even something small like that. They want us to give us their data so we can help them analyze it. But again, it takes months and months and months of technologies figuring out the right query as they get out of their systems into it. And so really, you know, I'm hopeful that that new systems in place will help speed up that process, but right now it is very, very, very slow.
Lauren Prastien: So why do machine learning algorithms need all this data in the first place? Well, it’s because machine learning doesn’t look like human learning. We talked to Tom Mitchell, who you may remember from our last episode is a professor of machine learning at Carnegie Mellon and the Lead Technologist here at the Block Center. He attributed this discrepancy to something called Polanyi’s Paradox. Essentially: we know more than we can tell. Like in the words of Freud, tell me about your mother.
Tom Mitchell: You can recognize your mother, but you cannot write down a recipe and give it to me so that I can recognize your mother. You can tie your shoes. But many people cannot reference, cannot describe how to tie shoes despite the fact they can do it. So there's a lot of things that we can do instinctively, but we don't have sort of conscious, deliberate access to the procedure that we are using. And, the consequence, the importance of this is that if we're building AI systems to help us make decisions, then there are many decisions that we don't know how, that we can make, but we don't know how to describe like recognizing our mother to give a trivial example. Now within the implication of that is well, either we'll have AI systems that just won't be able to make those kinds of decisions because we don't know how to tell the computer how we do it. Or we use machine learning, which is in fact a big trend these days where instead of telling the system the recipe for how to make the decision, we train it, we show it examples. And in fact you can show examples of photographs that do include your mother and do not include your mother to a computer. And it's a very effective way to train a system to recognize faces.
Lauren Prastien: So, in the case of that cancer detection algorithm, you’d be able to show that system pictures of people with darker skin tones with cancerous moles, pictures of people with darker skin tones with moles that aren’t cancerous, pictures of people with darker skin tones with no moles at all, you get the idea, until the algorithm is able to identify the presence of a cancerous mole on darker skin tones with the same level of competence that it can on lighter skin tones. But again, a lot of that data wasn’t designed to be utilized this way when it was first gathered.
Tom Mitchell: For the last 15, 20 years in the U.S. we've been building up a larger and larger collection of medical records online. That medical data is scattered around the country in different hospitals. It's not being shared partly because of well-founded privacy reasons, partly because of profit motives of the database companies that sell the software that holds those medical records. But there is a perfect example of how we as a society together with our policy makers could change the course in a way that, I think at no big cost could significantly improve our healthcare.
Lauren Prastien: So what would that look like?
Tom Mitchell: We should be working toward a national data resource for medical care. And the weird thing is we almost had it because all of us have electronic medical records in our local hospitals. What we don't have is the national data resource. Instead, we have a diverse set of incompatible, uh, data sets in different hospitals. They're diverse partly b ecause there are several vendors of software that store those medical records and their profit motive involves keeping proprietary their data formats. They have no incentive to share the data with their competitors. And so, step number one is we need, uh, some policy making and regulation making at the national level that says, number one, let's use a consistent data format to represent medical records. Number two, let's share it in a privacy preserving way so that at the national scale we can take advantage of the very important subtle statistical trends that are in that data that we can't see today.
Lauren Prastien: If we’re able to nationalize data storage like this, we could ensure that there are protections in place to keep that data secure and anonymized. And more than that, we can start to do some pretty cool stuff with this data:
Tom Mitchell: Imagine if we could instead have your cell phone ring tomorrow morning, ifit turns out that today I show up in an emergency room with an infectious disease and your phone calls you in the morning and says, somebody you were in close proximity with yesterday has this disease, here are the symptoms to watch out for. If you experience any of those, call your doctor, that simple alert and warning would dampen the spread of these infectious diseases significantly.
What would it take to do that? All it would take would be for your phone carrier and, and other retailers who have geolocation data about you to share the trace of where you have been with a third party who also has access to the emergency room data. There are obviously privacy issues here, although the data is already being captured, sharing it is a new privacy issue, but with the right kind of infrastructure with a trusted third party being the only group that has access to that combined data and for them to then that third party could provide the service and we would get the benefit. Again, at very low cost. The interesting thing about leveraging data that's already online is often it's not a big government expense. It's just a matter of organizing ourselves in a way that we haven't historically thought about doing.
Lauren Prastien: So, Eugene, what have we learned today and how is this going to apply to other sectors moving forward?
Eugene Leventhal: Despite how some headlines can make it seem as though you’ll be getting your next flu shot from a robot, that’s not something you have to worry about just yet. Given that machine learning is good at making predictions in pretty narrow areas, it’s more important to focus on how doctors could use such algorithms to help improve patient outcomes.
We heard from Professor David Danks about the importance of having a baseline education to be able to better regulate. There are so many complex factors at play that it becomes very challenging to have a single, clear-cut regulation that could solve all of a policymaker’s problems and concerns. The reality is that there needs to be a constant cycle of education on new technologies, working with technologists and those impacted by the use of the technology, and carefully assessing where tech can be most helpful without harming individuals.
Lauren Prastien: For our tenth and final episode of the first season of Consequential, we’ll be doing a policy recap based on all of the discussions we’ve had this season.
But until then, I’m Lauren Prastien,
Eugene Leventhal: and I’m Eugene Leventhal,
Lauren Prastien: and this was Consequential. We’ll see you later this week for episode 10.
Consequential was recorded at the Block Center for Technology and Society at Carnegie Mellon University. The Block Center was established to examine the societal consequences of technological change and create meaningful plans of action. To learn more about Consequential, the Block Center and our faculty, you can check out our website at cmu.edu/block-center or follow us on Twitter @CMUBlockCenter. You can also email us at consequential@cmu.edu.
This episode of Consequential was written by Lauren Prastien and was produced by Eugene Leventhal with support from our intern, Ivan Plazacic. Our executive producers are Shryansh Mehta and Jon Nehlsen.
This episode references the 2019 research article “Dissecting racial bias in an algorithm used to manage to health of populations” by Obermeyer et al. in Science, Dave Gershgorn’s 2018 article in Quartz “If AI is going to be the world’s doctor, it needs better textbooks,” the 2015 study “Racial Disparities in Pain Management of Children With Appendicitis in Emergency Departments” by Goyal et al. in JAMA Pediatrics, the 2016 study “Racial bias in pain assessment and treatment recommendations, and false beliefs about biological differences between blacks and whites” by Hoffman et al. in Proceedings of the National Academies of Sciences, and the 2019 literature review “Racial and ethnic disparities in the management of acute pain in US emergency departments: Meta-analysis and systematic review,” by Lee et al. in the American Journal of Medicine.
S1 E10: A Policy Roadmap
Eugene Leventhal: Right now, a lot of the media coverage on healthcare technology falls into two categories: feel-good success story or absolute nightmare.
If you’re wondering why this sounds so familiar and why it’s my voice you’re hearing,
Well folks, I’ve finally acted on my plan to overthrow Lauren as the host so we’re doing things differently today.
Lauren Prastien: Really?
Eugene Leventhal: Well, maybe not that differently. But we are taking a step back from the various deep dives that we’ve been taking over the past nine weeks in order to better understand the specific policy suggestions that came up throughout our first season.
Lauren Prastien: Imagine you receive the following phone call:
Eugene Leventhal: Hello! We’re calling to let you know that you’ve been selected to come up with solutions for the United States in the context of automation, jobs, training, education, and technology as a whole. Oh, and that’s in addition to making sure that everyone has access to basic health and safety services, that our economy runs, (START FADE OUT) that no fraudulent activity takes place, that we have good relations with as many of the countries around the world as possible, that…
Lauren Prastien: And while, okay, policymakers don’t get to where they are because they got one random phone call, it is true that the multitude of issues that they’re currently dealing with are both really wide in scope and also really deeply interconnected. So where can policy begin to tackle some of the concepts and challenges that we’ve been talking about for the past nine weeks or so?
This is Consequential: what’s significant, what’s coming, and what we can do about it. I’m Lauren Prastien and though I’ve been your main tour guide along the journey of season one, I’m leaving you in the very capable hands of your other host.
Eugene Leventhal: Eugene Leventhal, that’s me! On this episode, I will walk you through the relevant policy takeaways from this season. But before we do, Lauren, can you remind us of how we got to this point?
Lauren Prastien: So over the past nine weeks, we’ve talked about the human side of technological change. We started on the question that seems to be driving a lot of the dialogue on artificial intelligence, enhanced automation and the future of work: are robots going to disrupt everything and essentially render humanity irrelevant? And the answer was, well, no.
There are some things that technology will never be able to replicate, and if anything, these technologies are going to make the things that make us innately human all the more important. But that doesn’t mean we shouldn’t do what we can to protect the people that these technologies might displace. From there, we did a deep-dive into the algorithms that have become more pervasive in our everyday lives. We looked at some of the issues surrounding the rising prevalence of these algorithms, from our rights as the individuals providing the data to power these algorithms to greater issues of bias, privacy, fairness and interpretability.
From there, we looked at how these technologies are going to impact both the ways we learn and the ways we work, from making education more accessible to changing both our workforce and our workplace. We talked about some of the impediments to ensuring that everyone benefits from this technology, from access to reliable internet to access to reskilling opportunities, and some of the policy interventions in the works right now to try to close those divides, like promoting broadband access in underserved areas and the implementation of wage insurance programs.
And last week, we saw how all these issues converged in one sector in particular - healthcare - and how decisions made about that sector might have larger implications for the ways we regulate the infiltration of emerging technologies into other sectors. All told, we learned a lot, and when it comes to synthesizing the information we covered and think of how policymakers can begin to tackle some of these issues, it can be hard to figure out where to start.
Fortunately, Eugene has made us a policy roadmap. So stay with us.
Eugene Leventhal: We are going to break down this policy episode into three parts:
Part one: The human factor. Yes, this is a podcast about technology and policy. But we can’t look at either without first talking about people.
Part two: Education and regulation. This will relate to some foundational policies to make sure stakeholders’ rights are protected, as well as keeping individuals informed about the technologies that impact their everyday lives.
Part three: New possibilities, which covers some new ideas that would enable more collaborative efforts on the part of companies, policymakers and their constituents to ensure that technologies are effectively serving the individuals they’re intended for.
Stay with us as we’ll be exploring where policymakers can start with policy in relation to AI and emerging technologies in general.
Eugene Leventhal: When we began this season, we covered the ways in which we’ve seen technological disruption play out over the last century and the generally changing nature of intelligence. The reason we began here was to set the stage for the very personal elements that are inevitably part of this greater narrative of how AI will impact humanity and what we can do about it. Because like we said, as these technologies are rolled out, they’re going to make the things that make us innately human all the more important.
More than that, any technological innovation or legislation about technology needs to prioritize the interests of humanity. We turn to Professor Molly Wright Steenson for a reminder as to why.
Molly Wright Steenson: The way that decisions have been made by AI researchers or technologists who work on AI related technologies - it's decisions that they make about the design of a thing or a product or a service or something else. Those design decisions are felt by humans.
Eugene Leventhal: Because humans feel the impacts of those design decisions, those choices have stakes. And like Professor David Danks told us in our episode on fairness, those decisions will inevitably involve some pretty serious tradeoffs.
David Danks: Many of the choices we're making when we develop technology and we deploy it in particular communities involve tradeoffs and those trade offs are not technological in nature. They are not necessarily political in nature, they're ethical in nature.
Eugene Leventhal: It’s important to recognize that having a thorough plan of action to prepare for the impacts of technological change starts with recognizing that this discussion is about much more than technology. And it’s not just how individuals are affected, for better and for worse, but also about how communities are feeling the impacts of these developments. Which is why community building is a crucial element here, as Karen Lightman reminded us in our episode on Staying Connected.
Karen Lightman: And so I think again, we need to have that user perspective and we need to understand and, and to do that, you need to be in the community, right. And you need to connect with the community and understand their side.
Eugene Leventhal: Increasing the engagement is only part of the challenge. Just having more interactions is much easier than building deeper levels of trust or connections. This comes back to the idea of being present in the communities impacted by these technologies and interacting with the full range of constituents.
The topic of building community goes hand in hand with the topic of diversity. Coming back to Professor Anita Wooley from the first episode,
Anita Wooley: So collective intelligence is the ability of a group to work together over a series of problems. So, and we really developed it to compliment the idea of individual intelligence, which has historically been measured as the ability of an individual to solve a wide range of problems.
Eugene Leventhal: Over this season, we’ve seen what happens when technological interventions don’t take into account certain populations - remember the Amazon hiring algorithm that favored men because its data was trained on male resumes, or the automatic soap dispensers whose sensors were well trained to detect white hands, but not so much for people of color. And we’ve seen what happens when a community impacted by a technological change isn’t kept in the loop about what’s going on, from the individuals impacted by Flint’s pipe detection algorithm to the parents of students in Boston Public Schools affected by the school start time algorithm.
The fact is this: technology is but a tool. Given that these tools are changing at an ever-increasing rate, it’s all the more important to make a more concerted effort to ensure that we are doing all we can to keep everyone in the loop so that they can make informed decisions about how those tools impact their day-to-day lives. By committing to engage with communities, showing that commitment through long-term presence and interaction, and bringing people from different backgrounds together, policymakers can set the tone for how tech policy should and will look and to make sure that it will be in the best interest of the people it impacts.
We heard this sentiment echoed in the context of workers from Craig Becker, the General Council of the AFL-CIO.
Craig Becker: If you want workers to embrace change and play a positive role in innovation, they have to have a certain degree of security. They can't fear that if they assist in innovation, it's gonna lead to their loss of jobs or the downgrading of their skills or degradation of their work.
Eugene Leventhal: Which brings us back to the idea of service design mentioned by Professor Molly Wright Steenson back in episode four.
Molly Wright Steenson: Okay, sure. Um, there's a design discipline called service design, um, which is considering the multiple stakeholders in a, in a design problem, right?...There are whole lot of different stakeholders. There are people who will feel the impact of whatever is designed or built. And then there’s a question of how do you design for that?
Eugene Leventhal: And like Professor Steenson mentioned in that episode, taking into account the very human factor of these technologies and how they’re going to be implemented can’t be something decided in the 11th hour. To take Lauren’s personal favorite quote from the first season:
Molly Wright Steenson: I think that if you want to attach an ethicist to a project or a startup, then what you’re going to be doing is it’s like, it’s like attaching a post it note to it or an attractive hat. It’s gonna fall off.
Eugene Leventhal: Or if we’re going to take the design metaphor a little further, think of keeping community in the loop as not just the foundation upon which a building could be created, it’s the entire plan for the building in the first place. Because think of it this way: a shaky foundation can be reinforced and propped up in some fashion. But deciding a massive construction project doesn’t need a project manager or a plan. No matter how good your materials - virtually guarantees that it is simply a matter of time until the structure comes crumbling down. We truly believe that not starting with a human-centered approach that focuses on community and diversity sets us up as a society for one inevitable outcome – failure. And when it comes to topics such as limiting the negative impacts of AI, failure is just not an option. Because again, these are people we’re talking about.
But keeping the people impacted by a given innovation in mind isn’t just about the design of a technology, it’s also about education and regulation.
Today, algorithms aren’t really a thing you can just opt out of. Just ask Wharton Professor Kartik Hosanagar:
Kartik Hosanager: Algorithms are all around us and sometimes we don't realize it or recognize it.
If you look at algorithms used in making treatment decisions or making loan approval decisions, recruiting decisions, these are socially significant decisions and if they have biases or they go wrong in other ways they have huge social and financial consequences as well.
Eugene Leventhal: Though algorithms are intensely pervasive in our everyday lives, many people are not aware of the extent. Which is why it’s so important for policymakers and for constituents alike to understand where algorithms are being used and for what purpose. An algorithm may have led you to this podcast, be it from pushing it to the top of a social media timeline, sending you a targeted ad or placing it in your recommendations based on other podcasts you’ve listed to. So it’s crucial for people to be able to understand where they’re interacting with algorithms, as well as how algorithms are impacting certain aspects of their lives. This is something that could potentially be explored as an open-source type of solution – imagine a Wikipedia of sorts where anyone could enter a company or application name and find out all of the ways they’re using algorithmic decision-making.
Once we have a better understanding of where algorithms are being used, we can work towards gaining a more intricate knowledge of how these systems work overall. It’s great to know that both Netflix and YouTube use algorithms. However, if one of their algorithms is keeping people binging longer while the other is driving people towards more incendiary content, or if there’s one algorithm doing the former with the unintended consequence of the latter, it would be in our best interest to both know that and to understand why this is happening in the first place.
Now, we understand that the target of everyone on Earth having a degree in Machine Learning is not a realistic one, and that’s not what we’re advocating for. You don’t need to be able to code or know exactly how algorithms are written to have an opinion on where it is and is not appropriate to be deployed. Think of this in the context of literacy: you don’t need to have read Infinite Jest to demonstrate that you know how to read.
Lauren Prastien: What a relief.
Eugene Leventhal: Not everyone needs to break down dense texts on artificial intelligence to be able to discuss technology with some degree of confidence or competence. The existence of additional complexity has never stopped us from integrating the basics of things that matter, like literature or mathematics, into curricula. Just as we have integrated skills like sending professional emails and using search engines appropriately into our education system, we can update those educational frameworks to include a basic sense of algorithmic literacy. Aka - what are algorithms, how do they gain access to your data, and how do they then use that data. So while not everyone will need to have an advanced education in computer science, it is possible for us to have a common base of understanding and shared lexicon. As Professor Hosanager mentioned in our episode on the black box:
Kartik Hosanager: But at a high level, I think we all need to, it's sort of like, you know, we used to talk about digital literacy 10, 15 years back and basic computer literacy and knowledge of the Internet. I think in today's world we need to be talking about, uh, basic data and algorithm literacy,
Eugene Leventhal: Knowing how these algorithms work is important for two reasons: first, we’ll then know how to advocate and protect the rights of individuals, and second, we’ll be able to make more informed decisions about how communities choose to implement and utilize these algorithms.
To that first point: Once individuals know when and how their data is being used, they’ll be able to make judgments about what their values are in terms of protections. From a regulatory side, that might mean thinking of new ways to conceptualize and manage the role of data subjects, as Professor Tae Wan Kim explained in our third episode:
Tae Wan Kim: Data subjects can be considered as a special kind of investors, like shareholders.
Eugene Leventhal: In episode 3, we looked at how the legal precedents for data subject rights both do and don't effectively capture our current technological and social landscape. And to be fair, this landscape is changing really quickly, which means that the individuals responsible for determining how to regulate it may, you know, need a little help. I promise, this isn’t just a shameless plug for the Block Center, here for all of your tech policy needs.
But we do want to stress the importance of both tapping academics proficient in technology, ethics, design, policy, you name it, and the value of forming partnerships between universities, companies and government. In our very resource and time constrained reality though, we have to get creative about how to get policymakers exposed to more people with the required expertise. Especially as the pace of innovation is increasing fairly sharply: It took us forty years after the first microelectromechanical automotive airbag system was patented – for federal legislation to mandate the installation of airbags in all new vehicles. We might not want to wait forty years for regulations regarding the safety of autonomous vehicle to be implemented.
Providing a pathway for people to have more explicit rights about how their data is being used and monetized is great, though it does not put limits on when companies are able to deploy new algorithms. This brings us to the idea of needing to have much more moderated and regulated expansion of algorithms, and our second point about the rights of communities impacted by these algorithms. Professor Danks tells us more,
David Danks: I think one set of ethical issues that’s really emerged in the last year or two is a growing realization that we can’t have our cake and eat it too. And so we really have to start as people who build, deploy and regulate technology to think about the trade offs that we are imposing on the communities around us and trying to really engage with those communities to figure out whether the trade offs we’re making are the right ones for them rather than paternalistically presupposing that we’re doing the right thing.
Eugene Leventhal: And part of that means putting these parties in dialogue. As Professor Jason Hong said in our episode on fairness:
Jason Hong: There’s going to be the people who are developing the systems, the people might be affected by the systems, the people who might be regulating the systems and so on. And um, you have to make sure that all of those people and all those groups actually have their incentives aligned correctly so that we can have much better kinds of outcomes.
Eugene Leventhal: Which, again, drives home why this kind of education and engagement is so important. But, we can’t forget that just focusing on STEM won’t solve the fundamental tension between wanting to create new technologies and making sure that those developing and using these new solutions have the basic knowledge they need to deal with the impacts of the tech. That’s why the educational system has to prepare its students not only for the technologies themselves, but for the ways that these technologies will change work and shift the emphasis placed on certain skill sets. Dare we say, the consequences. As Douglas Lee, President of Waynesburg University, said in our episode on education:
Douglas Lee: We have to look at ways to, to help them, um, develop those skills necessary to succeed.
Eugene Leventhal: When it comes to rethinking education, it's not only curriculums that are changing. The technology used in classrooms is another crucial question that needs to be carefully examined. Back in episode six, we heard from Professor Pedro Ferreira on some of his work relating to experiments with tech in the classroom.
Pedro Ferreira: So you can actually introduce technology into the classroom in a positive way. And also in a negative way. It depends on how you actually combine the use of the technology with what you want to teach.
Eugene Leventhal: Another perspective on why we need to change up how we’re approaching education came from Professor Oliver Hahl, relating to our current system producing many overqualified workers.
Oliver Hahl: What we're saying is there's even more people out there who are being rejected for being overqualified. So even conditional on making the job, they, they seem to be disappointed in the job if they're overqualified.
Eugene Leventhal: All said, having that deeper understanding of how algorithms function, understanding where they’re being integrated, and looking at the larger consequences of technological change will help us tackle a really big question, namely,
Zachary Lipton: What is it that we’re regulating exactly? Model, application, something different?
Eugene Leventhal: In our last episode, Professor Zachary Lipton brought up this thorny but important question. If we don’t understand how the outcomes of these algorithms are being generated, how much care and attention can be provided to dealing with potential outcomes?
In our episode on the education bubble, Professor Lee Branstetter proposed an FDA-like system for regulating the roll-out of ed tech:
Lee: And so I think part of the solution, um, is for government and government funded entities to do for Ed Tech, what the FDA does for drugs, submit it to scientific tests, rigorous scientific tests, um, on, you know, human subjects in this case students, and be able to help people figure out what works and what doesn't mean.
Eugene Leventhal: This idea speaks to one of the major tensions that policymakers face in terms of tech - how to both support innovation without sacrificing the well-beng of individuals. While focusing on an FDA-style testing approach may work well for education, it’s deployment in, say, manufacturing could help worker safety but would not do much in terms of the impact of automation overall. To find an option for protecting workers, we turn again to Professor Branstetter.
Lee: The problem we're finding is that workers go through a disruptive experience generated by technology or globalization and they spent decades honing a set of skills that the market no longer demands. So they have no problem getting another job, but the new job pays less than half what the old job paid. We don't have any way of insuring against that.
I mean, the long term income losses we're talking about are in the same order of magnitude as if somebody's house burned down. Now, any of these workers can go on the Internet and insure themselves against a house fire quickly, cheaply, and easily. They cannot insure themselves against the apple obsolescence of their skill, but it would be pretty easy and straightforward to create this kind of insurance. And I would view this as being complimentary to training.
Eugene Leventhal: To recap, this portion focused on personal rights and protections, we started by exploring the idea of data rights, specifically viewing data subjects as investors, and the fact that we need to have a measured approach to rolling out new technologies. With that as the backdrop, we explored a variety of potential policy responses from requiring safety demonstrations to algorithmic reporting to audits to creating an agency to help assess new tools before they make their way into classrooms. Finally, we covered the idea of wage insurance as a meaningful way to help displaced workers.
In our final section, we’ll talk about new possibilities. If we’re bringing everyone to the table and we’re protecting the rights of the individuals impacted by tech, what kinds of positive innovations can we develop? We’ll discuss a few in just a moment.
Eugene Leventhal: Now that we’ve focused on the importance of personal rights and protections in the digital space, we can look at a few final ideas that we came across in preparing this season.
The first two ideas come from Professor Tom Mitchell, the first of which relates to standardized data formats.
Tom: We need, uh, some policy making and regulation making at the national level that says, number one, let's use a consistent data format to represent medical records. Number two, let's share it in a privacy preserving way so that at the national scale we can take advantage of the very important subtle statistical trends that are in that data that we can't see today.
Eugene Leventhal: If we’re able to standardize these data formats and share information in a privacy-preserving way, we’ll be able to develop useful and potentially life-saving interventions while still maintaining public trust. It's important to stress, that's no easy task. But let's turn back to Professor Mitchell to hear what we could gain from doing so.
Tom: What if we combined the emergency room admissions data with the gps data from your phone. Then if you think about how we currently respond to new infectious diseases like h a n 23, whatever the next a infectious disease will be called currently, we respond by trying to uh, find cases of it and uh, figuring out what's the source and then we warn people publicly and so forth. Imagine if we could instead have a your cell phone ring, um, tomorrow morning. If it turns out that today I show up in an emergency room with this infectious disease and your phone calls you in the morning and says, somebody you were in close proximity with yesterday has this disease, here are the symptoms to watch out for.
Eugene Leventhal: While the beneficial use cases do sound exciting, with the way things are today, many people are more wary than optimistic. And reasonably so. That’s why we started where we did - with focusing on individuals, bringing together and building communities, and making sure that they are diverse and represent the entire set of stakeholders. By doing so, we can build networks of trust where people might be more willing to explore solutions like these, especially once they are provided the education and training to really make the most of these new technologies.
In order for that to happen, we need to pay more serious attention to protecting individuals data and digital rights to make sure that people don’t just understand these technologies, but that they also personally stand to benefit from them. And so we turn to our final recommendation, which we saved for last because it’s meant for more companies more than the government. Of course, policymakers can incentivize companies to support such programs and run versions themselves, but we turn to Professor Hong for the idea itself,
Jason Hong: So what we're trying to do with bias bounty is can we try to incentivize lots of people to try to find potential bugs inside of these machine learning algorithms.
Eugene Leventhal: By following a model similar to cybersecurity related bounties, companies can direct resources towards mitigating bias-related issues. So you, whoever you are, can play a more direct role in the technologies that impact your life, by keeping them in check.
Because ultimately, we all play a role in how the changing technological landscape is going to impact our lives, from the ways we interact with each other to how we’ll learn and work and get around. So Lauren, where does that leave us?
Lauren Prastien: Back when we first introduced this podcast, we did so with the very frightening forecast that in just 45 years, there’s a 50% chance that AI will outperform humans in all tasks, from driving a truck to performing surgery to writing a bestselling novel. Which on the surface sounds alarming, but let me reiterate: those odds - 50% - those are the same odds as a coin toss.
Here’s the thing about a coin toss: it relies on chance and a little bit of physics. That’s it. The future is a little more complicated than that.
Like we’ve been saying this whole season, this isn’t a matter of chance. We aren’t flipping a coin to decide whether or not the robots are going to take over.
So who chooses what the future is going to look like? The short answer: all of us. And what actions do we need to take now - as policymakers, as technologists, as data subjects - to make sure that we build the kind of future that we want to live in? The long answer: you’ve got ten episodes of content to get you started.
Eugene Leventhal: I’m Eugene Leventhal
Lauren Prastien: and I’m Lauren Prastien,
Eugene Leventhal: and this was Consequential. We want to take a moment to thank you for joining for season 1 of our journey of better understanding the impacts that technology will have on society. Thank you for listening and for sharing, and we look forward to continuing the conversation next year.
As we’re getting ready for season two next year, we’d love to know about the tech-related topics that are on your mind. Please feel free to reach out - we’re @CMUBlockCenter on Twitter and you can email us consequential@cmu.edu. If you liked what you’ve heard throughout the season, let us know what you enjoyed in a review on iTunes.
Consequential was recorded at the Block Center for Technology and Society at Carnegie Mellon University. The Block Center was established to examine the societal consequences of technological change and create meaningful plans of action. To learn more about Consequential, the Block Center and our faculty, you can check out our website at cmu.edu/block-center.
This episode of Consequential was written by Eugene Leventhal, with editorial support from Lauren Prastien. It was produced by Eugene Leventhal and our intern, Ivan Plazacic. Our executive producers are Shryansh Mehta and Jon Nehlsen.
Consequential Season 2 Trailer
Eugene Leventhal: Hello dear listeners. We hope that you’re staying safe during these unusual and trying times of social distancing and self-quarantining. From staying home to waiting in lines to get into supermarkets lacking toilet paper to worrying more about those among us with health issues, life has definitely changed of late.
Lauren Prastien: Before Carnegie Mellon went remote, we were getting ready to release our second season of Consequential. But as we set up recording studios in our closets to put the finishing touches on season two, we couldn’t help but consider what so many of our episodes now meant in light of COVID-19. And so we had an idea.
Eugene Leventhal: Over the past few weeks, we’ve conducted a ton of new interviews - all remotely, don’t worry - about the intersection of technology, society and COVID-19.
Lauren Prastien: We talked to a lot of interesting people: like a professor who is figuring out how to teach and produce theater in the age of Zoom meetings, as well as an infectious disease epidemiologist who is using data analytics to improve pandemic responses.
Eugene Leventhal: And we’ve decided to put our new interviews in conversation with existing season 2 interviews, to launch a short mini-season related to some more timely topics. This mini-season will explore three main areas: the use of large-scale public health data, remote education, and the future of work.
Lauren Prastien: We might have a few more episodes beyond that, but this is something we’re figuring out as we go along. Our first episode on public health data analytics will be out on April 8th, and from there, we’ll be releasing episodes every other week.
Eugene Leventhal: If there are any tech and coronavirus related stories you want to hear covered, feel free to email us at consequential@cmu.edu.
Lauren Prastien: And we’ll see you next week for the first episode of our mini-season of Consequential.
Eugene Leventhal: Consequential comes to you from the Block Center for Technology and Society at Carnegie Mellon University. The Block Center was established to examine the societal consequences of technological change and create meaningful plans of action. To learn more about Consequential, the Block Center and our faculty, you can check out our website at cmu.edu/block-center or follow us on Twitter @CMUBlockCenter.
The music you are hearing was produced by Fin Hagerty-Hammond.
Be well.
S2 E1: Pandemics, Public Data and Privacy
Lauren Prastien: So, I cannot stop thinking about part of our interview from last season with Tom Mitchell, our Lead Technologist here at the Block Center. You know, from our episode on healthcare?
Excerpt: Right now, a lot of the media coverage on AI and healthcare falls into two categories: feel-good robot success story and horrifying robot nightmare.
Lauren Prastien: Good times. Anyway, as I was saying, I cannot stop thinking about something Professor Mitchell said during our interview. Here, take a listen:
Tom Mitchell: If you think about how we currently respond to new infectious diseases like H8N23 -
Lauren Prastien: Or maybe COVID-19?
Tom Mitchell: Currently, we respond by trying to find cases of it and figuring out what's the source and then we warn people publicly and so forth.
Lauren Prastien: And then here’s the part we put in that episode:
Tom Mitchell: Imagine if we could instead have your cell phone ring tomorrow morning if it turns out that today I show up in an emergency room with this infectious disease and your phone calls you in the morning and says, somebody you were in close proximity with yesterday has this disease, here are the symptoms to watch out for. What would it take to do that? All it would take would be for your phone carrier and and other retailers who have geolocation data about you to share the trace of where you have been with a third party who also has access to the emergency room data.
Lauren Prastien: So, here’s the thing. It happened. All over the world.
Clip: Well, governments globally are enacting strict measures to contain the coronavirus raising questions about individual freedoms and privacy rights during a pandemic. Israel has given its security agencies the power to track the mobile data of people suspected of having the virus.
Clip: Contact tracing is one of the hottest keywords in South Korea at the moment. It tracks the history, timeline and the locations visited of a coronavirus patient.
Clip: So a signal will be sent, basically exchanged, between the smartphone and the smart wristband. If the smartphone is disconnected, then an alert will be immediately sent to the Department of Health and Police for follow up.
Lauren Prastien: According to the journalist Isobel Asher Hamilton for Business Insider, at least 11 countries around the world - including the United States, South Korea, Iran and Italy - are using people’s smartphones to try to stem the spread of COVID-19. This includes leveraging geolocation data gathered by advertisers, big tech firms and telecommunications companies, asking people to download apps that track their position, or in the case of Poland, having quarantined people send a selfie to an app to confirm that they are, indeed, quarantining.
The use of mobile data, location history and yes, even tracking devices, has opened up a contentious debate about the tension between the protection of privacy rights and the urgency of public health during a pandemic. Which is a problem. Because, ironically, for any of these data-driven strategies to work, governments need their peoples’ trust.
Scott Andes: In domains where public trust and public submission of data is essential, the very fact that governments are not trusted undermines their ability to use that data effectively.
Lauren Prastien: From the Block Center for Technology and Society at Carnegie Mellon University and the makeshift studio in my hallway closet, this is Consequential. I’m Lauren Prastien,
Eugene Leventhal: And I’m Eugene Leventhal, coming to you not so live but from my bedroom closet. In this two-part deep-dive on the use of big data in public health, we’ll be we’ll start by exploring the benefits of using all available data to tackle a massive health emergency. As part of that, we’ll get into the importance of data for public health, the individual privacy concerns that arise, and how to strike a balance between societal benefit and personal privacy. From there, our next episode will look back in history to see what we can learn from the oversight of human-subject-based testing and we’ll look to the future to see what potential issues might arise if the privacy around health data isn’t preserved. So stay with us.
According to the journalist Ellen Sheng at CNBC, the United States government has been in talks with companies like Facebook, Google, Twitter, Uber, Apple and IBM to use smartphone location data to lessen the impact of COVID-19. And per the journalist Byron Tau in the Wall Street Journal, the CDC, as well as local and state governments, have begun collaborating with the mobile advertising industry to get geolocation data.
All of this isn’t just for surveillance purposes, though that’s also happening and we’ll discuss the implications of that a little later in this episode. But big data is actually an incredibly powerful tool when it comes to two important questions: The first, how do we treat a virus like COVID-19? The second: how do we make sure the fewest number of people possible contract this virus in the first place? To get more information on how scientists go about answering these questions, we talked to Wilbert Van Panhuis, an infectious disease epidemiologist at the University of Pittsburgh. And just a heads up, this interview - like many you’ll be hearing over the course of this mini-season - was conducted remotely. So the sound quality can get a little questionable here and there.
Wilbert Van Panhuis: So, in general, why is it important? As you see now, we can only use models or study characteristics of the outbreak or the impacts of interventions if we have the data about the disease. All these questions that are needed for planning a response depend on research models that are then fed by data. If they're not fed by data, then which is possible, we are doing hypothetical scenario modeling, but we don't know what the real-world scenario looks like. And so it becomes very difficult to use that for any kind of planning or real-world impact if you don't have the real-world data.
Lauren Prastien: It’s important to note that, as reported by the journalist Eric Niiler for Wired, it was actually an AI epidemiologist named Kamran Khan who sent the first warnings of COVID-19 to public health officials in countries like the US and Canada. He was using a disease surveillance analytic program in conjunction with human analysis that used news reports in 65 languages, global airline tracking data and reports of animal disease outbreaks. In 2013, Khan was able to use this method to successfully predict the location of the Zika outbreak in South Florida. Epidemiology, as a field, is incredibly data-driven, and AI has been helping out a lot in that regard.
For instance, here at Carnegie Mellon, a team of researchers has been using machine learning to forecast which strain of the flu virus is most likely to surface during each year’s flu season. That research group, which is designated as one of the CDC’s two National Centers of Excellence for Influenza Forecasting, is led by Roni Rosenfeld, who is the head of our Department of Machine Learning. As reported in a recent article in the MIT Technology Review by the journalist Karen Hao, the team has shifted its focus to COVID-19, using machine learning to look at anonymized, aggregated data from flu-testing labs, electronic health records and purchasing patterns for anti-fever medication.
But so, okay, you might be sitting here like, right, of course. Duh. Of course you’d need as much information as possible about something to be able to effectively fight it. Be it a virus, a fire, your arch-nemesis: you can’t go into that situation without some knowledge on what you’re facing. But there are two specific characteristics about COVID-19 that necessitate ascertaining this information quickly, and those are its speed and its severity. Again, here’s Professor Van Panhuis:
Wilbert Van Panhuis: Compared to other respiratory viruses that we typically see in the winter, mostly the flu, this virus spreads faster and seems to be more severe. And that's why most governments are very concerned about what may happen here.
The typical research sets in making an hypothesis and setting up your study and doing your data collection and analysis does not really apply. Things happen very fast. And so research has to happen very fast as well.
Lauren Prastien: In this age of exponential curves and heatmaps, I’ll spare you another run-down of the data that we have. But what I will do is reinforce one very serious fact: this is the data that we have. And that’s one of the primary issues that epidemiologists like Professor Van Panhuis have to try to overcome.
Wilbert Van Panhuis: As you see now, we can only use models or study characteristics of the outbreak or the impacts of interventions if we have the data about the disease. And the modeling results and the research results are directly used by health agencies to determine for example, what will be the requirements for vaccine development? What will be the impact of testing? What will be the impact of anti-viral medications? And if we are going to like maybe find a shortage of ventilators, well, how many ventilators would we need to have in stock?
But then the big basic question that we still don't have the answer to today is how many transmissions are occurring? How much virus this transmitted into the population? Because what we're seeing is only the tip of the iceberg, you must have heard it in the news. We’ve only seen the tip of the iceberg of severe cases that are being measured or that die and that have been confirmed, but there are, you know, we don't know how many cases get infected for every case that gets hospitalized or that’s died or that has been tested.
Lauren Prastien: So how do you get that real-world data? Like I mentioned earlier, a lot of countries have taken it upon themselves to try to compel individuals to provide their data or to work with entities already collecting that data, like telecoms companies, big tech firms and advertisers. And last season, Professor Mitchell brought up the idea of combining emergency room data with GPS data.
We checked back in with Professor Mitchell - remotely, I promise - to talk a little more about this idea and the inherent privacy trade-offs that come with something like this.
Tom Mitchell: The kind of the reaction that I've gotten before this outbreak when I talked about this idea with others is generally a fear of privacy invasion. And people would say, “oh, but then you'd have a system collecting data about me.” Well, we already have those systems. They are collecting the data. I'm talking about using data that's already been collected.
Lauren Prastien: So what kind of data is it, and how is it being collected? Usually the data necessary to do something like this lives in different places and needs to be combined creatively. Here’s one example:
Tom Mitchell: Well, you might go to a store and go to the checkout counter or pay for your stuff with your credit card. You know, type on the little tablet that you approve the charge, get your goods and leave. Wouldn't you really like to know if for example, the next person in line, who paid for their stuff too, ended up in the emergency room tomorrow with an infectious disease that you should know about? Well, that would be nice, but how could we possibly know that? Well, that data's already online. For example, the credit card companies know exactly the point physical location you were at when you made that transaction. They know the time and they know the next transaction that happened and the time. And if that next transaction, was say within a few seconds of yours, then it's highly likely that you were in close physical proximity to that person. So that data is there. It's not being used for public health purposes. But if you think about it, if we were to put together that data with the emergency room admissions data in the hospitals and simply write a computer program that whenever a person was admitted to the emergency room with the diagnosis of an infectious disease, that computer program could query this credit cards source to find out whether there were any pairs of transactions that happened close to yours so that the other people could be warned.
Lauren Prastien: And with regard to this specific credit card idea, Professor Mitchell sees how the technology itself could help preserve your privacy in this process.
Tom Mitchell: I do think regulations of different types have a big role to play here. So, again, going back to the example of linking the credit card transaction data with the health with the infectious disease diagnoses. If you think about that one, we could implement a computer program that does that and no human ever has to look at that data. You could have a computer program that's sitting in the emergency room and already the diagnoses are being recorded online on a computer as they get made in the emergency room. That computer could have a program on it that queries the computer in the credit card centers to ask for this particular person, were there pairs of transactions recently in the last four days that involve this person and another person within 30 seconds also making a transaction? No human would ever have to look at that data. It would only be computers. And so I think a kind of regulation that would be wise would be a regulation that says, in fact the organization running this program guarantees that humans are not looking at that data or they had only these two people who have signed the usual kind of medical data privacy HIPAA-like forms are the only two people who get to see the data. So I think there are many applications that could be implemented purely by computer regulations that limit the number of humans who actually do look at that data. It would be a natural example of something that would make all of us feel more comfortable with the implementing these systems and would also genuinely guarantee better privacy than if we don't have it.
Lauren Prastien: But banks and credit card companies are not the only places useful data like this can come from, and not every person owns a credit card. Some of these places involve a much hairier conversation about privacy and informed consent. As you may remember from earlier on in this episode, an overwhelming amount of the data currently being used in the United States to try to fight the spread of COVID-19 comes from big tech firms and the advertisers that run ads on these firms’ associated apps.
In their 2018 article for the New York Times, the journalists Jennifer Valentino-DeVries, Natasha Singer, Michael H. Keller and Aaron Krolik found that at least 200 million mobile devices in the United States - or about half of the devices in service that year - were providing anonymized, precise location data through their mobile apps to at least 75 companies, including advertisers and even hedge funds, after users enabled their location services. This included local news apps, weather apps and yes, even game apps. Which are, you know, overwhelmingly used by children. But that’s a whole other can of worms.
But the point is: this data isn’t anything new. Geolocation data from your phone is used to do things like update live traffic patterns on platforms like Google Maps – so yes, that’s where the red lines come from, it’s all just based on which smartphones are stuck sitting in traffic - or determining peak business hours on those little bar graphs you see anytime you Google a business or a location - again, just smartphones standing in line at the supermarket. So there are some advantages to working with companies like Google, who are essentially in the business of collecting these kinds of data on us.
Tom Mitchell: I think the obvious advantage of getting these large companies like Google involved, is that they already have all this data, a great deal of data and they also have an infrastructure and the computer knowhow about how to put together systems that make good use of that data. So, they really got both the data, or a large fraction of it and the knowhow to do it. So they could move quickly on some of these things. The obvious risks are privacy-related and so for example, in the past Google has published, uh, things like frequency of different keywords that are being searched for on the search engine. And they would show that there's some correlation between people searching for aspirin and colds and coughs, that peaks in those search keywords correlate with peaks in actual infections. Probably you can do a better job if Google did get the detailed city-by-city hospital or health organization data about how many a diagnoses there were on each day. So, they could probably do an even better job helping to track the flow of the disease if they put together their data with let's say, medical organizations and then that does raise privacy issues.
Lauren Prastien: Okay so I usually bristle at the knee-jerk impulse to compare some new tech development or big piece of technology news to the show Black Mirror. But, hold on to your hats, because I’m about to compare this to Black Mirror. In the second episode of season five of Black Mirror, called “Smithereens,” a large social media company ends up having to cooperate with the police when a rideshare driver takes an employee of that company hostage. And what becomes rather apparent rather quickly is that the social media company is actually much better-prepared to handle this crisis because they have, well you know, unprecedented access to information on the driver through his data, essentially.
And so it kind of makes sense that on March 16, the White House Office of Science and Technology Policy released a call to action to the tech community and artificial intelligence experts to develop new techniques to assist in understanding and fighting this disease.
Which could be really promising. But there’s an argument - and it’s a fair one - that just because this data exists and, in the case of data from a mobile app, you consented to having it collected, that doesn’t necessarily mean that you consented to having it used this specific way. In the second half of this two-episode look on the use of large-scale public health data, we’ll dig into the implications of what informed consent means in the age of big data from the regulatory side. But right now, there’s another conversation to be had, and it centers on the idea of our personal relationship to privacy and our trust that our privacy will be preserved.
Tom Mitchell: Right now I think with the kind of poor understanding that many of us have about what data is being collected, we sometimes think of privacy as a yes or no thing. Do I want to have privacy or not, but in fact it's just degrees. It's more of a continuous thing. So for example, would you like people to have your complete medical record published in the front page of the newspaper? Maybe not. But would you instead be willing to let somebody know who was next to you yesterday that you have and infectious disease that they should be looking out for. That's a much smaller privacy impact than publishing your medical record in the newspaper, both in terms of who gets to see that and what kind of detail they get to see. And so it really is not a one-zero notion of privacy. It's really a matter of degree and the tradeoffs involved have to do with these degrees of privacy versus degree of benefit to society. That's the discussion I think we need to have.
Lauren Prastien: As originally reported by the journalist Sidney Fussell at Wired, the United States’ coronavirus relief bill allocated $500 million to the development of a public health surveillance and data collection system intended to monitor the spread of COVID-19. And this has raised a lot of red flags for civil liberties advocates and government watchdogs, who see a potential for technology like this being used to set up a surveillance infrastructure for monitoring people’s locations and social media content for purposes other than pandemic prevention.
So some countries have tried to address this anxiety. The UK’s Department of Health and Social Care, who has been working with companies like Microsoft, Amazon and Palantir to use emergency call data to dictate which regions may need ventilators, has issued a statement that once the COVID-19 outbreak is contained, they intend to close their COVID-19 datastore. Additionally, their data processing agreements with these companies include provisions to cease this kind of data processing and to either destroy or return the data to the NHS after the public health emergency situation has abated.
