February 11, 2013
PITTSBURGH-A musical collaboration, be it Rodgers and Hammerstein or Robert Plant and Alison Krauss, requires a mix of shared and complementary traits that is not always obvious. Researchers at Carnegie Mellon University discovered elements of this unique chemistry by using an automated technique to analyze an online songwriting community.
Based on four years of data collected though an international songwriting challenge called February Album Writing Month, or FAWM, the Carnegie Mellon team found that common interests or skills do not cause collaborators to seek each other out. The researchers found, for instance, that two people were actually less likely to collaborate if they liked the same songs. More likely would be a writer in one genre seeking out a
collaboration with someone who works in a less familiar genre.
"Creative collaborators are trying to expand their horizons, so they seek out collaborators with complementary interests and skills," said Steven Dow, assistant professor in the Human-Computer Interaction Institute, who conducted the study with Burr Settles, a post-doctoral fellow in the Machine Learning Department.
Among other findings, FAWM participants - or "fawmers" - who share the same social status in the online community tend not to collaborate with each other as much as those who are slightly more (or slightly less) prominent. And, contrary to some previous findings, if one collaborator performs the bulk of work, both feel unsatisfied, not just the one pulling the load.
Settles and Dow employed a relatively new technique for analyzing the FAWM collaboration data set, which included 39,103 songs and 6,116 participants, as well as song tags, locations and forums. The "path-based regression" technique they employed could be a useful tool for additional social science research involving Big Data, they said. The study also included a survey of FAWM participants.
Their findings will be presented at CHI 2013, the Conference on Human Factors in Computing Systems, in Paris, France, April 27-May 2. They also have posted a YouTube video, http://www.youtube.com/watch?v=WpbJBJgTXcY, summarizing their findings and Settles has discussed the analytical technique on his blog, Slackpropagation.
FAWM, which Settles co-founded in 2004, challenges people to compose 14 new works of music - roughly an album's worth - within the 28 days of February. Last year, 1,325 people churned out 11,015 songs, with 465 people meeting the goal. FAWM is one of a number of popular online communities that enable creative collaborations, including Newgrounds for animation and Scratch for interactive media projects.
For this study, Settles and Dow used a "path-based regression" technique that was pioneered in a Machine Learning Department project called Never-Ending Language Learning, or NELL. In that project, a computer continuously analyzes hundreds of thousands of Web pages to extract facts, identify correlations and ultimately teach itself to read.
The path-based regression program takes "random walks" through network data, noting which paths between a pair of data points could connect two phenomena; in the case of collaborations, these paths would connect two individuals who may or may not be collaborators. The program automatically evaluates which paths have predictive power and discards the irrelevant ones. Thus, it can find connections within large data sets that may not be obvious to human observers.
"Scientists usually have to develop a theory, construct a model and then run an analysis to prove or disprove a hypothesis," said Settles, a former member of the NELL research team. "With this technique, the program can randomly sample thousands of paths and automatically identify the ones that seem most noteworthy."
One surprising finding related to the balance of work in a collaboration. Settles and Dow found that collaborators were happiest when work was split 50-50. When work was unbalanced, the person who did most of the work was unhappy, which is consistent with previous collaboration research into "social loafing." But in contrast to those earlier findings, the CMU researchers found that even the collaborators who did little work were dissatisfied.
This work was partially supported by the National Science Foundation and Google.
The Machine Learning Department and Human-Computer Interaction Institute are part of CMU's School of Computer Science. Follow the school on Twitter @SCSatCMU.
###
Press Release: Carnegie Mellon Analysis Shows Online Songwriters Seek Collaborators With Complementary Skills, Status
Big Data Approach Promises New Tool for Social Science Research
Contact: Byron Spice / 412-268-9068 / bspice@cs.cmu.edu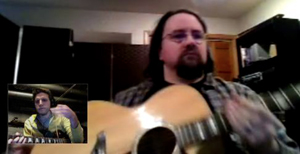
Based on four years of data collected though an international songwriting challenge called February Album Writing Month, or FAWM, the Carnegie Mellon team found that common interests or skills do not cause collaborators to seek each other out. The researchers found, for instance, that two people were actually less likely to collaborate if they liked the same songs. More likely would be a writer in one genre seeking out a
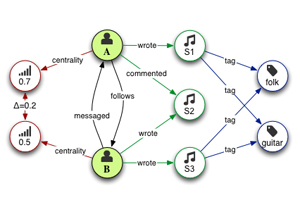
"Creative collaborators are trying to expand their horizons, so they seek out collaborators with complementary interests and skills," said Steven Dow, assistant professor in the Human-Computer Interaction Institute, who conducted the study with Burr Settles, a post-doctoral fellow in the Machine Learning Department.
Among other findings, FAWM participants - or "fawmers" - who share the same social status in the online community tend not to collaborate with each other as much as those who are slightly more (or slightly less) prominent. And, contrary to some previous findings, if one collaborator performs the bulk of work, both feel unsatisfied, not just the one pulling the load.
Settles and Dow employed a relatively new technique for analyzing the FAWM collaboration data set, which included 39,103 songs and 6,116 participants, as well as song tags, locations and forums. The "path-based regression" technique they employed could be a useful tool for additional social science research involving Big Data, they said. The study also included a survey of FAWM participants.
Their findings will be presented at CHI 2013, the Conference on Human Factors in Computing Systems, in Paris, France, April 27-May 2. They also have posted a YouTube video, http://www.youtube.com/watch?v=WpbJBJgTXcY, summarizing their findings and Settles has discussed the analytical technique on his blog, Slackpropagation.
FAWM, which Settles co-founded in 2004, challenges people to compose 14 new works of music - roughly an album's worth - within the 28 days of February. Last year, 1,325 people churned out 11,015 songs, with 465 people meeting the goal. FAWM is one of a number of popular online communities that enable creative collaborations, including Newgrounds for animation and Scratch for interactive media projects.
For this study, Settles and Dow used a "path-based regression" technique that was pioneered in a Machine Learning Department project called Never-Ending Language Learning, or NELL. In that project, a computer continuously analyzes hundreds of thousands of Web pages to extract facts, identify correlations and ultimately teach itself to read.
The path-based regression program takes "random walks" through network data, noting which paths between a pair of data points could connect two phenomena; in the case of collaborations, these paths would connect two individuals who may or may not be collaborators. The program automatically evaluates which paths have predictive power and discards the irrelevant ones. Thus, it can find connections within large data sets that may not be obvious to human observers.
"Scientists usually have to develop a theory, construct a model and then run an analysis to prove or disprove a hypothesis," said Settles, a former member of the NELL research team. "With this technique, the program can randomly sample thousands of paths and automatically identify the ones that seem most noteworthy."
One surprising finding related to the balance of work in a collaboration. Settles and Dow found that collaborators were happiest when work was split 50-50. When work was unbalanced, the person who did most of the work was unhappy, which is consistent with previous collaboration research into "social loafing." But in contrast to those earlier findings, the CMU researchers found that even the collaborators who did little work were dissatisfied.
This work was partially supported by the National Science Foundation and Google.
The Machine Learning Department and Human-Computer Interaction Institute are part of CMU's School of Computer Science. Follow the school on Twitter @SCSatCMU.
###