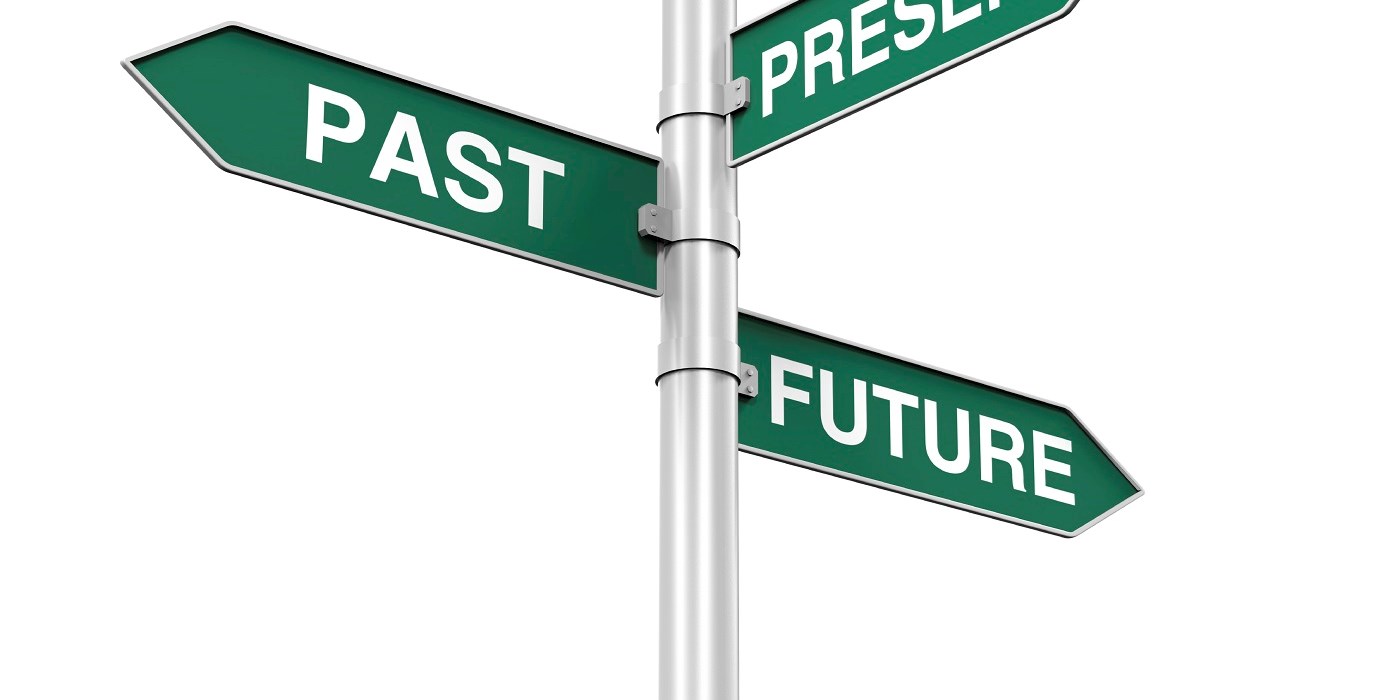
When Cristian Young saw a car advertisement pop up on the side of his Facebook newsfeed, it gave him pause. Most of us wouldn’t notice what seemed like just another ad among many during our daily Internet usage, but Young had a more nuanced perspective on the ad’s sudden appearance.
As an undergraduate at Carnegie Mellon University, he studied information systems; then he went on to get his master’s degree in information and knowledge strategy at Columbia University, where he’s also adjunct faculty. After working in analytics for marketing agencies, and now as the global knowledge manager for strategic branding firm Siegel+Gale, he understands why and how online advertisers target their ads, so seeing one for a car sent his mind scrolling back over his Internet activity in the previous weeks.
You see, as a three-year resident of New York City, dependent on public transportation, he had never bought a car, or had a car, or even had a car ad populate on a website before. He knew this meant that something about his recent online behavior suddenly indicated to marketers that he might be interested in buying one. And even more surprising? The ad was right. He really did want to buy a car. This indirectly troubles Anupam Datta, an associate professor in both the Computer Science and Electrical and Computer Engineering departments at CMU—but more on that later.
To fully appreciate Young’s bewilderment at the well-timed car ad, you have to understand the multistep journey every online ad takes before it pops up on your screen. For more than a decade, online marketers have been compiling or purchasing data about you from several sources in order to better target ads. The ads are deployed by software made up of algorithms—conditional operating instructions—that get “smarter” over time as behavior patterns emerge. These algorithms learn things about your online behavior and about the demographics to which you belong and then use this information to incrementally increase how effective they are at placing an ad on your screen you might actually click.
Sources for the data being collected are varied. They include:
- Clickstreams—or data collected from “cookies” sent by websites that track your browsing and clicking activity on the Internet
- Search engine inquiries
- Online purchases
- Data sold by credit card companies selling data about your offline purchases
- Profile data from social media platforms, what you hashtag, and whom you know
- Location data based on your Internet access
“There’s definitely a shift away from using descriptive demographics—people who are this race, this age, this sexual preference—and instead focusing on online behaviors,” reflects Young. “If the person types these search terms and clicks on this thing, they have a demonstrated interest in this topic, so these are the people that we should advertise to.”