Without cultivating public trust in the processes necessary to obtain personal data, build on it, and perhaps most importantly share it between institutions, it’s almost impossible to get the necessary data to combat something like COVID-19. Here’s the Block Center’s Executive Director, Scott Andes.
Scott Andes: I think in a world that's becoming more volatile, both from things like climate and increasing pandemics, such as coronavirus, we need to get better at talking about the tradeoffs between privacy, technology, social benefits, by way of considering these extreme long tail events that will likely influence, you know, the better part of a generation economically and from a health perspective.
Lauren Prastien: Real quick: you may remember the term long tail from our episode on entertainment and education last season, when we discussed the head and the long tail. In marketing, the head describes prime-time content and long-tail describes niche content. And so by extension, a long tail event is a term economists use to describe extremely rare events that often have really catastrophic outcomes. So think of it this way: the head event: the seasonal flu. The long tail event: COVID-19. But anyway, back to Scott Andes.
Scott Andes: So the high level first-response is we need a better job talking about those types of extreme events. And I think the second high-level response I would have is make sure we're actually articulating the tradeoffs in a way that's accurate, right?
Lauren Prastien: Three hundred years ago - or you know, in February - Andes published an article in The Hill titled “Public trust in data could have helped China contain the coronavirus.” You should read it, it’s great! But let me give you the big overarching idea for the time being. Currently, there are a lot of interesting data-driven methods for tracking the spread of a disease. For instance, there’s a tool called “pre-syndromic surveillance” that was developed by Daniel B. Neill of New York University and Mallory Noble of the MIT Lincoln Lab, which uses machine learning to comb through de-identified emergency room and social media data to discover outbreaks that do not correspond with known illnesses. And Boston Children’s Hospital has also developed Pandemic Pulse and Flu Near You, which both use data from Twitter and Google to detect biothreats.
Scott Andes: And I think the sort of common denominators of all of these processes are both using a natural language processing, which is just computer science jargon for taking what people say and what they write in a fairly unstructured way and developing patterns amongst it and being able to understand it. And then crowdsourcing the data, which is what we kind of talked about either people submitting information themselves or just pulling from Facebook and Twitter, different themes and things that emerge and bringing those together to identify patterns.
Lauren Prastien: Quick aside: Kamran Khan’s strategy, which we discussed earlier in this episode, also uses natural language processing. But unlike some of the other natural language processing strategies we’ve discussed, Khan’s methods didn’t use social media postings because, and I quote, social media is too messy, which, you know what, yeah.
Anyway, like Scott Andes said, these methods require some form of voluntary participation. I can decide not to post on my social media account about the fact that I’m not feeling well. And I can also just shut off my location services if I don’t want to make that trade-off between privacy and convenience. Because these are all choices that we make. And if we don’t trust these companies - and perhaps more significantly, the government - to use this data appropriately and not use these digital platforms to harm us, none of this works.
Scott Andes: This is an interesting point in that when we often talk about the role of technology in society, we sort of discuss that in such a way where authoritarian states because of their ultimate power can use things like facial recognition software and other things to really hammer their citizens. And while that's true, there's another way of looking at it, which is in domains where public trust and public submission of data is essential, the very fact that governments are not trusted undermines their ability to use that data effectively. So I think this is an interesting wrinkle in this sort of technology and society conversation where the more we get into aggregating the information preferences and information provided by citizens and to make policy decisions, the more citizens are going to need to play a role and feel like they have, they've got a seat at the table.
Lauren Prastien: So how do we go about that?
Scott Andes: So I think another way of thinking about your question is regardless of which direction the technology goes, at least in sort of the public health space, do we have systems in place where powerful public, private and civic actors actually have the appropriate incentives to understand where the next sort of epidemic might come from? To understand what are the sort of the genesis of where these things are happening and what to do about them?
If we can get to a point where public data is robust, it’s reliable, it's safe, and we do a good job on the politics side of the house and the communication side of the house of explaining to citizens why we have solutions to things like a coronavirus and the spread of misinformation and all kinds of other socially harmful activities by way of technology and we can do it in a way that doesn't compromise privacy, all the better. But ultimately where and when these public-private, and I should say civic, university partnerships exist, you know, those are all case by case.
Lauren Prastien: And for Andes, part of this is also recognizing that a lot of this stuff is very context-dependent, and so we need to approach regulation with that in mind.
Scott Andes: Could you have a series of sort of everyday usage, regulatory framework and way to consider the tradeoffs in public data use for public health then what it would look like in extreme situations? The reason why I like that idea is it lays out the choices ahead of time, it provides a framework for us as a country to be specific about when and where, and then it provides particular tools legislatively and otherwise to pursue those measures. And those tools have checks to them.
Lauren Prastien: And this kind of regulation is going to be really necessary, both during and especially after this pandemic. Because if we know that these big tech companies are amassing data like this and that these analytics firms are capable of working with that data and that our government is setting up an infrastructure fo sharing that data, what is that going to mean for other areas of healthcare and, dare I say, our lives in general?
In the second part of this two-part look on public health data analytics, we’re looking at regulations and consent. Traditionally, when an academic entity or research group endeavors to make something in the interest of public health, there are regulations in place to protect patients and research subjects. But if those regulations are too loose, too strict, or even just non-existent, a lot can go wrong, both for the researchers themselves and for the people whose data is being used. Here’s a sneak peek:
Tom Mitchell: One thing we do want to do is make sure that organizations who have new access to new kinds of data that might have privacy impacts, that those organizations adopt many of the methods that are already in use in research centers and hospitals that are routinely using sensitive data.
Wilbert Van Panhuis: Even now there was no such data. If you want to compare the coronavirus to the SARS outbreak, there is no place where you could easily get the SARC data even though it's been gone for over 20 years almost.
David Jones: One of the big questions that comes up now with big data and analyses of them is what counts as research or not research. And then if it's something that's not really research, how do you then think about this question of protecting the subjects since they're not really research subjects?
Henry Kautz: I think things are very scary though when we look at the mass use of this technology to do mass screening of populations or to stigmatize a people or for job candidates, look at all your social media posting to say, “oh, we think you suffer from anxiety, uh, no job for you.”
Lauren Prastien: That and more in part two, which will be coming to you on April 22nd. Until then, this was Consequential. Be smart, stay safe, and please wash your hands.
Eugene Leventhal: Consequential was recorded at the Block Center for Technology and Society at Carnegie Mellon University. The Block Center was established to examine the societal consequences of technological change and create meaningful plans of action. To learn more about Consequential, the Block Center and our faculty, you can check out our website at cmu.edu/block-center or follow us on Twitter @CMUBlockCenter, all one word. You can also email us at consequential@cmu.edu.
This episode of Consequential was written by Lauren Prastien and was produced by Eugene Leventhal and our intern, Ivan Plazacic. Our executive producers are Shryansh Mehta, Scott Andes, and Jon Nehlsen. The music you’ve heard throughout this episode was produced by Fin Hagerty-Hammond.
This episode references the following articles: “Government Tracking How People Move Around in Coronavirus Pandemic” in the Wall Street Journal; “The US is tracking people's movements with phone data, and it's part of a massive increase in global surveillance” in Business Insider; “Facebook, Google discuss sharing smartphone data with government to fight coronavirus, but there are risks,” published on CNBC.com; “An AI Epidemiologist Sent the First Warnings of the Wuhan Virus” in Wired; “This is how the CDC is trying to forecast coronavirus’s spread” in the MIT Technology Review; “Your Apps Know Where You Were Last Night, and They’re Not Keeping It Secret” in the New York Times; “The Coronavirus Relief Bill Promotes Surveillance for Health” in Wired and “Public trust in data could have helped China contain the coronavirus” in The Hill. It used clips from news broadcasts by Al Jazeera English and Arirang News, as well as a conference held by Hong Kong’s Government Chief Information Officer Victor Lam.
S2 E2: Sorry, Your Phone Says You Have Anxiety
Lauren Prastien: In March, a location data analytics firm called Unacast released a “Social Distancing Scoreboard” that assigned grades to states based on how well their residents were observing social distancing guidelines. As was first reported by the journalist Geoffrey A. Fowler at the Washington Post, Unacast was calculating these grades based on GPS data from the gaming, shopping and utility apps that sell their data to Unacast for location-based advertising, which was a thing that users would have consented to when they agreed to let that app track their location.
And something that I have found really compelling, and perhaps a little troubling, about Unacast’s work is that as a society, we’re not sure what to call it. A lot of news outlets have been calling this project a tool or a scoreboard or even a report card.
Clip: It’s from this tech company that uses data in cell phones, you know, local data in cell phones, gave us a grade of a B! You know, we are down about 40% from average how close we are getting to each other. So, all right, that’s ok…it’s not an A. But it’ll get us through.
Lauren Prastien: Something that stuck out to me was that some outlets are using the language of scientific research to describe this project.
Clip: A new study says Pennsylvania is one of the states doing the best at social distancing during the coronavirus outbreak.
Clip: A new study shows North Carolina could be doing a lot better when it comes to social distancing.
Clip: Denver 7’s Micah Smith joins us from Washington Park with new research on how well Denver is doing at keeping social distancing.
Lauren Prastien: But is this research? This may seem like a really silly semantic argument, but bear with me here, because there’s a point to how we talk about work like this, and it has serious implications going forward after COVID-19. As it stands, this pandemic has resulted in the temporary relaxation of certain regulations around how people’s data gets shared, and that has opened up new opportunities for healthcare entities, large tech companies and governments to fight the virus. But it’s also raised some important questions about what all of this is going to look like going forward. Because if it is research, it comes with certain rights, protections and regulations. And if it isn’t, then figuring out what exactly work like this is and how we’re going to regulate it is going to be essential for not only improving public health, but for protecting people’s privacy.
Henry Kautz: I think things are very scary though when we look at the mass use of this technology to do mass screening of populations or to stigmatize a people or to, for example for job candidates, look at all your social media postings to say, Oh, we think you suffer from anxiety, uh, no job for you.
Lauren Prastien: From the Block Center for Technology and Society at Carnegie Mellon University and the makeshift studio in my hallway closet, this is Consequential. I’m Lauren Prastien,
Eugene Leventhal: And I’m Eugene Leventhal, coming to you not so live but from my bedroom closet. Welcome to the second episode of our two-part look on pandemics, public health, and privacy. Stay with us.
Lauren Prastien: I really wanted to get to the bottom of this. So Eugene and I talked to David Jones, a psychiatrist and historian at Harvard University, whose work looks at the history of medicine and medical ethics, to talk about how big data has changed the conversation around research practices and the rights of people whose data might be driving that research. Or, you know, not-research:
David Jones: One of the big questions that comes up now with big data and analyses of them is what counts as research or not research? And then if it's something that's not really research, how do you then think about this question of protecting the subjects since they're not really research subjects?
Lauren Prastien: So does something like Unacast’s scoreboard count as research, then, or is it just kind of surveillance?
David Jones: I think different people would have different definitions of research that would either rule that in or out. Are the people whose cell phone data was being used subjects in this research? There are some people who argue that research is the wrong way to think about that because the subjects weren't being asked to do anything They weren't being asked to take on any risks. They didn't have to even know that this was how this knowledge was being generated from them. And so therefore it's not research in a traditional sense. That said, intuitively people think that the precautions that we have put in place to govern research should also be put in place about that kind of knowledge production. Even if it's not really research.
Lauren Prastien: Personally, I think this may be where some of the tension in how we describe Unacast’s work might be coming from. Because it kind of looks like research: there’s data, there’s a fun interactive map, there’s distinctive findings. But like Professor Jones said, we intuitively consider research on humans to be something with subjects who do something. So, a group of people are asked to come talk to some Columbia University researchers about the trials and tribulations of city living only to notice, oh, hm, the room seems to be filling up with smoke. Or a group of children are given a set of dolls of varying races and are asked to pick the best doll. Or a bunch of Stanford students are put in a makeshift prison in the basement of the psychology building and they start gleefully torturing each other until the head researcher’s girlfriend walks in one day and is like, “what are you people doing?”
But anyway: the concept of what research is and isn’t can get pretty nebulous when it isn’t in a traditional lab environment or in the form of say, a survey or focus group. Your contributions to this kind of work are largely passive, but the risks you can encounter by your status as a participant - unwitting or not - are still very real and, like Professor Jones said, might necessitate the same precautions that traditional research using human subjects usually has.
David Jones: I think there's a lot of activity taking place in this country that looks like research. It might not be a research, but it still might justify ensuring that adequate provisions are in place.
Lauren Prastien: But before we get into that, we need to take a quick step back to look at the current landscape for how your information gets shared, and how COVID-19 has affected that, both temporarily and potentially in the long-run.
Like we said in the first half of this deep-dive, the kind of knowledge production that big data can generate is actually pretty essential to global health efforts in the case of a pandemic. In January of this year, the World Health Organization released a bulletin called “Data sharing for novel coronavirus (COVID-19)” and it literally opened on the sentence: “Rapid data sharing is the basis for public health action.”
So, there are basically two things that hamper the rapid sharing of that data, for better or for worse. The first has to do with how the law is written. Essentially, your health information is protected under HIPAA, which is the Health Insurance Portability and Accountability Act. In addition to ensuring that your information is private and secure, HIPAA also gives you some authority over how your information gets used and shared. In normal, not-pandemic times, HIPAA - and specifically, its Privacy Rule - essentially puts down the necessary roadblocks to make sure that, for instance, if someone - be it a neighbor or a reporter - saw you being rushed to the hospital, they can’t just call the hospital and ask what’s wrong with you. Overall, HIPAA’s Privacy Rule makes sure that your business stays your business. But, preserving patient privacy can really slow down a lot of the efforts necessary to keep the public informed on, say, the progression of a pandemic.
In order to speed up the transmission of data on the outbreak, on March 15, the Secretary of the U.S. Department of Health and Human Services actually waived five provisions of HIPAA’s Privacy Rule. These are the requirements to obtain a patient's agreement to speak with family members or friends involved in the patient’s care, the requirement to honor a request to opt out of the facility directory, the requirement to distribute a notice of privacy practices, the patient's right to request privacy restrictions and the patient's right to request confidential communications. This was something that was already accounted for in existing legislation. Under the Project Bioshield Act of 2004 and section 1135(b)(7) of the Social Security Act, the Secretary of Health and Human Services is allowed to waive these provisions in the case that the President declares an emergency or disaster and the Secretary declares a public health emergency, rwhich is currently the case for COVID-19.
In addition to the regulatory landscape, the other factor that can slow down the transmission of patient data is a little something called interoperability, which here essentially means the ability of health information systems to seamlessly communicate and share data across organizations. And a lack of interoperability in the American healthcare system - and, really, across data systems in general - was already a pretty big issue before COVID-19. Here’s Henry Kautz, the Division Director for Information & Intelligent Systems at the National Science Foundation and a professor of computer science at the University of Rochester.
Henry Kautz: We have electronic medical records that are used essentially by all doctors and medical centers, the dirty secret is that that data tends to be written and never reused.
Lauren Prastien: Back in early December, Eugene and I were able to interview Professor Kautz while he was at Carnegie Mellon for a Workshop on Artificial Intelligence and the Future of STEM and Societies, and so keep in mind: this interview predates COVID-19. But like that interview with Professor Mitchell from last week, I literally cannot stop thinking about it.
Henry Kautz: The different practices that use different systems find it almost impossible to share data and transmit data back and forth. Now there’s supposed to be standards for this kind of transmission of data. They've not been enforced. And as a result, it's hard to try to share data. Even if two practices use the same vendor for their medical record system, if they've been configured differently, again, it's almost impossible to share data.
So it's difficult to share certain kinds of data sets even within the federal government. It's great that we have very strict regulations on health data privacy, but at the same time, the way those regulations have been interpreted has slowed progress in work in predictive health. So, I think we have to look at that whole picture.
Lauren Prastien: It’s a weird balance. The fact that a lot of really important healthcare data lives in different places does help preserve patient privacy and maintain the economic competitiveness of both the systems storing that data and institutions implementing it. But it does also really slow down responding to something like a pandemic.
Recently, there have been a lot of really huge interoperability efforts both in business and government to make sure that geolocation location and patient data can be shared quickly and easily between institutions. One of the biggest efforts is a collaboration between Apple and Google to use Bluetooth technology to help bolster contract tracing efforts. This tool would be able to exchange location information between Apple’s iOS operating system and Google’s Android operating system, which have been notoriously incompatible with each other in the past.
Likewise, the United States’ government has also been working - both before and during the COVID-19 pandemic - to try to promote greater interoperability of data within the American healthcares system. Over the last 4-5 years, there have been some efforts to overhaul the infrastructure so that data can be shared more easily. And some new standards were put in place in early March that are set to go into effect in September. While there have also been new efforts directly related to COVID-19, there are still many hurdles to clear. For instance, some healthcare institutions don’t have the capacity to be doing this kind of overhaul while all of this is going on.
So what does all of this have to do with the question of whether or not the Unacast social distancing scoreboard is research or not?
Like we said in our last episode, the United States - and many other countries - have begun collaborating some of the largest tech companies in the world on the development of an infrastructure to share everything from patient data to geolocation data. Which makes sense in the time of a pandemic, but what happens when the pandemic is over? How do we keep people safe if we don’t really know what this is or what it’s being used for?`
In a recent article in the Financial Times titled “The world after coronavirus,” the author and historian Yuval Noah Harari considered that the COVID-19 epidemic might, and I quote, mark an important watershed in the history of surveillance. Because while there’s an argument that this is all just a temporary measure - for instance, Apple and Google have said that this form of tracking and surveillance will be terminated after the COVID-19 pandemic has ended - as Harari says, “temporary measures have a nasty habit of outlasting emergencies, especially as there is always a new emergency lurking on the horizon.”
It’s a concern that’s shared by a lot of data rights advocates, technology journalists, and government watchdogs. During a recent Skype interview at the Copenhagen Film Festival, NSA whistleblower Edward Snowden also expressed serious concerns about the creation of this data-sharing infrastructure, particularly when this data is combined with AI.
Edward Snowden: What we have is a transition from government that’s looking at us from the outside in mass surveillance, they used to be looking at your phone and they wanted to know what you were clicking on. They wanted to know what you were reading, what you’re buying, this kind of associated information. But now when we get into this health context, they want to know are you ill? They want to know your physical state, they want to know what’s happening under your skin.
They already know what you’re looking at on the internet. They already know where your phone is moving. Now they know what your heart rate is, what your pulse is. What happens when they start to intermix these and apply artificial intelligence to it?
Lauren Prastien: Snowden has a point. Because if we know that these big tech companies are amassing data like say, the location data you let Uber use to pick you up at your home and drop you off at your place of work, or the accelerometer data that FitBit uses to calculate your steps, or even the tweets you write that might indicate that you have depression - and that firms like Unacast are capable of analyzing that data and that our government is setting up an infrastructure for sharing that data, what is that going to mean for other areas of healthcare and, dare I say, our lives in general?
When we talked to Professor Kautz back in December, I was actually really concerned about how big tech companies were using peoples’ data - and in particular their health data - in new ways that maybe hadn’t crossed someone’s mind when they first consented to having that data collected. Keep in mind, this was around the time that the story broke about Project Nightingale, wherein an anonymous whistleblower revealed that Ascension, the second-largest healthcare provider in the United States, had initiated a secret transfer with Google of the personal medical data of about 50 million Americans, and that this data was largely not de-identified or anonymized in any meaningful way. As first reported by the journalist Rob Copeland in the Wall Street Journal, neither the patients nor the doctors related to this data had been notified of this. However, as Copeland notes in his article, this was technically permissible, because HIPAA generally allows hospitals to share data with business partners without telling patients, as long as the information is used “only to help the covered entity carry out its health care functions.”
In addition to Google, companies like Amazon, Apple and Microsoft have also been trying to begin work with not only patient health data, but also data related to social determinants of health. Real quick, social determinants of health are things like your income, your employment status and working conditions, your race, your gender, your access to basic health services, you get the idea, that can have an impact on your health and wellbeing. So think of something like a FitBit - it’s not just recording how many steps you take and your resting heart rate, it’s also picking up GPS data that would indicate where you live and spend your time. So, yeah, at the time, I was super on edge about all of this! And Professor Kautz had some really useful considerations for how current HIPAA regulations both do and do not address this.
Henry Kautz: We need to carefully and thoughtfully modify HIPAA regulations to allow patients to consent to have their data be used in ways that we do not yet know about. Right? Because you can now consent to use your data in a particular study, right? But there could be some other study about some other condition that your data is really relevant for and yet you haven't consented to that new study. I think there are many people who are would say, you know, if I can trust, uh, the privacy protections that are going to be built in and how that data will be shared, then I'm going to be willing to allow my data to be used for, let's say, any kind of nonprofit, pure research while not allowing it to be used, for example, for marketing purposes.
Lauren Prastien: Lately, advertising has been really enthusiastic about big data, but often really tone-deaf in how it implements it. I’m thinking those Spotify ads that said things like: “Take a page from the 3,445 people who streamed the ‘Boozy Brunch’ playlist on a Wednesday this year.” and “To the person who played ‘Sorry’ 42 times on Valentine’s Day - what did you do?”. Or, like how on December 10, 2017, Netflix tweeted: “To the 53 people who've watched A Christmas Prince every day for the past 18 days: Who hurt you?” Which people really hated! And you have to imagine how absolutely upsetting this would be if it was people’s social determinant of health data being used to make ads like these. So like...
Eugene Leventhal: To the person who circled the block around the Krispy Kreme six times yesterday - just get the donut. It’s ok, we won’t judge.
Lauren Prastien: And so you could see how someone who might be willing to submit their social determinants of health data for, say, a massive study or to prevent the spread of a pandemic, might not feel so great about that same company using that same data to make some hyper-specific and uncomfortable ads.
Henry Kautz: Well I think one part is to separate the use of data for purely commercial purposes for selling new things from the use of data to improve health care. Now that becomes trick, for example, when you come to for-profit companies, hospitals and pharmaceutical companies that are doing both, right? But we clearly don't want vast amounts of healthcare data to be swept up to create the next advertising campaign for a drug. But we do want to be able to combine and integrate data to provide better treatment.
Lauren Prastien: So this is actually a really pivotal time. Because on the one hand, we have a lot to gain from the ethical implementation of AI into various aspects of healthcare.
Henry Kautz: Currently the third leading cause of death in the U.S. is medical errors, which is astounding, right? All of these, you know, range the gamut from oftentimes, you know, a piece of information is not transmitted, the wrong medication is put onto the cart and nobody notices. So I think there's great opportunity everywhere from the delivery of the treatment to the creation of the treatment plan and review for an AI system to suggest to the medical caregiver, Hey, I think there's something wrong here. Pay attention to this.
Lauren Prastien: But the collaboration and data sharing necessary to make those kinds of interventions possible does require making pretty important decisions about how that data is anonymized, shared, and most importantly, applied. Especially when data from bigger tech companies comes into play, and especially when that data isn’t specifically health data, but is still being used to talk about someone’s social determinants of health.
Henry Kautz: I think things are very scary though when we look at the mass use of this technology to do mass screening of populations or to stigmatize a people or to, for example, for job candidates, look at all your social media postings to say, “Oh, we think you suffer from anxiety, uh, no job for you.”
Lauren Prastien: Maybe you’ve noticed that an increasing number of job applications now have a spot for you to write your personal Twitter handle. And it’s no mystery that many jobs and educational institutions often check an applicant’s social media profiles if they’re public. Which can be a problem: A recent study by the social media monitoring service Reppler found that 91% of hiring managers screen their job applicants’ social media accounts and 69% have rejected an applicant because of something they saw on their social media profile. And it’s not always jobs. The comedian Ellory Smith recently tweeted that z a prospective landlord wanted, in addition to proof of her employment and income, her Instagram handle. Naturally, she didn’t follow that request any further.
So it’s one thing when a job recruiter or, uh, a landlord, I guess, looks through an account to see if you’ve posted anything recently that might be troubling, and certainly, there are already problems with practices like these. But web-scraping algorithms that have access to massive amounts of data really up the stakes for how in-depth and specific that analysis can get.
So what happens when it becomes a matter of - sorry, the mental health algorithm that scanned your social media profiles determined that you likely have an anxiety disorder, and so we don’t think that this job is for you. Or, listen, our algorithm went through your Instagram and determined, based on the messiness of your apartment in the background of your photos, that you would probably be a bad tenant. Also, its sentiment analysis of your posts thinks you’re depressed, so you might want to go see somebody. Or, okay, what if it’s your insurance provider who’s scraping and analyzing the health data collected by your smartphone. Or the person who you need to approve your loan.
And this is something that Professor Kautz is pretty worried about.
Henry Kautz: I think there's an incredible potential for abuse and that is one of the areas where we need to think about the proper kinds of regulations.
Lauren Prastien: Okay. It is easy to get really frightened about this. But it’s important to remember that we aren’t going into this with no protections at all. Here’s the Block Center’s Lead Technologist, Tom Mitchell:
Tom Mitchell: It's not like we have zero experience with these risks. We have plenty of experience with different organizations, including universities, working with very sensitive data, like medical data. We have experience with hospitals themselves working with the data. And hospitals who do work with their own data have in place regulations that require that people who have access to that data take courses offered by the NIH on how to protect the privacy of that data.
One thing we do want to do is make sure that organizations who have new access to new kinds of data that might have privacy impacts, that those organizations adopt many of the methods that are already in use in research centers and hospitals that are routinely using sensitive data.
Lauren Prastien: So, in addition to HIPAA, what protects healthcare data subjects - and human subjects in general - right now is a little something called the IRB, which stands for The Institutional Review Board. Essentially, it works like this: if I want to conduct some kind of research that involves human subjects, I have to submit a proposal to a board of at least five experts of varying backgrounds who will determine whether or not my proposed research could pose any harm to my subjects.
Henry Kautz: One aspect of people are not aware of is the extent to which anyone doing research in healthcare in universities is subject to very strict human subjects review. Right? So, it is not the Wild West in universities the way it is in companies.
When companies collaborate directly with medical centers, those medical researchers are all subject to IRBs and the work they do, even if it's funded by in collaboration with a big company that'll go through, through an IRB. I am worried though about, well what about the situation where a high tech company is not collaborating with a university, but is collaborating with an insurance company? Uh, is that subject to an IRB? If a project is entirely within a company, it's generally, unless it's an actual medical procedure, it's generally not covered. Right? So, but so I think we do need to have some system for industrial use that is like an IRB system.
Lauren Prastien: And according to Professor Kautz, part of this process is going to involve answering the question I posed at the top of this episode: is all of this stuff research or not?
David Jones: The fundamental concern with any human subjects-based research is that the subjects will somehow be harmed by their participation in this research. And you don't have to look too deeply into the history to find many reasons that would justify that concern.
Lauren Prastien: That voice you’re hearing is Professor David Jones, who we spoke to at the beginning of this episode about the Unacast social distancing scoreboard.
David Jones: So before there were IRBs, and so you're basically saying before the mid 1970s, going back really thousands of years that scientists or people we would identify as scientists have been doing experiments on other humans as well as on animals. And for that very long history, researchers had the discretion to do what they thought was right, limited only by their access to research subjects. And they had two ways to get access to research subjects. Some they would attempt to recruit through volunteer means or experimenting on themselves or on their family members or on their colleagues, or they could get access through some situation in which they, or a collaborator, had power over potential research subjects. And so these were the classic cases of researchers who did experiments on condemned criminals, on enslaved people, on prison inmates or on children at orphanages. And once that researcher had access to subjects, human or animal, through any of these mechanisms, they more or less could do whatever they thought was appropriate, uh, and would not bring on too much condemnation from their colleagues.
Lauren Prastien: A really troubling fact is that a lot of the advancements that we have in the fields of science and medicine have come from really questionable sources. The history of science is full of really daring and really strange people doing things like cutting themselves open at the dinner table one night, electrocuting themselves, exposing their poor wives to radiation, and drinking water filled with feces just to prove a point. And like Professor Jones argues, there was a lot of research - and just outright torture - being conducted on some most marginalized and vulnerable people in society. And with these kinds of advances in artificial intelligence and data analytics, we do run the risk of having another shameful age of really questionable research practices.
David Jones: In this era before IRBs, I said the conduct of research was left to the discretion of the researchers and many people who thought about this had a lot of faith that the researchers were virtuous. The kind of people who were scientists were seen as gentlemanly characters who of course would know what the right thing was and then do it. And even Henry Beecher, who is famous for publishing an expose in 1960s about research misconduct, his basic response was to say, we need to double down on this commitment. And he said, the best insurance we have, that research will be done appropriately going forward, is to trust - these are his words - the reliable safeguard provided by the presence of an intelligent, informed, conscientious, compassionate, responsible investigator. And so even in the midst of his expose, Beecher said, what we should do is simply trust the scientists.
Should we trust the virtuous IT executives to do the right thing? Uh, I'm not sure there's a lot of trust in those people these days. Should we trust the federal government to do the right thing with our data? Again, I'm not sure many people will be willing to do that.
Lauren Prastien: Heads up, I’ve actually filled out an IRB before. And the way it worked was I essentially explained what my research hoped to accomplish, how I was going to obtain informed consent from my research subjects, and what were the potential risks to the health and wellbeing of those subjects as a result of my conducting this research. But perhaps the most important part of that IRB, particularly considering the subject matter of this episode, was this: I had to explain to the board how, where and how long I was going to keep my subjects’ data, such that I would be sure that their information would be anonymized, confidential and secure. Because ultimately, what all of this hinges on - HIPAA, IRBs, getting a past a global pandemic - is trust. Trust in the companies that have our data, and trust in the institutions in place that are intended to serve us and protect us. And part of addressing this issue might also involve looking at the larger issue of the trust we do or do not have in these institutions, and where those breaches in trust have taken place.
Because if we trust that our data will be used for something resembling research and if we can trust that the kind of data that’s being collected now won’t be used to hurt us later, we can be doing a lot of really good work, including ensuring that we’re better prepared for the next pandemic. Here’s Professor Wilbert Van Panhuis, who you may recall is an infectious disease epidemiologist at the University of Pittsburgh:
Wilbert Van Panhuis: Everybody can access or lots of people can access genetic data right now. There's a system set up if you want to…if you have a sequence of a virus or cancer, you can post the sequences in a database and the whole research community can benefit from those sequences. And there is no need for people to sequence something, you know, a hundred times because if somebody has already done it, you can just use that sequence. But we don't have anything like that for epidemiology. There is no place where you can have the data from outbreaks. You can have the case data, you can have the other types of data you need. And so even now there is no such data. If you want to compare the coronavirus outbreak to the SARS outbreak, there is no place where you could easily get the SARS data, even though it's been gone for over 20 years almost.
Lauren Prastien: I want you to really think about that for a second. The virus we know as SARS, which surfaced in the early 2000s, is a coronavirus, right? SARS stands for the severe acute respiratory syndrome-related coronavirus. And while it’s important to keep in mind that SARS and the novel coronavirus we call COVID-19 are genetically similar but rather different diseases, there’s a huge benefit to having that information accessible and shareable at a time like this. And so through his work on a repository called Project Tycho and the MIDAS Coordination Center - MIDAS stands for the Models of Infectious Disease Agents Study - Professor Van Panhuis has attempted to make it easier for both people and computers to find, model and interpret infectious disease data.
Wilbert Van Panhuis: We have now almost 400 members across the U.S. but also abroad that are all working on modeling of infectious diseases. And so this was already in place before the coronavirus outbreak occurred. So, as a coordination center, for the first time, we could rapidly email and contact those 400 people and ask who is interested to work on coronavirus and monitor what the community is doing. And we found that almost half of the people were interested in coronavirus research. Currently we are running a coordination of four or five working groups, almost a hundred people each, working on different aspects of the coronavirus outbreak. And so this has been an unprecedented way to engage the scientific community as a whole in our community and to coordinate a level of collaboration here that can really be helping too rapidly find out more about the outbreak and also find out what kind of mitigation strategies may have an impact and which are unlikely to work. And so that's our community building and community coordination effort, the results have been really important. And so that puts us in a very nice position where we have both the data repositories, the data science and informatics component and the community that we can serve with those innovations.
Lauren Prastien: We can’t go back to the early aughts to create something like this for SARS. And given the scramble to effectively deal with COVID-19 in a timely manner, we’re realistically going to see solutions come to market very quickly because we’re facing a major crisis.
So I want to close us out today by saying this: it can be really easy to sensationalize and panic. And you are allowed - and dare I say, entitled - to feel concerned that right now, we’re opening certain taps that will be very difficult to close again later. It is hard to say with complete certainty what our lives are going to look like after this, but I want you to consider this: technology in and of itself is not good or bad. It really depends on how the technology is implemented and regulated. Right now is going to be a really serious time for thinking about certain really crucial decisions, but it’s not the only time we’ll get to make these decisions. Here’s the Block Center’s Executive Director, Scott Andes:
Scott Andes: Oftentimes we fall into sort of the slippery slope sort of paradigm with these types of conversations about, you know, well, what's next? And I think there's a place for those conversations. But I also think that we should recognize that that's part of what the legislative and public sector processes are meant to address. We never get one shot on goal. Right. You, you don't get to say- it is never the case that we say we are going to, you know, lax a certain regulation, create a certain regulation, do you know, pursue certain things around private sector data use and you never get another chance to have a conversation about it.
Eugene Leventhal: In two weeks - so that’s April 6th - we’ll be shifting gears to the state of higher ed in the age of COVID-19. Until then, this was Consequential. Be smart, stay safe, and please wash your hands.
Lauren Prastien: Consequential was recorded at the Block Center for Technology and Society at Carnegie Mellon University. The Block Center was established to examine the societal consequences of technological change and create meaningful plans of action. To learn more about Consequential, the Block Center and our faculty, you can check out our website at cmu.edu/block-center or follow us on Twitter @CMUBlockCenter, all one word. You can also email us at consequential@cmu.edu.
This episode of Consequential was written by Lauren Prastien and was produced by Eugene Leventhal and our intern, Ivan Plazacic. Our executive producers are Shryansh Mehta, Scott Andes, and Jon Nehlsen. The music you’ve heard throughout this episode was produced by Fin Hagerty-Hammond.
This episode references the following articles: “Smartphone data reveal which Americans are social distancing (and not)” in the Washington Post, “The world after coronavirus” published in the Financial Times, and “Google’s ‘Project Nightingale’ Gathers Personal Health Data on Millions of Americans” published in The Wall Street Journal.
It also uses clips from the Orlando Sentinel, CBS Pittsburgh, WMYA, Denver 7, and an interview with Edward Snowden and Henrik Moltke at the Copenhagen Film Festival. It references tweets from the official Netflix Twitter account (@netflix) and the account of the comedienne Ellory Smith (@ellorysmith). It also refers to a January 2020 bulletin released by the World Health Organization and a study conducted by the company Reppler in conjunction with Lab42 called “Job Screening with Social Networks: Are Employers Screening Applicants?”
S2 E3: How Will COVID-19 Change Higher Ed?
Lauren Prastien: At the beginning of March, Mika Ahuvia, a professor of Classical Judaism at the University of Washington - Seattle, found herself in the midst of one of the US’s first hot zones of the escalating coronavirus crisis, and she had to make the decision as to whether or not her course would continue to meet in person. Let me remind you: this was pretty early on in March, and the United States looked a lot different from what it looks like today.
She had a lot of concerns about this new arrangement. While she’d always been in the habit of uploading recordings of her lectures for students to later revisit, she wondered, would the move online ruin the dynamic of her class? And what if her students didn’t have access to reliable wifi? Or a quiet room? She was starting to doubt if continuing to hold the course in the face of a looming global health crisis made sense for her students, who seemed increasingly demoralized, and she was unsure if she was going to be able to give them the education that she felt she owed them as their teacher.
When I talked to Professor Ahuvia about these concerns, it was in a pretty brief conversation over text. Because in the midst of all of this, she still had to teach, she still had a draft of her book due, and classes still had to meet. Life still had to carry on for the 19.9 million students enrolled in higher education institutions in the United States.
But in the span of just two weeks, the entire American higher education system - with some exceptions - moved online. Some professors said goodbye to students in person on a Friday, not knowing that would be the last time their class would meet in person, only to see them on Zoom the following Monday. There have been some real growing pains here, but through the efforts of a lot of really dedicated, really flexible professors - some of whom we’ll speak to today - higher ed has adapted and changed in order to cope with the impact of COVID-19. Though this is often touted as a temporary measure, the truth is that higher ed may never fully go back to normal. And in some regards, we may not want it to.
From the Block Center for Technology and Society at Carnegie Mellon University and the makeshift studio in my hallway closet, this is Consequential. I’m Lauren Prastien,
Eugene Leventhal: And I’m Eugene Leventhal, coming to you from the popup studio in my bedroom closet. Today we’ll be discussing how teachers have been using technology as their classes have gone remote, as well as some of the resulting challenges. We’ll also look forward and think about what some of the lasting impacts of COVID-19 on higher education might be.
Lauren Prastien: In preparing for this episode, we had a lot of short conversations with educators around the country - all remotely - where we asked what their biggest takeaway was from this rapid move to online education. Here’s Inez Tan, who serves as an academic coordinator in creative writing at the University of California, Irvine, where she also teaches English in the School of Humanities:
Inez Tan: This whole experience has been really reinforcing for me that learning is social, it depends on community and so I think with Zoom teaching I’m finding that I actually need to do most of the talking while figuring out how I can use the chat to get input from them to feel like they’re part of the class, not just sort of passively watching a video, but that they’re participating and we’re still building some sort of class out of their being there and supplying what they’re thinking.
Lauren Prastien: So, you may recall that last season we did an episode on how technology was disrupting higher ed. Here, let me jog your memory:
Michael D. Smith: Technology is never going to change higher education, right? Cause we're the one industry on the planet who doesn't have to worry about technology coming in and disrupting our business. He says provocatively.
Lauren Prastien: Ah, yes, simpler times. So yeah, when we spoke to Professor Michael D. Smith, who you just heard, and Professor Pedro Ferreira, who you’ll hear in just a moment, last season, our conversation centered on the idea that higher education as we knew it derived a lot of its power from scarcity. So, there were only so many hours in a school day, so many seats in a classroom, you get the idea. But in the same way that technology disrupted the entertainment industry’s hold on scarcity and allowed for personalization, Professors Smith and Ferreira saw a very similar thing about to happen with education. Which, you know, now that we’ve seen the United States’ 19.9 million college students enrolled in Zoom University, is kind of playing out at a faster clip than we might have expected. Here’s Pedro Ferreira, a professor of information systems, engineering and public policy at Carnegie Mellon:
Pedro Ferreira: Well, what we have here is a large scale experiment where everyone is trying to personalize. It's like crowdsourcing the solution in zillions and zillions of people, right?
Lauren Prastien: So what do you see as some of the earlier findings of this experiment?
Pedro Ferreira: I see transition to online either through COVID-19 or anything else because of its scale, is going to allow us to understand biases much better and it can amplify existing biases. There's some people that can get access to the technology and will transition the best they can. Some people can just not make it or it might actually be, besides amplifying existing biases, creating new biases across fields, across skill levels. I can be really skilled face to face, but with a computer I have a problem and I'm not able to get the materials. Across genders, across race. The impact in particular of COVID, I mean, with kids at home might affect parents in male and female in very different ways. So from the policy making perspective, I think we should be really aware of biases that are amplified or new biases that are created and understanding which mechanisms, public policy mechanisms can help, address those gaps.
Lauren Prastien: It is important to remember that the rapid move to widespread online education was facilitated by necessity. Particularly because the spread of COVID-19 escalated during most universities’ spring breaks, and just about the worst thing that you can do when trying to contain a virus is take people who’ve been to a lot of different places and then put them together in another place and then send them back out into the world again. So moving colleges online was a pretty important step in making sure that campuses didn’t quickly become hot zones. As a result, a lot of students left campus for spring break only to never return, and some who’d stuck around were asked to vacate their campuses with less than a week’s notice.
But the ability to make those changes quickly does require a certain level of presumption. That a student can afford a plane ticket home. That it won’t impact their immigration status or student visa. That they have a stable home environment to return to. That this environment has a computer or other connected device and a reliable broadband Internet connection. Which isn’t always the case.
And that level of presumption also extends to the faculty’s ability to quickly transform their course into an online mode. Which is, by the way, not always super intuitive. Online educators spend a lot of time formulating their syllabi to suit the strengths and limitations of an online medium, and because of COVID-19, a lot of educators who’d never taught online before had to do this in, like, a weekend.
Pedro Ferreira: There's going to be significant heterogeneity. I didn't run any study, but it's hard to believe that everyone is at pace with technology. I think that if we go and look at what's happening, I think that the faculty and the students that were already using online tools are much better prepared. There's a learning curve like with any other technology and everyone that was already using these tools, everyone that was already using Zoom and Canvas productively are at a much better stage to do it at scale.
Lauren Prastien: In our conversations with faculty, we saw this significant heterogeneity in terms of which classes were already using technologies like video and learning management systems, even within the same discipline. Here’s Julian Silverman. He’s a professor of chemistry and biochemistry at Manhattan College, and he’d already been experimenting with streaming video as a supplemental medium for his teaching practice.
Julian Silverman: I do a little bit of education work in open educational resources, which is stuff that anyone can use at any point. And really it's just, there's too much stuff to pack into one course. And so the students need to do review problems and to do it once a week where no one shows, it didn't seem that useful.
Lauren Prastien: So at the beginning of this semester, Professor Silverman decided to take the review session part of his course online.
Julian Silverman: So I had been recording like hour-long problem sessions where you do practice problems for my general chemistry course and they were technically visible to anyone if you had the link.
Lauren Prastien: And because he’d already been doing this, he kind of had a leg up when it came to the move to fully digitizing his class.
Julian Silverman: But I'm really lucky. I mean some of my colleagues do stuff in class where they're recording like a piece of paper and drawing live. I have a camera set up to do a little bit of that. But this is only because I knew that they would respond well to a video early on in the semester. They have more views than there are students in the class. So I think that's a good sign.
Lauren Prastien: Something that was also kind of cool was that prior to COVID-19 and now, especially during it, Professor Silverman was also into using some more non-traditional platforms to connect with his students.
Julian Silverman: One of the things I'm going to try to use is Twitch, which is inherently open, so they might not have the recorded version, but they can at least watch live I think.
Lauren Prastien: Yes, you heard that right. Twitch. The live-streaming platform usually employed by gamers to broadcast themselves playing videogames. Which you know what, actually kind of makes sense, given that it allows you to show multiple screens at once and respond to your audience in real time.
Julian Silverman: I had promised my students that I would do this at the beginning of this semester and I haven't yet been able to meet up with them live cause it's always based on my schedule. I did have a student sit in on one live in person, but now I finally taught myself Twitch and I think that I can do it. So that will allow me to record it live and then I can put it on YouTube later.
Lauren Prastien: We spoke to Professor Silverman back in March, and today, I can confirm that he has a thriving Twitch channel for his lectures, review sessions and other teaching obligations. Which he says works really well with his course load, but would probably not transition very well to a professor who had to teach a lab course, for instance. Especially in a field like chemistry, where it would be pretty unsafe to have most of those materials just sitting around your house.
Which speaks to a larger point: for a standard lecture course, the move to online learning might be fairly intuitive. But what happens when you teach design, and your students were supposed to have a gallery show as their final project? Or you teach filmmaking, and now your students don’t have access to any of the equipment they need to actually learn something? Or, oh wait, now suddenly your students don’t all live in the same time zone, so asking for the normal seminar time means that one of your students now has to wake up at 4 in the morning?
So I asked Professor Ferreira where technology might play a role in addressing some of these problems.
Pedro Ferriera: Maybe a chemistry lab and music class is going to be harder to deliver over the Internet. And many other things that require peer-to-peer learning and peer-to-peer interaction. Probably. I'm not sure what kind of tools we're going to have to allow these ones. It's unclear to me, but what I can say is that many interesting innovations come from necessity and arise during times like this.
Lauren Prastien: It’s pretty important to remember that a lot of the tools we’re using now - Zoom, Blackboard, Canvas, Google Docs - were tools that existed well before COVID-19. So, like Professor Ferreira said, it’s going to be interesting to see what new pedagogical tools come of this.
Pedro Ferreira: I can only anticipate that new tools and new technologies will be developed pretty soon to exactly support teaching and learning in fields which is harder, such as the labs such as music, and so on and so forth, where current solutions, I think, fall short of the expectations.
Lauren Prastien: Generally, those expectations center on the idea of having a synchronous educational experience. We’ll explain that more in a second.
A lot of the instructors - and by a lot, I mean all of the instructors - that we spoke to, both on the record and not, be it in a larger lecture course or in a discussion course, expressed that one of their largest concerns was the issue of not being able to have their course happen in real time.
