MSE Seminar Series
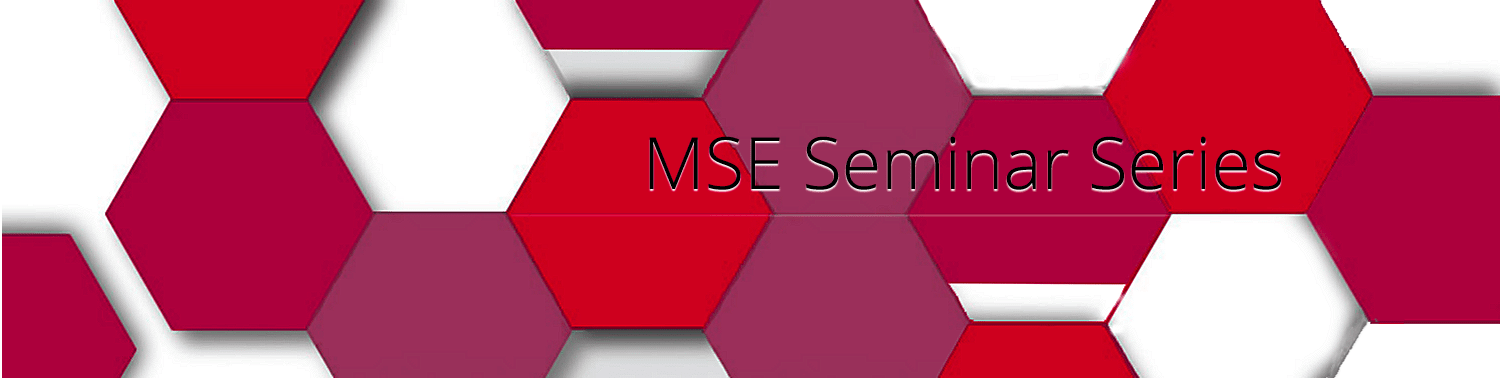
Friday, May 7, 2021 @11:40am
*Remote course - Zoom link will be provided
Stefano Sanvito, Trinity College, Dublin, Ireland
presents
From the periodic table to new magnets:
climbing the inverse design mountain
ABSTRACT:
The development of novel materials is a fundamental enabler for any technology, to the point that often technology and materials innovation cannot be separated. Unfortunately the process of finding new materials, optimal for a given application, is lengthy, often unpredictable, and has a low throughput. Here I will describe a systematic pathway to the discovery of novel compounds, which demonstrates an unprecedented throughput and discovery speed. The method can be applied to any materials class and any potential application. I will use the example of magnetism to introduce the main features of the method, and I will demonstrate the discovery of several new high-performance magnets. Furthermore, I will highlight how such high-throughout schemes can be combined with machine-learning methods for data-mining to extract novel materials designing rules and for identifying new prototypes for further investigation. Firstly, I will describe high-throughput electronic structure theory [1]. Out of an extensive electronicstructures library of Heusler alloys (236,115 prototypes) we filter those displaying magnetism and establish whether they can be fabricated at equilibrium [2]. In particular, we analyse in detail transition-metals intermetallic Heuslers. Among the possible 36,540 prototypes, 248 are found thermodynamically stable but only 20 are magnetic. The magnetic ordering temperature, TC, is estimated by a regression calibrated on the experimental TC of about 60 known compounds. As a final validation we attempt the synthesis and produce two new magnets. One, Co2MnTi, displays a remarkably high TC in perfect agreement with the predictions, while the other, Mn2PtPd, is a complex antiferromagnet. Then, I will discuss the use of machine-learning methods for predicting the Curie temperature of ferromagnets, based solely on their chemical composition (see figure) [3], and for sorting magnets into hard and soft. In particular I will discuss how to develop meaningful feature attributes for magnetism and how these can be informed by experimental and theoretical results. Finally, I will describe how an accurate description of the structure of materials, which is amenable to be used with machine-learning, can offer a quantum-chemistry accurate description of local properties at virtually no computational costs. The method is not just suitable for building energy models [4], namely force fields, but also for any other electronic quantity. These models may then be used to design new materials, as demonstrated here for magnetic molecules with enhanced uniaxial anisotropy [5]. All this together can pave the way for the large-scale design of novel magnetic materials at unprecedented speed. [1] S. Curtarolo, G.L.W. Hart, M.B. Nardelli, N. Mingo, S. Sanvito and O. Levy, The high-throughput highway to computational materials design, Nature Materials 12, 191 (2013). [2] S. Sanvito, C. Oses, J. Xue, A. Tiwari, M. Zic, T. Archer, P. Tozman, M. Venkatesan, J.M.D. Coey and S. Curtarolo, Accelerated discovery of new magnets in the Heusler alloy family, Science Advances 3, e1602241 (2017). [3] J. Nelson and S. Sanvito. Predicting the