MSE Seminar Series
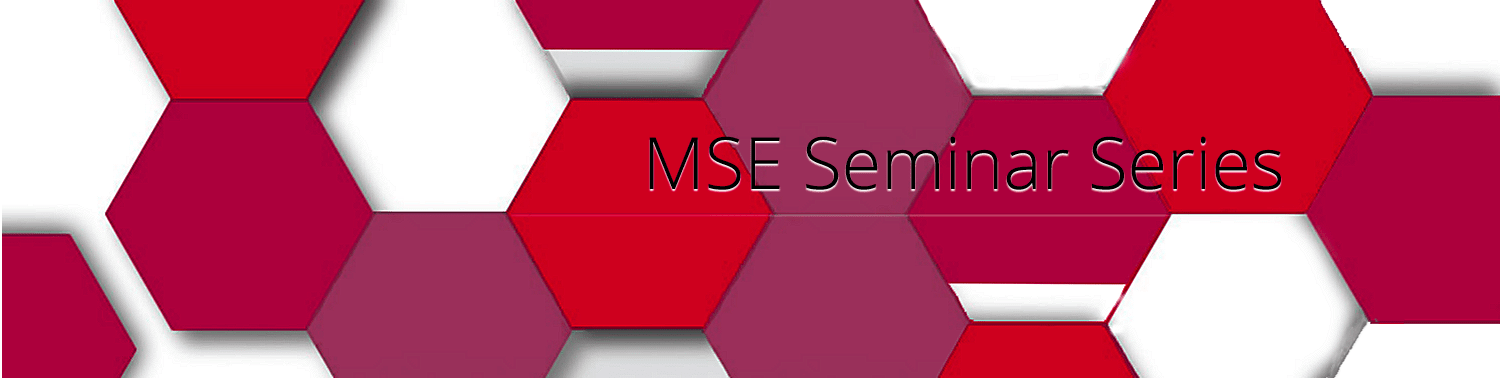
Friday, February 12, 2021 @11:40am
*Remote course - Zoom link will be provided
Dr. Patrick Rinke, Department of Applied Physics, Aalto University, Helsinki Finland
presents
Active Materials Exploration and Characterization with Machine Learning
ABSTRACT:
Data generation in materials science is often limited by the time it takes to perform experiments or simulations [1]. To facilitate the exploration and characterization of complex materials, we have developed the Bayesian Optimization Structure Search (BOSS) code [2]. BOSS uses an active learning technique that strategically samples the parameter space of material-science tasks be it experimental or computational. BOSS proposes new data acquisition points for maximum knowledge gain, balancing exploitation with exploration. I will demonstrate BOSS' smart and efficient data strategy for two examples: 1) sustainable biomaterials and 2) hybrid organic-inorganic electronic materials. For 1), we extract lignin from wood samples with hydrothermal treatment. Lignin is further processed by chemical modification into sustainable composite materials (e.g. carbon fibers, thermoplastics and three-dimensional printed objects) Lignin extraction and processing is coupled to BOSS to visualize process-structure-property correlations and to efficiently optimize extraction and modification conditions. For 2), we couple BOSS to density-functional theory (DFT) calculations to study the adsorption of a camphor molecule on a copper surface. We identify 8 unique stable adsorbates [3]. By matching the stable structures to atomic force microscopy (AFM) images, we conclude that the experiments feature 3 different structures of chemisorbed camphor molecules. This is the first time that the atomic structure of bulky 3D adsorbates has been decoded.
[1] Data‐Driven Materials Science: Status, Challenges, and Perspectives, L. Himanen, A. Geurts, A. S. Foster and P. Rinke, Adv. Sci. 6, 1900808 (2019)
[2] Bayesian Inference of Atomistic Structure in Functional Materials, M. Todorović, M. U. Gutmann, J. Corander and P. Rinke, npj Comp. Mat. 5, 35 (2019)
[3] Detecting stable adsorbates of (1S)-camphor on Cu(111) with Bayesian optimization, J. Järvi, P. Rinke and M. Todorović, Beilstein J. Nanotechnol. 11, 1577 (2020)
BIOGRAPHY:
Prof. Patrick Rinke received his PhD from the University of York in England in 2003. Subsequently, he was a post-doctoral scholar at the Fritz Haber Institute (FHI) of the Max Planck Society in Berlin, Germany, and in the Materials Department at the University of California Santa Barbara (UCSB) before becoming a group leader at the FHI in 2009. Since 2014, he leads the Computational Electronic Structure Theory (CEST) group in the Department of Applied Physics at Aalto University in Helsinki, Finland. He develops advanced electronic structure, data science and machine learning methods and applies them to pertinent problems in materials science. Since 2019, he coordinates the AI in Materials Science Highlight in the Finnish Center for Artificial Intelligence
(https://fcai.fi/) and the Digitalization Hub of the FinnCERES Bioeconomy Flagship (https://finnceres.fi/).