Eric Yttri: I’m already fairly familiar with the technology, but the real issue is I like to push the students to not just memorize facts but to really get to the concept, what in pedagogy is called analysis synthesis. And so that means a lot of in-class thought experiments, that means a lot of back and forth, even though there’s 70 people in the class.
Inez Tan: I am teaching a class this quarter kind of about poetry and fiction and approaching those as students who are poets and fiction writers themselves. And it’s a heavily class discussion-based course and I just could not imagine how I was going to run it completely online.
Brian Herrera: My main concern for my own class this semester is that, um, I want to find a, I don't know that I'm going to be able to find a way for the students to have the dynamic, spontaneous interactions with each other that my class had begun to really find a great groove with.
Lauren Prastien: That last voice you heard is Brian Herrera. He’s a professor of theater and gender and sexuality studies at Princeton University. Being involved in both higher education and non-profit theater, Professor Herrera has seen both of these industries struggle to overcome one of the largest unifying qualities between them, which is this idea of what he calls the synchronous.
Brian Herrera: So generally as a theater-maker, a theater teacher, and as an educator in contemporary education, we prize the synchronous. We prize the space of us coming together in a room together, sharing space and time. And indeed live performance and theater is basically about that, we come together in a room to share an experience that promises the potential of transformation. My feeling is that education is another space in which we use co-presence to sort of engage in encounters that might lead to transformative experiences. So there's often an impulse to preserve the synchronous.
Lauren Prastien: Except, here’s the problem with that:
Brian Herrera: The Internet, as we experience it, is really based on asynchronous realities, where a Facebook post or a Twitter tweet might go up in one moment, but somebody else will encounter it in another moment. Of course it becomes its own stream, its own flow, but it's not necessarily people in the same space and time. And so the idea of how do we sort of be very attentive to what are our cravings for the synchronous and what are the ways that asynchronous modes might actually serve us as well or better? And I have been quite concerned that so many universities, as well as so many theater companies, are saying, “let's just take it all online.” And I'm like, it doesn't really work that way.
Lauren Prastien: Fun fact, this was not the only time we heard a comparison made between the classroom and the theater in our interviews with educators. During our discussion with Professor Silverman, this little gem came up:
Julian Silverman: I don't think you're ever going to sacrifice one for the other. It's the reason we have movies and theater that both serve different purposes.
Lauren Prastien: I love that. Because it does speak to the fact that both of these modes do serve a purpose and have their own strengths and limitations. So, for his own course, Professor Herrera made a blended synchronous/asynchronous model, which used small group discussions with a somewhat non-traditional medium that we certainly appreciate: a podcast.
Brian Herrera: My plan is to have this podcast be guided in some ways by questions from my students that will inform the next week's episode. But then also I will try to build it in a way that can be content that's directly relevant to the curricular objective of the course, and therefore have direct service to my dozen students, but would also potentially be of service to other faculty members around the country or perhaps the field at large. To sort of leverage out from the classroom toward a broader community. Because indeed, part of the project of this class was really trying to figure out how the curricular work of this class might serve the theater field more broadly.
Lauren Prastien: Since our conversation, Professor Herrera has released 5 episodes of his educational podcast, which is called Stinky Lulu Says, that cover just only his class’s reading materials but also some really pertinent questions about theater in the time of COVID-19, like whether playwrights are job creators, when it would make sense to reopen theaters, and what to make of this new genre of celebrities live-streaming performances on Instagram.
But anyway, our conversation with Professor Herrera on synchronous versus asynchronous education got into a really interesting territory, and that was what actually constitutes a college experience.
Brian Herrera: There's been a real question of how to maintain the integrity of those so as not to disrupt or totally transform the quality. Not the quality in terms of standards, but just the experiential dimensions of what those research experiences are, which are considered as being fundamental to the experience of being a Princeton student. So I think we're going to see some questions about asynchrony. I've already had one student who's considering taking a leave because they feel like they're going to be missing something integral to what they wanted from their Princeton experience. So there's, I think there's different questions are going to be put in different relief as a result of this experience.
Lauren Prastien: Asynchrony versus synchrony isn’t just a pedagogical question. Because colleges aren’t just the sum total of their classes. There’s stopping into your professor’s office hours to ask a question or running into your friends on the quad or seeing a student theater production or any number of pretty organic, synchronous, in-person experiences that constitutes what is often one of the most formative periods in a person’s life.
Brian Herrera: I do think that there is, for many campuses, especially campuses that might sort of have as their primary selling point for their large-ticket tuition bill being the sort of the transformative experience you have while on campus, there may be a sense of is our value going to be diminished either monetarily or culturally or just sort of, I don't know, ontologically, ephemerally, sort of just something ineffable, are we going to lose?
Lauren Prastien: In an article in US News and World Report, the journalist Emma Kerr noted that in a petition asking New York University to provide a partial tuition refund, one student wrote, “Zoom University is not worth 50k a year.” Another said: “I didn’t pay to attend Zoom.” On Twitter, the popular hashtag #zoomuniversity, is full of tweets from students sharing amazing work that they’ve done, and also tweets about how the professor’s been on mute for the past 15 minutes and no one can get a hold of them or how they’re just really lonely.
So we asked Professor Herrera if he felt that this might endanger certain colleges or reframe what the purpose of a college education is supposed to look like.
Brian Herrera: I can't imagine it won't, let's just say that. I can't imagine it won't. I expect that where it might have the most immediate impact is not at schools like Princeton. Princeton will persist and will continue to survive whatever else happens in the industry, I would suspect, because there was a brand, there is a kind of prestige economy at work here that's a little bit different than say at a smaller liberal arts college that has a hundred years of tradition but just doesn't have the same access to donors, that same access to wealth, the same access...and so the question is, what is the special experience you get by being on site is going to be something that opens up. We're going to see some new questions.
Lauren Prastien: In a recent article in USA Today, the journalist David Jesse noted that with prospective student weekends disrupted by COVID-19, many small liberal arts colleges - many of which were already in a pretty precarious position - might see application and enrollment numbers go down. And another article in USA Today, this one by the journalist Chris Quintana, considered that with market issues impacting endowments, many students asking for refunds and the cancellation standardized testing, higher ed might be in trouble.
And you might be saying, well, okay, universities have weathered a lot. But, in a recent article in the Wall Street Journal, the journalists Melissa Korn, Douglas Belkin and Juliet Chung reported that a university that had survived the Civil War, the Great Depression and two world wars, had to shut its doors as a result of the coronavirus pandemic. As many universities are unsure as to whether they’ll be opening their doors again - both literally and figuratively - in the fall, about 200 of America’s private, liberal arts universities are at risk of closing in the next year.
So academia might have to change in order to survive.
Brian Herrera: Every institution is a conservative entity, but academia is very conservative. Its change happens very slowly. And so innovation happens within the structures of that conservatism. So the tradition of academia creates the containers in which a lot of innovation can happen. But there is this moment when...there was this collision and this collision of values and knee-jerk responses. I think what we do have the opportunity to do is to choose what we're maintaining and sustaining. We're not going to contain the whole...we're not going to be able to do the whole thing. I'm interested to see how the different tactics, in which of the tactics that we get feedback from students down the road, are the ones that they most value.
Lauren Prastien: More on that in a moment.
Eric Yttri: In our class, and in education in general, we seek a lot of things. We seek connection. We seek knowledge. We seek entertainment, amongst other things. The knowledge was still going through pretty well, but then my partner Jen had the brilliant idea of saying, you have that Batman mask, why don't you put that on and do the voice and I said, no, that is an incredible idea, but we go to Burning Man, we have a 5-year-old, and we like Halloween. We’re doing a whole different costume for every lecture from here on out. So we got all our costumes together, had two different dinosaurs and a caveman, had the Batman, Santa Claus made an appearance, did one entirely with my daughter's bunny puppet that a lot of people particularly enjoyed, I’m told. So yeah, I’m a bit worried I’ll be requested to show up in costume for every class next semester.
Lauren Prastien: So, by the way, that is Eric Yttri, he is a professor of biological sciences at Carnegie Mellon, and he is the reason why I walked in on my fiance the other day giving a virtual lecture wearing a horse head. We’ll be back in a second.
As we talk about the rapid changes that have happened to higher ed and the massive existential thread it’s posed to academia, it’s important to consider that not every student’s experience has been diminished by the move online. Here’s Michael D. Smith, a professor of Information Technology and Marketing at Carnegie Mellon, whose voice you heard earlier in this episode:
Michael D. Smith: I talked to a student who said, in class it's hard to get a seat in the front row. On Zoom, everybody's in the front row. There's an idea here that we can use the technology in a way that will help get the most engagement out of the students. I've talked to some faculty who say that students who were hesitant to participate in a physical classroom, seem more open to participating Zoom. That’s great. I think we need to continue figuring out how to use the technology in a way that helps students with different learning styles and different proclivities for participation to get their ideas out on the table.
Lauren Prastien: And according to Professor Smith, this isn’t just limited to learning style and participation preferences.
Michael D. Smith: I think the notion of being able to go asynchronous is not only going to increase the ability for our existing students to learn, I think it's also going to open the opportunity to reach out to students who aren't able to take 18 months off of their lives and move their whole world to Pittsburgh to get educated. It's going to allow us to really reach out to students that we just couldn't reach out to with our existing business.
Lauren Prastien: Even though most universities are not technically for-profit, it is important to remember that institutions of higher education still do often operate like businesses. In our current educational model, they need to be able to attract customers - or students - in order to compensate their faculty and staff so they can provide a service - or their education. But what Professor Smith and our next guest are both getting at here is that higher education has not always been able to serve every customer base. Here’s Jessie Male:
Jessie Male: Higher education is rooted in elitism in many ways and disabled people have been asking for online options for years. Like everything we're talking about now from how people are moving through the world is...these are all ideas that disabled communities have been pushing for for decades. And so now because it's affecting people from who moved through the world in a variety of ways, who identify as non-disabled, suddenly people are very interested in the possibilities. So I do hope that this changes, you know, the discourse around how one can achieve an effective learning environment and teaching environments. But I also hope a lot of acknowledgement and credit is given toward the disabled communities that have been preparing for this in a lot of ways.
Lauren Prastien: So, Professor Male is in a pretty unique position. She is a professor of disability studies and creative writing, and prior to the COVID-19 outbreak, she was teaching in person at NYU’s Gallatin School while also teaching online at the Ohio State University. Now, obviously, both of these classes are online today. But as an educator who taught both in-person and online, Professor Male saw an opportunity to help her peers that had been suddenly asked to digitize courses in just a few days.
Jessie Male: There's a huge community of educators who are using Twitter as a way to share resources. So, you know, all you need to do is hashtag Academic Twitter and things start to move. I'm sitting at my desk, I'm kind of watching a lot of this unfold on Twitter. A lot of people are so scared, teachers are panicking. And so my initial thought was, I feel that I've generated so much information that now I can utilize in shaping the class that I want other educators also to have this kind of information.
Lauren Prastien: So, earlier that day, Professor Male had met with her Disability Arts class, and they’d had a discussion about what it was going to look like to no longer meet in person.
Jessie Male: What I've learned is that at any crisis point, and basically, you know, I've taught in New York City post-Hurricane Sandy, I've taught after the 2016 election, and students really want to feel agency and that they're trusted with an understanding of their own, what's best for their own learning experience. That's really rooted in an understanding of interdependence, which comes from disability studies, this understanding that, you know, as an educator, I'm not just in front of the classroom informing them, teaching them, that interdependence means that we're all receiving different information from each other and benefiting from it and supporting each other in a variety of ways. So I knew immediately that the first thing I was going to do when I walked into my classroom was ask my students how they imagined moving forward.
Lauren Prastien: What came from this conversation was a pretty comprehensive list of resources and suggestions, from how to effectively use the discussion board section of your learning management system to archiving synchronous lessons so students can revisit them later to including as many pictures of your pets in your teaching materials as you can. So on March 9, Professor Male took to Twitter to share her students’ feedback and her own insights on the move to online education. The thread went viral, sparking a pretty big discussion among educators in a variety of fields and from different levels of experience, who shared resources and answered each others’ questions.
Jessie Male: Oftentimes as we think about resources, they're seen as very separate categories. And so it's been very exciting to watch how people in the sciences and people who identify as, you know, educators in the humanities are engaging with strategies. So that's the story of the Twitter thread.
Lauren Prastien: Professor Male’s thread, and the discussion that it initiated, helped a lot of educators think about what altering higher education in the short-term might look like, in order to accommodate the necessity of social distancing. And while I was talking to Professor Male, I was curious to find out whether or not she also felt that this might actually have a long-term impact on what higher ed looks like.
Jessie Male: Disruption is so scary because we find such comfort in the norm and in routine and what we've been taught to be appropriate and effective ways of being an educational environment. But I think that this moment to highlight all that is possible through online education is an exciting moment to say, you know, here are all my presumptions that I had about online learning, right? And let's see how they can be effective. But that in part comes from this association of online teaching like disrupting everything we know about higher ed. You know, and I encourage it, I encourage the rule breaking and now we have to break the rules out of necessity.
Lauren Prastien: Breaking the rules has taken many forms during this time. From having flexible turn-in times for papers to shifting to a more asynchronous model of teaching to the mere fact of letting students - and professors - wear sweatpants to class. Which are steps that might actually be important to making college more accessible to individuals whose lived experience may not be compatible with the traditional model but still absolutely deserve the educational experience and credentials a college education affords them.
When we talked to Professors Ferreira and Smith to prepare for this episode, we wanted to not just look at what’s happening now, but how what’s happening now might impact the state of higher ed going forward. Simply put, are we going to - and should we - go back to normal after this?
Michael D. Smith: I personally think this is a huge opportunity for us in higher education to use the technology to its fullest and do things using the technology that we couldn't do in the classroom, but that benefit our students. I worry that our instinct in higher education is going to be the same as every other industry, which is we want to go back to what we've always known and what we've always done. This is exactly what we saw in the entertainment industry when they got exposed to online technology, they tried to use it to replicate their existing processes. They were focused on, I've always sold DVDs, now I can sell that same file online for basically the same price. What they weren't thinking about was creating Netflix, a completely new business model with a new way of interacting with their consumers. I hope we in higher education focus on that, that Netflix shift that might involve a radical shift in our business model.
I really think it's going to be important for us to take a step back and ask ourselves what our mission is. Um, we talked about this a little bit on the first podcast, but I think when institutions go through times of change, they typically look at the change as to whether it threatens their existing way of doing business and not as, whether it enables their core mission. I worry that we're going to look at this as a threat to our way of doing business when we really should be looking at it as potentially an opportunity to do a better job of fulfilling our core mission, which I think is enabling people to develop their skills and gifts and then use those skills and gifts to the benefit of society.
Lauren Prastien: And Professor Ferreira raised a pretty important point about how this fits into the ultimate goal of higher ed in the first place, which is, you know, preparing its students for the future.
Pedro Ferreira: We cannot forget that in the end of the day there is a job market and we’re serving our students to be as better prepared, as best prepared as possible in the job market. That's what we answer in the end of the day, because after graduation there was a job market to attend to. But even there, I see that the mechanisms aren't aligned because the job market changed. The industry firms are all using or will be all using online in a much different way. So we need to prepare our students to use online in post COVID-19 work. And that requires a different sense of pedagogy and understanding how to prepare our students for these new worlds. So even the incentive to make things different because there is a job market, the job market is also going in the same direction. So I think goals are aligned.
Lauren Prastien: So will COVID-19 change higher ed? Undoubtedly, it already has. A lot remains to be seen for how all of this looks going forward, but like Professors Smith and Ferreira said, this could be a watershed moment for the institution of higher ed, and a lot of good could come of it.
Michael D. Smith: This is a unique moment where I hope we take a step back and really question the way we've always done things and whether that way still makes sense in our new technology world. People tend to fall back on their old habits until they're confronted with the absolute necessity of change. We're now in a world where we're facing that absolute necessity of change. Let's make the best of it and think really hard about what's the best way to educate our students using technology, not what's the best way to propagate the old classroom model using technology.
Eugene Leventhal: In two weeks - so that’s May 20th - we’ll be shifting gears to talk about COVID-19 and the future of work. Until then, this was Consequential. Be smart, stay safe, and please wash your hands.
Consequential was recorded at the Block Center for Technology and Society at Carnegie Mellon University. The Block Center was established to examine the societal consequences of technological change and create meaningful plans of action. To learn more about Consequential, the Block Center and our faculty, you can check out our website at cmu.edu/block-center or follow us on Twitter @CMUBlockCenter, all one word. You can also email us at consequential@cmu.edu.
This episode of Consequential was written by Lauren Prastien and was produced by Eugene Leventhal and our intern, Ivan Plazacic. Our executive producers are Shryansh Mehta, Scott Andes, and Jon Nehlsen. The music you’ve heard throughout this episode was produced by Fin Hagerty-Hammond.
This episode references the following articles: “Why Students Are Seeking Refunds During COVID-19” in US News and World Report, “Small colleges were already on the brink. Now, coronavirus threatens their existence” in USA Today, “US colleges scrambled to react to the coronavirus pandemic. Now their very existence is in jeopardy” in USA Today and “Coronavirus Pushes Colleges to the Breaking Point, Forcing ‘Hard Choices’ About Education” in the Wall Street Journal.
Thank you to all of our faculty interviewees from around the country, and to all of the educators who have shown strength, empathy and resilience in the face of COVID-19.
S2 E4: Death by a Thousand Emails
Lauren Prastien: So, I’ve got this friend. Let’s call him Dylan. We grew up together in a small town in New Jersey, and I’ve always kind of known that Dylan was going to do something interesting. Hopefully, something good. But, yeah, definitely something, and when we were younger, that something would usually get him in huge trouble.
Dylan: Yeah, and I think, like when I was younger, there was like this joy and glee in that where it was like “oh, yeah, this is gonna be the thing” but then when I got older, and you know, I started caring about like college and the future, I was like “oh, god, is this gonna be the thing that gets me in trouble?
Lauren Prastien: Dylan was entirely too smart for his own good, just this chaotic combination of incredibly creative and incredibly troublesome. He once rewrote all of our little biographies in the playbill for our school play so that they read like a murder mystery, and by the end, based on information in people’s bios, you’d be able to figure out whodunit. Which made our director furious. But, you know what, it was kind of a sign that he just needed an outlet.
Dylan: I think in both respects a lot of it just comes from - and even as a kid it’s the same thing - it’s the desire for attention. And I realized that the two biggest ways to get attention were either doing things like you said unprecedented, creative and doing something really amazing, or just getting in a bunch of trouble or getting everyone really mad at you. And those were the two easiest ways to draw attention to yourself. That’s kind of why I was always doing some cool creative thing or getting into massive amounts of trouble.
Lauren Prastien: When Dylan was just 22, a play that he’d written while he was a college student premiered off-Broadway. At the time, he was also working in food service, which is honestly pretty common for creatives trying to get their footing or their big break. And he wasn’t shy about this. In an interview he did about this play, he straight-up talked about his day job.
Dylan: There was one question that was like if you didn’t work in theater, what would you be doing right now. And I said something along the lines of I’m not working in theater, I literally work at a pizza place, you should be asking me what I would be doing if I wasn’t doing this.
Lauren Prastien: Over the years, he taught writing and theater at our high school, he did landscaping and telemarketing. He wrote campaign materials for a local election, and most recently, he was supporting himself and his art by working at a fast casual burger joint. Until, you know, COVID-19 hit, and the restaurant shut down.
Dylan: They laid off the entire staff and they kinda acted like they were doing us a favor. Like, we’re laying you off so you can apply for unemployment, but it was kind of a slap in the face, in a lot of ways, especially for people who had been there for such a long time.
Lauren Prastien: Dylan was back home at his parents’ place, trying to find a job in just about the worst climate to try to find a job. And this wasn’t new, he’d already been trying to leave the burger joint for some time to do something more creative, like maybe advertising. Now, he’d left the burger joint, but with next to no prospects for what came next.
Dylan: I was just kind of trying to find my way and I was having a very hard time, you know. I would apply to jobs online and then just not hear anything back for months and months and months. And I remember telling a friend, I remember telling someone that, um, you know, I'm applying for these jobs online but this isn't going to be how I got the job. It's going to be some random occurrence where I just run into someone or someone knows someone or that's how it's going to happen. I have to apply to these jobs because I have to feel like I'm doing something but I'm not confident that's going to be how it works out.
Lauren Prastien: And, sure enough, it was an exceptionally random occurrence. That happened months ago.
Dylan: It was a tattoo that I had just gotten. Yeah, it was a, um, it was a tattoo, that I had just recently, like that week, started work on.
Lauren Prastien: This past January, Dylan was at a birthday party when a man there noticed a tattoo he’d just gotten on his arm of an astronaut.
Dylan: And you know, he was like, oh, that’s nice. Is it finished? And you know, it wasn't, and I was kind of explaining the concept and he thought it was very cool. And that's what got us talking.
Lauren Prastien: It turned out, this man was an executive at an advertising firm that Dylan had heard of before, but had no idea how to get his foot in the door with. But, now, suddenly, his foot was in the door. By the end of the conversation, Dylan was asked to provide some samples of his work.
Dylan: I went through an extensive interview process. I had a phone interview and I interviewed with two people at the office and it was just on and on. And eventually they came to the realization that they didn't quite have something open for me and I kinda just let it be. I was like, okay, this didn't happen.
Lauren Prastien: But fortunately, that’s not the end of this story.
Dylan: I got a call from them and said, Hey, we think we actually might have something open for you. So I had another couple of phone interviews and eventually I was hired.
Lauren Prastien: And so when you got hired, COVID-19 was already like happening. Everyone was quarantining.
Dylan: Oh yeah, it was in full swing. It was maybe, yeah, maybe a week or two into the stay at home ordinance.
Lauren Prastien: So have you physically been in the office since you've been hired?
Dylan: Since I've been hired, no. I did visit the office once when I was interviewing, but since being hired I've not been inside.
Lauren Prastien: And in terms of like your coworkers, how many of them had you like met in person before any of this happened?
Dylan: Um, my supervisor who I answer directly to, I have met. And then other than that, I really haven't met anyone else in the office aside from one other person who interviewed me. But other than that, most of the people I work with, most of the people I'm interacting with on a daily basis are not people I have interacted with in real life yet.
Lauren Prastien: Naturally, Dylan was a little anxious at first. Because he was completely new to this industry. And while he had the chops to come up with some pretty compelling ads, he’d never worked on a team like this before, let alone had to do it online. But overall, it’s been going well. Like, really well.
Dylan: So I was teamed up with someone from the art department and we spent about a week brainstorming and coming up with ideas and flushing them out. And that was kind of the first time where I was like, Oh, this is cool. It was the first time that I was on someone's payroll and I'm being paid to come up with creative, interesting ideas. And that was new and it was very exciting.
Lauren Prastien: So you wouldn’t say that there’s been any kind of like, hindrance or issues from the fact that you and your team aren’t in the same place?
Dylan: I wouldn't say that in the weeks that I've been here so far there's any interaction or any sort of situation where I've been like, “Oh, this would be a lot better if it was handled in person.” Everything else has just, it has felt very as natural as it could feel doing it online.
Lauren Prastien: I think I always had this sort Mad Men perspective of advertising where everyone kind of had to be in a room together and bouncing all these ideas off of each other and hopefully not day-drinking but definitely sort of being able to come up with these brilliant ideas because they were in a space together. But Dylan’s story really got me thinking about something larger that’s been happening in a lot of industries lately, many of which have seen their workforce collaborating remotely for the very first time due to COVID-19. Which raises the point that teamwork might not be something that just happens in person.
From the Block Center for Technology and Society at Carnegie Mellon University and the makeshift studio in my hallway closet, this is Consequential. I’m Lauren Prastien,
Eugene Leventhal: And I’m Eugene Leventhal, coming to you from the popup studio in my bedroom closet. Today we'll be discussing how teams can adapt to the shift to remote work, during and after COVID-19.
Lauren Prastien: I have probably read about three hundred articles about whether or not remote work is the new future of work. Both before and during this crisis, by the way. And I’ve spoken to some people who’ve really found this has changed their perspective on their current work arrangements:
Caitlin Cowan: I’ve been working from home since March and I feel weird about saying this but I really enjoy it, and I’m doing so well with it that it’s something I’d like to bring up with my employer when things go back to normal or whatever normal ends up looking like.
Marie Brooks: So this is the first time in my working life that I’ve been able to see out of a window while I work, and while I love that, it does mean that it’s making me question my bargain with my employer where I give them ten hours of sunlight every day, especially in the winter, and I’m starting to wish I could figure out a thing where I could see the sun a little more often in normal work.
Lauren Prastien: And I’ve spoken to some people that have had genuine doubts about whether their job actually lends itself to this kind of physical separation:
Jess Brooks: The wild thing about science, so like, when we were starting to shut down, it was sort of this gradual thing, because psychologically it’s just shocking to shut down labs. That’s just not in the psyche of a scientist.
Michael Evitts: I’ll be providing therapy at a university, and it’s likely we might be doing teletherapy in the fall if quarantine isn’t loosened. So, that’s going to change a lot. It affects how I work with patients, it also affects patients’ ability to trust and be vulnerable in session.
Lauren Prastien: Genuinely, the jury is still out on whether or not this kind of distributed working environment is actually better for certain industries or if everything will just go back to - quote unquote - normal after we successfully flatten the curve. But after hearing my friend Dylan’s story about entering an entirely different industry remotely, I wondered if maybe there wasn’t some merit to the idea of a creative team - or really any team - being able to accomplish something without physically being together in a space.
But, Eugene, just to get all of our listeners on the same page: how has COVID-19 impacted employment in the United States?
Eugene Leventhal: Well, Lauren, there’s no way to tell exact figures yet. But what we do know is that in the two months prior to May 14th, almost 36 million Americans have filed for unemployment. And according to early data from MIT, about a third of the American workforce is now working from home due to the coronavirus epidemic.
Lauren Prastien: And, maybe this is a given, but are there jobs that lend themselves more easily to working from home than others?
Eugene Leventhal: Absolutely. A team at the University of Chicago found that about 37% of jobs in the United States could plausibly be performed at home. The industries that they found most easily lent themselves to this kind of work were computing, education, legal services, finance, and insurance. Whereas industries like transportation, warehousing, retail trade, agriculture, accomodation and food services did not translate as well to remote work.
Lauren Prastien: I think this actually brings up a pretty important point, which is that remote work is unfortunately, a privilege. Even before the coronavirus, the ability to work from home has always cut along the lines of class, right?
Eugene Leventhal: Right! According to a 2019 Survey from the Bureau of Labor Statistics, only 7% of non-government workers in the US had the option of working from home prior to the coronavirus epidemic. That’s roughly 9.8 million people out of the civilian workforce of a total of 140 million people. If we look at it by the wage level, for those who are in the bucket of highest 10% of earners in the US, 25% of them have access to working from home. For the 25% of individuals who are the lowest earners, that percentage drops to 1%.
Lauren Prastien: Wow.
Eugene Leventhal: And remote work is an option that workers have been interested in! In a 2019 report on State of Remote Work, Owl Labs found that in a survey of US workers aged 22 to 65, 25% would take a pay cut of up to 10% to have the option to work remotely at least some of the time, and only 19% of those on-site workers felt that they did not want to work remotely in the future.
Lauren Prastien: So, why weren’t more people working remotely already?
Eugene Leventhal: Well, that same Owl Labs report showed that the top sticking points for managers who were hesitant to adopt a remote environment were reduced employee productivity, reduced employee focus, issues with employee engagement and satisfaction, concerns over whether or not employees were getting their work done, a lack of communication and engagement with co-workers, reduced team cohesiveness, the…
Lauren Prastien: Okay, okay, I get it. They don’t think employees are going to get any work done, and they don’t think efficiency and teamwork are possible in a distributed environment.
Eugene Leventhal: Basically.
Lauren Prastien: But, I mean, I don’t know. I have this friend who joined this team where they have to work together on these very big, very time-sensitive projects, and it seems like it’s been working out. Maybe teams can be productive remotely.
Eugene Leventhal: I think we should consult an expert.
Lauren Prastien: I think you’re right.
In order to get to the bottom of this, Eugene and I caught up with Anita Williams Woolley. She’s a professor of Organizational Behavior and Theory at Carnegie Mellon, who we’ve had on before to talk about the way people collaborate, either with other people or with robots. Even before COVID-19, Professor Woolley has been really interested in figuring out how team dynamics are impacted by remote work. Here she is now:
Anita Williams Woolley: What we tend to find is that many of the factors that enhance collective intelligence in traditional face-to-face teams are the same as those that you need for a remote online kind of collaboration. It's just that there are certain features that become even more important.
Lauren Prastien: A quick reminder: collective intelligence is the ability of a group to use their shared knowledge to effectively solve problems. And as Professor Woolley has found, communication is one of the cornerstones of collective intelligence. You may remember that story that we referred to last season where she found that a team of regular people with strong communication skills handled a simulated terrorist attack better than actual counterterrorism experts, until those experts participated in a communication seminar.
Anita Williams Woolley: We talk about having the right people for traditional collaboration. Well, it's even more important in an online environment. And when I say, right people, I mean, people who have a high level of social intelligence, including the ability to take the perspective of the other person to anticipate what somebody might know or not know, or how they might respond, so that they can ideally, proactively, either ask or offer information that's going to be helpful to the other person.
Lauren Prastien: In preparing for this episode, Eugene and I spoke to a lot of people across a variety of industries that had gone remote for the first time, and the issue of being able to communicate effectively and, perhaps more significantly, organically came up a lot in a lot of different contexts when we asked how things were going.
Chris Doubet: It makes it harder to have a new teammate who can’t just like, turn to the side and ask you a question. The managers that work underneath me have expressed that a bunch of times, how you have to set up specific times to work with someone to teach them how to do something as opposed to just being able to step over and look over their shoulder and walk them through something.
George Hill: Academic advising has been really different the last couple of weeks working online. When students can’t just come into your office and ask you questions or you can’t just pick up the phone to say, hey, I’m going to send this student to talk to you, that’s kind of a difficult thing.
Lauren Prastien: So I asked Professor Woolley about some things that teams can focus on to improve their ability to communicate effectively in a distributed environment.
Anita Williams Woolley: Really trying to establish norms and routines that get everybody engaged. So for example: There are some teams I've been on where when people are communicating, you might have some people sending out emails say where the email kind of goes out and then there's no response, right? Like, people don't even indicate that they've read it. High performing teams often will establish norms where people will send a response, even if it's, “thanks. Got it. I can't get to this now, but I will by Friday.” Or whatever the response is. But there's just a norm that communication is sent and it is received.
And just also conducting meetings in such a way that enhance engagement. So avoiding having meetings where one or a few people are simply giving out information to everybody, but instead making sure that, especially those remote meetings that can run really long, that there are questions for everybody to engage around, things to discuss if we're making a decision or just getting people's input, trying to really maximize that engagement.
Lauren Prastien: By the way, I spoke to a friend of mine who was working remotely before coronavirus, and setting up clear, consistent norms for communication was at the top of his list for making things run smoothly.
Sean Drohan: I’ve been working remote for six years now and my one big tip for people is just to figure out what minor, important ground rules are important to you, and just find ways to assert them. Mine is that I do not do video calls.
Lauren Prastien: And, listen, he’s onto something. According to Professor Woolley’s research, teams that don’t use video when they collaborate are actually more efficient. So, I’m sorry to say, but it might be better if you save showing off your clever new Zoom background for a chat with friends.
In addition to the tips we’ve just gone over, Professor Woolley has developed a list of best practices to help teams adapt to working remotely, and some of them might surprise you, like that little tidbit about audio versus video calls. You’ll be able to find it on our website (cmu.edu/block-center/) under the Consequential Podcast tab, or as our pinned tweet on Twitter for the next two weeks over at @CMUBlockCenter, all one word. Stay with us.
Through talking to Professor Woolley, I was a little curious about some of the things that I’ve noticed help teams work together better that might be harder to replicate online. I’ve certainly noticed that I can get a lot more work done with a team if I have a pretty good idea of who they are as people. And I don’t think I’m the only one: there’s an entire industry devoted to corporate retreats to help the people on a team to collaborate more effectively and just get to know each other better. And, honestly, even just grabbing a coffee with a coworker can help build a sense of rapport and trust. So, is this something you could maybe lose in an online environment?
Anita Williams Woolley: So, yes. So one of the things that can fall by the wayside when teams move to working completely in an online environment is some of the informal kind of chit chat that you have when you are together face-to-face. So normally if we're gathering for a meeting, in a face-to-face environment, we don't all arrive at the same time. So as some of us are sitting there waiting, we might chit chat and hear about what's going on in each other's lives. And just having that information helps us build more of a relationship that not only creates trust, but also honestly just helps us function better. Then, I understand a little bit more about what's going on in the broader context and can interpret more things that you might say or do having that information more than if I didn't have that information. When we're online, we tend to do that less.
Lauren Prastien: But currently, Professor Woolley is looking at ways to translate this kind of bonding to an online environment.
Anita Williams Woolley: We're modeling it after a classic study that was done by a psychologist by the name of Aron, where he developed 36 questions that lead to love. And he had participants come for a series of sessions in the laboratory and discuss these questions and the questions get successively more intimate. We're not getting into the intimate questions, but even the early questions are quite interesting and revealing, and help give people insight into who you are and how you think and what's important about you. Our hope is that by helping kickstart some of these working relationships in this way, it will give people useful information that help build the trust in the relationships that facilitate work.
Lauren Prastien: You may have heard of this before from that Modern Love essay that went viral about 5 years ago, but let me get everyone up to speed here. In 1997, a psychologist named Arthur Aron at the State University of New York at Stony Brook published an article titled “The Experimental Generation of Interpersonal Closeness: A Procedure and Some Preliminary Findings.” In it, Aron and his team proposed a series of questions and exercises that would foster intimacy between two strangers or near-strangers. These were things like “Given the choice of anyone in the world, whom would you want as a dinner guest?” and “Tell your partner something that you like about them already.” Which, yeah, you can see how that might foster a better working relationship.
And while there are some new limitations that remote work brings up that do require some thoughtfulness to overcome, there are also some new opportunities that come with not needing to put your workforce in the same building. In addition to the environmental and infrastructural impact of no longer requiring your team to commute to work, Professor Woolley sees distributed working environments as highly advantageous when it comes to building a better team.
Anita Williams Woolley: Often it's easier to get the diversity of expertise when you're working in a distributed way because you have a broader array of people you can choose from. So while we find that that's important in any team, it's sometimes easier to accomplish in teams that are online.
Lauren Prastien: Right now, the presence or absence of certain industries in a certain region has had a lot of sway over where people choose to live. According to a 2015 report from the Centre for Cities, proximity to work is the third most important factor that people consider when choosing where to live, preceded by housing costs and proximity to family. But, if you’re working remotely, you don’t have to be physically close to your office. But the flip-side is that many regions whose largest draw used to be access to a given industry may have to reconsider their selling points. Right, Eugene?
Eugene Leventhal: Right. Recently, a lot of companies based in Manhattan, including JP Morgan, Morgan Stanley, and Nielson have encouraged more people to work from home, which could really heavily affect the future landscape of employment - and the greater economy - of a place like New York City, especially given that the borough’s population essentially doubles from 1.6 million to 3.1 million people Monday through Friday due to commuters working in the city.
Lauren Prastien: So not physically going back to work can actually have some pretty pronounced impacts on a local economy, then?
Eugene Leventhal: Yeah, definitely. But the tradeoff, like Professor Woolley said, is companies have access to a much bigger talent pool, and by extension, can assemble larger teams.
Anita Williams Woolley: Another thing that we've talked about before is the number of people in face-to-face teams. It's important to keep them relatively small, like say five to seven people. When we get into an online environment, it can be possible to actually have a larger number of people, but it means that you're also going to have to be really good with those coordination tools so that everybody knows what's going on. It's not death by a thousand emails.
Lauren Prastien: Hm. Death by a Thousand Emails. That sounds like a really good title for a podcast.
Anyway, to bring us back to what we discussed earlier in this episode, a lot of the hesitation that has prevented managers from providing the option to work remotely is this fear of being unproductive. So I asked Professor Woolley how organizations might want to evaluate productivity at a time like this.
Anita Williams Woolley: Often organizations function, and individuals within organizations function, with a real lack of clear goals. Or in some cases they are what we call process-focused, meaning a role is defined not by what I'm supposed to accomplish in a day, but by the number of hours I'm supposed to spend and where I'm supposed to spend them. If an organization can make a successful transition from that way of thinking to a way of thinking that says, “no, I'm hiring you to accomplish certain goals. And those goals could often be accomplished in a variety of places, in a variety of ways and a variety of time points.” That will make them more successful in transitioning to an online environment. So the organizations that have successfully weathered this change that we are experiencing now have either already been operating that way or have shifted their mindset to operating that way. And if they have, then they'll be able to evaluate whether or not it was successful.
Lauren Prastien: And just anecdotally here, a bunch of the people that Eugene and I spoke to have actually found this time to be more productive than when we were in an office. Here’s one now:
Cassie Lebauer: I realize that I actually work really well without public distraction because I used to think that things distracted me, but then I realized that I create my own distractions that then in turn distracted me. So, you know that same concept of your second grade report card saying, “this person talks to everyone all the time.” It’s kind of that inverted cycle.
Lauren Prastien: But also, there’s a pretty big caveat to all of this. Remember, we’re living through a global pandemic. No scientist would call this a controlled experiment in whether or not a workforce can adapt to and productive in a remote work environment.
Anita Williams Woolley: So that's gonna I think muddy a little bit the evaluation of how well this has gone because people don't have the support that they would normally have in a typical environment, if suddenly the only thing that changed was that they were working at home versus in the office. But overall, I mean, just anecdotally talking to people, I think there are a number of organizations that are finding that they are able to accomplish very similar goals very successfully even given all the constraints that we're operating under.
Lauren Prastien: You may have seen that now-viral tweet from Neil Webb. You know, the one that says: “You are not working from home; you are at your home during a crisis trying to work.” Which is really important to remember! This can be a testbed for your organization, but it’s not a perfect one. Particularly for parents who are watching and teaching their children while also being expected to be full-time employees.
Biz Nijdam: First of all, the fact that there are three children under five in our house makes it exceedingly difficult to get anything done, but our biggest problem is three adults working, quote unquote, full time on limited bandwidth, so we had to start using Google Calendar to ensure that we don’t have overlapping Zoom meetings. So I made sure that nobody was in a Zoom meeting currently so I could give you this sound bite.
Lauren Prastien: As we discussed in our episode on remote education, remote work is also predicted on certain presumptions, like access to reliable Internet. And according to Professor Woolley, as remote work becomes more common and as people are expected to stay home for longer and longer periods of time to wait out the virus, there may need to be some policy changes to accommodate the new infrastructural demands this requires.
Anita Williams Woolley: Right now those of us who suddenly had to work, moved to remote working are bearing a lot of the costs of setting up our infrastructure at home. I mean there are some people who are working with insufficient bandwidth for example, you know, to be able to really be effective online or to do all the things they would want to do. So I think there could be a variety of policies that would support more access to broadband internet, maybe universal access. So that that is a level playing field and everybody has the ability to work or learn from home.
Lauren Prastien: It would be great to see people have the opportunity to be able to take advantage of these opportunities on a level playing field, like Professor Woolley said, particularly as more companies have decided to extend their option to work from home to the end of this year and the beginning of next. Just the other day, Twitter’s CEO Jack Dorsey gave most of his workforce the option to work from home forever, and it seems like other companies might follow suit to ensure that their workforce remains safe. But again, to accomplish that, it’s going to be important to see the infrastructural and organizational growth necessary to sustain this.
Eugene Leventhal: Before we go, we wanted to take a moment to thank our fantastic intern, Ivan Plazacic. Ivan has been with us since the beginning of this podcast, and this is his last episode. Ivan, we have been so lucky to have you as part of our team. Getting this podcast really wouldn’t have been the same without you. We appreciate all your hard work, and we wish you all the best in your new adventures.
Lauren Prastien: Ivan, thank you for bringing your talent, creativity and insight to these episodes. We’re all going to miss you very much.
Ivan Plazacic: Thank you both. It has been a major pleasure and a wonderful journey, and I hope our paths will cross again.
Eugene Leventhal: In two weeks - so that’s June 3rd - we’ll have our mini-season finale, where we’ll be talking about the lofty task of figuring out what it takes to safely reopen a state. Until then, this was Consequential. Be smart, stay safe, and please wash your hands.
Lauren Prastien: Consequential was recorded at the Block Center for Technology and Society at Carnegie Mellon University. The Block Center was established to examine the societal consequences of technological change and create meaningful plans of action. To learn more about Consequential, the Block Center and our faculty, you can check out our website at cmu.edu/block-center or follow us on Twitter @CMUBlockCenter, all one word. You can also email us at consequential@cmu.edu.
This episode of Consequential was written by Lauren Prastien and was produced by Eugene Leventhal and our intern, Ivan Plazacic. Our executive producers are Shryansh Mehta, Scott Andes, and Jon Nehlsen. The music you’ve heard throughout this episode was produced by Fin Hagerty-Hammond.
This episode uses findings from the Bureau of Labor Statistics’ National Compensation Survey, the working paper “COVID-19 and Remote Work: an Early Look at US Data” by Brynjolfsson et al., and a white paper by Jonathan I. Dingel and Brent Neiman titled “How Many Jobs Can be Done at Home?”, Owl Labs’s report “State of Remote Work 2019,” the 1997 paper “The Experimental Generation of Interpersonal Closeness: A Procedure and Some Preliminary Findings” by Aron et al., and a 2015 report from the Centre for Cities titled “Urban demographics Why people live where they do.”
It also references a viral tweet posted by @neilmwebb on March 31, 2020.
Thank you to our respondents for speaking candidly about their experience working remotely. These include Caitlin Cowan, Marie Brooks, Jess Brooks, Michael Evitts, Chris Doubet, George Hill, Sean Drohan, Cassie Lebauer, and Biz Nijdam. Thank you as well, to my friend, Dylan, who had to remain anonymous for this project.
S2: An Update from Consequential
Lauren Prastien: Hello everyone.
Eugene Leventhal: It’s us, Eugene and Lauren, your hosts.
Lauren Prastien: As you may know, today we planned to release our episode on coordinating local and state efforts to minimize the public health and economic impacts of COVID-19.
Eugene Leventhal: But in light of the events of the past week, we wanted to be respectful of the current public discourse, and we felt that we couldn’t just release this episode as though these events weren’t happening.
Lauren Prastien: So we’ve decided to hold off on releasing the episode for the time being.
Eugene Leventhal: And we’d like to echo the sentiment expressed by Carnegie Mellon’s President Farnam Jahanian that it is up to each one of us – no matter our background – to confront and dismantle racism and injustice wherever they exist.
Lauren Prastien: Thank you for sticking with us this season, and we’re looking forward to sharing our fifth episode of season two with you soon. In the meantime, we’ll be thinking of all of you, and hoping for your safety during this time.S2 E5: How Do You Reopen a State?
Lauren Prastien: Responding to a crisis like COVID-19 requires coordination on several levels and between a lot of different public and private entities. Over the course of this past mini-season, we’ve looked at some of smaller pockets of that coordinated effort, from discussing the benefits and drawbacks of collaborations between public health entities and private companies in order to track and learn about the virus to looking at how the move to remote work requires infrastructural support.
So, the other day, Eugene and I were talking to Professor Rick Stafford, who you might remember from last season is a public policy professor here at CMU. We were about to start an interview with him about the university’s efforts to help fight COVID-19 when something that happened 40 years ago came up right when we were about to hit record. And so then we hit record, and this is what happened:
Lauren Prastien: You mentioned the Three Mile Island accident. And this was - I guess also sort of for our listeners - this was about forty years ago now, wasn’t it?
Rick Stafford: Exactly. Yeah. Good memory there. You want me to reflect a little bit on that?
Lauren Prastien: Do you mind?
Rick Stafford: So to put this in context, I was the governor's - I'll call it - policy chief for the administration for a brand new governor. Governor Dick Thornberg. He took office in January 1979. And he and I were sitting side by side with a group of legislators on March 28th, 1979 when an aid at 7:50 in the morning came into the room and handed the governor a pink slip. Now this is before cell phones, so he wasn't being buzzed on his cellphone. That pink slip said, please call the director of the Pennsylvania Emergency Management Agency immediately. So, he whispered in my ear that he would have to leave the meeting for a moment. And I carried on with this group of legislators who we were trying to convince that we had a great a budget proposal for the coming year, and they were supposed to be voting on it ultimately. So, he left the room. I took charge of that discussion. And he came back a couple of moments later and whispered in my ear, “we've had an accident at Three Mile Island.”