So for example, if you’ve been Googling flights to Italy, when you then navigate to The New York Times website, you might see advertisements for cheap airfares to Italy. Makes sense; the advertisers are showing you an ad that’s both relevant and timely.
But a little research into the mechanics of online advertising will reveal something you may not know: while any webpage loads, there is actually a live auction for each spot on the page where an ad could appear. Companies that collect and broker information about your behaviors and preferences—and there are many hundreds of them—reach out to marketers who have identified you as their target and essentially ask, “How much is this particular ad worth putting in front of this particular person?” The little spaces on the page that populate with flight suggestions or shoes or graduate programs are from the advertisers who’ve paid the most in that moment for your personal attention. And that all happens in the span of the second or two it takes for a page to load. And algorithms automate it all.
But it’s not as simple a formula as “preferences in, advertisements out,” according to professionals like Young. This means you won’t see ads only for the exact item or service you were searching for or have previously purchased. Googling flights to Italy might also prompt ads for luggage or travel insurance—or even for engagement rings if you’re searching for tickets around Valentine’s Day and you’ve been “In a Relationship” on Facebook for a while.
Not just meeting but anticipating customer needs and wants is the real goal for online advertisers. This means greater convenience for consumers, but it’s also making it harder to distinguish whether it was really your idea to propose on your trip to Italy, or if it was De Beers using an algorithm to recognize that many consumers before you clicked their way down the same digital paths before popping the question.
And that’s the chicken-and-egg situation Young found himself in. Had he already been contemplating purchasing a car? Or had the algorithms perfectly anticipated a change in his needs based on his recent online activity? Either way, what information was being collected on him that led the algorithms to such a conclusion?
Datta, the CMU professor, worries about questions like these. Relatively little research has been done on the subject of online advertising, and even fewer tools exist to examine and analyze the methods and repercussions of these advertising algorithms. So he built one himself.
Datta, along with CMU PhD student Amit Datta and CMU alumnus Michael Tschantz, built a tool called AdFisher to study Google’s ad network. AdFisher can simulate hundreds of hypothetical users surfing the Internet and then document the types and frequency of ads they are shown as a result of browsing.
One study Datta’s research team performed has implications in the privacy sector. After simulated users researched sites related to substance abuse, they were served ads for rehab centers. They were relevant, to be sure, but that information can now be used in perpetuity to target users, collected and sold the same way your last Zappos shoe purchase was. The Health Insurance Portability and Accountability Act (HIPAA), passed in 1996, ensures that patients’ medical, mental health, and substance abuse diagnoses can’t be disseminated without consent, but the law, like many others, doesn’t reach this far into the Wild Wild West of the web.
Relatively little research has been done on the subject of online advertising, and even fewer tools exist to examine and analyze the methods and repercussions of these advertising algorithms. So Anupam Datta built one himself.
Datta stresses that it’s not just what’s being done with the information collected that needs oversight, but the collection process itself. “Unfettered collection of personal data has a chilling effect on individual freedoms,” he explains. Of course, users may alter their behavior and not browse the Internet freely knowing that almost every click is being recorded. However, Datta brings it back to health conditions, positing that a patient may not research a medical issue for fear of that information getting into the hands of a prospective employer or an insurance company that may hike up rates or refuse coverage.
Although many people balk at the veritable encyclopedia of information being collected on everyone who uses the Internet, marketers typically counter by touting the benefits of a customized browsing experience. Wouldn’t it be irritating, for example, for childless consumers to see advertisements for diapers and preschools when marketers are perfectly capable of knowing they don’t have children? Wouldn’t you rather see a product that a sophisticated algorithm has determined you might actually want to purchase?
A few years ago, a spate of stories circulated about Target’s eerily accurate analytics and predictions. According to reporting by Forbes and The New York Times, the retailer looked at purchasing patterns of pregnant women in different trimesters who had already signed up for a baby registry. By sniffing out those same patterns among all its female customers, Target could then assign a “pregnancy prediction score” and estimate due dates to send relevant coupons. When higher-ups at Target realized that customers might find unsolicited coupons congratulating them on their first baby an invasion of privacy, the retailer responded not by stopping the practice, but by “hiding” the coupons for baby products in booklets of unrelated offers so that customers assumed they were random. And it worked.
Recalling what might have led up to the car ad on Facebook, Young pinpoints a moment on a JetBlue flight when a car advertisement played on the seatback satellite TV. It was a green Kia Soul.
Young immediately liked it. “I was like, ‘That’s an awesome car. I want that green car!’”
Through the free wi-fi available on the flight via JetBlue’s web portal, he began researching a few upcoming trips he was planning to take, looking into the cost of flights, which came out to what Young calls an “obscene amount of money.”