Curie temperature of ferromagnets using machine learning. Phys. Rev. Mat. 3, 104405 (2019). [4] A. Lunghi and S. Sanvito, A unified picture of the covalent bond within quantum-accurate force fields: from simple organic molecules to metallic complexes’ reactivity, Science Advances 5, eaaw2210 (2019). Advances 5, eaaw2210 (2019). [5] Alessandro Lunghi and Stefano Sanvito. Surfing multiple conformation-property landscapes via machine learning: Designing magnetic anisotropy. J. Chem. Phys. C 124, 5802 (2019). Figure: TC prediction as a function of composition for the ternary system Al-Co-Fe. Data are presented as a function of the atomic fraction of the three species and the TC is expressed as a heat map. The figure also introduces a detailed analysis of the three relevant binary phase diagrams, where the blue line traces the ML prediction, black crosses (green dots) are experimental points included (not included) in the training set. The light-blue shadowed area in the binary plots corresponds to the range of predicted TC’s, namely it indicates the uncertainty of the machine-learning model. The solid square (circles) included in the ternary TC diagram are for experimental data included (not included) in the training set, with the colour code describing the TC. Numbers correspond to four known stoichiometric phases: 1) Co2FeAl, TC = 1,000 K, 2) Fe2CoAl, TC > 873 K, 3) Fe4CoAl, TC = 420 K, 4) Fe3Al, TC = 573 K [figure from . Nelson and S. Sanvito. Predicting the Curie temperature of ferromagnets using machine learning. Phys. Rev. Mat. 3, 104405 (2019)].
BIOGRAPHY:
Prof. Sanvito studied Physics in Milan, Italy, (“Laurea”) and Lancaster, UK (PhD). After two years at the University of California Santa Barbara, in 2002 he joined the School of Physics at Trinity College Dublin. In 2006 he became associated Professor and in 2012 Professor of Condensed Matter Theory. Since 2013 he is the Director of the Center for Research on Adaptive Nanostructures and Nanodevices (CRANN) and for the period 2013-2015 he has been the Director of the AMBER Center.
Prof. Sanvito leads the internationally recognized Computational Spintronics Group, which develops new algorithms for materials and device modeling and applies them to problems underpinning information technology. One of Sanvito’s flagship achievements is the Smeagol code, the world-leading software for simulating devices at the atomic scale. Smeagol, distributed worldwide to more than 200 groups, has impacted a multitude of technologies ranging from data storage to DNA sequencing. Prof. Sanvito is author of more than 300 papers (h-index 70, Google Scholars), 3 books and numerous book chapters and he has attracted funding in excess of 70,000,000 Euro (including the 50M AMBER center).
In 2007 he received the Young Scientist Prize in Computational Physics from the International Union of Pure and Applied Physics (IUPAP) and in 2012 the prestigious European Research Council Award. Prof. Sanvito is a Fellow of the Institute of Physics, a fellow of Trinity College Dublin and a Member of the Royal Irish Academy. In 2017 he was conferred the title of “Cavaliere della Stella d’Italia” (knight of the star of Italy), an Italian knighthood order given to Italians abroad, who have contributed to enhance the prestige of Italy and to establish relations with foreign countries. In 2020 he was included in the list of the Highly Cited Researchers from ClarivateTM.