Clip: For many years, there has been a vigorous debate in this country about the safety of the nation’s 72 nuclear energy power plants. That debate is likely to be intensified because of what happened early this morning at a nuclear power plant in Pennsylvania.
Clip: The accident occurred here at the Three Mile Island nuclear plant, a dozen miles south of Harrisburg.
Clip: It happened at the number two generator about four o’clock this morning. Something caused the secondary cooling system to fail. It shut off the reactor, but heat and pressure built up and some radioactive steam escaped into the building housing the reactor and eventually out into the plant and the air.
Clip: A nuclear safety group said that radiation inside the plant is at eight times the deadly level, so strong that after passing through a three-foot-thick concrete wall, it can be measured a mile away.
Clip: There is no imminent danger, that’s the official advice tonight, but many people aren’t buying it. Thousands have packed their luggage and they’ve left. Banks report many withdrawals, telephone lines have been busy, outdoor activities like the horse races have been cancelled. People are afraid of what they can’t see, radiation. And they’ve heard so much contradictory technical jargon from officials that the first casualty of this accident may have been trust.
Lauren Prastien: So, yeah, you can see why the subject of Three Mile Island came up with Professor Stafford. But anyway, let me hand this back to him.
Rick Stafford: And that began a sequence of actions in which it was classic in the sense of who is in charge? The utility of taking care of things? The nuclear regulatory commission of the federal government, which has jurisdiction over all nuclear power plants and regulatory matters? The people who live right there in the municipality and the county that the facility insisted in? Obviously they had a big stake and every legislator in Congressperson whose district it resided in…everyone began to worry.
And, the reason that fear was particularly enhanced was a movie called The China Syndrome, which starred Jack Lemon and Jane Fonda and Michael Douglas. It was all about a nuclear accident in California that threatened the lives of everybody in Southern California. So, it was a pretty popular movie at the time playing in Harrisburg. So, circumstances collided to make this a particularly worrisome thing.
Lauren Prastien: So, The China Syndrome was released in theaters on March 16, 1979. That’s twelve days before the Three Mile Island nuclear accident on March 28. And, the movie doesn’t really make emergency managers look that trustworthy. Just listen to the first like 10 seconds of the trailer.
Clip: It’s about people...people who lie, and people faced with the agony of telling the truth.
Lauren Prastien: So the City of Harrisburg is seeing this movie - which is, by the way, a work of fiction - about a massive coverup of the severity of a nuclear accident and then like a little over a week later, they’re told by their public officials, “hey, so there was a nuclear accident, but, we’ve got it under control.”
Rick Stafford: But what became increasingly worrisome was the information that we needed to understand what leadership the governor should take. Because Pennsylvania Emergency Management Agency actually has a role in managing any such emergencies, and every county has its own emergency management agency director, believe it or not. So, there was a lot of confusion about, well, what is the situation? How bad is it?
Lauren Prastien: In order to address the Three Mile Island incident, the governor established an ad hoc team of staff members and cabinet members to look at how best to communicate to local and federal officials. By that Saturday, which was 3 days after the incident at Three Mile Island, President Jimmy Carter and Governor Dick Thornburgh were on the phone coordinating how the state and federal governments would work together on this issue.
Rick Stafford: And by Sunday, the president, President Carter, Jimmy Carter, came literally to Three Mile Island and to the control room, joined by the governor and the lieutenant governor.
Lauren Prastien: But people were still pretty anxious.
Rick Stafford: Basically, four County areas of Pennsylvania have basically, people just had left! They made the decision that we don't understand what's going on, we're getting out of here.
Lauren Prastien: I kind of can’t get over the parallels here, but the point is that as all of this was happening, the Commonwealth of Pennsylvania was figuring out that there were two big issues to take on. The first, fortunately, they didn’t feel a lot of the brunt of, as a formal evacuation order wasn’t put into place. But, as the counties began comparing their existing evacuation orders, they realized that they weren’t as coordinated as they could have been.
Rick Stafford: And a good example of that was that two counties across the river from each other, connected by a big bridge had emergency evacuation plans, one to go west over that bridge and the other east over that bridge. So, it would have been a pretty interesting situation to contemplate.
Lauren Prastien: And the second problem came to a head ten days after the incident, when the government determined that while there had been radiation releases, they didn’t necessitate the use of potassium iodine or other measures to combat radiation. Which meant that it was safe for people to return home.
Rick Stafford: As it turned out when after ten days and people began to say, well, heck, this is a heck of an accident.
Lauren Prastien: Can I just say that I love Midwestern understatement so much?
Rick Stafford: This is a heck of an accident. Estimates even at that time exceeded a billion dollars to clean up, this injured a nuclear reactor. And no one under public policy at the time could be designated as directly responsible for financing that. The company had limited insurance. In order to get money out of their rate payers, you had to go through the Public Utility Commission. The nuclear energy industry was sort of saying, well, heck, that didn't happen to us. That happened to GPU. The federal government said, well, wait, yes, we have the laws that govern nuclear energy, but we don't have any liability here. We don't have any money to finance the cleanup.
So basically, after almost two years, the governor - who by the way, the state under state law had no responsibility for this, but he had an…he felt an obligation for leadership - unveiled at the TMI site a cleanup plan that involved all of those parties to step to the plate and put money into a pool to enable the financing of the cleanup.
Lauren Prastien: Ultimately, it did cost about a billion dollars to clean up Three Mile Island, and it took more than fourteen years, ending in December of 1993.
Rick Stafford: But it, it does demonstrate, once again, these crises require the response of many different levels of government and the individual. And we don't anticipate them adequately in many times. And I think we're - editorial comment - in exactly that situation with the pandemic.
Lauren Prastien: Today, Professor Stafford is part of a team at Carnegie Mellon University that’s collaborating with the office of Governor Tom Wolf to help guide the state in making economic and public health decisions for addressing the COVID-19 crisis.
Rick Stafford: We're really facing a situation, which triggers my interest in public policy making, which is really my area of expertise. It isn't analytics. It's how do governments respond with policies that deal with both routine and unusual circumstances? Basically, the candidates in broad speaking are the federal government in some way, shape or form, the state government in some way, shape or form, my county or municipality in some way, shape or form, and perish the thought in the end, the final policy maker is the individual person who says, well, what am I going to do in the face of all this information?
So in the longer run, one of the interesting things to take away from this pandemic will be how do we adjust our public policy and our preparation at the federal, state and local level to have more clarity, more direction, better information for that individual who has to make up his or her mind about their own families, their own situation?
Lauren Prastien: From the Block Center for Technology and Society at Carnegie Mellon University and the makeshift studio in my hallway closet, this is Consequential. I’m Lauren Prastien,
Eugene Leventhal: And I’m Eugene Leventhal, coming to you from the popup studio in my bedroom closet. Today we're asking our experts: how do you coordinate a crisis response to an issue like COVID-19, where every public health decision has economic ramifications, and every economic decision has a direct impact on public health?
Lauren Prastien: Like Professor Stafford said, decision-making around the response to the COVID-19 pandemic is happening at every level, from federal decisions around issues like economic stimulus, to the states’ decisions about reopening non-essential businesses, to individual decisions that people like you and I make when we decide whether or not we’re going to adhere to certain guidelines, like wearing a mask in public.
But like Professor Stafford said, all of that decision-making has to do with what information we have at our disposal and what we decide to do with it. And right now, as you might be aware, a lot of the decisions on both the federal and state levels have to do with the notion of reopening the economy.
It’s a series of decisions, each one with ramifications for public health and the economy. Because those two spheres don’t exist in a vacuum. Every public health decision we make has economic ramifications, and every economic choice could positively or negatively impact public health.
To get a better understanding of what’s happening and how those decisions get made, Eugene and I talked to Ramayya Krishnan. He’s the Dean of the Heinz College of Information Systems and Public Policy here at Carnegie Mellon, and he’s also the faculty director of the Block Center. Currently, Dean Krishnan is leading CMU’s efforts to help guide the state’s safe reopening.
Ramayya Krishnan: I think first and foremost, the concern was to really understand what the state of our public health systems were and were they capable of absorbing and being able to serve the needs of citizens who fell ill and needed access to medical care? So, you might have all heard about the phrase flattening the curve. and the whole idea there was to sort of through the application of shelter-in-place and social distancing to reduce the likelihood that people would transmit infection among one another so far, community transmission. And that in turn would then reduce the number of people that fell ill and had bad outcomes that would then result in their need for hospitalization.
Lauren Prastien: And choices about how that might look and how long it could go on were decided on a mostly state-by-state basis.
Ramayya Krishnan: Each state took into account the extent to which it was being affected by the pandemic and following White House guidelines, decided to only keep a set of essential industries open and requiring a set of industries that were deemed nonessential to be closed.
Lauren Prastien: In an article for CNN Business, the journalist Scottie Andrew considered, “what constitutes an essential business?” What he found was that from state to state, there really wasn’t a consistent answer. This was back in March - which now feels like twenty-five years ago, I know - and written around the time when only 17 states had issued stay-at-home orders. But there were some interesting deviations between which industries were considered nonessential in which states, and which were considered life-sustaining. Particularly when it came to gun stores, liquor stores, marijuana dispensaries and golf courses.
Ramayya Krishnan: If I might use Pennsylvania as an example, I believe the executive order that Governor Wolf signed, initially was on March the 18th, that identified the set of industries that were deemed essential. And these industries were identified at what are called four-digit net codes. That’s the level of specificity with which they were identified. and that was done initially on March 18th and then tweaked on April the first. And then there were a three-phase color coded system developed to determine the state in which each of the 67 counties in Pennsylvania were in. So, were, so a red phase, which is a shelter place face. A yellow phase, which was the next phase after, which some relaxation was offered of these orders. And then what's called the green phase. So, when we talk about reopening, one has to think about what happened between closure to where this consideration was given to the relaxation of the orders that were in place.
Lauren Prastien: It’s worth noting that when we say closure, this was never a complete closure. Essential businesses were still operating, like pharmacies, gas stations, mail services and banks, often with modifications to ensure the safety of both employees and patrons.
Ramayya Krishnan: I'm sure each and every one of you who’s been to a grocery store, have noticed that, at Giant Eagle, which is a local grocery store here in Pittsburgh, that now you have plexiglass that separates you from the checkout clerk. And where you used to swipe your card is no longer is right between you and the checkout clerk, but it's actually six feet away. And that's an example of a workflow reengineering. As much as you see painted one-way signs along the aisles in a grocery store to ensure that people flow in one direction along an aisle and therefore able to better maintain social distancing.
Lauren Prastien: So, some things have been operational. And as more and more regions of Pennsylvania have moved into the yellow phase, we’ve seen more workflow reengineering in industries that were previously closed. Like construction, where the sequence of what work is done when has been reordered to allow workers to maintain social distancing.
But, the issue, of course, is that many aspects of our economy have not been restored. Like Eugene told us last week, prior to May 14th, almost 36 million Americans had filed for unemployment. Today, that figure is estimated to be around 41 million. To put that in perspective, that’s like if you told everyone in California, the state with the highest population, and everyone in Wyoming, the state with the lowest population, that they had lost their jobs. But reopening industries too early could expose people to the virus in a way that we’re not equipped to manage.
Ramayya Krishnan: So you can see how public health and economics are not siloed and distinct from one another, but actually have to be dealt with together as one thinks about the next phases, as we get to the other side of the pandemic.
Lauren Prastien: So how do you even begin to tackle something like this?
Ramayya Krishnan: I think, this is certainly, a response to a pandemic such as what we're going through requires a system of systems perspective.
Lauren Prastien: Hey, Eugene, you went to policy school. What does that mean?
Eugene Leventhal: Okay, so a system of systems is a collection of trans-domain networks of heterogeneous systems that are likely to exhibit operational and managerial independence, geographical distribution, and emergent and evolutionary behaviors that would not be apparent if the systems and their interactions are modeled separately.
Lauren Prastien: I’m sorry, what?
Eugene Leventhal: Basically, it means taking a bunch of seemingly independent systems and viewing them instead as one big, complex system, within which all those smaller systems are interacting with each other.
Lauren Prastien: So like a body?
Eugene Leventhal: Yeah, exactly like a body. You have your circulatory system, your respiratory system, your skeletal system, etcetera etcetera. And those systems all have their own independent functions.
Lauren Prastien: Okay, I think I get it. Because then those systems also interact with each other and depend on each other. Like how your circulatory system is responsible for delivering blood to the bones in your skeletal system, and then your skeletal system, in addition to, you know, maintaining your corporal form, is also responsible for making new blood cells for the circulatory system.
Eugene Leventhal: And then the whole thing together makes a functioning body. That’s a system of systems.
Lauren Prastien: And so by extension, then, a system of systems problem is when you have an issue that impacts a lot of different, interdependent systems. And that’s COVID-19 right now.
Eugene Leventhal: Exactly.
Lauren Prastien: Okay. Great. I’m going to hand this back to Dean Krishnan now.
Ramayya Krishnan: So given that it's a system of systems problem, you need data from multiple systems. Many of the States are finding themselves having to cobble together these data systems and the associated curation and governance that's required to support their policy making.
Lauren Prastien: I think I always make this mistake of considering data to be this sort of ephemeral thing, but it is and also it isn’t. Like we’ve talked about this season when we looked at coordinating public health efforts and contact tracing, data lives in different places, is recorded in different ways, and is compatible or incompatible with other forms of data. And so the data you might need to solve a problem associated with COVID-19 might be residing in very disparate places and have never been combined before. Here’s a good example of that from Dean Krishnan:
Ramayya Krishnan: So given a county like Elk County, might have a relatively small county hospital that might not have a large number of medical beds or ICU beds or ventilators. ICU beds and ventilators were widely regarded as a critical resource because people who had complications needed access to those facilities. So one of the policy questions that comes up, which is for which data about hospital systems as relevant, is to ask the question, is there adequate supply of ICU beds and ventilators to serve the needs of people that might potentially have complications from COVID-19 and require these kinds of services?
Lauren Prastien: Which seems like a simple enough question, right? Like, okay, just count the beds and ventilators, and tell the state what your county has available, let them figure out how to close the gap of what you need. Done. But here’s the problem: if you live in Southern Elk County, you’re part of the health referral region of Allegheny County and Pittsburgh, but the Northern part of Elk County gets sent to Erie. So, you don’t just need to know the capacity and availability in Elk County, you also need to know which health referral region patients are part of. And, by extension, you need to know what resources those health referral regions have. That’s a lot of data, and that data lives in different places and is changing constantly as patients are admitted, treated and transferred.
And that also applies to reopening operations of industries, which are often interconnected.
Ramayya Krishnan: There are other such examples that arise when one thinks about the economic, aspects of the crisis since both on account of the closing of certain industries, as well as on account of the fact that some industries are part of a supply chain and are embedded in a supply chain, either globally or nationally.
Lauren Prastien: So, yeah. Hypothetically, let’s say you and I run a pizzeria. We decide that we can stay open for carry-out and delivery. But the problem is that while we probably make our own dough, we’re not harvesting the wheat to make the flour that goes in that dough. We’re not growing our own tomatoes to make the sauce. We don’t have a bunch of cows in the back that we’re milking to then curdle into cheese. And, oh wait, we’re open, but the factory that makes the boxes that we put our pizzas in to deliver them is not, and we have some boxes, but what happens when those run out?
And this also pertains to supporting closed industries and helping individuals who have lost their jobs.
Ramayya Krishnan: You could imagine, being a manufacturing company, located in Pennsylvania, that is one of the industries that was closed and everybody who is employed in that particular industry is unemployed. And one needs to understand where are the people who are unemployed, where do they reside, what is their gender, how old are they, and what kind of unemployment benefits are they going to go to receive? And in addition to that, as you know, the federal government passed the CARES Act, which provides individuals with an additional amount of money, about $600 over and above the $459-odd that Pennsylvania provides by way of unemployment benefits compensation. so that you get a holistic picture by county, by industry of where these individuals reside and who are the ones that are impacted.
Lauren Prastien: Not to mention, not all of this data is just something that the state has at its disposal, because it might not even be data that the state is collecting.
Ramayya Krishnan: Oftentimes there may be a need to even combine data from private sector sources. So for instance, banks by virtue of having direct deposit information about paychecks, when somebody gets unemployed may have a signal of what's happening with respect to unemployment or employment, pardon me, by industry, by county, again, at the level of these industry codes, the net codes that I spoke about. So you might be able to combine private data sources and public data sources to provide policymakers with a rich picture of what's going on.
Lauren Prastien: So once you accumulate all of that data, what do you do with it?
Ramayya Krishnan: To give some concrete examples of the COVID response. Let me begin by talking about the work of two colleagues. The first is work on a tool called COVIDcast that provides a very granular view of what the extent of demand that is for hospital services or for medical care at the level of a county. This is particularly important and relevant, especially from a state governor’s standpoint, because the unit of analysis for which decisions are made is that the level of a county.
Lauren Prastien: Looking at these issues at the granularity of a county might seem arbitrarily specific, but it’s actually pretty important. In just the Commonwealth of Pennsylvania, we had counties in the red phase and the yellow phase at the same time, and now we have counties in the green phase and the yellow phase at the same time. By the time you’re hearing this, there will be counties like Lawrence and Montour that have moved into the green phase, while all the counties that surround those counties are still yellow. And you can’t capture that level of variation just by looking at a state as a whole entity or even just regionally.
Ramayya Krishnan: And an interesting aspect as it connects to the data discussion we just had is that COVIDcast has a partnership with Google and with Facebook where individuals, and you can think of this as, you know, civil society or the individual sector, if you will. We talked about the private and the public sector. Think of these as individuals who can report in how they're feeling and what symptoms they're having, either via Google or via Facebook, which then becomes another input to COVIDcast.
Lauren Prastien: In addition to having that clear picture of what’s happening on a county level so that you can make informed decisions, something else that’s pretty important when you have industries reopening is being able to know if people have been potentially proximate to others who have been infected. So you’ve probably heard this more broadly in the context of contact tracing earlier this season, but then when you take that to a county level, that process is pretty time-intensive.
Ramayya Krishnan: A tool that, one of our colleagues at CMU has developed, which is now available on the app store, both for the Apple iPhone as well as for Android, is an app called NOVID. And what NOVID does, is in a very privacy-preserving way, it permits individuals to be notified if they were proximate to somebody else who has indicated that they tested positive for COVID-19. A particular and interesting facet of NOVID is its use of ultrasound.
Lauren Prastien: So, when Dean Krishnan says ultrasound, he’s not referring to the kind of ultrasound you’re probably thinking about. But like an ultrasound machine, NOVID uses ultrasonic sounds - which are sounds that you and I can’t hear, but a phone could hear - to perceive the presence of another person. NOVID - which doesn’t collect any of your personal information - just asks you to check off a status “I have not tested positive for COVID-19” or “I tested positive for COVID-19” and then uses ultrasonic sound waves to make phones with the NOVID app aware of each other. And if someone who hasn’t tested positive was near someone who did test positive during the window where they could have been contagious, the app will let you know, without collecting or sharing any personal information or data.
Lately, a lot of contact tracing apps have been criticized for using methods like Bluetooth and GPS, because those signals can pass through walls and floors. It’s why you can put on Bluetooth headphones, be listening to a song on your phone, and then walk into the next room without your phone and still hear the song through your headphones. But that doesn’t happen with ultrasound. So it’s more accurate, and it wouldn’t give you a notification, if, say, your downstairs neighbor who you’ve never seen or spoken to informed the app that they tested positive.
COVIDcast and NOVID are still in their early phases, and so we’re hoping to check in with the teams working on them later on in the fall to see how everything’s going.
I want you to think about data like pointillism. You know, that art form that’s just a bunch of little dots. Like, Seurat’s A Sunday Afternoon on the Island of La Grande Jatte, which is made up of millions of dots. And you if get really, really close to it, you just see the dots. And then if you stand back far enough, that’s when the dots stop being just a bunch of dots and start to make sense. But if I just stood with my nose against that painting - first of all, I’d get kicked out of the Art Institute of Chicago, and second of all, I wouldn’t understand what I was looking at. If you told me that painting was say 300,000 green dots and 75,000 white dots and 50,000 yellow dots etc etc, there’s literally no way I would be able to tell you what that painting was of. Because it matters where those dots are placed in relation to each other. And that’s kind of what raw data is like - it’s often just the 300,000 green dots and 75,000 white dots and 50,000 yellow dots. But data can work together to form a picture of something precisely because there’s an abundance of it, but you usually can’t just look at its constituent parts and immediately get an answer.
Rayid Ghani: Everything is connected, right? It's not as if your health is independent of your employment. It's different from your family, which is different from, I don't know, food and transportation. It's all connected. and I think it's obvious to anybody who lives in the world, but typically in these types of things, you know, we live in data silos, right?
Lauren Prastien: That’s Rayid Ghani. He’s a professor of machine learning, information systems and public policy here at Carnegie Mellon, and like Professor Stafford and Dean Krishnan, he’s part of the team working to help formulate data-driven insights to guide Pennsylvania’s public health and economic response to the COVID-19 epidemic.
In gathering all those disparate and changing pieces of data that we talked about with Dean Krishnan, you end up with a lot of data, which is hard to work with. So one of the ways that a municipality or state might work with that data is through using machine learning to quickly process and analyze that data. But Professor Ghani says that needs to be taken with a grain of salt.
Rayid Ghani: I mean, machine learning is isn't some sort of a silver bullet that you install it or apply it and magic happens. So I think, I think I would sort of think about it more as, can evidence based policymaking, can evidence based approaches to policymaking, help reopen the economy, the world, state, country, county, in a better way than a non-data-driven, not evidence based approach? And I think the hypothesis is yes.
Lauren Prastien: So what can something like this accomplish?
Rayid Ghani: I think any sort of data driven approach helps us understand better what's happened in the past. Monitor what's actually going on right now. Anticipate or predict what might happen. And then help take interventions, take policy, make policy decisions and changes that hopefully improve the outcomes. Right?
Lauren Prastien: Right.
Rayid Ghani: In order for this to work, we kind of need a lot of different things to happen. It's not, you know, install machine learning and the magic wand works. It's really, we need good data. We need good infrastructure and good people and tools who can help analyze that information. I mean, good interventions that actually change outcomes, and all of that has to be governed by good policy goals. That's a lot of good that needs to happen before the economy can reopen and can function.
And so I think the whole notion of, you know, when you're trying to figure out, the way we've been thinking about this problem is really less from the side of what does the health department do? We're thinking of it as more, the state has certain policy levers, right? They're thinking about opening or reopening as a set of choices to have to make, you know, what should they reopen when? And if they do that, what are the consequences of that? Like there are going to be economic impacts, there are going to be health impacts of that, and there are going to be social impacts of that? And those impacts will be different on different types of people in different geographies and different industries. And they're all connected, right?
Lauren Prastien: And these things are connected in ways you might not expect.
Rayid Ghani: So the state has these guidelines around, if you have fewer than 50 out of a hundred thousand cases in the county, then we're going to consider putting you into the yellow zone. You know, the yellow phase. But one thing to kind of keep in mind as you might be, the county rate might be lower than that, but if you open that county, there are surrounding counties that have a lot of people who live there who commute to work to this county. So if your surrounding counties have a higher rate and the people who are living in those counties are going to commute to work to your county. In effect, opening your county effectively increases your infection rate. And so are you ready again, ready for that? Have you thought about that? So we've been doing it sort of generated a list of these risk scores, risk indices, across counties and providing that to the state to think through comparing different counties, comparing different industries, and provide that additional input as they make reopening decisions.
Lauren Prastien: Like we said in our episode on remote work, many cities’ populations ebb and flow in accordance with commuters. Like New York City, whose population effectively doubles on Monday through Friday while people are on what is essentially an island, going to work. And in the case of somewhere like New York City, those commuters don’t just return to other boroughs or even different counties in New York, they sometimes cross state lines into places like New Jersey and Connecticut. And it’s not just big cities, this happens all over the country. According to the US Census Bureau’s 2013 report on county-to-county commuting flows, more than a quarter of workers in the United States - 27.4%, to be exact - worked in a different county than their county of residence. In one region of Virginia, called Manassas Park, 91.2% of workers were found to work outside of their county of residence.
This kind of reminds me of Professor Stafford’s example with the conflicting evacuation plans that would have sent two counties careening into each other while they tried to cross the same bridge from opposite directions. Responding to a crisis like this requires an intricate kind of coordination. And while machine learning can help you coordinate something like that, it can’t make the decisions about the goals that you’re coordinating around.
Rayid Ghani: What we've been kind of working on is helping build some of these tools that can help implement that policy, but we're not going to come up with a policy goal. That's a societal question. The type of things we're doing is kind of helping think through the impact of that and the risks of that, but also a way to implement those goals.
One of the things that's been happening is a lot of states have been sort of talking about, well, we can open, but we need to have things like robust testing capacity and contact tracing ability. And there is no definition for robust and enough, right? I think that's where some of the direction that we've been taking is also what does it mean to have testing capacity? We're not gonna have enough tests. According to all the estimates, we need somewhere between 1 and 5% of the population being tested every week. We're not going to have enough tests. So, then the question is given that we need testing in order to reopen, well, given that we're not going to have enough, we need to deploy these tests in a smarter way. We need to figure out what are the policy goals that we want to achieve with this testing program. Do we want to make sure that we prioritize vulnerable people? Do we want to make sure we prioritize people who are likely to spread things? Do we prioritize people who are facing comorbidities in other ways and are at risk of other things? And then that that's going to be the harder decision, is what are your policy goals?
Lauren Prastien: And that policy extends beyond the concept of figuring out how to get people back to work safely, and that’s where values come in.
Rayid Ghani: As you said, you know, there's a system of systems thing, right? As soon as we do that reopening, we think magically the world's going to go back to what it was two months ago. And we know it's not, but we're not…we need to kind of focus on those programs to support the people who have been affected, but who are going to continue to be affected even after we reopen.
So one part is the reopening and what you reopen, when you reopen, where you reopen, and how you reopen, under what conditions. But the second piece is what do you do after you reopen in terms of support programs? And the third piece is how you monitor and test and contact race and all that stuff, right? So people have been talking a lot about the first piece, which is reopening, when and where, not necessarily under what conditions as much, but a little bit. People have been talking a lot about the third piece, which is testing and contact tracing and isolation. The middle piece of what do you need to do to support the one monitoring, but also, support programs that need to be created? For example, people who have filed for unemployment, over the last few weeks, some of them are at risk of long-term unemployment. Some of them are not going to get…even when the industries reopen, they are unlikely to get their jobs back. We need to prioritize supporting them. At least that's the policy goal I would want the government to have.
Lauren Prastien: According to Professor Ghani, there’s a lot of ways to go about this, and there are ways that using existing data could help. For instance, using the data from individuals applying for targeted support programs now to create and tailor programs later to continue to support those individuals who may be at risk of long-term unemployment.
The fact is, there’s a long road ahead in reopening states and returning to whatever normalcy might look like after this. And like Professor Stafford said at the beginning of this episode, crises like these require collaboration between different levels of government, different private and public entities, and what he calls “the individual.” In other words, us. Because it’s like Dean Krishnan said, we are within a system of systems. In the words of my favorite Internet meme: we truly live in a society. I want you to remember that. By this, I mean - as an avid consumer of science fiction, something that’s always bothered me about the genre is that often - but not always - there’s an emphasis on this kind of rugged individualism when things get chaotic. I don’t know what the world is going to look like the next time we hear from each other, but what I do know is that everything is connected, like Professor Ghani said. Not just our data, but us.
Eugene Leventhal: This was our final episode of our mini-season on the intersection of technology and society in the time of COVID-19. Thank you for listening and sticking with us during these difficult times. We’ll be taking a little break, and we’ll be back in the fall with new episodes on all things tech and society. If there are any topics you’d like to hear covered in future seasons, we’d love to hear from you. You can email us at consequential@cmu.edu. If you’ve liked what you heard this season, make sure to subscribe so you get our updates and new season when it comes out, and leave us a rating on iTunes.
Lauren Prastien: Until then, this was Consequential. We’ll see you in the fall.
Eugene Leventhal: Consequential was recorded at the Block Center for Technology and Society at Carnegie Mellon University. The Block Center was established to examine the societal consequences of technological change and create meaningful plans of action. To learn more about Consequential, the Block Center and our faculty, you can check out our website at cmu.edu/block-center or follow us on Twitter @CMUBlockCenter, all one word.
This episode of Consequential was written by Lauren Prastien and was produced by Eugene Leventhal. Our executive producers are Shryansh Mehta, Scott Andes, and Jon Nehlsen. The music you’ve heard throughout this episode was produced by Fin Hagerty-Hammond.
This episode uses clips from ABC News, CBS Evening News with Walter Cronkite, and the trailer for the 1979 film The China Syndrome.
It references the article “What constitutes 'essential businesses'? States seem to have varying standards” in CNN Business, as well as data from the US Census Bureau’s 2013 report “County-to-County Commuting Flows: 2006-10."
We also used the definition of system of systems problems as written by the Potomac Institute for Policy Studies.
Consequential Season 3 Trailer
Eugene Leventhal: You know the saying: knowledge is power.
Lauren Prastien: And yeah, there’s power in knowing things. But there’s also power in deciding who else gets to know things, where that information lives, and what people have to do in order to get it.
Eugene Leventhal: Just like there’s power in deciding who gets to add to our knowledge base, what counts as a fact, and whose opinions are worth disseminating.
Lauren Prastien: But, through new innovations in tech and the expansion of global information infrastructures, those power dynamics might be changing.
Eugene Leventhal: The ways we generate, share and apply knowledge stand to get a lot better.
Katie Willingham: Information is never just information. So the more people have a hand in creating this material, in theory, the better that is.
Lauren Prastien: Or, they might be getting a lot worse.
Kathleen Carley: If you can get a group to rally around an issue, then you can start spreading the disinformation into it.
Eugene Leventhal: This season of Consequential, we’re looking at knowledge production in the age of information.
Lauren Prastien: We’ll be discussing subjects like bias in data acquisition:
Arnelle Etienne: If your tool for acquiring the data already has bias in it, then how can you truly say that your data is generalizable?
Eugene Leventhal: And how that bias will impact the technology that is then developed based on that data.
Amanda Levendowski: it reveals a bias toward whose narratives are important and whose narratives end up becoming training data for these algorithms that can reinforce those same hierarchies and bias.
Lauren Prastien: We’ll look at how knowledge production and the future of AI bring` up really important issues related to labor:
Jeff Bigham: We're training these machine learning algorithms on data collected by people who are low-paid and don't benefit over the long term for their labor.
Eugene Leventhal: And representation.
Laura Dabbish: According to the Bureau of labor statistics, 26% of software developers, um, are women, um, and less than 5% are, um, black. So then leads to this even worse representation in open source
Lauren Prastien: All coming this season on Consequential. From the Block Center for Technology and Society at Carnegie Mellon University, I’m Lauren Prastien,
Eugene Leventhal: And I’m Eugene Leventhal. We’ll see you soon` for Season 3, starting October 21st
S3 E1: Knowledge production and the bias pipeline: The story of the EEG
Lauren Prastien: Hey everybody. It’s Lauren
Eugene Leventhal: And Eugene
Lauren Prastien: And we are so excited to be back for Season 3 of Consequential.
Eugene Leventhal: Today, we’re kicking off our season on the topic of knowledge production in the information age by exploring some of the issues that can arise when technologies are developed with underlying biases.
Lauren Prastien: Specifically, we’re looking at the story of the EEG, a nearly century-old technology whose built-in biases have major ramifications for Black people and other individuals with what researchers call coarse and curly hair, and how these biases have impacted healthcare, medical technology, and scientific research.
Eugene Leventhal: So stick around.
Lauren Prastien: Last winter, Eugene and I had the opportunity to talk to Ben Amaba. He’s the Chief Technology Officer of Industrial Manufacturing at IBM. And he told us about this really amazing, really ambitious mission he has:
Ben Amaba: We've got to be able to educate the public and what I call democratize AI in order for it to be really effective on a societal level. Over 70% of the Fortune 500 companies have done some kind of artificial intelligence or data sciences, but very, very few of them have gotten it from a basic research or science project to actually infuse it or synthesize it into our everyday lives.
Lauren Prastien: Keep in mind, I’m not a historian. But there is a lot of historical precedence for the idea of new technologies getting democratized like this. Or, essentially, starting with a fairly small, fairly elite, usually very technically literate group, and then eventually, becoming more widely available to businesses, organizations, and the general public. Like how the Internet was initially just something the Department of Defense used for time-sharing computers, and then, eventually, was something that CERN and the NSF used for collaborating on research and education networking, and then eventually became a set of constituent networks over which everyday people could do everything from talk to friends, talk to strangers, buy things, sell things, start blogs, find recipes, and share pictures of cats. And that’s because of something called cloud computing.
Ben Amaba: So cloud computing really came about, right? Because of the capital cost of owning a mainframe, right? Just the power. So it went from a capital cost to a variable cost and that allowed other businesses to share the economies of scale. So it became a utility cost. I actually paid for what I got. I didn't have to buy this 1-million-dollar structure and only use 80% of its capacity. So cloud technology allowed us to democratize the computing power.
Lauren Prastien: And in addition to democratizing technology just being a good thing, it also usually makes good business sense.
Ben Amaba: We can't create these new technologies as an elitist group. That's why the smartphone got so popular. If you recall, IBM actually had a smartphone, I believe in 1998 it was called Simon, but very few people remember it. Because what it didn't meet, it didn't meet Metcalf's law. That means if the three of us today had a phone, the value of that phone and that network is much, much less than if we added a hundred, two hundred, three hundred people. So first we've got to realize that not to democratize AI is going to lower the value. We've got to understand that and that all technologies go through this metamorphosis.
Lauren Prastien: Here’s the thing, though. If there’s a problem with democratized cloud computing, the worst thing that happens is that you can’t get on the Internet. And if there’s a problem with democratized smartphones, then sorry, you might have to use a landline. But, if there’s a problem with democratized AI, things get a lot hairier. Because that AI might be what you’re relying on in order to get a loan. Or it might be what’s determining whether or not you get a job. Or whether or not the streets in your neighborhood are getting their potholes filled. The stakes are so much higher, but so is the potential. The potential for a fairer loan decision not based on what you look like or what your last name sounds like. Or a faster, fairer hiring process. Or more equitable investments in infrastructure. But just as there’s a great potential for success, but there’s also a great potential for abuse. Which is something that Amaba also really emphasized during our conversation:
Ben Amaba: You've got to show people how to use it in a disciplined approach. Just because it's there doesn't mean it's going to be used in the way it was intended to be used. Although you can improve it, you can improve it and you can improve it, if a platform is incorrect and the way you're dealing with that is wrong, people will not embrace the AI. Or you know, go down to the data collection. If you're only collecting pressure and not collecting temperature, there might be a correlation that you just missed or even a causation.
Lauren Prastien: And this brings up a really strong point. When I talked about those issues of abuse, I’m talking about just one aspect of this. But like Amaba was saying here, there’s also the platform itself that could have a problem. Or a problem in how that data was interpreted. Or a problem in how that data was collected in the first place.
Which brings me to the focus of this season. If we want to democratize AI, we probably have to democratize data. And if we want to democratize data, or information for that matter, we have to start fundamentally rethinking a lot of the ways that we collect, interpret and share knowledge. So this season of Consequential, we’re looking at knowledge production in the Information Age. We’ll talk about everything from research practices to data interpretation to who’s in the room when the technology itself is developed. And to that end, tech is actually going to play a big role in this season, because we’ll be looking at how technologies like AI and machine learning are improving these processes while also, perhaps, sometimes making things a little worse.
To start us off this week, we’ll be looking at a case that really embodies a lot of these ideas and concerns, and that’s the story of the electroencephalogram, or EEG. For nearly one hundred years, the EEG has been the gold standard of clinical decision-making for epilepsy, stroke, and other neurological conditions, because it’s relatively inexpensive and totally noninvasive. But just a few years ago, a team of researchers at Carnegie Mellon published a paper showing that conventional EEGs are less effective for patients with coarse and curly hair, which is common for individuals of African descent. This has massive ramifications for not only how doctors treat patients with coarse and curly hair, but also the design of other healthcare technologies, as well as how we understand the nature of these neurological conditions themselves.
Arnelle Etienne: If your tool for acquiring the data already has bias in it, then how can you truly say that your data is generalizable?
Lauren Prastien: From the Block Center for Technology and Society at Carnegie Mellon University, this is Consequential. I’m Lauren Prastien,
Eugene Leventhal: And I’m Eugene Leventhal.
Lauren Prastien: Before we get into the story of the EEG, I want to back up for a second and give you a framework that we’ll be referring to a lot this season. It’s a set of four components that comprise knowledge production and tech development. A pipeline, if you will.
The first is the world, which is, you know, where we live and where data comes from. The second is the data itself - who was it collected from, where did you get it, and like Ben Amaba said, what might it be leaving out. The third is the finding or technology developed based on that data, including how it got made, who made it, why they made it, and who can use it. And our fourth and final component is the implementation of that technology or finding: is it being used for its intended purpose? What are the stakes of the context in which it’s being implemented? So, for instance, the technology of facial recognition has a wide variety of implementations, and those implementations have very different stakes. The facial recognition that you might use to unlock your smartphone is a little different from the implementation of facial recognition as a major component of criminal justice. Not only is it a functionally different form of facial recognition, but there are a lot of ways that the latter can go really, really wrong with much higher stakes, or be used in a way to reinforce already troubling aspects of our world.
And this is kind of the scary part, because after implementation, that’s when we loop back around to the world. Because this is a cycle. Because the world has changed, however incrementally, because of the use of that technology or finding. Or, some aspect of the world that wasn’t necessarily objective or fair has been deemed objective or fair because of a very unobjective and unfair piece of technology. And then that makes new data, which makes new technology, which gets implemented, and the cycle continues.
Which brings us to the story of the EEG. This story begins in the 20s. The 1920s. Actually, no. Wait a minute. Rewind. It begins earlier. It’s 1890s. A German university dropout named Hans Berger is serving in the army when he falls off his horse during training. His sister, who is miles away, sends an urgent telegram based on a bad premonition, and because of that, Berger becomes obsessed with the idea of telepathy. So, he goes back to school to become a psychiatrist. And, now it’s the 20s. Again, the 1920s. Berger wants to know if there’s a physiological basis for telepathy, and he finds something, but not what he’s looking for.
He discovers that if he uses silver foil electrodes, a capillary electrometer and a galvanometer, which is a tool for measuring electrical currents, he can detect and record the signals being transmitted between neurons in the brain. Except this was normal brain activity, not psychic phenomena. Berger lives in a world where the research subjects he has access to - while varying across age and gender - are white. In fact, two of them are his children. And so the data he produces to show that he can read those waves leads to development of a new device, an electroencephalogram, or EEG. That EEG is then implemented as a research tool, and it becomes part of the world. Later, Berger uses it to gain data on everything from brain tumors to epilepsy, a seizure disorder. And based on that data, he can describe those conditions, which is in turn implemented as a clinical standard for helping to diagnose them.
And now fast forward nearly one hundred years.
Pulkit Grover: EEG is the most widely used neural sensing technology. It's very well accepted in the clinic. There are many new technologies that sort of are making their way slowly into the clinic. But EEG the gold standard for epilepsy diagnosis. It's used in diagnosis today for stroke brain injuries, coma, many, many, many disorders.
Lauren Prastien: That is Pulkit Grover. He’s an associate professor of electrical, computer, and biomedical engineering at Carnegie Mellon’s Neuroscience Institute. He’s an expert in neural sensing and neural stimulation, especially in clinical settings, so a lot of his work looks at EEG technology.
Pulkit Grover: And what is amazing about EEG is that you can listen into neurons that are talking to each other through these electrical impulses, by just putting electrodes on your scalp. No invasiveness, no breaking of skin required.
Lauren Prastien: In case you’ve never seen an EEG before, I asked Shawn Kelly, an electrical and biomedical engineer and senior systems scientist here at Carnegie Mellon who works on developing new EEG technologies, to quickly describe what it looks like and how it works:
Shawn Kelly: So an EEG works by amplifying and filtering signals from the scalp noninvasively. Classically a technician puts small electrodes onto the scalp between someone's hair. Those electrodes have long wires that connect to a box that has amplifiers filters, digitizers, and the information is then shown on a screen, a computer screen of some sort.
Lauren Prastien: Which is kind of incredible, that essentially, just by sticking some electrodes to a person’s head, we can eavesdrop on those electrical signals, which helps researchers study how the brain works and helps doctors diagnose patients. All without cutting someone’s head open.
Shawn Kelly: Some of the alternatives to EEG that may give signals that are slightly better in one way or another are to cut into someone's scalp and put electrodes directly on the brain. And this is actually done in certain cases, but it's very invasive and expensive. Or you can get some information by MRI machine, but that is a very, very expensive technology and it doesn't have the temporal resolution that EEG does. So it's better in some ways, worse in others, and much, much more expensive.
Lauren Prastien: And according to Grover, EEG isn’t just used for diagnosis.
Pulkit Grover: ESG is one of the ways when they make decisions in epilepsy on how to treat the patient. And so it is one of the classic technologies that is used to make every clinical decision in epilepsy.
Lauren Prastien: Every clinical decision. Which is pretty convenient, as far as just using this one piece of equipment goes, until you consider this really glaring problem:
Arnelle Etienne: So EEG is not compatible with, um, all hair types just because when it was created in 1924, the people that they were using it on were pretty homogenous. So it was all straight hair and it worked better for shorter hair.
Lauren Prastien: That is Arnelle Etienne. She is a recent graduate from Carnegie Mellon, where she studied Technology and Humanistic Studies, which is a major she created herself. Back when she was a summer research assistant in Pulkit Grover’s lab, she noticed something troubling with the high-density EEGs that she was working with:
Arnelle Etienne: When you have curlier hair, it can kind of push up against electrodes because they're pretty much being pasted or suctioned or taped onto your scalp. And so if that is not tight enough, it can slide. And also, with caps. For higher density caps, which are used often in neurological research studies, the hair underneath the cap doesn't sit well. Like the cap doesn't have the access to the scalp that it would. So you can try to work around it using like sticks to kind of push around the hair to make sure that you have a good contact, but oftentimes you'll have to stop a recording and readjust electrodes, which takes extra time. And time is very precious often in the types of situations that you use EEG for.
Lauren Prastien: At first, I was really shocked to think that something like this could go on for almost one hundred years. Until I considered that first component that I discussed earlier in this episode: the world. And the fact is that the world - and the things in our world - are really designed with only certain people in mind, and those are almost always the people in power or whoever was at the table or in the room. It’s why it took until the 1990s for companies to develop cameras capable of achieving the dynamic balance necessary to properly photograph darker skin tones and until 2003 for the National Highway Traffic Safety Administration to introduce a female crash test dummy.
The point is that it wasn’t really that the field wasn’t aware of the problem, as much as that there really wasn’t a universal acknowledgment of that problem or a standardized solution. Both of which are major aspects of Kelly, Etienne and Grover’s work.
Shawn Kelly: While some of the clinicians we've spoken with fully recognize that this has been a problem, there have been some others who didn't seem to think it was very difficult or didn't seem to think it was a problem. And I don't know if they haven't had as much experience or maybe the technician takes care of the problem and the clinician doesn't see it, but we've seen mixed responses from clinicians about this issue. So some of them may not be aware of it.
Arnelle Etienne: I actually had an EEG experience in Virginia in a hospital. And my specific technician actually did a pretty good job at applying electrodes in my head, but the area that we were in had a very large black population. So I feel like she had experience with her own work arounds. And so the fact that it depends on a technician and their experience in daily life to determine whether they can address all the populations that they serve is kind of interesting. And I think it adds to the systemic problem that exists, that there is no direct training that just addresses it across the board.
Lauren Prastien: I then asked Etienne if that meant that having some degree of cultural sensitivity was also important in addition to having technical proficiency.
It's one of those things where cultural competency really helps. Access to participants who have a coarse and curly hair, African American, black participants, helps to illuminate the problem because people that we've talked to who have those participants in their research studies and their clinics have noticed that it's a problem. But I think just collaborating with people who understand that hair texture and the culture and the ways of like manipulating the hair really helps to create solutions because other researchers that I've talked to who are black also just immediately understood like how to fix that problem.
Lauren Prastien: And that kind of variability means that if you have coarse and curly hair, there is a chance that your clinician might know exactly what to do or a chance that they have no idea that this is a problem in the first place. Because, right now, there really isn’t any standardization of these practices.
Pulkit Grover: I think it makes a big difference when you have a publication out there that shows that statistical differences. And I think it's important to do your rigor in sort of publishing something and showing something is important rather than just sort of talking about it.
And I think that's the part where engineering and science and rigor can help by examining different solutions, proposing new solutions and comparing them rigorously on participants.
Lauren Prastien: But even with these workarounds, the fact remains that EEG technology itself does still have this fundamental flaw inherent to the design of the electrodes. So, Etienne came up with a new design, for a set of electrode clips that would fit in between cornrows, braids that are made very close to the scalp. And not only would these clips fit between the cornrows, but they would use the cornrows as a guide for their placement. Which literally came to Etienne in a dream.
Arnelle Etienne: So we looked at how the 10/20 system, which is the internationally standardized way of applying electrodes in specific locations on the scalp. We looked at how that lays across the scalp and it mirrored a style of braiding called cornrowing. So when you cornrow the scalp, you have consistent and very long lasting strips of exposed scalp, essentially that are like secured. And then you can use the electrodes, which are inspired by hair clips to secure them underneath the braids. And so, we were looking at those solutions, we have other solutions coming out and it's just looking at the properties of the hair and understanding that that's also a scientific element that you should be analyzing. So I think that's why our innovation works so well as compared to other systems.
Lauren Prastien: When we asked Etienne if she thought that her own experience was important to the development of this technology, she raised the point that people with coarse and curly hair weren’t the only people not being served by traditional EEG technology, which is something that her own experience made her sensitive to:
Arnelle Etienne: People have bald heads also face difficulties. And I wouldn't have thought about that. So that's why discussion and collaboration is so important to developing the technology.
Lauren Prastien: Which Grover agrees is pretty central to solving problems like these:
Pulkit Grover: I think solutions to societal problems are developed when people who examine and understand the problem come together with people who can solve the problem.
Lauren Prastien: Having a solution like Etienne’s new electrodes does a world of good in ensuring that anyone who needs an EEG gets reliable, accurate results, regardless of their race or the texture of their hair. But unfortunately, that doesn’t mean that the field of neuroscience is completely fixed, everything is equitable, and everyone can go home. Because there’s still the matter of all the data that was collected for the nearly one hundred years using EEGs, which is now being used for developing new technologies and for understanding the nature of certain diseases and disorders. We’ll talk about that in a second.
[break]
Lauren Prastien: Like I’ve said a few times now, the EEG has been around for just under one hundred years. It’s a landmark, foundational medical technology. So, according to Kelly, there’s a real ripple effect that comes from not acknowledging such a fundamental problem with it:
Shawn Kelly: When clinicians are using the devices they have and either consciously or unconsciously excluding a population, there's this perception that those devices work fine. And so future designs are based on past designs of the devices, and there's a propagation of this Inappropriate design for certain hair types in iterative generations of these EEG systems.
Lauren Prastien: So part of the solution to that is just having access to the new technology in the first place.
Shawn Kelly: We plan to send samples to different research labs, to different clinical environments and have people try them out. And if they find it's just much easier to use these EEGs on African American hair because of these clips, then we feel that just naturally there will be more EEG is done on people with coarse, curly hair. And there will be more representative data.
Lauren Prastien: Good data is really important. It’s that second component we talked about earlier, and it’s part of what convinces clinicians to use one EEG technology over another. Because, think about it: if you can show me that one technology is producing readings that are less noisy than another one, I’m going to pick the better one. Maybe. Right?
Arnelle Etienne: Funny enough, I'd say we've had a mixed response. An overwhelmingly supportive response, but I think that there's some people who have to be explained like whether this is a nice to have versus a need.
Lauren Prastien: This isn’t just a matter of convenience or comfort for patients, although that’s also really valuable. But on top of that, it’s important to remember that the EEG isn’t just a diagnostic tool. It’s also a tool for research. As in, gathering data to build knowledge about the human brain and the conditions that can affect it. Which, according to Etienne, doesn’t work when the data is biased.
Arnelle Etienne: One of the things that always comes to my mind is generalization of data, because if your tool for acquiring the data already has bias in it, then how can you truly say that your data is generalizable? I think the impact that we have for the future is that people are thinking about how can I reduce the implicit biases and my tools for whatever reason, so that I can acquire data that is truly generalizable and hasn't been based on a specific group
Lauren Prastien: Before we talk about the resolution, it’s important to quickly talk about how this bias arises. Here’s Grover again:
Pulkit Grover: There is bias that arises because you acquire data on coarse and curly haired participants, and it is poorer quality because the electrodes did not have good contact. And so you might end up throwing away this data. This does happen. This is a common phenomenon. That data needs to be discarded because it's poorer quality and the person discarding the data may not be the person who acquired the data. So they may not even know that this is because of the hair.
Pulkit Grover: Another factor that introduces the bias is that there are participants with coarse and curly hair who are denied participation in a neuroscience EEG experiment because of their hair type. We are aware of this through discussions with multiple labs that this has happened. and once Arnelle’s paper came out, the response from the community on Twitter and social media was that, yes, we deny entry to participants because our systems don’t work for participants with coarse and curly hair.
Lauren Prastien: I asked Grover if we know what kind of impact that this kind of data gap has had on the field more generally, and the answer more or less that it’s complicated.
Pulkit Grover: Whether it leads to lasting neuroscientific impact is something that remains to be studied, but it is well known that for many disorders, there are correlations between race and the signals that you would measure. This happens because of sociological factors.
So a disease like schizophrenia, talking about a collaborator, Sarah Haigh, who studies that disorder, can have multiple causes. And one of the correlates it has is low socioeconomic status. And so if we are not able to record on a certain section of the population to understand schizophrenia, we might be limiting our understanding for causes and hence treatments for schizophrenia as well.
Lauren Prastien: It’s also important to note that African-Africans are actually more likely to be misdiagnosed with schizophrenia, as well as major depression and other mood disorders. Again, misdiagnosed, as in they do not have it, but they are diagnosed with it. But this is a lot more complicated than just a matter of what’s coming up on a EEG scan, particularly when it comes to this country’s really complex history of weaponizing schizophrenia and other diagnoses against Black Americans during the Civil Rights Movement. It’s too capacious a topic for me to cover on this podcast. But what you need to know is this: when this data is missing from an entire portion of the population or is underrepresenting an entire portion of the population, that’s a problem when it comes to holistically and accurately figuring out what the pathology and treatment of these conditions actually is.
Which begs the question, how do you fix that? How do you go from years and years of data and mistakes and people being left out and find a way to make things right? Before the cycle continues, and this fourth component folds back into the first, and the world is indeed not changed for the better. According to Grover, a lot of it has to do with shifting the perspectives, priorities, and sensitivities of the humans involved, rather than the technology itself.
Pulkit Grover: I think there is a lot of understanding that is now developed, which is dedicated to understanding whether the algorithms that people are using to analyze the data and make predictions have biases in them. That's great. I really love that direction of research, but it has, it's sort of not that that research hasn't influenced the community of device designers quite as much. And I think there are other systems out there that are being used for important applications, including healthcare. Where such biases have crept in because of implicit assumptions of the inventors and the designers and the people they are imagining who they're catering to. And that can lead to really influential and impactful problems.
I think one, one outcome that is a less tangible, nevertheless, potentially even more important than what we are accomplishing is for engineers and device designers to go back to their drawing board or to go back to their experiments and testing. And for them to sort of try to observe whether they have biases that exist implicitly because of the systems they're using to acquire the data.
Lauren Prastien: At the end of our conversation, I asked Etienne what she hoped would come of this work, and her answer went well beyond the EEG.
Arnelle Etienne: In the future, introducing the idea that you think beyond yourself when you design. And I think that's definitely taught, but I think we're in a space where we're as a society. I think just expanding what that means and expanding who we include, whether it's thinking about the disabled community, the differences between cultures and races and what ways that that can be brought up. I just think that's going to be an exciting future for science.
Lauren Prastien: Here’s the thing: Identifying this problem inherent to EEGs and proposing a new solution is unfortunately just the first step of this journey. But what’s encouraging is that, yes, this is a journey worth taking. And it’s going to be really hard. But when we talk about the future of science and technology and that thing we like to call AI, it is worthwhile. Over this season, we’re going to keep trying to tease out these issues in the pipeline, and tackle some of these difficult questions about how knowledge gets produced, shared and applied.
Eugene Leventhal: This season we’ll be releasing episodes every other week, with the exception of episode 2, which will come out in one week, on October 28th. Given that it’s an episode on disinformation, we wanted to put it out a week before the election. From there, you can expect to hear from us every other week.
Until then, this was Consequential.
Lauren Prastien: Consequential is produced by the Block Center for Technology and Society at Carnegie Mellon University. The Block Center was established to examine the societal consequences of technological change and create meaningful plans of action. To learn more about Consequential, the Block Center and our faculty, you can check out our website at cmu.edu/block-center or follow us on Twitter @CMUBlockCenter, all one word.
This episode of Consequential was written by Lauren Prastien and was produced by Eugene Leventhal. Our executive producers are Scott Andes, Shryansh Mehta and Jon Nehlsen. The music you’ve heard throughout this episode was produced by Fin Hagerty-Hammond.To learn more about our guests and see a list of sources we referenced for this episode, visit consequentialpodcast.com.
S3 E2: If banning bots won't stop disinformation, what will?
Lauren Prastien: Let’s play a game. It’s called two truths and a lie. I am going to make three statements, but only one of them is the truth. The other two are lies. And you have to guess which is which. Are you ready?
Okay.
One: A Texas morgue worker who fell asleep on the job was actually mistaken for a dead body.
Two: President Trump once ordered the execution of five turkeys previously pardoned by President Obama.
And three: An elderly woman in Ohio trained an army of 65 cats to steal things like valuable cutlery and jewelry from her neighbors, and the cats stole more than $650,000 worth of property before she was caught.
Eugene Leventhal: Woah woah woah. Lauren!
Lauren Prastien: What?
Eugene Leventhal: None of those statements are true. They are all lies.
Lauren Prastien: You’re right. And they’re not just lies. They’re actually some of the most frequently-shared fake news stories of 2017. Meaning that they are all examples of this week’s topic: disinformation. Disinformation may seem really silly and benign when it’s about things like a woman and her army of cats. But disinformation campaigns have been used to do things like undermine elections. Like, in 2016, when viral disinformation campaigns informed voters in North Dakota that if they wanted to vote, they would have to forfeit their out-of-state hunting licenses first, or told Latinx voters that they could now vote via Tweet or text message.
-Recently, the COVID-19 pandemic has been a hotbed for the development and dissemination of disinformation, pertaining to everything from cures and preventative measures - such as campaigns claiming that eating raw garlic and coating your body in sesame oil would protect from the virus - to the origins of the virus itself - as in, campaigns claiming that COVID-19 was developed by the CIA or it was a bioweapon that was accidentally leaked - to how cities and states have responded to it, such as fake news claiming that New York City was under martial law.
And now, mere days away from an election during a global pandemic, we’ve hit the perfect storm. A lot of people say this is one of those unstoppable force, immovable object paradoxes, or an unstoppable force meets another unstoppable force. But the thing is, COVID-19 isn’t an unstoppable force, there are things we can be doing. They just require assuming some personal responsibility, as well as massive, large-scale coordination and intervention. And by the same token, disinformation also isn’t an unstoppable force that we can do nothing about. It’s just the way we’ve currently been going about it hasn’t been entirely correct.
Lauren Prastien: From the Block Center for Technology and Society at Carnegie Mellon University, this is Consequential. I’m Lauren Prastien,
Eugene Leventhal: And I’m Eugene Leventhal. This week, we’re looking at disinformation. What is it, how does it get shared, why is it such a problem, and what can be done to stop it? Though we’re releasing episodes every other week this season, we wanted to release today’s episode ahead of the election given the importance and relevance of the topic.
Lauren Prastien: In order to put together this primer on disinformation, we turned to an expert. Her name is Kathleen Carley. She’s a computer and social scientist at Carnegie Mellon University, where she leads a center called IDeaS, or the Center for Informed Democracy and Social Cybersecurity. And the first thing we wanted to know was, what actually is disinformation, and is it any different from the concept of misinformation.
Kathleen Carley: So typically scientists separate out disinformation or misinformation by focusing on intent. Disinformation is information that you know is false when you spread it. Whereas misinformation is stuff that you don't know is false and you just spread it.
Lauren Prastien: The clips you’ll be hearing from Kathleen Carley throughout this episode are from two places: the first is an interview that Eugene and I had with her, and the second is from a panel discussion on disinformation that the Block Center and IDeaS hosted earlier this month. You’ll be hearing a few other clips from some of the other participants on that panel as well throughout the episode, but I’ll let you know who those are when they come up.
But anyway, what this means is that a piece of information can actually morph from one category to the other. And if you’re on social media, you’ve probably seen this. You have a friend or a relative who is a generally well-intentioned person, who’s then suddenly sharing a piece of information that is patently untrue. And that information was possibly created by a malicious actor as a piece of disinformation, but your friend or your relative thinks it’s news, and is spreading it as a piece of misinformation because they really think it’s true and they’re just trying to get the word out. You may have done it yourself. Because, full disclosure, I definitely have, and some of the most powerful politicians in this country have, either intentionally or unintentionally.
It’s important to know that disinformation isn’t a new problem that came about during the 2016 election. Fake news is as old as the printing press, if not older. In fact, according to the historian Jacob Soll, even our Founding Fathers were guilty of it. In 1782, Benjamin Franklin wanted to influence public opinion on peace negotiations following the Revolutionary War. In particular, he sought to influence Great Britain into paying reparations and, I quote, “make them a little ashamed of themselves.” So he wrote an article about groups of Native Americans sending colonists’ scalps to King George III, along with messages like “Father, We send you herewith many Scalps, that you may see we are not idle Friends.” He put this in a fake edition of the Boston Independent Chronicle. It was patently untrue and understandably really harmful, especially when other newspapers started reprinting the story as fact. Which is, by the way, a little eerily reminiscent of how people still spread disinformation today. Somebody intentionally creates a piece of fake news, people believe it is real, and they pass it along.
So while it’s a leap to say our country was built on fake news, the fact remains that disinformation has always been around. And more specifically, disinformation as a form of political interference isn’t even a new concept. So what’s the difference now?
Here is to explain is Congressman Mike Doyle, the U.S. Representative for Pennsylvania's 18th congressional district and the Chairman of the Subcommittee on Communications and Technology:
Rep. Mike Doyle: I think what differentiated 2016 was the scale on how this was done through the social media. And the amount of foreign interference that took place on our social media sites. Basically, entities were able to take the tools that Facebook and Twitter and Google had to micro-target certain types of voters, and provide them with information that was not accurate. Some of these weren't even real people. And many of it was coming from foreign countries, and it was done on a scale that we've never seen before. And we sort of were asleep at the switch. It’s like nobody really grabbed the handle on it until after it happened.
Lauren Prastien: Because of that scale and the availability of new, powerful technologies, disinformation played a destructive role in the 2016 election. From targeted ads, like the examples I gave you earlier in this episode, to the rise of deepfakes and coordinated armies of bots, we saw unprecedented attacks on the electoral process.
Rep. Mike Doyle: So it got a little bit better in the 2018 election. But we all know 2020’s here, there's going to be more interference. But, the federal government could and should be doing a lot more. Now the companies are stepping up, too, I mean, this has become a big issue for the companies too. And I think that you know, hopefully, that it will be minimized.
Lauren Prastien: It’s important for me to briefly contextualize this. Eugene and I had the opportunity to speak to Congressman Doyle earlier this year. In February, to exact, when we lived in a very different world and we thought we were making a very different season. We’re hoping to share more of our conversation with Congressman Doyle with you in the future.
But anyway, as I’ve mentioned, since our conversation, something happened. Something that has sharpened the precision, increased the quantity and upped the stakes of these disinformation attacks. Yeah, I’m talking about COVID.
To put this in perspective, here’s Kathleen Carley again:
Kathleen Carley: The pandemic itself, it is often thought of as an infodemic or a disinfodemic, because the amount of disinformation that has spread is orders of magnitude higher than we've ever seen before. Some accounts placed the number at over 9,000 different types or bits of disinformation that have been spreading. In contrast to give you a context in most elections and the most natural disasters, the number of disinformation campaigns is on the order of somewhere between seven and maybe a hundred or so, whereas we've got, you know, thousands and thousands going on now.
Lauren Prastien: The COVID-19 pandemic saw an explosion of disinformation campaigns, both pertaining to the virus itself and pertaining to the impact that the virus has had on individuals’ ability to effectively participate in the 2020 election. And this factors into a larger, decade-long trend where disinformation campaigns have gone from a tactic deployed by fringe groups to a comprehensive attack strategy used by state and non-state actors to undermine the legitimacy of elections, public health initiatives and other forms of public life. And as Congressman Doyle said earlier, the reason why these campaigns are now so pronounced and effective is the fact that social media not only gave malicious actors an unprecedented access to an audience, but it provided the tools to specifically tune and target that message. It’s why that whole “you can vote by text message” campaign was able to find its way onto the feeds of Latinx voters. And there has been some definitive progress in minimizing the ways that micro-targeting works.
But it’s not just about targeting the message to a specific audience. It’s also about being able to proliferate that message quickly, consistently, and succinctly. Or, as Carley puts it:
Kathleen Carley: The key problem with disinformation of course, is that it not only spreads, but it is spread and re spread often by bots, trolls, memes and cyborgs.
Lauren Prastien: Bots, trolls, memes and cyborgs. If this sounds a little sci-fi, don’t worry. I’m going to break all of these down for you.
Bots are essentially computer algorithms that are programmed to spread information. And they’re not always bad. Bots are actually a really effective tool when it comes to disseminating information really, really quickly or combing through massive amounts of data to find a trend and get a point across. So, for example, there’s a bot called Rosie that combs through public expenses of members of Brazil’s congress and tweets anytime there’s any kind of troubling or irregular spending, and Rosie has been pretty integral to anti-corruption and social accountability in Brazil. So bots aren’t necessarily bad. But like a lot of technology we talk about on this podcast, its strengths are also its drawbacks.
In 2017, a group of researchers at MIT estimated that between just Twitter, Facebook and Instagram, there were one hundred and ninety million bots disseminating false information, which is more than the half population of the United States. And to give you an idea just how much of a role bots play in discourse today, Carley and her team of researchers at IDeaS recently found that between 45 and 60 percent of Twitter accounts discussing COVID-19 were likely bots. And these bots weren’t just shouting into the abyss. On Twitter, 42 percent of the top 50 most influential mentioners and 82 percent of the top 50 most influential retweeters related to the COVID-19 pandemic were bots. Yeah. Yikes.
So it’s understandable why a lot of the legislation around disinformation and a lot of the discourse, even, focuses on bots. For example, a California law that went into effect last year required that bots identify themselves as bots when they are used to sell a product or influence a voter, as punishable by fines. And while it makes sense to target bot-led amplification of disinformation messages, Carley cautions against just focusing on bots.
Kathleen Carley: Social media at this point is so infected with disinformation, the classic strategies of simply banning a message or banning a bot simply do not have the effect that they used to have in part, because if you ban it on Twitter, it will show up, back up on Facebook. If you bandit on Facebook, you'll show up on YouTube and so on.
Lauren Prastien: Not only is there this sort of hydra effect or hungry-hungry-hippos effect or whatever metaphor you want for the fact that social media is so blighted with disinformation that simply focusing on bots ignores the real problem, the fact is that, yes, you are ignoring the real problem. Which, isn’t really bots at all.
Kathleen Carley: It's people. People are way more likely to broadcast disinformation than they are to rebroadcast true information.
Lauren Prastien: This brings us to our second item on our sci fi list of disinformation perpetrators: trolls. These are people that post content online for the purpose of provocation. Usually for the purpose of amusement. But not all humans who share disinformation are trolls. In fact, a lot of humans don’t realize they’re spreading disinformation when they’re spreading it.
And to that end, a significant amount of the disinformation shared by humans is actually rebroadcasted, as opposed to completely new information. Researchers at IDeaS have actually found that 77 percent of the disinformation spread by people in the U.S. related to the COVID-19 pandemic was rebroadcasted material from other humans in the United States.
But in addition to being bored on the Internet or being deceived by information you believed to be true, there are other, more nefarious reasons people get into spreading disinformation.
Kathleen Carley: Some people at also though do it for money. A spreading disinformation is now a big money marketing thing. And there are actually marketing firms throughout the world and troll farms throughout the world who create and spread disinformation and can be hired to do so. It is also being spread by others to foster particular political agendas, to actually build communities that have a particular way, way of looking at the world. And of course, to disrupt civil society.
Lauren Prastien: According to research from Oxford University, the number of countries targeted by organized disinformation campaigns doubled between 2017 and 2018. And in part, a lot of these firms deploy item number four on our list of the sci fi horsemen of disinformation. Yes, I know we skipped three. We’ll loop back around. I promise. But, that fourth one, which I want to cover for two seconds, is cyborgs. And when I say cyborgs, I don’t mean like Donna Haraway cyborgs or Inspector Gadget. Cyborgs are accounts that are sometimes run by humans and that sometimes function as bots. So, a lot of the content coming out of a cyborg is automated, but sometimes a human will grab the wheel. And these are really dangerous, because they’ll often lend a degree of legitimacy - like, oh, this is a person - while having the ability to achieve the virality and immediacy of a bot.
But you don’t need a sophisticated marketing firm or a coordinated cyborg effort to do real damage with a disinformation campaign. In fact, some of the most effective strategies are a little less formal.
Kathleen Carley: The most insidious types of disinformation are those that began in the form my friend said, or my uncle said that they know someone who, so for example, in the anti-vax campaigns, one of the most insidious disinformation comes in the form my friend did not get her kids vaccinated and they don't have autism. Totally true, totally irrelevant.
Lauren Prastien: Suggestion is a powerful, powerful tool. As is innuendo. Particularly because a traditional filter might struggle to pick up on it. That kind of nuance is something that Yonatan Bisk, a computer scientist at Carnegie Mellon, drew attention to at that panel on disinformation I mentioned earlier:
Yonatan Bisk: There's a very big difference between someone from an ingroup stating a joke and someone from an out-group stating the joke. And so you have to use all of that additional context.
Lauren Prastien: Right now, traditional content moderation, particularly algorithmically driven moderation, struggles with this. It’s why an AI tool once marked drag queens’ Twitter accounts as more toxic than the Twitter accounts operated by white nationalists. Because the tool would see a tweet from the drag queen Mayhem Miller saying “Miss Carla has been my #rideordie b-word since #1996!!! More than just #besties we are #family” and find that more toxic than, say, actual homophobia. The language of the Internet is pretty odd, often vague, and super suggestive. Which brings me to that third sci fi element.
Yeah, I’m talking about memes. If you’re not familiar, a meme is defined as a humorous image, video, or piece of text that is copied, often with slight variations, and spread rapidly by internet users. The term was actually introduced in 1976 by the evolutionary biologist Richard Dawkins, so it’s actually a concept that predates modern Internet culture. And while they often seem really silly or a really central, but benign part, of how people communicate on the Internet, according to Carley, they’re a really powerful tool.
Kathleen Carley: We do know that a lot of political debate is carried through memes that you often get meme families and that you can do subtle changes within memes to add additional information. We also know that a well-constructed meme has the ability to have more impact than a well-constructed story in and of itself.
Lauren Prastien: This is because a meme can elicit a pretty strong, visceral reaction, and it’s super easy to just click share. So, when you see a meme, do me a favor and take a second before you decide to share it. Essentially, I’m asking you to treat that meme like a serious piece of discourse. Because, you know what? It is one.
Kathleen Carley: They're actually used to get groups to rally around issues a lot of times. And so if you can get a group to rally around an issue, then you can start spreading the disinformation into it. The meme has served a purpose because it's created a group. Because a lot of what goes on social media and online is that where it's not just about the stories that are told to the images, it's about building ties between people and creating groups where none existed or breaking those down and memes can be used to do that.
Lauren Prastien: So what do you do when you see a meme that is patently untrue? Or spot a person that is decidedly not a bot, but a friend or a relative or an acquaintance sharing a piece of disinformation? Professor Carley has found a few solutions, thanks to the movie Black Panther.
Kathleen Carley: That was a great study. Um, so what we did here in the Black Panther study is that we'd been doing work on disinformation and we said, look, we need to be able to understand entire timeline from start to finish. You know, how it pops up, who promotes it, how long does it last, when does it die, et cetera. So we thought about, well where could we find a disinformation campaign right from the beginning where we know one will occur because you had a way to predict where it would occur. So, the Black Panther movie, it's going to, it's just going to ring so many bells in America. It's going, somebody is going to put a disinformation campaign around it. And so that's why we did it and where we started. And so we actually collected information from all over, from before the movie till after it came out on it. And we found not just one, but four different disinformation campaigns associated with it.
And the great thing about this is that we've found in that that only one of them lasted a long time. And that was the one where they were focusing on a specific group. In fact they were focusing on the alt-right. Now the fun part of this is not that, the fun part of this was the one of a couple of ones that did not last. And that is we have the first ever record of actual approach that canceled, that stopped the flow of disinformation. And this was because there's this other disinformation story that came out, which basically said, I went to the movie and I got beaten up, or my uncle went to the movie and they got beaten up and they show, you know, white guys at the movie with blood or whatever. Now these were all fake pictures and had one I think had ketchup on their face, but they were meant to be real, but none of them actually occurred in the theaters. So very soon after this came out, a second disinformation story came out that said the same kind of things I went and I got beaten up, whatever. But the images now were different. They were SpongeBob with a bandaid they were to face. There were things like this.
Lauren Prastien: Yeah, sometimes one of the best weapons in your arsenal for this kind of stuff is humor. But...use that at your discretion. There’s also a big advantage to just being direct and to-the-point. Which is something Professor Carley also saw in the reaction to that Black Panther meme.
Kathleen Carley: The other thing that happened is that human beings in a kind of participatory democracy fashion started saying, Hey, this is fake. That's not a movie. You know, I've seen that picture on this other site. And they started calling it out.
So calling things out is also another way. Okay. Um, the one thing that hasn't really worked has been when there's a disinformation campaign that is really heavily embedded in a group and then you tried to just send facts against it and just sending out facts usually does not counter disinformation campaigns.
Lauren Prastien: By taking advantage of the kinds of communities that the Internet can foster, memes - and disinformation campaigns in general - have been able to become incredibly powerful. And this is something that bots can amplify, but like we said, this is also a human problem. So what can you do, and what about our current approach to disinformation needs to change? We’ll get to that in a second.
[break]
Lauren Prastien: Before we get into the larger, more policy-oriented goals, I want to talk about what you can do personally. Carley got into this a little bit with managing memes, but I wanted to know: How do you identify disinformation in the wild, and what can you do about it? It’s a complicated question, especially as disinformation becomes more pervasive and more sophisticated.
Kathleen Carley: An old way of spotting disinformation was simply to say how many sources is this coming from? Okay, check your sources. Today because the way you repeat things in social media and so on, this it may be hard for you to find the original source. First off, is it just a mere mortal looking at the data? But the second thing is multiple sources. You may get things from lots of sources that are all confirming each other even though really they're from a third party that's on the different media bringing it in. So that kind of critical, “oh well are these sources agreeing” is not as good of a heuristic as it used to be.
Lauren Prastien: So, what can you do before you click share?
First - do some mindfulness. Ask yourself: Is this article written in such a way as to intentionally provoke a visceral, emotional reaction? If you’re feeling kinda heated after reading something, which given the world we’re living in is totally understandable, just take a minute to interrogate why you feel that way before you decide to react and disseminate that information.
Second, look at the author’s credentials and the credentials of the people the author quotes. And, more importantly, check to see if the article is even credited to an author or to an editorial board. I’m not asking you to be elitist here, but I am asking you to consider if this person is an expert or has consulted experts on a given subject.
Third, and this may seem like a given, but read the whole article before you share it. Headlines can be misleading.
Fourth, don’t underestimate satire. While satire and humor can be an effective way of combating disinformation, it can also be a way of spreading it. A survey from the Centers for Disease Control and Prevention found that 4% of respondents actually consumed or gargled diluted bleach solutions, soapy water and other disinfectants in an effort to protect themselves from the coronavirus, which was - you know - a satire.
And fifth, if you’re not 100 percent sure about the veracity of something, just don’t share it. Social media recommendation algorithms rely on engagement. Don’t help propagate disinformation by driving traffic toward an unreliable source.
While many of these tactics may seem intuitive or obvious, it is important to be vigilant, particularly in times of crisis.
On a more organizational and policy level, there’s a lot of work to do. Particularly because policy really hasn’t kept pace with these problems. Here’s Nicol Turner Lee, the Director of the Center for Technology Innovation at Brookings, who spoke on this issue on the panel:
Nicol Turner Lee: I'm really concerned the last four years that technology has actually advanced before policy. And as a result, misinformation, disinformation will continue to be unhinged.
Lauren Prastien: A lot of the holdup on this has to do with the fact that there is often partisan disagreement on how to best address disinformation. Progressives often believe regulating social media platforms is the most effective route, while conservatives worry about impeding free speech. These differing views have made political and legislative action difficult. But just like how disinformation itself has made really universal things like preserving public health politicized, the discourse around disinformation has politicized an issue that honestly shouldn’t be partisan. Particularly when it comes to disseminating information that is patently lethal, that should be a criminal issue, not a partisan one.
There’s also a financial issue at hand. A study by the University of Baltimore found that online fake news costs the global economy $78 billion each year, yet most cities and states have virtually no resources explicitly targeted to combating this.
In addition to directly allocating these funds to combating disinformation, there’s also an immense value in funding education for this, to give people the tools to fight this themselves. Here’s Daniel Castro, the director of the Center for Data Innovation and vice president of the Information Technology and Innovation Foundation:
Daniel Castro: Probably the most important thing in this space is increasing digital literacy. Right now, you know, we have some digital literacy that focused on focuses on technical skills. We have some civics education that focuses on understanding how our democratic systems work at work. We really don't have a good blend of digital literacy and media literacy, where we teach people how to consume information online and, and how the question it, and we have seen another countries that have focused on this in the past that that's a very effective technique for was getting people to be responsible consumers of information. So that, again, that human factor isn't, what's accelerating the spread of the misinformation. And it's the one that can also stop the spread when they encounter it online.
Lauren Prastien: But also, some of this is going to be about a much bigger, much hairier conversation that goes well beyond legislative action and digital literacy. Here’s Nicol Turner Lee again:
Nicol Turner Lee: A lot of what we saw in 2016 was based on the historical legacies that were embedded in the truths of this country. People know more about us that we know about ourselves. And that was actually used to sort of glean these vulnerabilities that led to these types of massive surveillance, as well as persuaded people to stay home.
Lauren Prastien: And unfortunately, those little kernels of truth make it easier to tell the kind of falsehoods that can do immense damage.
Nicol Turner Lee: This is the preying upon the historical vulnerabilities and conscience of the United States by actors who sort of know where the blind spots are. And until we actually face that reality, I think we're going to actually see more and more of it because it's going to go, you know, it's beyond did Prince die this year, or did he die last year, too? How do we actually stop people from exercising democratic rights?
Lauren Prastien: This is what’s called a social cybersecurity attack. Because you’re not hacking the machine anymore, you’re hacking people. By going into those communities, exploiting those vulnerabilities, and bombarding people with the kind of disinformation that makes them feel heard or important or vindicated. And taking on those kinds of attacks requires research, resources, and talent. We just have to decide that’s an investment worth making.
Until then, this was Consequential. We’ll see you in two weeks.
Eugene Leventhal: Consequential is produced by the Block Center for Technology and Society at Carnegie Mellon University. The Block Center was established to examine the societal consequences of technological change and create meaningful plans of action. To learn more about Consequential, the Block Center and our faculty, you can check out our website at cmu.edu/block-center or follow us on Twitter @CMUBlockCenter, all one word.
This episode of Consequential was written by Lauren Prastien and was produced by Eugene Leventhal. Our executive producers are Scott Andes, Shryansh Mehta and Jon Nehlsen. The music you’ve heard throughout this episode was produced by Fin Hagerty-Hammond. To learn more about our guests and see the sources we referenced for this episode, visit consequentialpodcast.com.
To watch the entire event on what state and local policymakers can do to combat disinformation, and read a short white paper outlining short term and long term strategies, visit consequentialpodcast.com.
S3 E3: Is Crowdsourcing the Answer to our Data Diversity Problem?
Lauren Prastien: Hey, Eugene. What do the Stanford Prison Experiment, the Marshmallow Experiment, and Milgram Obedience Experiments all have in common?
Eugene Leventhal: Well, they’re all considered really important, really famous social psychology studies.
Lauren Prastien: Keep going.
Eugene Leventhal: They...were all done at elite universities in the 60s and 70s?
Lauren Prastien: Getting warmer.
Eugene Leventhal: Oh! I know! It’s that they’ve all been disproven.
Lauren Prastien: Well, that’s actually complicated. I mean, there have been claims that participants in these studies either knew what was going on or that the sample size wasn’t large enough or diverse enough to be broadly applicable. Which was my point: They were done on basically homogenous groups of wealthy, educated white people.
Eugene Leventhal: Wait a minute. The Marshmallow Study was done at a preschool. You know, they had a bunch of preschool kids see if they could go 15 minutes without eating a marshmallow. And if they could, they got a second marshmallow. And years later, they found that the kids who could just wait for the second marshmallow were more successful. It’s all about delayed gratification, and again, they were in preschool!
Lauren Prastien: Yeah. A preschool on Stanford University’s campus. So who do you think their parents were? When a team of researchers repeated the study on an even larger and more diverse sample of children a few years ago, they found that a child’s ability to wait fifteen minutes for a second marshmallow had a lot more to do with their family’s socioeconomic background than their own sense of willpower and their likelihood of success. And, by extension, they found that children’s long-term success often depends on that background, rather than their own capacity to delay gratification.
Eugene Leventhal: So the answer is just to have the most diverse sample of research subjects possible? Got it. Cool. Good point. Well, this was a short episode. Good work keeping it brief, Lauren. Scott’s going to be thrilled. I’ll see you in two weeks.
Lauren Prastien: Wait! Hold on a second!
[cut music]
Eugene Leventhal: What?
Lauren Prastien: It is so much more complicated than that! How do you get those subjects?
Eugene Leventhal: Easy. Have you heard of the Internet? Crowdsourcing, like Mechanical Turk, maybe? Did you know that in 2015 alone, more than 800 research studies, including medical research, were published using data from Mechanical Turk? And there are other crowdsourcing platforms besides Mechanical Turk. There’s Prolific Academic, StudyResponse, Survey Monkey...
Lauren Prastien: Oh, you want to talk about crowdsourcing research?
Eugene Leventhal: I get the feeling that you do.
Lauren Prastien: Yep! From the Block Center for Technology and Society, this is Consequential. I’m Lauren Prastien
Eugene Leventhal: And I’m Eugene Leventhal. Today, we’re talking about how the relationship between those running studies and those partaking in them has evolved, especially as crowdsourcing platforms have become more common place. Stay with us
[break]
Lauren Prastien: Obtaining research subjects has always been a complicated task. To learn about how researchers went about finding their subjects and how that’s changed, we checked back in with David S. Jones, who you may remember is a psychiatrist and historian at Harvard University, whose work looks at the history of medicine and medical ethics.
David S. Jones: I think if you were to look at the types of populations that researchers have been using back to ancient times, then especially back to the 19th century, when the scale of human subjects research increases, just about every research population that was used would now be considered unacceptable, with a few caveats.
Lauren Prastien: According to Jones, usually, this basically boiled down to who the researchers had access to and who they could exert power over.
David S. Jones: So a lot of physician researchers did experiments on their own children. In the United States, where some researchers had access to enslaved populations, there was a tremendous amount of research that was done by white physicians on enslaved Africans and their descendants. In the late 19th century as bacteriology moves into its heyday, a lot of the researchers started to work with various institutionalized populations. Again, that might be prison inmates, but it was often children who were stuck in orphanages or otherwise wards of the state. And the researchers felt like as long as you got consent from the superintendent of the orphanage, then it was open season to use these children in the service of medical research or human betterment or whatever you hoped would happen.
Lauren Prastien: So I asked Jones how exactly college students came into the mix.
David S. Jones: As far as I know, people haven't yet written a full and adequate history of how it is that undergraduates came to be such a mainstay, not just to the psychology research that you mentioned, but also various kinds of medical research. I assume it was a gradual move in the early 20th century, you would see a lot of researchers doing research on themselves. Self-experimentation had been seen as the heroic ethical gold standard in the late 19th into the 20th century. And you would find researchers, as I said earlier, experimenting on their children. I have accounts of researchers doing experiments on their wives, on their secretaries, on office staff. And they had a lot of this was whether it was, these were people the researchers had control over, or whether it was people who the researchers had access to just because they were convenient. They were working in the office, and the researchers thought this was such benign research, that there was no concern. It wasn't unreasonable to ask your wife to drink some radioactive iron because you thought it was totally harmless for her. And so this kind of thing was happening a lot in the mid-century. So I think it was a relatively easy move. I would suspect it happened first with psychology, but certainly it’s happening in other fields after World War II, to say, look, you have all of these students who are available, who are eager, who are enthusiastic. And there was a rapid growth of the university system in the United States after World War II, many of them are going to become researchers. What a great way to become a researcher than to be trained on the experience of being what a research subject is like. And so you could make the claim that it was pedagogically important for these people to participate in research. And then they would subsequently in their careers go on to design and implement this research.
Lauren Prastien: And at first glance, this seems like a much better idea! Everybody benefits, right? Nobody gets exploited or hurt or forced to drink radioactive iron because it was your wifely duty to do so. So, great.
David S. Jones: What you do have is the problem that you alluded to earlier. You're sampling a very small subset of the population. I imagine that a lot of the studies that pass as conventional wisdom in psychology are studies of white relatively well-off 18 to 22 year old, mostly men, probably some women mixed in, who come from a certain kind of educational background. And they might not actually reflect everything there is to know about human psychology. And there are a variety of ways in which this could be very limiting student or subject population for this kind of research. And I think people are just beginning to take seriously, the kind of problem that has come up.
Lauren Prastien: As an undergrad, I signed up for a lot of psychology department studies. Usually I would sit in a room and take a survey, and then I would get paid about 8 to 10 dollars for an hour of my time. Or I would have to watch a video and let a machine track where my eyes moved. Or I would play a game, and then answer questions about that game. There’s some metal in my mouth, so unfortunately, I couldn’t do any of the MRI studies, which were the ones that paid the big bucks.
But take it from me: it’s not a great idea to make conclusions on how human cognition or moral reasoning works based on a bunch of undergrads at elite institutions. And literally everyone we spoke to for this episode reiterated this exact point. But it was more or less who these researchers had access to. Even if researchers try to go beyond explicitly recruiting undergrads, the communities that these universities are based are still pretty homogenous for the most part. And you also have to consider: who has the time to do a study, the access to a university’s campus, or even the information about a study taking place.
It’s something that Jones noticed in his own community, where depending on which subway line you took, you’d see ads for studies at places like Harvard and Massachusetts General Hospital.
David S. Jones: If you ride the various subway lines in Boston, you notice that one of them, the Red Line advertises aggressively for research participation and the Green Line, the Blue Line, and the Orange Line do not.
Lauren Prastien: Quick clarifying note: the Red Line runs between a lot of very white neighborhoods in Boston, Cambridge and Somerville. But this isn’t because Harvard and Massachusetts General Hospital are explicitly looking for white research subjects.
David S. Jones: MGH will say, well, we're on the Red Line, so of course that's where we advertise, because we want to make sure that people can get to the hospital easily. And they also know that Harvard is on the Red Line. So if you advertise with the Red Line, you get Harvard undergraduates.
Lauren Prastien: So, geography and access to subjects are really critical problems when it comes to having a diversity of perspectives for research. And like we talked about in our first episode with the EEG, not having diversity in your dataset has serious implications down the line, often some that you didn’t even expect or account for.
But, like Eugene said earlier, we have a little something called the Internet. And it is, for the most part, a democratized technology. But, the Internet is also vast. You can’t just show up on the Internet and bam, research subjects. You need an infrastructure to support that.
And that’s where Mechanical Turk comes into play. Here’s Ilka Gleibs. She’s a social and organizational psychologist at the London School of Economics, where she serves as the deputy head of their ethics committee.
Ilka Gleibs: One of the most used crowdsourcing platforms is Amazon's Mechanical Turk. And the idea of that is that there are some jobs that can be easily done by people that are more difficult for machines. So it's in a way artificial artificial intelligence.
Lauren Prastien: Some quick history. Mechanical Turk was first created by Amazon in 2005 as, like Gleibs said, a kind of artificial artificial intelligence. The name is from a chess-playing device first commissioned by the Austrian Empress Maria Theresa in the 18th century, where essentially people thought they were playing chess against an automated opponent, known as the Turk. But the Turk wasn’t actually a robot. There was a person inside the machine, turning the gears and making the moves.
Some of the typical jobs you might find on Mechanical Turk are things like removing redundant entries from a dataset, taking a survey, writing a short description of a video, or image tagging, which is when you identify the content of a photo. These are tasks that are relatively simple, and like Gleibs said, they’re actually a lot easier for a human to do rather than a machine.
Ilka Gleibs: So the idea was that these platforms were kind of created to bring together people who have these kinds of tasks and people who are looking to do the tasks, right? And then researchers and especially social scientists thought, well, we often do experiments or studies and we look for people who would fill out my survey. I could post that as a job on these crowd sourcing platforms and then people can answer my survey or my experiment for a little bit of money. And so it was, yeah, that's kind of the history of how that developed from a platform where people can outsource some easy to do jobs to one where people post experiments or surveys that people then fill in.
Lauren Prastien: Amazon’s Mechanical Turk -- or, MTurk, as it’s colloquially called -- isn’t the only platform like this. There’s actually tons of them. There’s Clickworker, Microworkers, and CloudFlower, just to name a few. And there’s even platforms like MTurk that are specifically designed for research, like Prolific Academic and StudyResponse. But MTurk is going to essentially be shorthand for this kind of online crowdsourced research because it’s essentially the Xerox of online crowdsourced research. As in, it’s the private company that has become so ubiquitous that its name is basically synonymous with the practice.
Anyway, according to Gleibs, MTurk and platforms like it overcome a lot of the problems we talked about earlier on in this episode.
Ilka Gleibs: So in psychology and in, especially social psychology in the last, let's say almost 10 years now, there was a huge acknowledgement that most of our research was completely underpowered. And it was underpowered because we did these very time intensive and labor intensive lab experiments, where we might be able to get a hundred participants in, let's say a few months. Whereas when you post an experiment on MTurk, you can have a thousand participants within days.
Lauren Prastien: Again, it’s the Internet, so these participants don’t have to be people from your community. And especially in the era of COVID-19, being able to conduct research online is pretty clutch. It means you don’t have to halt your study just because you can no longer meet in person or occupy a lab space.
But, there are a few pretty big tradeoffs to no longer having this research done in person. According to Gleibs, being in the same physical space as your research subject is sometimes pretty important for protecting your subjects and ensuring the efficacy of your study.
Ilka Gleibs: We were in touch with these people, we could see how they react. There could also be a moment where you could interact and interfere if someone got upset, for example, or if you realize that someone you had an experiment and no one really understood what you were doing. And so they might not have done it in the way you intended it, you would actually realize quite early on when people come back to you and say, “well, I don't know what I just did.” So I think that was much more in a way control over the situation and interaction and connection to the participants.
Lauren Prastien: One time in undergrad, I did this study in an eye tracking laboratory where I essentially had to have my head strapped into a machine and have my eye movements tracked while I watched a short cartoon. And at one point the researcher came back over to me and was like, “hey, are you ok? You keep looking at the door.” And I was like, “well, actually, I am extremely nervous because my head is essentially trapped in a vice.” So, we took my head out of the vice, I took a second to calm down and talk to the researcher and build a sense of trust and rapport, and then we were able to put my head back in the machine and do the experiment.
If that experiment was conducted remotely, and with advances in AR and VR that might one day be the case, that probably wouldn’t have happened.
Ilka Gleibs: So there's a real human connection, but if you post something on MTurk you don't know any of the people, you don't have a face, they don't have a…they just have a number and you might see them as just…in a way you might see them as a form of artificial intelligence. Just a service, but it's not a service. These are people as well.
Lauren Prastien: The dehumanization of research subjects on a platform like MTurk doesn’t necessarily mean that the researchers are abusing subjects in the ways we saw in, say, the troubling social psychology experiments of the 1970s. Often, it’s manifested in more sort of utilitarian ways that turn the research process into a client-contractor relationship, rather than a researcher-subject relationship.
Ilka Gleibs: As the requester on MTurk, so the person who posts the job, you have the right to reject the work of someone because you think they might not have done it the right way. In a way it makes sense because you don't want to pay for a service that was not delivered, but in terms of academic research, it becomes problematic in the sense that we, in terms of ethics framework, we always tell people that they don't have to answer questions if they don't feel comfortable with it. So they might not answer part of our survey, or they might decide not to proceed, but then we don't pay them. I mean, in the MTurk logic, we wouldn't pay them. And so they might feel forced to continue despite the fact that we actually tell them they should not continue if they don't feel comfortable. So I think that's a power relationship that is different to what we did offline, where we always would pay people, even if they did not finish an experiment.
Lauren Prastien: And according to Gleibs, payment is just a huge problem more generally on platforms like these.
Ilka Gleibs: I think we felt as researchers that all, we compensate them fairly for a very easy task without realizing that for some people suggest at least 25% of people working on MTurk, it's a major source of their income, right? So these people are not just doing that because they like to do surveys or experiments. They actually do that because they have to make money. And if that is the case, we should, I think our responsibility as researchers is to treat them like a worker and like someone we want to give a fair wage or fair pay.
Lauren Prastien: In 2016, the Pew Research Center conducted a really comprehensive study on how scholars and companies are using Mechanical Turk to conduct research. And one aspect of this study focused on the demographics of Turkers, or people who perform tasks on Mechanical Turk. And some of the major findings were that about half of Turkers earn less five dollars an hour for their work, the average Turker is younger, better-educated and not-as-well-compensated as the average working American, and that statistic that Gleibs gave us: MTurk is the primary income source for 25 percent of Turkers. The Pew Research Center also found that 25 percent of workers who earned money from online platforms, including MTurk but certainly not limited to it, used these sites because there was no other available work in their area.
Which does raise the question -- is doing research on crowdsourcing platforms like MTurk form of work, and if it is work, is it also inherently a little exploitive?
Jeffrey Bigham: When I taught a crowdsourcing course, one of the first assignments that I gave students here at Carnegie Mellon was to go on Mechanical Turk and try to earn $2. It turns out it's pretty hard.
Lauren Prastien: That’s Jeffrey Bigham. He’s a professor in Carnegie Mellon University’s Human-Computer Interaction Institute. In the summer of 2014, Bigham set out to see how much he could earn in four hours - that’s half a work day - on Amazon Mechanical Turk. And the answer was not that much.
For a dollar, Bigham was asked to write a roughly 350-word article on owning a pet chicken. The essay had some pretty good advice, like checking your city’s specific rules on chicken ownership before obtaining your pet chicken, buying an identification tag to put on your chicken’s foot should it escape from your yard, and informing your neighbors that you own chickens, in order to prepare them for your chicken’s escape attempts. Honestly, a lot of it was about your chickens trying to escape. Like so much so that it seemed more based on the 2000 movie Chicken Run than any kind of personal experience.
Chicken Run Clip: Mr. Tweedy, what is that chicken doing outside the fence?
Lauren Prastien: And my instinct turned out to be pretty correct. Not the Chicken Run part. The fact that Bigham’s actually never owned a chicken. But he was able to earn a dollar on MTurk pretending to. Because ultimately, what Bigham discovered was that in order to make any kind of substantive, worthwhile money on MTurk, you had to be able to work fast. And that was, understandably, pretty concerning to him.
Jeffrey Bigham: This is the worry. The worry is like, well, what kinds of problems are we going to manifest in our research if we're relying on these pools of people who are getting paid small amounts of money and thus rushing through. It is sort of worrisome I think.
Lauren Prastien: You know the saying. Good, fast, cheap -- you can only pick two. And if we’re about human knowledge, I’d say the top priority is good! But that’s not really what crowdsourcing is about. And that’s the tradeoff you make.
Jeffrey Bigham: You know, it used to be the people we would worry about you know, how much of our psychology research is being done by undergrads at elite institutions? Now it's kind of like how much of our psychology or behavioral kind of research is being completed by people on Mechanical Turk? On the one hand, like this might actually be good. People on Mechanical Turk might be a much more diverse sample than undergrads at elite institutions in the United States. At the same time, you're exactly right because of this kind of interplay between the low wages and also people not really paying all that well and this lack of a longterm relationship between employer and employee. The optimal strategy is to rush through.
Lauren Prastien: And if you’re sitting there thinking, well, wait a second, this is not supposed to be an employer-employee relationship, I want you to think again about who MTurk is attracting. Twenty-five percent of those participants are on there as their primary source of income. And twenty-five percent of participants are using gig platforms like MTurk because that’s what’s available to them as a source of income. And all of that data predates COVID.
Through his own work with using MTurk for guiding his research, Bigham was confronted time and again with the fact that the people on the other end of that platform were sometimes - but not always - dependent on the payment they received.
Jeffrey Bigham: I remember posting a job on Mechanical Turk. It was one of these computer vision jobs and we were actually paying pretty well. Like we have a lab policy of paying $10 an hour or more. Um, but what we were, I was contacted by from a worker, the message was roughly, you know, not, you know, pay me more or whatnot. It was basically, can you approve my payment earlier because I need to get formula for my baby. And that was a job that just happened to be, we are doing different experiments, happened to be geographically constrained to West Virginia. Right. So it gets pretty complicated thinking about this.
Lauren Prastien: So, Bigham started looking at MTurk as a workplace, and his findings have been pretty troubling. It’s hard to make a living wage, despite the fact that a lot of people on the platform are on there for just that. And it wasn’t always an accessible workplace for people with disabilities.
Jeffrey Bigham: There are interesting aspects of this where, you know, some of the protections for say, people with disabilities and employment may not or certainly don't yet at least get exercised on platforms like this, which are very kind of free market free form. So we did work looking at how almost none of the jobs, even the jobs that would be easily done by people with different disabilities are put up in a way that people could access.
Lauren Prastien: In a study that he conducted in 2015, Bigham found that people with disabilities were already using these platforms as a means of income. Which was a good thing, in terms of having the experiences of people with disabilities represented in data and in providing work, but there was virtually nothing in place to make this a viable long-term option, ensure stability, and provide the kinds of protections guaranteed by the ADA in a traditional workspace.
Another finding about the difficulties of crowdlabor as a workspace was that somehow, even within anonymity of the Internet, there was still a pay gap.
Jeffrey Bigham: The thing that's strange about it is that because the work is anonymous and employers and employees don't have much ways to communicate with each other, there's no explicit way that we could see that gender would be used as a basis for discrimination because the employers don't know if an employee is likely to be a man or a woman. And so how does that happen? And one of the hypotheses is that people, some people, are more likely to be working harder, doing a better job, sort of counter to what we talked about at the beginning of this conversation where some people seem to be less likely to be rushing through or not doing as good of a job to optimize their wages. And it could be the case that women are more likely to do a better job and thus they get paid less, which is sort of this terrible consequence of the incentives of a platform.
Lauren Prastien: So, yikes.
Jeffrey Bigham: I think that it's concerning to think about, well, what is the effect of workers and what are we doing to the workforce? And a lot of related issues about fairness when, you know, we're training these machine learning algorithms on data collected by people who are low-paid and don't benefit over the long term for their labor.
Lauren Prastien: You might be sitting here right now thinking, okay, but what does this have to do with research? I thought this was an episode about research, not about workplaces of the future. And I would argue that this has everything to do with research. Because, unfortunately, by turning to platforms like MTurk, we made it a conversation about work. Here’s Gleibs again:
Ilka Gleibs: We rely so heavily on Amazon and Amazon Mechanical Turk, which is not built as an infrastructure for research, but it's built with a commercial interest. Right. And that also gives us as researchers, have very little control of that environment and infrastructure. And if Amazon decides to change rules about fees or rules about participation, we, as the researchers, I have no input of that and we can only react, but we cannot shape that infrastructure. And so I think it would be interesting to have a similar infrastructure that doesn't rely on commercial interests.
Lauren Prastien: Amazon is ultimately a company organized around the principle of making money. And MTurk itself was a platform designed for work. This isn’t necessarily praise or criticism. It just is. And according to Gleibs, the platforms that have succeeded MTurk, even the ones centered on just supporting research, are still oriented towards profit. So, what happens when you try to make a noncommercial platform?
Ilka Gleibs: Of course it's not without problems. And the question would be who is responsible, who controls it? It will cost money, so who pays for that? And so on. So I think in an ideal world, it would be amazing to have a noncommercial platform. And in, in reality, it is a very expensive thing to do. And the question is who would pay for that? Lauren Prastien: When we braid scientific research, or just the advancement of human knowledge more generally, into a structure designed with a commercial interest, then we’re arguably commercializing the process of knowledge production itself. And there are arguments for why this is a good idea and why is this a bad idea, and they’re the usual arguments for and against commercializing anything: competition, productivity, exploitation, the cost of a noncommercial infrastructure. And at some point, we’ll have to decide what’s important to us.
Eugene Leventhal: The fact is that a lot of human knowledge, and the data that constitutes that knowledge, was produced using largely homogenous subject pools. Particularly as that data begins to feed the technologies of our future, it’s going to be imperative to diversify the subject pools we draw on for conducting research. While the Internet and platforms like Mechanical Turk have provided new avenues to finding research subjects, these platforms have a whole new set of advantages and drawbacks. Going forward, we’re going to have to decide if commercializing participation in scientific research is worth these inherent tradeoffs. Until then, this was Consequential. We’ll see you in two weeks.
Lauren Prastien: Consequential is produced by the Block Center for Technology and Society at Carnegie Mellon University. The Block Center was established to examine the societal consequences of technological change and create meaningful plans of action. To learn more about Consequential, the Block Center and our faculty, you can check out our website at cmu.edu/block-center or follow us on Twitter @CMUBlockCenter, all one word.
This episode of Consequential was written by Lauren Prastien and was produced by Eugene Leventhal. Our executive producers are Scott Andes, Shryansh Mehta and Jon Nehlsen. The music you’ve heard throughout this episode was produced by Fin Hagerty-Hammond. This episode also uses a clip from the movie Chicken Run. To learn more about our guests and see the sources we referenced for this episode, visit consequentialpodcast.com.
S3 E4: Can Automation Make Peer Review Faster and Fairer?
Lauren Prastien: Hey Eugene.
Eugene Leventhal: Yes, Lauren?
Lauren Prastien: You know what area of knowledge production I’m really excited about lately?
Eugene Leventhal: No, what?
Lauren Prastien: AI and machine learning.
Eugene Leventhal: That actually makes a lot of sense.
Lauren Prastien: Why?
Eugene Leventhal: Well, we have a whole podcast about it.
Lauren Prastien: Huh. Good point.
Eugene Leventhal: Well, something that’s really cool is that the amount of research and development in AI is growing exponentially.
Lauren Prastien: You know, I’ve heard about this. Because in their 2019 AI Index Report, our friends over at the Stanford Institute for Human-Centered Artificial Intelligence found that AI paper publications had increased by 300 percent between 1998 and 2018.
Eugene Leventhal: Attendance at academic conferences related to artificial intelligence has also skyrocketed.
Lauren Prastien: And it’s not just that there’s more research happening.
Eugene Leventhal: It’s also becoming easier to quickly train algorithms on a given dataset. That report from Stanford also found that the time needed to train a machine vision algorithm on the ImageNet dataset dropped from 3 hours in 2017 to a mere 88 seconds in 2019. It was also significantly less expensive.
Lauren Prastien: So, innovation in the areas of AI and machine learning is getting faster and faster, meaning there’s a lot more research being submitted and published.
Eugene Leventhal: Yeah, I wonder who’s reading all of them.
Lauren Prastien: What do you mean?
Eugene Leventhal: All the papers. I wonder who’s reading and selecting all the papers that get submitted to those journals and conferences.
Lauren Prastien: I mean…I would assume it’s people, right?
Eugene Leventhal: Which people?
Lauren Prastien: I don’t know, like experts. They would get experts to read the papers, like in any other field. It’s called peer review.
Eugene Leventhal: All of those papers? You mean all the papers that get published in those journals and presented at all those conferences?
Lauren Prastien: I mean, how many papers can it be?
Eugene Leventhal: Well, just as an example, the 2020 Association for the Advancement of Artificial Intelligence Conference received a record-high number of more than 7,737 submissions, which they had to narrow down to just over 1,500 papers.
Lauren Prastien: That’s…a lot of papers.
Eugene Leventhal: Yeah. And that’s just one conference.
Lauren Prastien: That has to be pretty hard on the experts.
Eugene Leventhal: Hmm. I wonder if automation could help.
Lauren Prastien: Why, Eugene, are you asking a leading question?
Eugene Leventhal: You caught me!
Lauren Prastien: From the Block Center for Technology and Society, this is Consequential. I’m Lauren Prastien
Eugene Leventhal: And I’m Eugene Leventhal. Today, we’re looking at the question of automation in the scientific publication process. Could it help, is it happening already, and what does it have in common with the job application process?
[break]
Lauren Prastien: Let’s back up a minute. I want to be sure we’re all on the same page about how the publication of scientific research works in the first place. To explain, I invited Nihar Shah to talk to us. He’s a computer scientist at Carnegie Mellon, whose work focuses on statistical learning, information theory, game theory and social choice. And in particular, how these principles apply the evaluation of work submitted to journals and conferences.
Nihar Shah: When you conduct some research, you will write it up in the form of a research paper. You would then submit it to a conference or a journal where other researchers, typically two to five other researchers, will evaluate your work.
Lauren Prastien: And this process is something called peer review. The name is pretty self-explanatory. Essentially, your peers - or other established researchers with similar competencies to you - perform a review of the research you’ve done.
Nihar Shah: They will then provide feedback on your work, any suggestions to improve it, as well as a recommendation or a decision on whether to accept or reject the paper from the conference or the journal.
Lauren Prastien: According to Shah, peer review is part of the backbone of scientific research, because it maintains the standards of quality, accuracy, originality and relevance within a given field’s body of knowledge.
Nihar Shah: It can help filter out problematic research. So for example, in a research paper, the reviewers may identify that, “Hey, this experimental methodology is inappropriate and hence the conclusions drawn from it or inappropriate.” Or, for instance, in a paper which is theoretical or mathematical, some reviewers may find bugs in the proofs, for example. So in the absence of peer review, these papers would have been published. And then the society at large might have come to these incorrect conclusions.
Lauren Prastien: It’s important to clarify that peer review isn’t just a yes/no kind of system. Often, the way it works is that a reviewer will offer feedback to improve the quality of a given research manuscript in order to meet the standards for publication. This might include increasing the sample size, pointing out an angle of inquiry that the authors initially ignored, or asking for more evidence to support a claim. To this end, a survey published in the Journal of the Association for Information Science and Technology actually found that nine out of ten authors of scientific research believed that the peer review process ultimately helped improve the paper they published.
Essentially, peer review is one of the things that keeps scientific research honest. But, it’s also pretty time-consuming, and, come on, let’s admit it for a second, like anything else involving human judgment, it’s pretty subjective.
Nihar Shah: There's actually a very nice quote by Drummond Rennie in an article in Nature about four years ago, which summarizes the many issues in peer review. It says that peer review is a human system. And so everybody involved brings in prejudices, misunderstandings and gaps in knowledge, and hence there are biases, inefficiencies, and corruption in the process.
Lauren Prastien: These are pretty standard for what you might expect of any human judging another human’s work. Some reviewers are stricter, and some are more lenient. Some subjectively find some research more interesting or groundbreaking than others would. And then there’s some really exceptional, really alarming examples.
Nihar Shah: So in 2015, there was a paper in the PLoS journals. This was submitted to the PLoS One journal by two women authors, Fiona Ingleby and Megan Head. And the review that they received was quite negative. And then as the part of the review, it said something like, “it would probably be beneficial for you to find one or two male researchers to work with.”
Lauren Prastien: I was really taken aback by this, but I also wasn’t particularly surprised. Still, I wanted to know more. Like, what was the research? And what did the journal do about this reviewer?
So, here’s the story. Basically, Fiona Ingleby - who was, at the time, a postdoctoral researcher in evolutionary biology at the University of Sussex - co-wrote an article with Megan Head of the Australian National University that sought to highlight the gender differences inherent to who was advancing from PhD candidacy to post-doctoral research positions. And they submitted it to PLoS, which stands for the Public Library of Science.
So, this was literally a paper about sexism in academia. And, ironically enough, the reviewer wrote that it would, quote
Eugene Leventhal: “Be beneficial to find one or two male biologists to work with (or at least obtain internal peer review from, but better yet as active co-authors)”
Lauren Prastien: In order to keep the article from, quote
Eugene Leventhal: “Drifting too far away from empirical evidence into ideologically biased assumptions”
Lauren Prastien: So, basically, the reviewer went, “ladies, I hear ya, but could you go find a man or two to back you up?” Which is a little outrageous in and of itself. But here’s the part that really got me, Ingleby and Head were using actual evidence. They conducted a survey of men and women with PhDs in biology and looked at who had the better job prospects and publication records. And then in response to that, the reviewer said, quote:
Eugene Leventhal: “Perhaps it is not so surprising that on average male doctoral students co-author one more paper than female doctoral students, just as, on average, male doctoral students can probably run a mile a bit faster than female doctoral students,”
Lauren Prastien: I’ll say it, it’s just so telling to me that Ingleby and Head presented actual research, and then this guy went on this like weird biological essentialism argument like, “if sexism to blame for problem in academia, why woman run so slow?”
Anyway, after Ingleby tweeted about the review and appealed the reviewer’s decision, the case gained quite a bit of media attention. And as a result, PLoS One - which was the Public Library of Science journal where the research had been submitted - had to issue an apology, fire the reviewer, forward the manuscript to a different editor, and called for the resignation of the Academic Editor who handled the review.
Nihar Shah: Now this is kind of an extreme example where, you know, the reviewer is really explicit about the bias, but that said, no, we can imagine that given the biases prevalent in the society, they would also be reflected in the peer review process.
Lauren Prastien: Shah raises a really important point. For every reviewer like the one that Ingleby and Head encountered, there are probably reviewers that just think like this, but don’t flag it quite so explicitly. And this isn’t just restricted to gender.
Nihar Shah: There is quite a lot of literature in terms of investigating the existence of biases with respect to various groups of people, particularly in peer review settings, where the reviewers know the identities of the authors. Researchers have previously investigated biases with respect to gender, with respect to race, with respect to how famous the authors are, with respect to their affiliation, with respect to their geographical location, and so on.
Nihar Shah: So that's one issue. The second issue is that now there is a huge interest in machine learning and in artificial intelligence. Every year, there are thousands of papers submitted. So we don't have… we don't even have enough really expert reviewers to rely on. So currently what the communities are doing, and this might be really surprising to researchers in various other fields, but currently what we are doing is, you know, graduate students do a large fraction of the reviewing and in fact graduate students who are very early in their graduate studies do that.
Lauren Prastien: Really quickly, I want to clarify why this would be surprising. There’s a general understanding that peer review is conducted by individuals with some degree of authority in their field. While it’s not unheard of for graduate students to do peer review, and certainly, I know graduate students in other scientific fields that have done peer review, the idea that students that are early on in their studies have to take on a lot of this work does point to the idea that maybe...maybe there’s a little bit of a problem.
Which begs the question that often comes up in any existing process where there’s a lot of bias, the human beings involved are getting overloaded, and there’s just too much stuff to sort through...could machine learning maybe help?
[break]
Lauren Prastien: This idea that we brought up at the beginning of the episode isn’t a new one. Scientific research has indeed begun turning to practices of automation in order to lighten the load for overburdened reviewers.
In fact, one 2018 article in Nature declared “AI peer reviewers unleashed to ease publishing grind.” Which kind of makes it sound like there’s this army of robots that somebody trained to be really picky about p values and then just released on the world. According to Shah, the truth is a little less evocative, maybe, but it’s still pretty compelling.
Nihar Shah: Some journals are using machine learning to identify certain commonplace issues in papers. For example, as just as an example, it can try to identify if this paper has an appropriate sample size in that experiment or not.
Lauren Prastien: So, yeah, still picky about p values, but maybe not capable of world domination. There are already a lot of these tools out there, by the way, and they perform a variety of functions from identifying papers with really glaring errors, like sample size, to flagging cases of plagiarism, to verifying outside statistics that are being referenced in the paper. And you can see how this might save reviewers some time, and even catch things that they might miss themselves, which is usually why we implement algorithms into a workflow in the first place.
Algorithms are extremely good at putting things into categories and picking up on patterns, and this is why they’re often so promising and sometimes also really troubling, because there’s often a fine line, for example, between categorizing and stereotyping. Which is also a decidedly human error as well, as we saw in the case of Head and Ingleby.
So, generally, avoiding that on the algorithm side usually means keeping the filtering criteria pretty cut and dry – so, is this statistic confirmed or not? Is this an appropriate p value? – and keeping a human in the loop. So, to that end, we asked Shah if there would ever be a case where there might be a human out of the loop in this process.
Nihar Shah: There has been some discussions on trying to use machine learning to review the entire paper. So you submit a paper, and the goal is to design an ML algorithm which will review the entire paper and make a decision of whether to accept or reject. I personally think that we have to be much more careful and, you know, trying to achieve this goal is still a little far away.
Lauren Prastien: So, yeah, this is a long way off, and it might be something that academia decides is actually not worthwhile to do. Or, at least, as most automated decision-making systems go, it would still have a human in the loop somewhere. Ideally, the most prepared human. By that I mean something that Shah and other researchers have begun to look at is leveraging the sorting and categorization capabilities of automation in order to more effectively assign reviewers to a given paper and, more broadly, evaluate the best reviewers for that paper.
Nihar Shah: So currently machine learning and AI conferences have 5,000 to 10,000 papers submitted in each conference. So whatever you're doing needs to scale to this level. An example is the problem of assigning reviewers to papers. So if you had an entire year to assign reviewers to papers for, let's say a thousand papers, you might be able to do it manually. But now in the conference, you just have a couple of days to do that. And so it's impossible to do it manually, and we do need assistance of algorithms there.
Lauren Prastien: So we asked him what that might look like.
Nihar Shah: In these large conferences where you have thousands of papers and you have thousands of reviewers, it is not possible for the conference organizers to manually assign the reviewers to papers. So here is where machine learning is really helping where we have machine learning algorithms that take in the profiles of all reviewers, take in the text of the submitted papers, and then these algorithms match the reviewers and papers. And for each paper find the reviewers which have the best similarity.
Lauren Prastien: Which totally makes sense, right? If you assign a paper to a reviewer that is truly an expert in that area, then the review is probably going to go faster and be conducted from a more informed perspective, meaning that it would ideally be a fair review. And with regard to the notion of fairness, Shah also sees an opportunity for machine learning to play a role.
Nihar Shah: Now if I'm organizing a conference, in my conference, each reviewer is reviewing, say three papers. So just based on three reviews for a reviewer, it is really hard for me to draw a conclusion that this reviewer is actually really strict in general. But instead, hypothetically, if I had access for this reviewer's review for 10 other conferences, then based on those 30 reviews, I can tell more conclusively that yes, it appears that this review is generally really strict. And so let me recalibrate this reviewer’s review in a certain fashion.
Lauren Prastien: Ultimately, it’s important to consider that the concepts of bias and fairness in publishing are a lot to take on, and they’re often deeply intertwined with other aspects of the academic research community that aren’t immediately related to peer review itself. But in order to take on these problems, or even understand how much of a problem they even are, Shah argues that you need to be able to make an informed argument.
Nihar Shah: So now towards addressing certain challenges in peer review, ideally it would have helped if we had access to a lot of peer review data.
Lauren Prastien: But right now, that isn’t really the case. Because according to Shah, this data is both secure and siloed.
Nihar Shah: So currently in conferences, each conference keeps the data from that conference. You don't see everything being made public. There are some conferences which publish the reviews for each paper, for example, but you don't know who reviewed which paper. And this is because in peer review, the identities of the reviewers are kept secret and this information is quite sensitive.
Lauren Prastien: It’s actually debatable whether keeping the names of the judges – and for that matter, the authors as well - private is a productive thing to do or not, and there are certainly valid arguments on either end. Keeping the authors anonymous means that the reviewers can’t judge the work based on the authors’ names, reputations or affiliations, but also, it is actually pretty hard to keep authors completely anonymous. Some areas of study, even within the massive fields of AI and machine learning, are pretty tiny, and so it’s sometimes fairly easy to guess who wrote what, especially when authors might need to reference their own past published work to prove a point. On the reviewer side, protecting the anonymity of a reviewer means that junior reviewers or people earlier on in their careers wouldn’t be afraid to criticize the work of senior researchers. But, on the flip side, research has shown that actually, maintaining the anonymity of the reviewer might not always be helpful. Way back in the year 2000, a study published in the British Journal of Psychiatry found that reviewers that had to disclose their identities to the authors whose work they reviewed ended up writing reviews that were more constructive and civil than those who conducted those reviews under the shield of anonymity.
Right now, privacy practices generally vary from journal to journal and conference to conference. Which means that when it comes to doing research on the practice of peer review and being able to quantify some of these potential barriers to fairness and equity, researchers don’t always have a lot to go on.
Nihar Shah: There is no transparency on say the process of how the conference judges which are the best papers, or for example, how the conference program committee was chosen and so on. And the lack of transparency in these processes means that we don't know if there are any implicit or perhaps explicit biases therein.
Lauren Prastien: So, what could be done?
Nihar Shah: Recently, some of us have started looking into this and we are looking to use techniques from the privacy literature, like differential privacy notions, like differential privacy, and looking to see how we can design some techniques in peer review so that we can release some more data while still ensuring privacy in under these notions.
Lauren Prastien: So, differential privacy is a mathematical principle that essentially sets up a system whereby information can be shared publicly about a given dataset without actually sharing the individual information within that dataset. It’s something that Tom Mitchell brought up last season in looking at ways to safely share public health data during a pandemic, and what it basically would mean is that researchers would have access this kind of sensitive data without violating the privacy of the people performing peer review.
And Shah sees a way to use the approaches of differential privacy in order to anonymously evaluate the track records of given reviewers and ensure that maybe some measures could be taken against biases that might be trickling in, that oftentimes reviewers might not even know they have.
But peer review isn’t the only place where practices like this might come in handy, and certainly, it’s not the only practice of candidate selection that Shah and his collaborators are looking at. Research in this area is setting the groundwork for the application of methods such as these in hiring.
We’ve talked before on this podcast about how automation is also reducing the load of human resources staff looking to fill jobs but let me just give you a very quick info dump to get you up to speed. On average, a given listing for a single job in the United States will attract applications from 118 candidates. And according to a study conducted by PwC, 40% of the HR functions of international companies are already using AI, and 50% of companies worldwide use data analytics to find and develop talent. For instance, companies such as Hilton, AT&T, Procter & Gamble, and CapitalOne all use AI and machine learning to perform initial functions of the hiring process like sort through thousands of applications, schedule interviews, and perform initial screenings, So, similarly to automation in peer review, it targets those very early phases, before relinquishing judgment to a human.
But, as in peer review, those human judges are not infallible, and they’re often working under a certain time constraint to process a certain number of applicants. And so it’s the same fundamental question: how do you keep the process fair, but still make sure it’s fast enough to fill a position in a reasonable time? It’s something researchers like Shah hope to take their experience in peer review to start exploring.
But in the meantime, this was Consequential. If you like what you’ve heard, let us know in a review on Apple Podcasts, and we’ll see you in two weeks.
Eugene Leventhal: Consequential is produced by the Block Center for Technology and Society at Carnegie Mellon University. The Block Center was established to examine the societal consequences of technological change and create meaningful plans of action. To learn more about Consequential, the Block Center and our faculty, you can check out our website at cmu.edu/block-center or follow us on Twitter @CMUBlockCenter, all one word.
This episode of Consequential was written by Lauren Prastien and was produced by Eugene Leventhal. Our executive producers are Scott Andes, Shryansh Mehta and Jon Nehlsen. The music you’ve heard throughout this episode was produced by Fin Hagerty-Hammond. To learn more about our guests and see the sources we referenced for this episode, visit consequentialpodcast.com.
Further reading:
- A tutorial on fairness and bias in peer review: https://www.cs.cmu.edu/~nihars/tutorials/AAAI2020/peerreview_slides_aaai2020.pdf
- Stelmakh et al. “PeerReview4All: Fair and Accurate Reviewer Assignment in Peer Review.”
- Mulligan et al. “Peer review in a changing world: An international study measuring the attitudes of researchers.”
- Tomkins et al. “Reviewer bias in single- versus double-blind peer review.”:
- Carole J. Lee, “Commensuration bias in peer review.”
- The Research on Research blog entries: “There’s lots in a name” (https://researchonresearch.blog/2018/11/28/theres-lots-in-a-name/ ) and “Gender distributions of paper awards” (https://researchonresearch.blog/2019/06/18/gender-distributions-of-paper-awards/)
- Jason M. Breslow, “Reviewing peer review.”
S3 E5: Enron, Wikipedia and the Deal with Biased Low-Friction Data
Lauren Prastien: Hey Eugene. You know how I like goats?
Eugene Leventhal: Yes.
Lauren Prastien: Did you know that goats were first domesticated about 10,000 years ago?
Eugene Leventhal: That’s a long time!
Lauren Prastien: And did you know that from 2001 to 2009, there was a lance corporal in the British Army named William Windsor, and he was a goat!
Eugene Leventhal: All right, neat.
Lauren Prastien: And in 1986, in Lajitas, Texas the incumbent human mayor lost his election to a goat named Clay Henry who liked to drink beer and headbutt people.
Eugene Leventhal: That cannot be true.
Lauren Prastien: No, really, the goat won by a landslide, and goats have been mayors in Lajitas ever since.
Eugene Leventhal: Where are you getting this from?
Lauren Prastien: Wikipedia, duh.
Eugene Leventhal: Wow! You can really find anything on Wikipedia.
Lauren Prastien: Mmmm, I mean, you’d think that.
Eugene Leventhal: What?
Lauren Prastien: Oh. Nothing.
Eugene Leventhal: No, what is it?
Lauren Prastien: It’s just that right now Wikipedia has a bit of an issue of representing women and people of color. Like, those pages are either incomplete or even nonexistent. Gender and racial bias on Wikipedia is such a prevalent issue that the National Science Foundation has granted over $200,000 to fund research on how to try to overcome it, and the Wikimedia Foundation itself has devoted $250,000 to try to improve Wikipedia’s gender diversity problem.
Eugene Leventhal: Where’d you learn all that?
Lauren Prastien: Wikipedia.
Eugene Leventhal: That seems like a pretty big problem.
Lauren Prastien: Yeah. Especially considering that Wikipedia is used as the foundation of a lot of fact checking and natural language processing algorithms. In fact, the Watson computer that absolutely wiped the floor on Jeopardy and beat both Ken Jennings and Brad Rutter in 2013 was trained in part on data from Wikipedia.
Eugene Leventhal: And let me guess, you got that all from…
Lauren Prastien: Wikipedia, yeah.
Eugene Leventhal: I think I see where this is going. Today, are we talking about how Wikipedia and other low-friction data sources contribute to bias in algorithms, and the barriers to accessing less troublesome sources of knowledge and data?
Lauren Prastien: Yup! Good guess! From the Block Center for Technology and Society, this is Consequential. I’m Lauren Prastien
Eugene Leventhal: And I’m Eugene Leventhal. Stay with us.
[break]
Lauren Prastien: Before we get any deeper into how Wikipedia is powering a lot of today’s and tomorrow’s algorithms, I need to back up a second. Like, nineteen years. To 2001. This was actually the year that Wikipedia was first registered as a domain name, but that’s not what I want to talk about. Instead, our story begins in an unlikely place: Enron. Bear with me.
In late 2001, the energy trading and utilities company Enron was in huge trouble. Not only for the biggest case of corporate fraud in history, but because it was also accused of manipulating electrical prices during California’s energy crisis.
In order to conduct their investigation, the state of California and the Federal Energy Regulatory Commission, or FERC, sifted through massive amounts of memos and company communications to see exactly how Enron intended to manipulate California’s energy markets. And a big part of the evidence they were sifting through were emails.
Lots and lots and lots of emails.
And, yes, some of them were useful evidence. But most of them? Most of them were, to borrow an expression from Newton Minnow, a vast wasteland. Inside jokes. Invitations for coffee and drinks. Meeting reminders and cancellations. There were accounts of office romances and arguments and enduring friendships. There was a picture, frozen in amber, of a company buckling beneath its own culture of dishonesty and greed. And there were tons of chain emails, because remember, this was 2001.
After the investigation, the FERC determined that the emails were in the public’s interest, and this became the Enron corpus. In 2003, a computer scientist at the University of Massachusetts - Amherst named Andrew McCallum purchased the Enron corpus for $10,000, organized it into a set of 600,000 messages from 158 employees, and made it readily available to academic and corporate researchers.
And then something kind of incredible happened. Not because they were particularly exceptional or good. But because there were a lot of them, and they were right there, ready for the taking.
Amanda Levendowski: They remain close to or on par with Clinton emails as the largest dataset of real emails in the world. And they are machine readable. They are easily accessible online. You want to talk about low friction data; these are as low friction as they come. So they became this appealing source of training data.
Lauren Prastien: That’s Amanda Levendowski. She’s the founding Director of the Intellectual Property and Information Policy Clinic at Georgetown University, where her work looks at how the availability of certain intellectual property and content, like the Enron Corpus, has impacted the biases inherent to artificial intelligence.
In a 2013 article in the MIT Technology Review, the computer scientist William Cohen compared the use of the Enron Corpus to scientists’ extensive reliance on yeast as a model organism. Per Cohen: “It’s studied and experimented on because it is a very well understood model organism. People are going to keep using it for a long time.” And to that effect, about 30 scientific papers each year, by Cohen’s estimation, cite the Enron corpus.
And the Enron corpus has actually done a lot of good. It’s behind some of the algorithms that we use everyday and don’t really think about. For instance, in 2004, two researchers at Carnegie Mellon’s Language Technologies Institute found that the Enron emails were really useful for successfully identifying spam messages before they got to your inbox. So, yeah, the Enron emails helped give us spam filters. Google also used them for the smart compose function in Gmail, to help anticipate what you might say next in an email. Ironically, Enron has helped make working life and using the internet a lot more convenient.
And the Enron corpus is also used in a lot of ways that you wouldn’t expect. Because Enron’s emails represent the dynamics of a community, those emails have been used to study other ways things move through communities, like the spread of a virus or the evolution of linguistic patterns and jokes within a group.
But it’s not without its problems.
Amanda Levendowski: If we take a step back and consider why we have access to the Enron emails in the first place, we can spot some pretty big problems with it. They were collected as part of an investigation in two way, systemic, widespread fraud rooted in an unethical corporate culture that was facilitated and cultivated over the course of many years. But because they are biased and low friction, they remain appealing and we still don't have a great grasp of how that affects the way that we interact with these algorithms. What kinds of biases are picked up by the algorithms by relying on bias training data?
Lauren Prastien: I’m going to be really frank with you. Some of the Enron emails are so racist, so classist, so sexist, and so inappropriate that I can’t read them to you on this podcast, even heavily censored. And then there are the more insidious problems with the language being used in these emails that has nothing to do with profanity. Baltimore is referred to as a crime-ridden cesspool. There’s a lot of anxiety about outsourcing that gets incredibly xenophobic. The men - and also sometimes the women - of Enron discuss going to strip clubs and candidly detail their romantic lives. There’s even an entire email chain devoted to whether or not it’s a bad thing that a local bar has decided to stop serving alcohol to underaged women, which some of the men at Enron who like to frequent that bar are disappointed about. It’s not a good culture, but it was what we had available.
Which brings us to Wikipedia. Which is no Enron by any means. But here’s the situation:
Wikipedia, if you are not aware, is not just a site for goat facts. It is the Internet’s free encyclopedia, home to over 52 million articles - 6 million of which are in English - containing over 3.7 billion words. Every day, an average of 594 new articles are posted to Wikipedia. So you can see how it would be a really appealing, really plentiful data source. Like what Levendowski said of Enron, it’s what you’d call a low friction data source. As in, it doesn’t require a lot of effort or expense to obtain it. It is literally just right there online.
If we want to talk about democratizing knowledge and the accessibility of information, Wikipedia is kind of incredible. And as the journalist Michael Mandiberg said in an article in The Atlantic this past February, we treat Wikipedia like a utility. It’s where most search engines direct you when you’re looking up facts on a certain topic.
But it’s important to consider that Wikipedia exists because people volunteer to put that information on the Internet and organize it. Just like how the Enron emails had to be organized to be useful, the information on Wikipedia is present and digestible because of the labor of its autonomous group of volunteer editors.
Right now, we don’t know a ton about exactly who edits Wikipedia, but we do know some things. Like, the fact that a lot of Wikipedia editors are cis white men from the global North, and that 77 percent of Wikipedia is written by just 1 percent of its editors, or a group of approximately 1,300 people. You can imagine how this might go wrong, like a few months ago, when it was revealed that a huge portion of Scots Wikipedia - that is, Wikipedia in one of the indigenoius languages of Scotland - was written, edited and moderated by an American teenager from North Carolina who didn’t even speak the language. This one nineteen-year-old had authored more than 20,000 entries and added about 200,000 edits. The tagline of an article about this in The Register even read: “None of you trained an AI on this data set, right? Right?”
This is, however, an extreme example. But as the extreme examples often do, it shed light on a greater problem inherent to low friction data like Wikipedia and the ways we generate and organize knowledge more generally. We’ll talk about that in just a second.
[break]
Katie Willingham: I actually can't remember when I made the account. I think it might've been in college. You can get directed to pages that need help, and I thought it might be fun to go on and correct some grammar. I am clearly a very fun person, I know, but truly the experience was kind of fiddly and technical and not as seamless as I imagined. So I didn't spend that much time on it doing that kind of thing. I got more into it when I realized that material was missing.
Lauren Prastien: The voice you’re hearing is Katie Willingham. She edits Wikipedia, mostly the arts and literature entries of Wikipedia, and she’s a huge proponent of the site. In fact, she even thanked Wikipedia in the acknowledgments of her book.
Katie Willingham: I think the first thing that interested me was the ease of access. I think that's true for a lot of people. I mean, if you're old enough to remember using a hard copy encyclopedia to do research, not only was it annoying to flip through the pages, but then a team of editors spent countless hours deciding what deserved a picture and it was never what you were looking up is what I recall. And it’s not just access to something on Wikipedia, it's actually access to both brevity and depth at the same time, which was just not a reality of the genre before.
Lauren Prastien: In addition to brevity and depth, Wikipedia is kind of incredible in terms of its immediacy, which Willingham demonstrated for us in real time in looking at the COVID-19 page.
Katie Willingham: As you might expect, the article on COVID-19 was last edited today, only minutes ago. And as of this recording, it has material sourced from 190 sources. So this is real value, to the speed and scalability of what we can collect. And this page has comprehensive sections and graphs and visuals. It's, you know, all being constantly updated, which is incredible. And that would not be possible if you were talking about printing the encyclopedia, we wouldn't have any information in this kind of format about COVID-19.
Lauren Prastien: Today, that page has 459 sources. And whenever you’re listening to this, I expect that number might be higher. Which, like Willingham said, is kind of incredible, compared to how long it would take for that information to be put into a hard copy encyclopedia.
Katie Willingham: So when talking about hard copy encyclopedias, I mentioned that team of editors who were doing this professionally and getting paid to understand history and information and to speak to experts and reduce it down into these volumes and those are skills and I don't want to discount that. But I understand that information is never just information. So the more people have a hand in creating this material, in theory, the better that is.
Lauren Prastien: Wikipedia taps into something that we’ve addressed before on this podcast: collective intelligence. Which is the idea of a kind of shared intelligence that arises when a group is collaborating and drawing on the strengths of its members, and like our guest Anita Williams Woolley said in the very first episode of this podcast, as a group’s diversity increases along the lines of things like gender and race, the collective intelligence of that group has also been shown to increase. Which Willingham argues is especially pertinent for a resource like Wikipedia.
Katie Willingham: It's not just about letting people with expertise pour it into this collaborative space. it can feel like a privilege to write whatever you want on Yelp or to, you know, be able to say, “oh, I liked this. I didn't like this.” But when it comes to knowledge, it's important to remember that it's not a privilege. It's also a labor. And when we think about it as work that can help us answer these diversity problems, I think, you know, who has time or feels they can make the time to edit Wikipedia. And when they get there, how are they heard? Or how are they silenced?
Lauren Prastien: In the Information Age, as it were, it’s easy to take for granted that information doesn’t just appear from on high. It comes from people processing things like data and events and sources. And it’s also people that decide what’s worth mentioning and how we describe it. What’s amazing is that Wikipedia allows, like Willingham said, in theory, more people to have a say in that.
But, like we’ve been saying, Wikipedia has a diversity problem, and the content on Wikipedia has sometimes suffered because of that. In 2006, the historian Roy Rosenzweig published an article in the Journal of American History titled, “Can History Be Open Source? Wikipedia and the Future of the Past.” In it, he considers that the completeness of a given article on Wikipedia has a lot to do with the interests of Wikipedians, and not necessarily the subject matter of the article itself. So, for instance, a lot of black history and accomplishments by women and people of color are missing from Wikipedia, which does unfortunately make sense, if a lot of Wikipedians are white men and went through education systems that also prioritized teaching the accomplishments of white men. To give you a really solid example of what that might look like, here’s Amanda Levendowski again:
Amanda Levendowski: It's actually the same way that the sort of quote unquote, Western Canon holds bias because it's all about whose facts are selected to be presented. And one of the examples from my research that I think really just illustrates the point beautifully is comparing the facts of two articles, one that exists and one that doesn't, and interrogating what that tells us about what editors are prioritizing. And that example is Rob Gronkowski. He's a New England Patriots tight end, and his article is nearly 4,000 words long, and it boasts 66 citations. On the other hand, the first woman admitted to the New York State Bar, Stanley Etta Titus, does not even have an article. Those are both about facts, but it reveals a bias toward whose narratives are important and whose narratives end up becoming training data for these algorithms that can reinforce those same hierarchies and bias.
Lauren Prastien: We see those hierarchies and biases played out today in a lot of algorithms that we use every day. In 2016, a high school student in Virginia named Kabir Alli found that Googling the phrase “three white teenagers” yielded pictures of smiling white people, whereas Googling the phrase “three black teenagers” resulted in police mugshots. Which is to say that it’s not just completeness that matters, it’s also context and categorization, like the difference between the concepts of sex and rape.
Katie Willingham: In some of my research, I have enjoyed visiting the talk -- it's called the talk page -- that's kind of the back end of a page on Wikipedia. And that is where you'll see all the conversations that people are having about language, about different things. And, sometimes that is contentious for political reasons. Sometimes someone wants to use particular language to describe a rape scene in a film and they want to call it a sex scene. And there are other editors on there that want to maintain the language of a rape scene being represented, because they think that that is important and there will be a big, you know, argument that ensues.
Lauren Prastien: If you’re sitting here like, this is a semantic argument on Wikipedia, what’s the point, let me present you with this: when our guest Amanda Levendowski was a law student at the New York University Law School, she actually wrote the first Wikipedia article on the concept of revenge porn, which is, according to the Wikipedia article, the distribution of sexually explicit images or videos of individuals without their consent. That Wikipedia article was actually cited in the first criminal court case related to revenge porn in New York State, People v. Barber, in 2014. Which, if you’ve got algorithms using existing court data to start to help in adjudication, yes, it matters if the Internet’s knowledge base can tell the difference between sex and rape, even if it’s just in a movie.
And that kind of seems to be the moral of the story, is that you need people stepping in who are sensitive to these topics and concepts. So, if Wikipedia is truly an open platform, why aren’t there, say, more women editors? There’s actually no significant difference in readership rates of Wikipedia between men and women, but only about 9% of global contributors and 15% of contributors in the United States are women. And there have been efforts to bridge these gaps, like edit-a-thons for women to fill out, say, the articles on female scientists on Wikipedia. So, what’s stopping them from pitching in more generally? There’s been a lot of research, both formal and informal, on this very subject, and the answers vary. Women find the talk section of Wikipedia that Willingham was referring to to be aggressive, not user-friendly and sometimes outright misogynist. Some women said they gave up contributing because their material was edited out after being deemed “insufficiently significant.” Or, one of my favorite responses: “Want to know why I’m not editing Wikipedia? I’m busy doing science.”
Remember, Wikipedia is voluntary, and the people who edit it, like Willingham and others like her, do it because they love the resource and what it stands for. But it’s also important to remember that a labor of love is still labor.
Katie Willingham: I want to go back to that image of smashing the idea that knowledge gets bestowed upon you from on high, and we all need to be liberated from that notion. Yes, categorizing is what we do as a species. And my favorite thing I read in the book Sapiens was that perhaps something that differentiated Homo sapiens from earlier sapiens was their ability to gossip, meaning to categorize who is trustworthy and who to trade with and who to avoid. And if this is what we humans do, that's also a reminder that this is invented. All of it's invented, all the systems that rule our lives are invented, which means we could make very different realities if we can help ourselves imagine them.
Lauren Prastien: I asked Willingham if it made her anxious at all that Wikipedia was underlying a lot of fact checking and natural language processing algorithms, given some of the problems inherent to the platform.
Katie Willingham: It's interesting because, you know, I want to be scared by that. And at the same time, this is where humans get their information. So of course, machines get their information there, right?
Lauren Prastien: Which, you know what? Fair. So, where do we go from here? We can improve the internet’s encyclopedia, but that only gets at one part of this issue of low friction data. It’s important to consider that right now, we actually don’t yet know how exactly data does or doesn’t bias an algorithm. This is still an early field of scholarship, and there are a lot of people busy at work trying to figure that out. But is it worth the risk - or, rather, the inconvenience - to use data that we know has these super engrained, inherent problems? And if not, what’s the alternative?
[break]
Lauren Prastien: Enron and Wikipedia are just two examples of biased low-friction data, but they certainly aren’t the only ones. So, fixing the issues inherent to Wikipedia - while a worthwhile and important pursuit - isn’t going to completely fix this problem. Here’s Amanda Levendowski again:
Amanda Levendowski: So many of the works that people want to use to train their algorithms from images of faces to train facial recognition algorithms to long pieces of expressive text, like are used in a novel, to long pieces of sort of drier more factual texts like you might find a newspaper, all three offer different things to different types of algorithms in the form of training data. But the reason I think people are attracted to biased low-friction data is that if you want to appropriately license all of those pieces of training data, it costs a lot of money and you still might not be able to access everything you want.
Part of the reason is because of copyright law and whether or not engineers realize that this is a fear or a concern that's animating their decision making or not, there's an attraction to free, open low-friction data because it comes without restrictions. But parts of the reason that things are free and unencumbered is because they're not always the best quality for the use. And so if you're talking about developing a natural language processing algorithm, and you only want to rely on public domain works, you're locking yourself and you're committing yourself to the same biases that were embedded in all of the published works available before the year of 1926. And as I'm sure you can imagine there's a lot of historical, socioeconomic, social biases embedded in works before 1926.
Lauren Prastien: Copyright owners have been historically rather litigious when it comes to computational technologies using their property, which, to an extent, does make sense. First of all, that’s your property. And second of all, you don’t know if your copyrighted material is going to be used for some form of technology that you might ultimately disagree with. But according to Levendowski, copyright law also impacts low-friction data resources. You may remember that one algorithm that made the news by associating men to computer programmers and women to homemakers.
Amanda Levendowksi: The real kicker here is that this was based on a corpus of Google News articles. So by reading and processing articles into Google News, the algorithm picked up on that as a word embedding pairing, which revealed the inherent gender biases in the news we consume. However, just because we know that about Word to That, we can't go into the Google News corpus and figure out exactly where these biases are coming from. We don't know if it's from a particular geography of reporting. We don't know if it's a particular cohort of reporters. We don't have that data because the underlying corpus is still under copyright and it's only usable by Google. It's not usable by the general public.
Lauren Prastien: So, yeah, the lines of copyright law undercut a lot of the issues inherent to the diagnosis and understanding of algorithmic bias. But in a paper tilted, “How Copyright Law Can Fix Artificial Intelligence’s Inherent Bias Problem,” Levendowski considered that this wasn’t a matter of completely changing copyright law itself as invoking existing areas of the law through a new lens:
Amanda Levendowski: Fair use is this special copyright doctrine that says you can use other people's works without permission as long as it's for one of these statutory purposes. And when we balance out these four different factors, we feel comfortable that this use is a fair one and that's sort of what this piece of the law is meant to do is to offer the opportunity to use work without permission to create new things that kind of transform our meaning and understanding of the work as it was before. And I think that as I described in the paper, there are lots of ways in which using copyrighted works to train AI systems, get to the heart of this challenge.
Lauren Prastien: At this point, I asked Levendowski if she thought this was something that might be settled in an actual courtroom in the near future.
Amanda Levendowski: I think what's going to be tricky is that yes, there's going to be litigation at some point probably sooner rather than later. And judges are not always well equipped to grapple with the nuances of how technology impacts copyright law. We can look to how the Supreme Court dealt with the Aereo Decision, not so long ago, and they really struggle with the idea of television being sent over computers, which is something that I think a lot of millennial or gen X or gen Z cohorts would be super comfortable with. And I think the question there is going to be, how do courts see how this technology interfaces, how do they weigh the benefits of opening up broader access to copyrighted works specifically to de-bias algorithms and whether they think that that's a fair use. And as I talk about in my paper, I think that it ultimately is.
Lauren Prastien: Interpreting and maybe even modifying copyright law is complicated. However, when it comes to moving past certain problematic data sources and studying the biases inherent to AI systems, it could end up being one of the more promising places to look. But in the meantime, this was Consequential. If you like what you’ve heard, let us know in a review on Apple Podcasts, and we’ll see you in two weeks.
[music]
Lauren Prastien: Consequential is produced by the Block Center for Technology and Society at Carnegie Mellon University. The Block Center was established to examine the societal consequences of technological change and create meaningful plans of action. To learn more about Consequential, the Block Center and our faculty, you can check out our website at cmu.edu/block-center or follow us on Twitter @CMUBlockCenter, all one word.
This episode of Consequential was written by Lauren Prastien and was produced by Eugene Leventhal. Our executive producers are Scott Andes, Shryansh Mehta and Jon Nehlsen. The music you’ve heard throughout this episode was produced by Fin Hagerty-Hammond. To learn more about our guests and see the sources we referenced for this episode, visit consequentialpodcast.com.
S3 E6: Is the presence of a human enough to regulate an AI decision-making system?
Lauren Prastien: So, I was watching the HBO series Westworld, and there was this one scene that sat kind of strangely with me. In it, the board of a company is about to make a major decision and, well, here, just listen:
Charlotte Hale: He already stipulated a machine shareholder as proxy and the machine and I are in agreement. Aren’t we?
The Machine: Proposition approved.
Charlotte Hale: Then it’s decided.
Lauren Prastien: To which a member of the board says:
Board Member: You’re letting an algorithm decide the fate of the company?
Lauren Prastien: Which is, yeah, a question that merits exactly this reaction! And to be fair, Westworld is speculative fiction, emphasis on the fiction. But, as I have done in the past, I ended up badgering a member of the faculty here at the Block Center to see if this was at all realistic. Namely, I turned to David Danks, who you might recall from our first season is a professor of philosophy and psychology here at Carnegie Mellon, where he also serves as the Chief Ethicist of the Block Center.
David Danks: So that's unrealistic at any time in the near future. We are a long way off from having systems that have the kind of broad cognitive autonomy that I think would be required for any company or any group to ever give them some kind of a vote.
Lauren Prastien: That’s a relief.
David Danks: That being said, there's also a sense in which that's already here. Automated trading systems on Wall Street conduct huge amounts of trades without any human okaying that decision. There is at least some reason to think that there are significant groups on Wall Street that are making decisions in their votes - you know, they have voting rights and various companies where they own large amounts of stock - and those votes are at least in part driven by algorithmic decisions.
Lauren Prastien: The key phrase here is “in part.” And what’s the other part you might ask? That’s a human. But just like well-intentioned advice, there’s no guarantee that the human involved will understand it, agree with it, or even use it. So, then what? From the Block Center for Technology and Society, this is Consequential. I’m Lauren Prastien
Eugene Leventhal: And I’m Eugene Leventhal. Today, we’re talking about human-in-the-loop AI. What does it look like, how do humans respond to AI-generated decisions and judgements, and what would regulating it involve?
Lauren Prastien: Like David Danks said, algorithms do make decisions, and they are often involved in business processes. From automating trade on Wall Street to selecting ads for your social media feed to determining your likelihood to repay a loan. But unlike Westworld, they aren’t some totally cognitively autonomous machine making a deciding vote. To understand what this all looks like in a practical sense, we spoke to Sumeet Chabria. He’s the Head of Global Business Services at Bank of America.
Sumeet Chabria: At Bank of America, AI is really grounded in client servicing opportunities or making the internal processes more efficient.
Lauren Prastien: In his role at Bank of America, Chabria and his team are responsible for business, technology and operations capabilities, some of which involve AI.
Sumeet Chabria: AI has tremendous potential to change the world for good if done right. AI gives us the ability to take vast amounts of data and produce forecasts, risk assessments on changing variables to understand the impact. It is a great opportunity for fast, worldclass risk management, a great opportunity to get insights on a vast amount of data, to build correlations that we could not have found without AI. You could find those hidden patterns. It could also do great in customer service, augmenting what people do
Lauren Prastien: For instance, Bank of America has an AI-driven virtual assistant called Erica, which answers customers’ questions and provides insights and guidance on managing your finances. So, she’ll tell you how much you spent on groceries last month. Or, she can alert you if there’s been a transaction that’s posted twice to your account, and help you resolve it. But Erica is not the only space where AI is implemented at Bank of America or even in the financial sector in general.
Sumeet Chabria: Then there’s efficient processes. You know, where can we drive operational excellence across our businesses? Then the category of safety for clients, managing their money and their data, things like fraud detection. Research, improving the accuracy and timeliness of market insights. And lastly, small things like incident management and technology. Can AI help us proactively, uh, find an incident or fix an incident before it happens?
Lauren Prastien: According to Chabria, Erica and these other implementations of AI at Bank of America are basically driven by what the customer might want and how the use of AI would affect the customer:
Sumeet Chabria: The responsibility to me means several things. First is really being customer-focused and being customer-led. And that really means deeply understanding what the customer wants. What is the consequence of a solution to the customer? How is the customer going to be impacted? Second It's about being process led, making sure that the business process dictates the solution that you employ. Which again, means understanding the business need and the process that runs that need and if the AI fits into the solution or not. There’s a difference between can do and should do clearly, it also means having rigorous procedures, policies, standards, governance, frameworks, that's a must in banking.
Lauren Prastien: In addition to the regulations that the banking industry falls under the purview of, the use of AI in these contexts is also mitigated by the presence of a human. Or, not just a human. Many different humans, at many different stages of the process.
Sumeet Chabria: We have a diverse team involved in building our systems. That means diversity at every level: in thought, in style, age, sexual orientation, gender, race, ethnicity, culture, and experience. Over 50% of our employees are female. Over 40% people of color. That is a diverse workforce that is involved in building our systems, testing it, training it, and actually using it daily. Because when you use systems or the diverse workforce, you get the right feedback loop. And when your pair that all of it, the right risk culture, identifying, escalating and debating risks and issues, that's the way you have diversity work to mitigate any risks.
Lauren Prastien: According to Chabria, having input like this is key, especially considering who all of this technology is actually for.
Sumeet Chabria: For this to work, we - people, humans - and AI need to collaborate to amplify the outcome. There's always a greater outcome or a better outcome, or we can get more value, if we do collaborate. It's not A versus B. It’s A and B. We both have to work with each other. AI doesn't serve AI. AI serves people, and the human interaction feedback to AI is critical for it to be successful and sustainable, to be successful longterm.
Lauren Prastien: So what does this kind of feedback look like? We’ll talk about that in just a moment.
[break]
Lauren Prastien: When we talk about human-AI teaming or having a human in the loop, what we usually mean is that there is some kind of algorithm making a determination about something and then a human doing something with that information. Algorithms make those decisions based on past data, usually from decisions that humans themselves made. So for instance, an algorithm that would determine whether or not someone is likely to default on a loan will use data from past lending decisions. Like our example a few weeks ago, an algorithm programmed to evaluate paper submissions to a conference would filter out those papers with improper sample sizes or irrelevant topics. Or an algorithm programmed to determine the ideal school bus routing schedule would quickly calculate every possible route before narrowing down the most ideal options based on, say, budget, environmental impact, and convenience to students. What these algorithms are doing is performing a faster analysis than what a human could reasonably accomplish in a given period of time, and in many cases, being able to more thoroughly look at all the past precedents and cases and distill them down into something workable.
And then that goes to the human, who decides to do something with that. So, the lender will see the likelihood of that person defaulting, and then make a decision based on both the algorithm’s input and their own expertise. A peer reviewer would look at the papers the algorithm had determined had an appropriate sample size and relevant topic. The school board will look at the algorithm’s recommended bus route and accept it, alter it or reject it.
In this way, humans are important. They’re the ones that are impacted by these algorithms, and they’re the ones keeping these algorithms in check.
Eugene Leventhal: Wait, Lauren.
Lauren Prastien: Yes, Eugene?
Eugene Leventhal: I thought it was the algorithm that keeps the human in check.
Lauren Prastien: No. It’s the human, because, you know, humans have emotions and AI are emotionless, data-processing, mathematical functions that don’t really understand how the world works.
Eugene Leventhal: Exactly, humans have emotions! They are emotional! And sloppy! And biased!
Lauren Prastien: Yeah, AI is biased, too.
Eugene Leventhal: Yeah, because of humans.
Lauren Prastien: If this silly, overacted performance of an argument sounds familiar to you, it’s because these are two really common narratives that come up in discussions of human-AI collaboration. So, I asked David Danks if there was any validity to either of these.
David Danks: I think that the narratives both have an element of truth. That's why they linger around. But they also both overstate the benefits and flaws of each. Machines are objective in the sense that they...typically algorithms are just sort of pure mathematics. There's not anything emotional about them. On the other hand, that mathematics reflects what the systems are being trained and taught is important in the world. And so in that sense, they're not objective. They are valuing some things over others. For example, they might value accuracy of a diagnosis over equitability of access to healthcare resources. So they're objective, but in a very special way. Humans are able to recognize unusual cases and really come up with incredibly clever solutions to hard, what we call wicked problems. But we also sometimes - to use the old adage - sometimes mistake the sound of hooves for zebras when it's actually just the horses we see every single day. Uh, it's an old saying in the medical profession that just because you hear hooves, you shouldn't necessarily think that it's zebras. It's probably just horses if you're here in the United States, for example.
Lauren Prastien: I actually love the clarification here of the fact that this adage works in the United States. Because, yeah, this idiom is actually context- and location-specific. There are parts of the world where if you’re hearing hooves, you actually might be hearing zebras. Which, like Danks said, is the benefit of the human: they can spot the unusual cases. The outliers. The zebras, if you will. Or, the horses, depending on where you are.
David Danks: When we think about what algorithms and machines are really good at, they're good at doing the exact same thing - perhaps it's a complicated function, but it's the same function - over and over and over again. They're good at sustained vigilance. Being able to pay attention all the time, even when they're doing the same tasks. And when we think about what humans are good at, we're good at dealing with sort of the opposite of those. The complement of those. We're good at recognizing that this is a special case. This isn't like that thousand instances that I've seen before. We're good at recognizing something's a little bit anomalous here. And so I need to dig deeper. I need to pay a bit more attention. And so in that sense, I think the reasons that we want humans is because we think the world is complicated. The world is weird. The world has surprising, unusual things happen in it. And humans are much better at flexibly and quickly adapting to a changing world or to a surprising world than the machines are.
Lauren Prastien: To really understand this, it’s important to clarify where and why a human might receive algorithmic input on a decision they’re making. Human-AI decision making isn’t as much of a factor in really large scale, generalized contexts that don’t have huge amounts of variability. You’re not going to see a person weighing in on every single choice an automated spam filter makes or those automated trading systems that David Danks mentioned earlier in this episode. Instead, humans are a thing when context matters and there’s usually some complexity, like how switchboard operators still exist for places that receive huge, context-dependent calls like hospitals, but they’re not a thing anymore for when you call your neighbor. A machine just does that. Or how a plane spends most of the flight on autopilot, but you’ve still got a human in charge of landing and takeoff.
Things get more complicated in those examples I gave earlier of the lending decision, the peer review decision, and the school bus schedule. Because these are small-scale decisions, or case-by-case might be a better way to put it, with large-scale consequences.
And while each of these components - the AI and the human - bring their respective strengths to the table, they can each go wrong and keep each other in check in very different ways. To understand this, we spoke to Maria De-Arteaga. She is a professor of Information, Risk and Operation Management at the University of Texas - Austin. There, her work focuses on algorithmic decision support, and she’s written a paper on the role of humans in the loop in the case of erroneous algorithmic scores, or when the AI gets it wrong. Because, yes, sometimes the algorithm gets it wrong.
Maria De-Arteaga: Any algorithm is going to make mistakes. So an important element when we're thinking about having a human in the loop is thinking about the algorithm as a decision support rather than a tool for automated decision making.
Lauren Prastien: In her work, De-Arteaga looked at what was happening when human experts were given the option to accept or reject risk scores generated by an algorithm. These risk scores were intended to supplement the experts’ own judgments on a given case, rather than automate out expert altogether, which is a pretty important distinction to make.
Maria De-Arteaga: So when we think about a human in the loop, we think about the fact that the algorithm is supporting this human and the task of the algorithm is precisely to provide decision support if not to provide the best possible predictions.
Lauren Prastien: Essentially, the risk score generated by the algorithm was just one tool in the toolbox, and De-Arteaga was interested in seeing if humans actually took a unilateral approach to implementing algorithmic feedback, or if they were functioning on a case-by-case basis.
Generally, there were two ways for this more unilateral approach to manifest itself. The first is automation aversion, which is when the human is like, “I am an expert and I have no interest in taking advice from a robot.” And the other is automation bias, when the human is like, “clearly this algorithm knows better than I do, it’s using data and cold hard facts.” Essentially, it’s those two narratives that Eugene and I pretended to argue about earlier in this episode. But according to De-Arteaga, things are actually a little less polarized.
Maria De-Arteaga: In this paper, we found that in this setting, the humans were not falling in either of these extremes, but were instead able to identify cases where the algorithm was making a mistake and ignore the recommendation. And that is one of the key elements of why it is important to have a human in the loop, that is any algorithm will be making mistakes. And even if the algorithm is not making mistakes, the algorithm is solving a very narrowly defined task that the human can then put into context for broader, more complex societal goals.
Lauren Prastien: In the words of my favorite meme, we live in a society. Algorithms do not live in a society. They exist in one and they are impacted by it, but they don’t live in it. Humans do.
Maria De-Arteaga: And in that way, it may be better to talk about algorithms in the loop systems rather than humans in the loop systems.
Lauren Prastien: The fact that the human experts in De-Arteaga’s study were less likely to follow those recommendations that actually turned out to be erroneous does feel really encouraging. It means that we do have an instinct for those special cases, and we won’t just blindly follow what the algorithm suggests to us. But it does swing the other way as well. Here’s David Danks again.
David Danks: There've been a number of studies looking at human-machine interaction that have shown that if you just allow the human to override the machine whenever they want, to take the algorithm’s judgment and say, “yeah, I don't think so,” then in fact, the humans systematically will tend to over-override the system. They will override the system far more frequently than they should in most settings when you're dealing with expert humans. And so I think that shows we sometimes have an overconfidence or a certain amount of hubris in our ability to recognize whether something really is an unusual case.
Lauren Prastien: Like I said earlier, algorithmic input is kind of like advice. Sometimes, you take it. Sometimes, you don’t take it because you don’t agree with it. And sometimes, people aren’t really looking for advice at all, they’re just looking for permission. Notably, when it comes to algorithmic advice, so to speak, sometimes humans take it, but they don’t really understand how the algorithm reached that conclusion in the first place. And sometimes, they don’t take it exactly because they don’t understand how that algorithm reached the conclusion.
David Danks: I think right now one of the big questions that's being asked by a lot of different groups is what is the role of explanations and of transparency in the ways that humans regard the outputs of algorithms? So if I understand more about why the algorithm gave the predictive judgment that it did, if I understand more about how the machine works, does that actually change my willingness to follow the judgments of the system? Does that change how I think about or perceive those outputs?
Lauren Prastien: According to Danks, this is still a growing field of scholarship.
David Danks: So we know something about what people consider to be a good explanation. We know something about what people consider to be a transparent system, but we don't know very much about how that's really incorporated psychologically into the human decision-making. So I think that's one of the big questions that everybody's looking at right now in part, because it's clearly a place where the technological challenges - how do we build systems that can offer explanations? How do we build accurate systems that are more transparent? - those connect directly into practice. You know, if you can come up with a system that is both explainable and improves human machine teaming, then you can roll that out into a corporate environment really quickly.
Lauren Prastien: The whole transparency versus explainability question is a big one. We’ve even talked about it before on this podcast. But in addition to algorithms being able to clearly explain why they’re right, Danks also sees potential in algorithms one day being able to explain why they’re wrong or alert a person that this is a situation that requires more human judgment.
David Danks: I think one of the big questions that we're struggling with and starting to come to grips with as a community is how do we get algorithms that recognize that they shouldn't be trusted? So if I build an algorithm, most predictive algorithms, they'll give you a judgment. It might be a terrible judgment. And we might even have a confidence score that sort of signals whether the system thinks that its own judgment should be trusted. But we haven't, I think, really understood what happens when, for example, the human figures out that the machine is not doing a good job. How do we close those feedback loops so that we have more, in some sense, self-aware algorithms and systems?
Lauren Prastien: If this sounds super sci-fi and a little too HAL 9000, don’t worry, I was also struggling to wrap my head around this. So, I asked Danks to give an example.
David Danks: So a classic example of this is the Tesla autopilot, where most of the people who have used it, at least anecdotally report, that they have a pretty good feel for when the system will work and when it won't. But importantly, the system itself doesn't necessarily have that same level of awareness, that same level of understanding. So I think a very natural question is how do we start to get that information that the humans have hopefully validated that in fact, the machine doesn't perform as well in certain conditions or contexts, and then get the machine to recognize, “ah, I'm moving into one of these contexts. So I need the human to take over.”
Lauren Prastien: You could see the advantages of an algorithmic system that knows when to tell a human to take the wheel, both literally and metaphorically. Because remember, sometimes humans have a little bit of hubris about this. And there are indeed cases of automation bias, so where the humans will just sort of just inherently trust an algorithm because it’s science.
To follow this thread further, I asked how work in this area might inform regulatory practices around human-AI decision making.
David Danks: I worry that increasing human-machine teaming is actually going to slow down and impede what we might think of as progress towards better regulations. And the reason is because I think as we see increasing levels of human-machine teaming, and as those teams become better integrated and better functioning, there is going to be, I predict, a decreasing interest in worrying about the regulation. Because the view is going to be well, there's a human in the loop. There's a human involved in the decision-making. So we don't have to add a bunch of extra regulation.
And the worry is that the human becomes what the writer Madeleine Elish has described as a moral crumple zone or a regulatory crumple zone. So crumple zones are these parts of cars that readily collapse when you're in a collision to absorb the energy and thereby protect the people inside of the car. And the worry that Elish raises is that what we're risking is that the human becomes the sole locus of legal, moral, social accountability, that even though the human and machine are functioning as a team, the worry is that regulators look and say, “Oh, well, we've got a human to blame. And so we don't have to worry about regulating the machine. The human has to do this.”
Lauren Prastien: Right now, the accountability of AI systems under US law is still in rather early stages, and is really dependent on the specific sector you’re talking about. On a federal level, most AI regulations pertain specifically to defense contexts, aviation and autonomous vehicles, or AVs. On a state level, most AI accountability laws are also restricted to AVs, and according to the National Conference of State Legislatures, roughly 60% of states have adopted some form of legislation concerning autonomous vehicles. But notably, in January 2020, the White House Office of Management and Budget proposed a set of AI regulatory principles on use of AI in the private sector, and many industries are beginning to look at how AI might alter more traditional regulatory practices in areas such as healthcare and finance. However, a lot of this is still in its early stages, and as these technologies advance and become a part of decision-making processes in key sectors, questions of explainability and accountability are going to be complex, but they’re questions we’re going to need to answer.
In the meantime, this was Consequential. If you like what you’ve heard, let us know in a review on Apple Podcasts, and we’ll see you in two weeks.
[music]
Eugene Leventhal: Consequential is produced by the Block Center for Technology and Society at Carnegie Mellon University. The Block Center was established to examine the societal consequences of technological change and create meaningful plans of action. To learn more about Consequential, the Block Center and our faculty, you can check out our website at cmu.edu/block-center or follow us on Twitter @CMUBlockCenter, all one word.
This episode of Consequential was written by Lauren Prastien and was produced by Eugene Leventhal. Our executive producers are Scott Andes, Shryansh Mehta and Jon Nehlsen. The music you’ve heard throughout this episode was produced by Fin Hagerty-Hammond. To learn more about our guests and see the sources we referenced for this episode, visit consequentialpodcast.com.
S3 E7: Why does open source have such a wide gender gap?
Eugene Leventhal: Hey. Have you used open source software today?
Lauren Prastien: The answer might surprise you.
Eugene Leventhal: Not really. The answer’s just yes. You probably have.
Lauren Prastien: If you’ve gone on the Internet today, you’ve definitely interacted with some form of open source software.
Eugene Leventhal: That little lock in the corner of your browser that tells you your website connection is secure? That’s open source.
Lauren Prastien: It’s behind things like online banking, medical records, and the stock market.
Eugene Leventhal: And if you work at one of the millions of organizations that have gone remote, if you’re currently a college student, or you just went to a Zoom birthday party, you interacted with open source software when you downloaded Zoom.
Laura Dabbish: So open source really is behind so much of the software that we rely on today. Nearly all the software that's kind of driving our society is somehow reliant on open source.
Lauren Prastien: That voice you just heard is Laura Dabbish. She’s a professor at Carnegie Mellon whose work looks at computer-supported cooperative work, like open source.
Laura Dabbish: So open source refers to this idea that developers can publish free and public software for anyone to use. So the original source code itself is freely available. Anyone can look at it, but they can also redistribute it or modify it. So that means anyone from like other software developers, but also hobbyists or companies, or even the government. So fundamentally it allows people to reuse other people's code instead of writing it themselves.
Lauren Prastien: Open source is essentially the infrastructure of the Internet. In her report Roads and Bridges: The Unseen Labor Behind Our Digital Infrastructure, writer and software developer Nadia Eghbal said it best when she wrote: “Much like roads or bridges, which anyone can walk or drive on, open source code can be used by anyone—from companies to individuals—to build software.” And that’s the thing: even those key aspects of the Internet that we would consider closed source, like Gmail or Twitter or Facebook, all rely on code that’s written by open source developers.
Laura Dabbish: We don't really become aware of this kind of open source infrastructure until it breaks. Economists kind of talk about it as this digital dark matter, that it's this kind of hidden information that we rely on, or infrastructure that we rely on. And it itself is infrastructure in that it requires maintenance. So if you think about this example of left pad, this package that effectively broke the internet when it was taken down, that was an example of a piece of infrastructure that someone was actively, you know, maintaining and, and it was connected in these hidden ways to so many other pieces of software that we all rely on.
Lauren Prastien: This is a wild story. Essentially, left-pad, like Dabbish said, was just 11 lines of code. And on March 23, 2016, the 28-year-old developer behind left-pad, named Azer Koculu, had removed all of his code from the Javascript registry npm after a disagreement with the messaging app Kik because one of Koculu’s software programs was also called kik. So, Koculu takes down his programs. which shouldn’t have been a big deal, except the problem was that a lot of the Internet was using those 11 lines of code. I’m talking about huge, high-traffic sites like Netflix and Facebook. It would be like if someone didn’t turn on a light switch and then the entire City of Pittsburgh ceased all operations for two hours.
And not only is open source an invisible and foundational aspect of the Internet, it’s also becoming an increasingly vital component of being able to get a job in software development. To paraphrase a tweet from John Resig, the creator of the jQuery interface library, when it comes to hiring, he’d rather see someone who commits to participating in open source software development over an impressive resume. And this is not just one anecdotal example. Research has shown that employers in the tech industry have found participation in open source to signal a candidate’s capacity for commitment to their work and their ability to be a team player. A lot of recruiters also considered that participation in open source spoke to candidates’ character that they would choose to code in their free time and often on a voluntary basis.
But here’s the problem. Open source has a gender gap, and it’s a pretty big one. So what’s keeping women from participating in open source software development? And how would increased participation benefit society as a whole?
From the Block Center for Technology and Society, this is Consequential. I’m Lauren Prastien
Eugene Leventhal: And I’m Eugene Leventhal. Stay with us for this deep-dive on gender in open source software development.
Lauren Prastien: In order to understand the gender gap inherent to the open source community, it’s important to briefly discuss who exactly is working in open source and why they’re doing it.
The development of open source software is usually a collaborative process. It’s a form of crowdlabor, which is a term I’ve used a few times this season to describe things like Mechanical Turk, where people are usually compensated for their work, and Wikipedia, which is entirely volunteer-driven. According to Dabbish, participation on open source collaboration platforms like GitHub lies somewhere in the middle of that.
Laura Dabbish: So historically open source development was thought of as kind of this hobbyist activity that people were really into software development and had this ideological orientation that software should be free, would kind of work on it, put it out there. But it's really transformed. And today there's a great deal of commercial involvement in open source. So, the big corporations like Google and Facebook and Apple have packages or software that they have put out there and that they themselves rely on. They’re, in fact, in many cases paying their employees to work on these pieces of software and maintain them.
Lauren Prastien: So sometimes it’s just a hobby, and sometimes it’s a job.
Laura Dabbish: So they've surveyed the developers who are contributing to public projects and they found of those, 70% of those were employed full-time and a majority of those were effectively doing software development work. So, there's a sense that a large proportion of the people involved in the open source community are in the software development profession. And of those employed contributors, 45% of those were contributing as part of their work. So they were either, you know, contributing to a project that they used or they were directly being paid to contribute to a specific project. So there's this huge proportion of folks that are effectively coming from the professional software development community.
Lauren Prastien: And for some people, it’s also a way to build skills and credentials.
Laura Dabbish: So, folks that are trying to learn programming or learn a certain area of programming or a new programming language. They'll go and contribute to an existing project, , or folks that create something that they personally need or are passionate about putting it out into the world. And so they'll create a project and open source it. And as they see a community response, they'll sort of be involved in continuing it and maintaining it.
Lauren Prastien: So open source is coming from hobbyists and people directly employed in the software and tech industries. And you might expect that, given that tech itself has a rather well-cited diversity problem, that there would be these kinds of issues with the demographics of who makes open source as well.
Laura Dabbish: According to the Bureau of Labor statistics, 26% of software developers are women, and less than 5% are Black. So, that then leads to this even worse representation in open source.
Lauren Prastien: Like Dabbish just said, the open source community is even less diverse than the tech industry overall. Surveys have shown that open source has lower representation of women, people of color and members of the LGBTQ community than the tech sector in general. There’s actually a lot to unpack with this, and a lot of nuance that especially comes into play that is specific to why, say a woman feels discouraged as to someone who isn’t straight. There is some overlap, but because Dabbish’s work focuses on gender, we’re also going to focus this episode on the gender gap in open source.
But let me say this, with regard to gender, and beyond the scope of gender, the lack of diversity in open source is particularly disappointing when you consider that the combination of a set of diverse perspectives is basically baked into the ideals of open source itself. Or, at least, ideally it is.
Laura Dabbish: In open source, the idea is fundamentally that by being open and letting in a diversity of contributors, you're going to create a better product, or piece of software, right? That the diversity of perspectives and the possibility of having more people involved is going to improve the output. So if you don't have an environment where it's welcoming to all kinds of people that basically cuts that off.
Lauren Prastien: We have talked quite a bit on this podcast about how involving a diversity of perspectives on a given project, and particularly anywhere AI is involved, is pretty crucial. For a number of important reasons, both in how the technology is made and how it performs in the real world. With regard to the former: a team’s collective intelligence is actually higher when that team is diverse, and that applies whether that team is a group of coworkers sitting at desks a few feet away from each other or a set of people from all over the world collaborating over the Internet.
To explain this in more detail, here’s Anita Williams Woolley, who you may remember is a professor of organizational behavior and theory here at Carnegie Mellon.
Anita Williams Woolley: There are a variety of jobs and opportunities available in these online settings that perhaps women are not having access to because of their comfort level or their choices or their perceptions of whether or not they're welcome. We do find, as some of our research shows, that collaboration in these settings is just as important, as it is in organizations. And so these settings would also benefit from the involvement of women.
Lauren Prastien: Included among those settings was collaborative software development. In one study, Woolley and her team looked at people working remotely in a software competition, and what factors contributed to the team’s ability to exhibit a high level of collective intelligence. So, this was more or less mimicking the setting you’d expect for open source, which isn’t exactly a competition, but does feature teams of people working remotely to develop software. Interestingly, she found those teams of people working remotely would exhibit collective intelligence in similar ways and for similar reasons to what you might expect from traditional teams in the workplace. So, teams with members with higher social awareness exhibited higher collective intelligence. Teams with a greater diversity of perspectives exhibited higher collective intelligence.
There was one indicator of high collective intelligence that was unique to those teams working in a remote, open source environment, and it’s now one of my favorite words:
Anita Williams Woolley: They exhibited a pattern we called burstiness, where people would quickly respond to the communications of their fellow team members.
Lauren Prastien: Burstiness! Those collaborative software development teams with high collective intelligence got bursty, experiencing periods of high productivity, innovation and communication and ultimately producing better software.
In addition to exhibiting burstiness, diverse teams are also usually better prepared than homogenous teams. Here’s Dabbish again:
Laura Dabbish: There's also some evidence that people on diverse teams prepare more thoroughly since they know they might get challenged from a different or an unanticipated point of view, and they can actually think through those.
Lauren Prastien: And in addition to its impact on the way the team works together, having a diversity of perspectives just makes a better product that serves a greater number of people.
Laura Dabbish: With non-diverse teams, the bias that they might have can get embedded into the design of systems themselves. So there's nice work now on algorithmic bias that shows that who codes is critically important to the inclusiveness of the technology we create. So Joy Buolamwini’s work showing the inability or difficulty of facial recognition technology to recognize black faces or faces of people of color, because it was developed by and tested on mostly people with lighter skin tones. That’s kind of an example of that. So there's this opportunity of if enhancing the inclusiveness of the technology itself.
Lauren Prastien: Of course, there is a flip side.
Laura Dabbish: There's a potential for, for conflict and there's a potential for negative interactions, gendered interactions or interactions that might make certain people feel less comfortable.
Lauren Prastien: We’ll talk about that in just a moment.
[break]
Lauren Prastien: A hard pill to swallow is not just that representation of women and minorities in technical fields is disappointingly low, but that it’s also getting lower. There was actually more gender diversity in computer science fifty years ago than there is today, and coding was basically considered women’s work, which, not to open a whole can of worms, also had its own issues. But it’s worth noting that back in 1967, James Adams, the director of education for the Association for Computing Machinery, told Cosmopolitan Magazine, "I don't know of any other field, outside of teaching, where there's as much opportunity for a woman." Women in computer science have done a lot of the foundational work of the field itself. They put us on the moon, they gave us domain naming schemes - so think, like .com and .org - and they were actually the early pioneers of the concept of open source. Today, it’s a little more bleak. Women are still making incredible breakthroughs in computer science, and I do not want to downplay that. But as Laura Dabbish said earlier in this episode, only about 26% of software developers today are women. And according to the National Center for Education Statistics, women receive only 18% of all computer science-related degrees in the United States, and the rates are particularly low for women who aren’t white. Meanwhile, back in 1984, women were 37% of all CS bachelor’s degree holders.
And here’s another hard pill, sorry, lot of pills today: job opportunities in the field of computing are growing at a faster rate than is possible to fill them. By 2026, there are expected to be 3.5 million computing-related job openings in the United States, but only 17% of those jobs could be filled by the expected number of computing bachelor’s degree recipients in the US. So it’s not that there isn’t enough space, it’s that it’s not being filled by women and people of color, or anyone, for that matter.
There are a lot of different reasons for this, and a lot of different places to target, from things in early childhood education to dynamics in the tech industry. But one area that Dabbish has looked at is how the open source community - which, like we’ve said, can be a pipeline to a career in software development - can help or hinder women’s advancement.
Laura Dabbish: So our research on the issue of gender representation and open source, where less than 5% of the contributors are women, has really focused on how are people becoming core. So a lot of the previous work had focused on that initial contribution experience. So, what happens when you try to submit a code change to a project? And we wanted to see what are the pathways by which people become core contributors and how those experiences differ for men versus women?
Lauren Prastien: Real quick: in the world of open source, being core is short for being a core contributor to a project. Core contributors are also sometimes called maintainers, and their role is pretty extensive. They do everything from maintaining security within the software to corresponding with users of the software to troubleshooting problems to onboarding new contributors to soliciting feedback, among many, many other things.
So Dabbish wasn’t just looking at what gets someone interested in open source, but what ensures that people who are interested in open source stick around and what aspects of the open source environment itself might force otherwise interested participants out.
Laura Dabbish: So we did deep interviewing with a set of men and women who are core contributors to what we were calling digital infrastructure projects within the PI ecosystem, and really discussed their participation history and their experiences. We also have been looking at the GitHub trace data to see how with different projects, their environment might influence the rate at which women join. So one of the biggest insights we got from our work was really the value of direct ties to other members in that joining process. So for both men and women building relationships with other people on the project was something that made people stick around and sustain contribution for the women. Interestingly, invitation was instrumental in their joining process. Um, so actually being invited into the project contribute, and direct connections to other members, provided a safe space for asking questions without looking stupid or receiving mentorship. And that helps sustain contribution. We also saw that women in our sample were more motivated over time to increase their contributions by serving users. So they were actually more invested in helping support that community around the project and the people whose code was dependent on this project's code. They were also more motivated by connection to the community. So this idea that the developers behind the project are a community and not just a set of random strangers contributing code but are all invested in working on something together.
Lauren Prastien: This notion of community was a really powerful part of what kept the women that Dabbish interviewed involved in open source. And by extension, when women didn’t feel as though they were part of that community or weren’t meant to feel welcome, that’s where things also broke down.
Laura Dabbish: The women that we talked to faced unique challenges around visibility pressures. So being the only woman on a project or very rare in the environment, they were very aware of that. They also face challenges around excessive scrutiny on their contributions, perhaps as a function of that visibility, and fear of looking stupid, which sometimes prevented them from asking questions, or feeling like they needed to have a certain level of expertise before they could ask a question, especially publicly. They also had to deal with sexist comments or remarks, or sexualized language.
Lauren Prastien: Being a woman on the Internet, just in general, can be a real minefield. Especially if you’re the only woman in the room, or you know, on the Slack channel. So how did women manage that? According to Dabbish, sometimes, by pretending they weren’t women at all.
Laura Dabbish: So one of the women we talked to was contributing to open source really because she found she enjoyed it. She found it fun to code. But she was aware of the fact that she was one of the only women on the project. And so very intentionally chose her username in Slack, which was the main space where the developers on the project would talk to each other, but also where new contributors would come in and talk to her so that it was not gendered. So she just used a single letter to represent her name.
Lauren Prastien: I don’t think it’s controversial to say that in this day and age, women shouldn’t have to pretend they aren’t women in order to participate in things. But that also means that there needs to be some recourse for situations where there are people on the project doing things like making sexist comments or using sexualized language. But remember, even though a lot of people are doing open source because it’s a part of their job, this isn’t exactly like a traditional workplace where you can contact human resources and say, “hey, this person is making sexist jokes about me.” In fact, the conduct of participants in an open source project is usually overseen from within, typically under the governance of what is called a code of conduct.
Laura Dabbish: It's been a more recent trend for open source projects to adopt a code of conduct effectively. What a code of conduct is, is a policy document at the project level that states that by participating in this project, you agree to conform to this set of standards of behavior.
Lauren Prastien: I asked if there were any sorts of standards in place for codes of conduct, like if projects were required to have them on certain platforms, or if particular platforms had template ones or rules about what those codes needed to include.
Laura Dabbish: So it's interesting. Projects aren't necessarily required to have a code of conduct, although projects that are associated with foundations or corporations may be more likely to, to have one just because that organization might have a policy about how interactions are supposed to be conducted or they might, their own employees might have, there might be a certain expectations for them. But in large part, the projects themselves make decisions about, you know, whether and how to adopt a code of conduct and what the contents should look like.
Lauren Prastien: In her research, Dabbish found that codes of conduct basically fell into two categories. The first were more cut-and-dry rules. So, no insults, here’s a list of banned words, etc etc. The second was a more values-based code. So, be respectful, don’t be a jerk, this is an inclusive environment. Which sounds really great, but the thing is that respect is a social construct, and enforcement is mostly left up to the maintainers. Which, yeah, there are some cut-and-dry things that are disrespectful and sexist. But what happens in situations that are harder to point out?
Laura Dabbish: One of the women we talked to had this sort of “aha” moment where she had been experiencing a lot of pushback to the contribution she was making on a specific project. Lengthy discussions in comments, that would go back and forth and back and forth. And she kind of took a step back and was asking herself like what's going on in this community because in the other communities, I'm a part of the other projects I'm contributing to. It's just not this lengthy back and forth. And she noticed just the number of comments that she was getting on each contribution here were so much higher than the men that were also contributing to that same project.
Lauren Prastien: Essentially, this woman would submit something and she’d get roughly 35 comments, going back and forth, before it was accepted. And the men on the project were submitting similar things and having them accepted right away or after just a couple of rounds of messaging. Which is understandably really frustrating and feels pretty sexist, but is not as overt as being called a gendered slur. This probably wouldn’t violate a set of rules, and it might not overtly conflict with a project’s values.
But it’s also important to consider that rules aren’t static, and the standards of a community often emerge or gain nuance when something lies a bit outside of their purview.
Laura Dabbish: So through lengthy discussions around maintainers behavior, either sanctioning a user or correcting someone, or kicking someone off of a project in a couple of rare cases that we observed, the community will kind of push back and say, we don't agree with that decision, or there'll be questioning it. And that's when they actually are articulating through discussion, their values or what they really believe behavior should look like on the project.
Lauren Prastien: Of course, if certain aspects of the culture of a community only gets defined when someone breaks the rules, that still means that someone took the impact of that. Additionally, Dabbish also observed that sometimes the pushback occurred to the entire concept of a code of conduct itself.
Laura Dabbish: I would say one thing that's interesting that we observed, that's also mentioned in some other research on diversity in open source is this occasional pushback to adopting a code of conduct, where it's seen as a distraction from writing the code itself. So that there's this idea that people should be able to, you know, behave freely, however they want on these projects. And you're able to stay involved and in case as long as your code is good. So the quality of your contributions are the only thing that matters and how you behave really shouldn't matter. That's sort of one of the interesting kind of pushbacks that that comes up when you look at these things. And there's this question of how do you convince people who see things that way, that it actually, there is a value in being respectful or that you actually are accountable for your behavior towards others.
Lauren Prastien: This is also further complicated by the fact that the work that maintainers do often isn’t just restricted to the open source platform. There’s a lot that happens on Twitter, for instance, where maintainers often advocate for projects, perform recruitment, and conduct other public outreach.
Overall, it’s important to remember that diversity and inclusion doesn’t stop at just putting people in the room. People need to feel welcome there, and as though their contributions are valued and respected. And if we know from research that diverse teams make better products and work together more cohesively, it’s just wrong to say that having to be accountable for one’s behavior is unnecessary effort or a distraction from the work.
Laura Dabbish: If you look at one of the most widely used code of conduct, the contributor code or the contributor covenant code of conduct, they actually talk about having that code of conduct in your project as a signal to potential contributors that you value inclusion and that your project is a welcoming place to be. And from talking to maintainers, we see that they want to grow their project and grow their contributor base to try to take some of the burden off of themselves, have their project be more widely used. It's all of these things that are requirements for project health. So having the code of conduct there, means positives in one way that it might mean more people will join your project, but also means as a maintainer or as a participant that you'll be treated with respect that people won't be insulting to you, that people will not directly yell at you about changes that they need. That ideally will improve the wellbeing for everyone and create a more inclusive and positive climate on projects. So there it's really this idea of how can we encourage respectful and constructive conversations around code. And I do believe that leads to a better outcome in terms of the code itself.
Lauren Prastien: Like I said earlier, participation in open source has become a pretty big stepping stone into a career in software or the tech sector more broadly. With tech already facing its own diversity problems, the lack of diversity in open source further bottlenecks that talent pipeline.
But this isn’t just about software or even the tech industry more generally. The open source model of open, remote collaboration is something that extends beyond the realm of writing code. Given the current climate of remote work in order to limit the spread of COVID-19, it’s not just the future of work, it’s the present. As more and more domains become digitized and more and more workspaces recognize the benefit of allowing the flexibility to work from home, the kind of goal-oriented but loosely coordinated work that defines open source could become a new standard of work. Understanding where people could be ostracized or left behind within this kind of framework means potentially closing gaps in other sectors with issues of diversity and inclusion. In the meantime, this was Consequential. If you like what you’ve heard, let us know in a review on Apple Podcasts, and we’ll see you soon.
Consequential is produced by the Block Center for Technology and Society at Carnegie Mellon University. The Block Center was established to examine the societal consequences of technological change and create meaningful plans of action. To learn more about Consequential, the Block Center and our faculty, you can check out our website at cmu.edu/block-center or follow us on Twitter @CMUBlockCenter, all one word.
This episode of Consequential was written by Lauren Prastien and was produced by Eugene Leventhal. Our executive producers are Scott Andes, Shryansh Mehta and Jon Nehlsen. The music you’ve heard throughout this episode was produced by Fin Hagerty-Hammond. To learn more about our guests and see the sources we referenced for this episode, visit consequentialpodcast.com.
S3 E8: Language, Power and NLP
Lauren Prastien: Hey, Eugene, do you believe in the color blue?
Eugene Leventhal: Oh boy. What’s all this about?
Lauren Prastien: It’s just a question. Do you believe in the color blue?
Eugene Leventhal: Yes, Lauren. I believe in the color blue. It’s a primary color. It is the color of the sky. It is between green and purple on the visible color spectrum.
Lauren Prastien: Yeah, about that. Most languages actually colexify the concepts of blue and green. So in a lot of cultures, especially older ones, blue wasn’t really seen as a color as much as just a shade of green.
Eugene Leventhal: Really?
Lauren Prastien: Yeah, actually, one of the only ancient cultures that had a word to describe what we now understand as blue were the Ancient Egyptians, and that might be because they were also one of the only ancient cultures that knew how to make blue dye.
Which is so funny to me when I think about the fact that idiomatically, the English language loves to treat the sky being blue as an example of an absolute. Like, if someone asks you a question that seems kind of obvious, you might reply, “I don’t know, is the sky blue?”
Eugene Leventhal: I guess it really depends on who you’re asking.
Lauren Prastien: Exactly. So yeah, there’s a lot of really cool scholarship on this, but to boil it down: the way we perceive and interact with colors in our world has so much to do with language.
Eugene Leventhal: But can’t that basically be said for the way we interact with just about anything in the world?
Lauren Prastien: Oh. Totally. Language and categorization are really powerful concepts, and they’re not objective. Like the concept of the color blue. But these aren’t just aesthetic choices. There’s power in giving things names, and by extension, giving them legitimacy. And there’s power in saying that something belongs to one category over another category. And there’s power in deciding which terms we use to define a specific concept.
So think about the difference between the terms secretary and administrative assistant. Technically, these describe the same job. But based on our cultural assumptions, secretary has a more feminine and subordinate connotation, whereas administrative assistant has a more official connotation and more effectively describes what the role actually does. Same thing with the difference between calling someone a stewardess versus calling them a flight attendant.
And to that end, language is also so elastic and it changes over time. Like how terrific used to mean something absolutely terrifying, but now means something really awesome. And how awesome now means something really cool, but it used to mean something really horrifying or something that would inspire reverence. Or my favorite, decadent, which used to describe things that were in decline or a status of decay - like, decadent, decay - but now we just use it to describe really good chocolate or really cozy duvet.
Also, not every region that speaks the same language uses that language in the same way. Like how in the United States, saying “move your fanny” sounds like something kinda silly that your grandmother might say to tell you to get off the couch. But saying something like that in the United Kingdom means something really different.
And again, all of these words and idioms and categories are things we made up to describe specific trends or patterns or behaviors that would allow us to understand the world and communicate that understanding to others. Whether it’s describing the color of the sky or explaining someone’s job or discussing both the end of an empire and milk chocolate with one all-encompassing word, it’s hard enough for humans to say what they mean most of the time. So, when it comes to computers understanding what exactly we mean when we’re talking to them, it’s even harder. And sometimes, we might not like what we hear when they repeat what we’re saying back to us.
From the Block Center for Technology and Society at Carnegie Mellon University, this is Consequential. I’m Lauren Prastien.
Eugene Leventhal: And I’m Eugene Leventhal. Today, we’re talking about the relationship between language and artificial intelligence, specifically natural language processing or NLP . So stay with us.
[break]
Lauren Prastien: Hey, I want to play you something really quickly.
Tom Mitchell: So, if we want to talk about AI, I think the very best way to begin is to think about the ways in which we’ve seen really dramatic progress in AI over the past ten years, it’s hard to recall because we get so used to the technology. But if you think back to the year 2007, when the iPhone came out, do you remember that? You could not talk to the iPhone.
Lauren Prastien: The voice you’re hearing is Tom Mitchell. He’s professor of computer science at Carnegie Mellon University, and the Lead Technologist here at the Block Center. And this is from a talk he gave back in early 2019.
Tom Mitchell: You could not talk to your iPhone! Speech recognition did not work - but today a little bit over ten years later, computer technology for speech recognition has reached roughly the level of humans in transcribing spoken speech to the equivalent written form.
Lauren Prastien: The rest of the talk - called “How AI Changes Work and What We Should Do About It” - is available on the Heinz College at Carnegie Mellon University’s YouTube channel. But something that I wanted to focus on is this point that computers can recognize human voices at about 95 percent accuracy, which is the threshold of accuracy that most humans achieve. To further put this in perspective, back in 2013, speech recognition was only about 75 percent accurate.
Let me just quickly clarify, though, speech recognition isn’t new as a field, it’s just only recently become a really democratized technology. Even back in the late 90s, computers had vocabularies of more than 65,000 words, and algorithms could perform continuous-speech recognition, just not in commonplace or commercial settings. But nowadays, we run into situations like what happened back in 2017, when a news anchor went on television and reported on a little girl asking Alexa to order her a dollhouse, only for other people’s Alexas to overhear the news anchor saying, “Alexa, order me a dollhouse,” and because Alexa is listening, suddenly, they also had a dollhouse on its way to their homes.
Sorry, by the way, if I just accidentally ordered you a dollhouse. Or, I don’t know, you’re welcome, maybe, if I just made self-quarantining a lot more fun for you.
But anyway, there’s a difference between listening and understanding. And you might argue that Alexa was absolutely listening, but she didn’t really understand what was going on. And it’s so easy to take for granted that this requires a lot of work to pull this off. Teaching computers to understand human language, spoken or written, is no easy feat. Especially the nuances of human language. Be it in your iPhone understanding what you mean when you say “hey Siri,” or a search engine understanding that you mean A Quiet Place when you try to Google “the movie where they can't talk because of aliens,” or a text-to-speech system on a GPS knowing that that one city in Michigan is pronounced “Ip-Sil-An-Tee” and not “Yip-Sil-Ante.”
All of this falls under an area of scholarship known as natural language processing, or NLP. Put simply, NLP is the branch of artificial intelligence that helps computers understand, interpret and manipulate human language. In addition to those examples I just provided, NLP also underlies things like automated translation services, content categorization, automated document summarization, and sentiment analysis, which is when an algorithm is able to analyze a piece of content, like, say, a review of a product, and determine the emotional state of the person that produced that content. So, NLP doesn’t just produce language, it also analyses the nuances of human language.
To better understand this, we spoke to Alvin Grissom II, a computational linguist at Haverford College whose work looks at language processing in AI and machine learning systems.
Alvin Grissom II: There's almost nothing that humans do, which doesn't make use of language in some capacity. Whether you're giving someone directions, reading a sign, writing an essay, telling your doctor where the pain is, negotiating a treaty, you know, whatever it is. If I asked you to go through an entire day or even an entire hour without using any language at all, like, could you do it? Maybe, maybe not.
Lauren Prastien: And language doesn’t just communicate the information the speaker or writer wants to impart. It often conveys a lot more than that.
Alvin Grissom II: Language provides a window into our cognitive states. So, we can't for the most part read people's minds, but language allows us to relay a noisy, imperfect version of what's going on in our own heads. Even when we're not consciously aware of it in some cases.
Lauren Prastien: And that is where computational linguistics comes in.
Alvin Grissom II: Computational linguistics allows us to use technology to examine or deduce many sorts of scientific analyses more efficiently. So, for example, if you want to examine some phenomenon, some psychological phenomenon, and you want to use language, then you can use computational tools to try to examine the data with some more sophisticated - potentially maybe, maybe more simple - techniques, if you have a lot of data.
Lauren Prastien: You might remember a few episodes ago, when we looked at the impact of the Enron corpus on modern AI systems, and the fact that they had so many of these publicly available emails meant that they had an abundant resource to train spam filters, understand how gossip spreads, and develop predictive text - which is also, by the way, another example of NLP. But this can also work in reverse. Just as researchers can take these large corpora to develop AI systems, they can also use AI systems to better understand what’s going on in these large corpora, and from there, be able to say something important and meaningful about society itself. Things like, oh, I don’t know...how we talk about football players, maybe?
Alvin Grissom II: Mohit Iyyer at UMass Amherst was interested in this. He's one of the authors on the paper. And at the time, I think he was, he was following coverage of Kaepernick's kneeling protests and the subsequent blackballing that came down on him and I think this piqued his interest in racial bias in sports. And I think black people in general already know intuitively that people talk about us differently in sports and other contexts, but it's often more like a thousand small cuts that creates a kind of emergent milieu of subtle dehumanization. It's the kind of thing that makes it difficult or impossible to point to one explicit example, except in the most egregious of cases. So Mohit and I were discussing it. And it sounded like an interesting idea. So I got involved.
Lauren Prastien: After looking into previous social science research that examined gendered and racialized language use in sports commentary and news coverage, Grissom noticed something about everything that had been done up to that point.
Alvin Grissom II: So the previous research in this area was out of necessity on a smaller scale. So we thought it would be great if we could do a massive study on decades worth of commentary to see whether it comported with previous findings. So we created a Corpus which anyone can download. It's called the FOOTBALL corpus of commentary across several decades, annotated by perceived race non-white or white.
Lauren Prastien: FOOTBALL combines data gathered across the span of six decades, using 1,455 broadcast transcripts. These transcripts contain more than 270,000 mentions of more than 4000 individual players. So, if you want a thorough, large-scale analysis, it’s FOOTBALL.
Alvin Grissom II: So we found that white players are more likely to be referred to by their last names, in offensive positions and nonwhite quarterbacks and wide receivers are about twice as likely to be referred to by their first names than white players in the same position. This is interesting, you can imagine, because one of those is less respectful than the other, or more familiar, you might put it that way. But that's a very stark contrast across such a large data set.
Lauren Prastien: Grissom also used what I described earlier as sentiment analysis. Essentially, taking a list of positive and negative words, and seeing which of these words were more likely to be used to describe white or non-white players.
Alvin Grissom II: So what we found was that the most positive words associated or used to describe, or at least in close proximity to white players were words like calm, cool, smart. For non-white players, they were words like speed, versatile, gifted, natural, athletic.
Lauren Prastien: In other words, the white players were viewed as more intellectual and in control, and the non-white players were described on more physical terms and their talent was viewed as more of a natural ability than a skill they’d honed. Which, by the way, corroborated what a lot of the earlier, smaller-scale studies in the field had previously shown.
Alvin Grissom II: So the default thing that you would expect if this weren't happening is that there would be basically no substantive difference between them. And there are some caveats. So position and race are highly correlated. So for example, we found that quarterbacks are mostly white, but most other positions are largely non-white and these percenages change drastically over time. So in 1970, for example, almost every mention of a quarterback in our dataset was white. So to account for this, we also looked at only quarterbacks and saw similar patterns, but it's worth noting that disentangling these variables is tricky.
Lauren Prastien: At this point in our conversation, I asked Grissom what the larger implications of this research were for the social sciences, which is where a lot of the previous scholarship that his group consulted before beginning this research typically lives.
Alvin Grissom II: Well, I think one way of looking at it is that if you view language as a kind of window into our mind, then it tells us something about something that's going on in our minds. Or at least in the minds of whoever we're examining. So why is it that there is a substantial difference between the language that commentators used to just describe white versus non-white players, right? It's not that we're suggesting that they're malicious or you know, consciously racist. But it speaks to much more subtle concepts and ways of thinking that permeate our society. The assumptions that we have, that we may not even be conscious of. And the kinds of things that you can kind of get a sense of by living it over a time, but it's very difficult to point at a specific example and, you know, prove that this is happening, right? And so that's why we thought that, or at least that's one of the reasons that we thought that examining this over such a large corpus would be useful because a lot of these patterns don't really emerge unless you have enough data to make an argument that it's happening.
Lauren Prastien: The issue is that human language is messy. Not just “do you believe in the color blue” or “is decadent a word for an empire in decline or the best cake in the world” messy. But this kind of messy, because it is a mirror to the subtle and overt values and prejudices of the society that produces it. And while the FOOTBALL corpus or the Enron corpus themselves might not be what trained Siri, they are both examples of how these small, subtle linguistic patterns can add up to larger biases in the kind of corpora that did.
It’s a classic adage in computer science: garbage in, garbage out. And like we’ve discussed in this season, sometimes, all programmers have available to them is garbage. Or, okay, not garbage, per say. But the lived messiness of human existence. Or these cases of a lot of subtle little microaggressions that don’t feel impactful until they’re blown up to a larger scale.
Alvin Grissom II: So let's say we're building a virtual assistant for speakers of English to operate a car. The system was probably trained on some data from certain demographics. So whose English will it work well on? Will it handle regional dialects? Will it handle African American Vernacular English, non-native English? Probably not. I have family members who can't use any of these technologies because the systems just can't deal with their vocal inflections and timbre, even if they code switch to standard American English.
Lauren Prastien: This is a problem that comes up time and again in NLP technologies. This year, a study from Stanford University revealed that speech recognition systems from Amazon, Apple, Google, IBM, and Microsoft misunderstood Black users 35 percent of the time. By contrast, they only misidentified white speakers’ words about 19 percent of the time.
Alvin Grissom II: And so that was a choice that someone or someones made to use a particular approach to tackle this problem or to create this product and to deploy it in that state.
Lauren Prastien: It can be really easy to label this as just an oversight, or the case of incomplete datasets. But sometimes, these choices are actual, conscious, serious choices. Like the anecdote recounted in Ruha Benjamin’s Race After Technology, wherein an Apple employee was told by a supervisor that Siri was designed for a “premium market,” and so it wasn’t necessary to teach her AAVE, or African American Vernacular English, but it should learn Australian, Singaporean, and Indian English dialects. It can be difficult or uncomfortable to acknowledge that these choices get made, just as it can be upsetting to consider that in order to deploy a technology like that, there were decisions made at numerous levels to not test its compatibility with non-white users.
But it’s also important to remember that many natural language systems are not simply built on one dataset and then released into the world. These systems learn and change as they interact with humans. For better, or for worse. We’ll talk about that in just a moment.
[break]
Lauren Prastien: As the name might imply, there’s a learning side to machine learning. Systems are intended to pick up on the information that is being put into them, and based on how users respond to them, adapt to better suit those users’ needs. But like you may remember from, say, that chatbot called Tay that Twitter managed to turn into a racist, sexist menace to the Internet, systems meant to learn from the ways that humans talk can be, uh...not exactly seamless.
One of the places we can see this most clearly manifested is in search engines. And, in particular, search completion suggestion. This is a form of NLP that you’ve probably encountered on a near-daily basis. It’s what happens when you start to type something into a search engine. So, for instance, when I typed “consequential” into a certain search engine, I got the following suggestions: “consequential damages,” “consequential definition,” “consequential synonym,” “consequential meaning,” “consequential ethics,” and oh, hey, would you look at that, “consequential podcast.” I wonder what that is.
But anyway, search completion is a really cool NLP technology that attempts to predict what exactly it is you’re looking for, or present you with options to help narrow down your search to provide a more exact set of results that correspond with what you’re looking for. But sometimes, the ways that search completion functions are kind of troubling. To give us a better idea of what’s going on, here’s Alexandra Olteanu, a principal researcher at Microsoft Research. Though, quick clarification, Olteanu is providing her personal opinion and is not speaking on behalf of Microsoft.
Alexandra Olteanu: Search completion suggestion is one of the earliest predictive text applications. With Google Suggest being launched, for instance, in 2008. And this makes it critical to examine why identifying problematic textual suggestions remains an open problem.
Lauren Prastien: When it came to looking at issues like these, Olteanu seemed like a great person to talk to, because, well…
Alexandra Olteanu: I am interested in understanding when predictive texts or natural language generation systems and applications fail and why those failures may be in some way harmful.
Lauren Prastien: There are quite a few ways in which these kinds of systems can fail. A lot of scholarship in this area has focused on the fact that search completion suggestion, which is also sometimes called search autocomplete, will render suggestion that are either deeply problematic, think perpetuating racism, sexism, ableism, etc. or are linked to disinformation, so for instance, someone typing in climate change and autocomplete suggesting, “climate change is not real.” Which has happened! And, you can imagine, if you’re just someone who doesn’t know all that much about climate change, and you’ve got search engine suggesting that to you, and then you’re following that through and seeing content about climate change not being real, well, that’s pretty bad.
But it’s not that the search engine is a climate change denier. It’s not capable of having those kinds of thoughts.
I asked Olteanu to elaborate on the mechanics actually contributing to these systems in the first place. Because prior to making this episode, I actually wasn’t entirely familiar with what exactly makes search autocompletes...do the autocompleting. And I’m probably not the only one.
Alexandra Olteanu: They vary from system to system and they have evolved a lot over time. But despite that, many still rely on past queries to determine which is the most likely completion or for a user that starts writing a query.
Lauren Prastien: So, one reason someone might be getting the suggestion “climate change is not real,” it’s because a lot of other people typed that in, and the system learned that people - and, perhaps, people whose search patterns resemble yours - wrote that kind of query.
Alexandra Olteanu: Because they rely on past queries, they are very sensitive to the propensity for a given completion to be seen as likely, and that propensity can change over time due to various, let's say news events or just things like seasonality.
Lauren Prastien: By seasonality, Olteanu means that at different times during the year, people are interested in different things. So, for instance, people are probably searching “best Valentine’s Day presents” in late January and early February, and not so much in June and July.
Alexandra Olteanu: They're also sensitive to variations in data quality and availability as a large volume of queries tend to be rare or may have never been observed by the search engine.
Lauren Prastien: So, searching “I can’t taste anything” in the very, very early days of COVID-19 would probably not have turned up a suggested autocomplete related to the coronavirus. But now, when I just typed “I can’t taste anything” into a search engine, it suggested I complete the query with “covid,” now that we have a lot of data and research backing up the fact that losing your sense of taste is one of the most telling symptoms of having COVID-19.
Alexandra Olteanu: Why this is happening is variations in, in data quality and availability or the presence of so-called data voids. It also happens because a lot of the queries tend to not be representative of the entire let's say, user base, but only of a subset of users that are actually likely to issue those queries. But also because oftentimes these systems have to adapt to changes in the propensity of a query to be observed due to news events or seasons or all kinds of temporal aspects.
And the other third aspect here is that many queries also correlate to a certain subsets of users that for instance are interested in given topics or that tend to formulate their queries in certain ways. So I would never, for instance, start the query with the name of a person plus something like is, or are, or should.
Lauren Prastien: Heads up, if you try that, it’s...usually not that great, and it’s where a lot of criticism of search engine autocomplete has focused. In fact, a lot of search engines have made it actually impossible for autocompletes to function with these sorts of queries. Like, autocomplete won’t suggest anything to you if you type in “feminists should” on Google or Bing. It’s like, nope, sorry, not going to help you out there, this road doesn’t go anywhere great. Because, as you might imagine, this is where the hate speech and stereotypes get perpetuated. Because, generally, an entire group of people usually doesn’t all fall under one blanket idea or shouldn’t all do the same thing.
Except it’s really hard to account for every single problematic phrasing that might lead someone to content that’s slanderous, bigoted, or just false.
Alexandra Olteanu: This is difficult for several reasons, including with YouTube, the long tailed and the open ended nature of search and writing tasks, which often means that both of the possible inputs and outputs of the systems are very large, but also due to the sheer diversity of factors that could lead to a certain text generation scenario to be perceived as problematic. And that can be things like who is making that the assessment? What are the peculiarities of a given usage scenario? What are the system affordances? And so on.
All these sort of aspects makes it very difficult to balance between providing this assistive feature to users, as it is known to improve the outcome of their search tasks, and effectively avoiding any sort of adverse impacts like reinforcing existing stereotypes or nudging users towards lower quality content such as misinformation.
Lauren Prastien: There’s the big tradeoff. And it is a huge tradeoff, when you consider that the problem here is that, like Safiya Noble wrote in her book Algorithms of Oppression, search suggestions don’t just reflect our values as a society, they also contribute to shaping them. It might seem like such a minor detail until you consider that the search engine is more or less treated by our society as some all-knowing arbiter and generous provider of information writ large. And according to Olteanu, it’s the fact that these search engines are so burgeoning with content that makes it so hard to try to rein them in.
Alexandra Olteanu: It's difficult to even understand which are the issues you should be looking at, because it's impractical to sit and enumerate all the ways in which the systems may fail. And, and that's because the input is open ended and the output is open ended. And that characteristic alone likely results in a long tail of issues.
Lauren Prastien: So what could be done?
Alexandra Olteanu: We need to examine even our own modeling and data labeling assumptions and reconsider what and how we could incorporate more contextual cues into those processes which could be about, right, who is referenced in the queries, what is being said about them, how the queries are formulated, right. What was the actual input that the user provided and so on, but we also need to think about how we can leverage the variations in data availability or the fact that we know that there are data voids or for certain classes of queries, and try to use those to identify issues that might become more prevalent in there.
Lauren Prastien: Language reflects the society that builds it, and it also shifts and evolves in accordance with that society. And NLP can be an incredible mirror for that, be it in supporting smaller scale social science studies, as Grissom’s work has shown, to helping you communicate with someone in another language while you’re still learning that language. But it can also reflect and further perpetuate the negative stereotypes permeating that society, like you might see in search engine completion. And given that search engines have really become one of our most relied-upon sources of information, this can be pretty troubling. Which all kind of begs the question, if the information is out there and we now have unprecedented resources, is it actually accessible and can we say it’s actually democratized? Next time, we’re going to be looking into this question. In the meantime, this was Consequential. If you like what you’ve heard, let us know in a review on Apple Podcasts, and we’ll see you soon.
[music]
Eugene Leventhal: Consequential is produced by the Block Center for Technology and Society at Carnegie Mellon University. The Block Center was established to examine the societal consequences of technological change and create meaningful plans of action. To learn more about Consequential, the Block Center and our faculty, you can check out our website at cmu.edu/block-center or follow us on Twitter @CMUBlockCenter, all one word.
This episode of Consequential was written by Lauren Prastien and was produced by Eugene Leventhal. Our executive producers are Scott Andes, Shryansh Mehta and Jon Nehlsen. The music you’ve heard throughout this episode was produced by Fin Hagerty-Hammond. To learn more about our guests and see the sources we referenced for this episode, visit consequentialpodcast.com.
S3 E9: Is information democratized?
Eugene Leventhal: Here’s a lofty question: is information democratized in the United States?
Lauren Prastien: Or, okay, here’s a less lofty question first: does the average person walking around today have access to say, the names of all of the US presidents or the location of the nearest gas station or the proper way to fold a fitted sheet?
Eugene Leventhal: There’s a long answer and a short answer to that.
Lauren Prastien: The short, simplified answer is, yeah, basically, they do. And in most cases, it’s because of that one democratized technology we talked about in our first episode of this season: the Internet. And as a quick refresher: a democratized technology is a technology that has become more or less accessible to the general population.
Eugene Leventhal: Like how the more than 2.87 billion people around the world who own smartphones today have regular, routine access to the level of processing power that put humanity on the moon a few decades ago.
Lauren Prastien: So, you have a lot of power in your hands. Literally.
Eugene Leventhal: But while all that might be the easy answer, it’s not the whole answer.
Lauren Prastien: On the one hand, we have put a lot of power at the fingertips of the individual.
Eugene Leventhal: We have decentralized information. We can get information as individuals that we used to have to go to a library for or have to achieve a certain level of education or prestige to access or have to pay for.
Lauren Prastien: But, by the same token, just because the information is out there doesn’t mean it’s always accessible, verifiable, or legible to the general public.
Eugene Leventhal: Which can make a huge difference, like in this situation described by Stephen Caines, a legal technologist and Fellow at CodeX which is The Stanford Center for Legal Informatics, and one of our guests today:
Stephen Caines: If someone who's about to be evicted, for instance, um, let's say that they're working two jobs and they're working really hard, but, you know, they're just not able to make it about, to be evicted that person when they go online to search for resources, they might only set, let's say a finite time of, let's say 30, 45 minutes to find a resource. And if not, they're just going to abandon all hope in a sense.
Lauren Prastien: So, yeah, you might be thinking, wait a minute: didn’t the CDC issue a federal eviction moratorium last September? And, you’re right, they did. But let me ask you a question: if your landlord filed an eviction application between the expiration of the state moratorium and the CDC order, does your eviction hold up in court? What if your landlord says they didn’t receive the declaration and so they’re going forward with your eviction? And who do you call in order to enforce the CDC order if your landlord is still trying to evict you? It’s actually really disheartening to try to look it up. Because is your source reliable? Are you sure? Does it pertain to your state? Is it the most up-to-date information? Are you positive?
Stephen Caines: If you Google, like, “can I be evicted?” for instance, and let's say your zip code, you're going to get a lot of sponsored links before you get any actual content that may apply to you. And even if you do get what seems to be a legal answer, for all you know, it could be tailored and written for another state for laws that were relevant 10 years ago. And so there's the issue of misinformation, both in terms of deliberate misinformation, but then also just simple out-of-date information and things that are just not relevant to the actual searcher that people often fall prey to in kind of this new age.
Eugene Leventhal: So, is information democratized in the United States?
Lauren Prastien: From the Block Center for Technology and Society at Carnegie Mellon University, this is Consequential. I’m Lauren Prastien,
Eugene Leventhal: And I’m Eugene Leventhal. Today, we’re talking about information inequality and accessibility. Stay with us.
[break]
Lauren Prastien: So, information inequality refers to the disparities inherent to accessing necessary information. And in the age of the Internet, it might seem like information inequality would be a thing of the past. But that’s not really the case. To learn more about this, we spoke to Myeong Lee, a professor of information systems at George Mason University, where he directs the Community Informatics Lab.
Myeong Lee: In order to deal with information inequality issues we need to approach this problem in two ways. In one way it is more about like a digital divide and information literacy. These are literacy kind of education or training perspective. And the other side is the structure portions, like, you know, how technology and the distribution of information makes it hard for them to access information in an equitable way.
Lauren Prastien: Lee’s work concerns more the structural side of this problem. Because it’s not just a matter of getting people on the Internet and teaching them how to parse factual, reliable information from misinformation and disinformation - though bridging the digital divide and promoting digital and media literacy are worthy and important endeavors that we have talked about before on this podcast. But there’s another level to this, and it has to do with how that information is gathered and distributed.
To this end, part of Lee’s work looks at something known as information deserts. The concept of an information desert is based on the idea of a food desert, which is a term that refers to a region where people have limited access to affordable and nutritious food. Similarly, an information desert is where people have limited access to affordable and reliable information. This might be in a very physical sense, like the region doesn’t have a library or the library isn’t reachable by public transit.
Myeong Lee: And it is not only in the context of the physical spaces. On the Internet, if somehow a system is not managed well, then information could be transient. It could be easily gone. In that case, an information desert could be created.
Lauren Prastien: Remember, information doesn’t just appear on the Internet, even though it might seem like it does. Think of our examples from some previous episodes. There’s a person updating Wikipedia, for instance. Just like there’s a person updating the official website or news resource that Wikipedia is getting that information form.
Myeong Lee: When nobody provides information to the containers, there are many different kinds of containers such as online communities, databases, flyers, or other types of written forms. If people don't provide enough information to those kinds of available containers, information deserts can be created. And finally when information is fragmented across different sources.
Lauren Prastien: That last point is pretty critical. Because an important distinction is that information deserts aren’t always information deserts just because there’s no information around. If I have to go to seven different websites to figure out if I am eligible for a certain public benefit or to determine how to get support during an urgent crisis, like an eviction, that’s technically an information desert.
Myeong Lee: We know that there are hundreds and thousands of websites available on the Internet, but, you know, physically we cannot have access to them. And in our daily lives, we use only part of the systems. And if information is fragmented, highly fragmented across different platforms, then you know, the systems that I usually use might not have enough information about the topics that I'm looking for.
Lauren Prastien: It can be easy to consider that the Internet would have gotten rid of information deserts as we know them. But, by the same token, the very nature of the internet can make it a lot easier for an information desert to open up.
Myeong Lee: So in terms of the impact of the technology on the creation of information deserts, there might be many different reasons. Uh, one of the reasons might be uncoordinated design at the interface level. When people have access to different websites, if the website design is super bad or complicated, then you know, many people just give up.
Lauren Prastien: And giving up has very different stakes depending on what you’re looking for. Especially when it comes to matters like eviction, mask ordinances, vaccine distribution, or just generally knowing what your rights are, which we’ll talk about a little bit later in this episode.
But first, something else that Lee really emphasized in our conversation on how technology has contributed to the formation of information deserts is the changing nature of the actual technology itself.
Myeong Lee: Several decades ago, information was stored in a magnetic tape and nowadays information is usually stored in the cloud. Cloud servers, right? And because that kind of materiality of information, how they store the data, affects people's search use and management of the information, right?
Lauren Prastien: Like you may remember from our conversation earlier this season with Ben Amaba of IBM, cloud computing did a lot to democratize both computing power and the spread of information. But cloud computing is, to an extent, ephemeral. Which means that it’s a resource that can be distributed, reclaimed and redistributed, but it’s also something that can be overwritten.
Myeong Lee: And this is not only about interfaces. It's also the systems. Many different kinds of systems are created in an ad hoc manner. And these market-driven forces affect the creation of information deserts in an unpredictable way. Because, you know, market-driven forces are kind of unpredictable from a community perspective. And we are, I think the current trend of building different kinds of information systems creates an unintended information landscape from a community perspective.
Lauren Prastien: At this point, I asked Lee to give an example of how this works, and he pointed to the nature of job listings online.
Myeong Lee: If we talk about job information, knowing job openings or not is a critical opportunity issue. And if there's a disparity in accessing those job information, it is a critical issue in providing equitable opportunities to the people, right? So and also because these disparities in having similar opportunities with each other are directly connected to people's socioeconomic wellbeing in the community. So I think information inequality is a super important issue.
Lauren Prastien: Remember, job listings aren’t just in a section of the newspaper anymore. They’re on the Internet. Which, in one regard, is amazing in terms of your ability to find opportunities. But on the other hand, think about the platforms that organize this information - because otherwise, like Lee said, we’d have the kind of disparate or under-updated containers that give rise to information deserts. These platforms often use AI and other forms of data analytics to guide users towards that information, and those platforms, as well as the algorithms they use, are not always infallible. We’ll talk about that in just a moment. So stay with us.
[break]
Lauren Prastien: Knowledge systems, be they search engines or online job portals or Wikipedia, function, in part, by trying to understand user intents when they query for information, like we discussed in the last episode with Alexandra Olteanu, and understanding how to personalize that information to a given user. So, for instance, if I, a person based in Pennsylvania, search “tenant’s rights” or “jobs in finance” and I have my location services turned on, the search engine is going to ideally show me something different than a person in Wyoming who searches “tenant’s rights” or “jobs in finance.” But in order to personalize, knowledge systems need to make numerous judgments about the users querying them, and that gives them quite a bit of power in determining who sees what.
To look at this a bit closer, here’s Asia Biega. She leads the Responsible Computing Group at the Max Planck Institute for Security and Privacy, and her work looks at the complexity inherent to connecting people to information online.
Asia Biega: Knowledge systems operate over huge collections of data and because only a tiny portion of all of this available knowledge can be surfaced to the user, platforms have a huge power to determine what's relevant, what's high quality, what's reliable.
Lauren Prastien: When Biega said this, it made me think of what Noel Carroll considers in the 2014 article “In Search We Trust: Exploring How Search Engines are Shaping Society.” This quote, especially, “digital search engines have, in a sense, a dual control to enable or to constrain knowledge through their search practices, algorithms, and rules. In essence, search engines have the capacity to govern and influence content, ideas, and information to which users are exposed to. And I would argue that extends more broadly to information systems in general, be they the recommendation system on an online shopping platform or the job portal where you’re trying to find your new job.
Asia Biega: Information systems and knowledge systems are complex. And there is so much knowledge about how these systems and the general knowledge ecosystems operate is implicit and is distributed. And it's really hard to understand how these systems operate, even to people who have appropriate backgrounds. And I think this imbalance in the know-how gives platforms a lot of power to influence users in all kinds of ways.
Lauren Prastien: This isn’t an episode about how knowledge ecosystems are making us passive consumers of information or completely destroying society. Give us a little more credit than that! But what I do want to emphasize is that these systems do have the power to influence what information their users are actually consuming and as a result influence user
behavior and decisions. And that is achieved through a strategy that Biega calls nudging.
Asia Biega: Nudging is a tactic to influence people's behavior in a controlled way. And within the context of online ecosystems, it can be a powerful tool and I think it should not be used lightly. But here's one thing I think that researchers and tech creators often underappreciate: Technology is never neutral and especially a system design or an interface that we use to present information to people is never neutral.
Lauren Prastien: Essentially, nudging is the term used to describe the strategy that digital platforms use to guide users’ choices when navigating the interface. In their 2012 article “Beyond nudges: Tools of a choice architecture,” Johnson et al. at Columbia University describe this phenomenon really well by explaining that in these kinds of knowledge systems “what is chosen often depends upon how the choice is presented.” We’re not saying that you’re just a rat in a cage here. But what Biega is saying sounds a lot like what our friend from Wikipedia, Katie Willingham, said in episode 5: that information - and the ways we decide to present it - are never neutral. The fact that the knowledge system has to essentially make assumptions about the user in order to surface the most relevant content and nudge them towards that content means that a lot of stuff can go wrong.
Asia Biega: There are many different forms of bias in knowledge systems, such as search systems and recommendation systems. We have to take into account biases in the way we present information, and that might include the topical diversity or other forms of diversity of the content that is presented. This might also include the diversity of the content creators, who we expose to people who search for information in these systems.
One other form of bias that is really important to take into account when designing the system is the biased behavior of the users who interact with the system. This is important because many systems nowadays learn from these interactions. For example, they take behavioral cues, and these behavioral cues might include click information or, you know, how long people inspect different pieces of information that is presented to them. And the system takes this information and infers which content might be relevant and high quality. So if the way people interact with the systems is biased, and that might include for example different forms of personal and societal biases, the systems are going to learn from these interactions and then replicate all of these biases.
Lauren Prastien: So think back to that job portal example that Myeong Lee gave earlier in this episode. There has been a lot of scholarship on the fact that, for instance, algorithmic ad platforms are pretty biased. Which, unfortunately, kind of makes sense. These algorithms are based on a biased world, and though they’re often employed to ensure that recruiters are connected to the most qualified people for a given job and that candidates see the job they’re more qualified for, the notion of what “qualified” means is based on past human decision-making. So it’s not just the decision of whether or not to hire someone, but the decision of whether or not someone even sees the job listing in the first place at all. Even one of the most notorious cases of “algorithmic hiring gone wrong,” that Amazon hiring algorithm that disadvantaged women, was not about picking between applicants, but about finding promising potential candidates to recruit. And in a recent study, researchers at Northeastern University, the University of Southern California, and the research group Upturn found that targeted ads on Facebook for supermarket cashier positions were shown to an audience of 85% women, while jobs with taxi companies went to an audience that was approximately 75% black. In other words, information inequality - and particularly the kind perpetuated by technology - has an actual impact on society, including reproducing the very biases that were already inherent to this society.
If you’re interested in learning more about this, one really great resource that collects a lot of these findings together is Miranda Bogen’s 2019 article in Harvard Business Review, which is rather aptly named: “All the Ways Hiring Algorithms Can Introduce Bias.”
And, of course, this isn’t just restricted to hiring. This happens with housing, too. And financial services, like loans. So, at this point in my conversation with Biega, I asked what could be done?
Asia Biega: Fixes to the data are one potential solution, but we need to think about these things on a more systemic level. So not only fix the data, but we should also try to adapt the models so that they take, for example, those temporal dynamics into account. We might also look at the outputs and try to fix the output at the interface level. so that's actually, for example, people's personal biases are not triggered in the first place. So there are multiple places in the system where we might want to design interactions that try to counteract the different forms of biases that are at play when people interact with those systems.
Lauren Prastien: On the flip side, it’s important to remember that technology isn’t necessarily the problem here. In fact, there are a lot of examples of technology breaking down the barriers to pertinent information. We’ll talk about one such example in just a moment.
[break]
Lauren Prastien: When it comes to democratizing information, technology can also do a lot of good. To look at one example, here’s Stephen Caines. You heard from him earlier, but to remind you, he’s a residential fellow at the CodeX Center for Legal Informatics, whose work focuses in part on the safe and ethical implementation of technology in the public sector.
Stephen Caines: While information is probably the most democratized it has ever been, there's still certain boundaries. And we could talk about, you know, physical boundaries such as like, you know, the net neutrality discussion, but then also I think that there just seems to be a lack of true, like human centered UI or user interface. and also just the notion of clearly accurate and tailored information.
Back in March, when the pandemic was just starting in the US, I was really blown away how different jurisdictions that were literally just next to each other, were handling this pandemic very differently. And through the legislative action of executive orders, whether it be from let's say a governor or a public health group, or even a county judge in certain cases, things like mask ordinances, shelter-at-home orders, and all these various kinds of legislative actions. I found that it was astounding that these independent jurisdictions were kind of left to do what they wanted on their own and there was very little leeway. So you were seeing this patchwork of legislation across the US on a very serious subject.
Lauren Prastien: So Caines and collaborator Daniel Carvajal founded the CoronAtlas dashboard back in March. Originally, it was intended to track all of these legislative orders at the state, county, city and town level, to create a kind of clearing house for all of this information.
Stephen Caines: And so the goal with this was to achieve three things. And the first one, a very basic level, was essentially a legislative tracking. So you'll be able to answer simple questions, so, do I need a mask at this specific grocery store? So just tracking the existence of legislation, knowing if it was still effective and then to whom it applied to. On the second level, because I've done some work in the public interest space, I was interested to know if some of these legislations would have disproportionate impacts on communities, specifically elements such as fines. So whether a mask ordinance that had a fine to it, it would disproportionately impact minority communities. But for me to prove that I would need to have all of the places that had mask orders that had fines and both not had fines before could make that kind of empirical claim. So I was looking for the ability to have summary analysis of the legislation to kind of almost like earmark key components. And then the third level is I wanted to create a very clean legal data set that would go a little bit, even further past that summary legal analysis, and be able to tell you things like the exact span of time that it was enacted, some more nuanced details about legislative construction. And the goal of this was so people outside of the legal field would be able to better utilize these legislative orders and they would be able to make their own distinctions.
Lauren Prastien: So for instance, if you were trying to figure out whether an ordinance had a grace period, or a period of time before it comes into effect, and if that grace period allowed for a more robust implementation of say, a mask ordinance, you’d be able to understand how effective that legislation was, even if you had very little in the way of legal training. Which, by the way, involved a lot of labor on the back end. Because a system like the CoronAtlas dashboard, or anything like it, does require real, human labor to make that information legible and understandable. It’s not just about throwing it on a site.
Stephen Caines: And so I hand-coded 450 pieces of legislation myself. My friend Daniel built the site, figured out how to put this on a map and a very beautiful visualization. But then we kind of decided to pivot due to resource constraints. And so the new model of CoronAtlas as it exists today is actually geared specifically towards the public.
Lauren Prastien: Today, CoronAtlas includes tailored links related to state-specific resources. So, if you were moving to a new state, you’d be able to see if there was a quarantine period or a specific travel advisory. By using information collected from government sites and nonprofits, it also includes information related to issues like housing. Not only with reference to state-specific eviction protections, but also the names of nonprofits that can help you if you’re facing eviction.
Stephen Caines: I would say at a basic level, I think that if you almost imagine data as digging for gold, I think that people can recognize when a piece of information is useful, but they struggle with, kind of, the cleaning and presentation elements. So in our first iteration of CoronAtlas, originally we were just finding the original government documents. And then it quickly became apparent to us that unless you're a legal professional, you really have, generally, you have no interest in reading, you know, four to ten pages of strict legalese. Sometimes you just want the summary. And so one iteration that we put in is that for each kind of legislative event, we included multiple types of it. So when I say that, we would include a news link, so if you're just in the general public and you just want to know something kind of really simplified and reduced, it was there. But if you were a government official and you wanted the official document, you have there. But then also if you want something in between, then we would include something like a press release, let's say, right?
Lauren Prastien: People often want the same information, but for different reasons. And those reasons often dictate the level of detail and the language in which that information should be presented. It’s not about condescending or pandering. It’s about truly making that information accessible in a manner that suits the person looking for it and doesn’t present unnecessary barriers to acquiring knowledge. Because according to Caines, something important to be gained from having information readily available is the cultivation of public trust.
Stephen Caines: One thing that I'd like to point out is that we often think of kind of within the legal system, that the public trust is always when the government is kind of at an adversarial point. Meaning that like, you know, when the government’s kind of coming after you, whether it be for like, let's say law enforcement proceeding, an immigration proceeding. But I think that one thing that's often missed within the public interest and nonprofit space is that some of that frustration is, and rightfully so, directed at us as the service providers if we do not provide, like, let's say, clear, coherent, professional service in that sense. And I think that although legal services throughout the country are underfunded, and there's so many things that like we're tasked with doing, I think that it's very important that we also make sure that our digital representation of resources and materials is also authoritative and it's accurate to kind of the same kind of stringent kind of protocols that we put off and on our counterparts on the other side, simply because people often become frustrated with even nonprofits that can help them in that later kind of taints their willingness to kind of go against certain proceedings that may be adversarial or kind of pursue different resources.
Lauren Prastien: So, is information democratized?
Over the course of this season, we’ve asked this question in a lot of forms. Like, is data democratized, is AI democratized, is knowledge democratized. For that last one, you could say knowledge and information are kind of synonyms, maybe, though not perfect ones. And in a way, what we’ve really been asking, is: if knowledge is power, and the information age has changed how we obtain, organize and distribute knowledge, has the information age changed those power structures at all? And this is also just as messy and difficult a question to answer as just about any of those questions I just rattled off. And when we asked the many guests we’ve had on this season, the answer was basically always the same: yes and no.
Because yes, knowledge is power. There’s power in knowing things, and there’s also power in deciding who else gets to know things, where that information lives, and what people have to do in order to get it. Just like there’s power in deciding who gets to add to our knowledge base, what counts as a fact, and whose opinions are worth disseminating. Technology can do a lot to help shake up these power structures, like lowering the barriers to accessing information or quantifying and correcting racial bias in EEGs and other diagnostic tools, and it can also just strengthen and reinforce these power structures by imbuing them with a sense of objectivity and legitimacy.
In the past nine episodes, we’ve looked at how information systems have changed with the emergence of new technologies like AI, and how those technologies have also been impacted by the systems that generate the data on which they rely. We’ve seen how knowledge and innovation have become even more widely collaborative than ever before, be it in the maintenance of Wikipedia or the open source software that serves as the invisible infrastructure of the Internet. We’ve looked at how opening the marketplace of ideas can mean that more people have a seat at the table in determining what is relevant and important, and also how that can also give rise to disinformation and radicalization. We’ve looked at the bias pipeline that impacts everything from data to technologies to the systems that use those technologies, be it in the way an EEG works on a white patient versus a non-white patient, or the way the Enron emails gave us spam films, or the way natural language processing technologies can reflect the biases of a society while also amplifying those biases. We’ve looked at how humans can both diminish bias and increase it, and how technologies, like peer review algorithms, can do the same. We’ve discussed topics like data diversity, crowd labor, and human-AI collaboration. And the answer on all of these smaller levels was also basically that: yes and no.
So, what can be done? There is no cut and dry answer, though our guests in these episodes have laid out a few solutions, like improving digital literacy to combat disinformation, evaluating whether using a profit-based platform is the best for obtaining subjects for scientific research, facilitating access to higher quality data, and increasing the diversity of people involved in developing technological solutions and creating environments that uphold their input as valid and, yeah, I’ll say it, consequential.
And there are things that you can also do on an individual basis. If you recognize there is an information desert forming related to some topic that you have expertise or understanding in, consider the role you might play in filling it, even if it is as simple as starting an account with Wikipedia and filling in the page for, say, achievements by women computer scientists or tenant’s rights. Because you don’t know what information system, algorithm, or just human being down the line will benefit from having that information.
But until then, this was Consequential.
Eugene Leventhal: This was our final episode of our season on knowledge production in the information age. Thank you for listening and sticking with us. If you’ve liked what you heard this season, let us know on Apple Podcasts.
[music]
Lauren Prastien: Consequential is produced by the Block Center for Technology and Society at Carnegie Mellon University. The Block Center was established to examine the societal consequences of technological change and create meaningful plans of action. To learn more about Consequential, the Block Center and our faculty, you can check out our website at cmu.edu/block-center or follow us on Twitter @CMUBlockCenter, all one word.
This episode of Consequential was written by Lauren Prastien and was produced by Eugene Leventhal. Our executive producers are Scott Andes, Shryansh Mehta and Jon Nehlsen. The music you’ve heard throughout this episode was produced by Fin Hagerty-Hammond. To learn more about our guests and see the sources we referenced for this episode, visit consequentialpodcast.com.