“So I had those two bits of information,” he recalls. “I like this green car, and, separately, looks like I’ll have to spend a lot of money on flights coming up.”
When he saw the car advertisement on his Facebook page a few days later, it suddenly occurred to him that it might be more cost effective in the long run to buy a car in order to make these trips rather than spending thousands in airfare and having no equity after his purchases. Somehow, the algorithms had also made the connection that Young was primed to buy a car. They already knew where he lives, that he’s a renter rather than a homeowner, that he has a dog, that he has a steady job, that he travels frequently. … Something about his behavior online tipped the scales and made him attractive to car companies for the first time. He began researching cars in earnest.
Online marketing is ubiquitous, and it’s not going anywhere. If anything, it’s only becoming more invasive, inventive, and robust. But aside from creeping out customers with how well they can anticipate their wants and needs, what real harm are marketers doing by using these methods?
The research of Datta’s team offers one possible answer.
Using AdFisher, they ran a simulation of a thousand users—500 profiles identified as men and 500 as women—and sent them on the same mission of browsing the top 100 job sites to look for employment. Then, these “men” and “women” navigated to a third-party website that uses Google Ads—specifically, the Times of India—and a statistical analysis was run to see whether the ads being served were different depending on the gender of the user.
“They were very different,” says Datta. “That already gives us evidence in support of differential treatment.” But that doesn’t, he says, explain whether the reason behind the difference was actually cause for concern, something more problematic than men seeing more clothing ads for men’s clothing than women’s, for instance.
The next step was figuring out which ads were most influential, that is, which ads appeared most frequently. “That’s where we got the startling result,” Datta says. The two most influential ads being served to male users were from a career counseling service for “executives only,” boasting salaries of more than $200,000.
This ad was shown to the male users about 1,800 times. But for the women?
“There were only about 300,” Datta reveals. “These kinds of ads are a gateway to opportunities for employment. This is where we felt that we started moving from differential treatment toward discriminatory treatment.”
The difference is stark, but who’s to blame for the inequality? Datta says it’s impossible to know at this point in the research whether the fault lies with marketers who specify “men” as a demographic, or whether it was just an unintended bias introduced by Google’s algorithm as it “learned” that men more often click on the ad so it should show the ad to more men—a kind of self-fulfilling prophecy.
“At a public policy level, if you look at the physical world, there are protections,” says Datta, referring to protected statuses like race, age, and gender. “We will have to think carefully about how those laws can be expanded to cover the online domain.”
“Unfettered collection of personal data has a chilling effect on individual freedoms.”
But as in his other study involving privacy concerns, the question of who or what is to blame is much more complicated than simply recognizing that these red flags exist. Finding where that flaw lies, he says, is the first step to instituting corrective measures. He’s currently pursuing a project with Microsoft Research to develop a methodology for assigning responsibility, and then applying it to Microsoft internally, where the researchers will have more visibility into the ecosystem—getting under the hood, if you will. He hopes other organizations will use tools such as AdFisher to monitor the behavior of their online ad targeting software and that regulatory agencies such as the Federal Trade Commission will use the tool to help spot abuses.
This idea is what he and other researchers call information accountability. As he explains, it’s not just these algorithms that are making decisions; it’s a combination of man, machine, and the interactions between them.
“In modern society, there is a combination of algorithms and human actors who operate inside ‘black boxes’”—meaning systems with unknown inner workings—“who are making important decisions. And what the field of information accountability is trying to do is to ensure that there are methods for examining these systems.”
The goal is to provide global oversight of these ecosystems to detect any deleterious effects like the aforementioned discrimination and privacy violations, or just a lack of transparency.
Or even intentional wrongdoing.
And this is where we loop back to Young again, the recent owner of a diesel Audi A3. That make and model may sound familiar for a very unfortunate reason: Yes, Young bought one of the 11 million cars affected by the recent scandal involving Volkswagen’s intentionally deceptive “defeat device” software. (Audi is owned by Volkswagen.) This software is governed by—you guessed it—algorithms that told the car to react differently in certain situations, like an emissions test. The car’s emissions were markedly lower when it was being tested than during everyday use, when in reality pollutant levels were up to 40 times the legal limit. Since the scandal broke in September, Volkswagen has been excoriated in the media and faces up to $18 billion in fines alone, to say nothing of the cost to recall or fix the cars and any damages it may pay to car owners. The car manufacturer recently announced that it would be giving all affected customers a $500 gift card and $500 in credit at dealerships. No word on when actual repairs might begin, but in the meantime, VW stock has plummeted by as much as a third.
Young had debated between a hybrid and a diesel engine before settling on the A3, which he can laugh about now, if a little bitterly. “I specifically bought the car so that it was better for the environment because I knew I was going on a lot of road trips!” he says exasperatedly. He notes, with irony, that he also has asthma—a condition irritated by car emissions.
The algorithms populating Young’s Facebook feed with ads may have convinced him to buy a car, but the ones lurking under the hood got the last laugh.
Datta reflects on the depth of this seeming betrayal: We expect technology to be fair and impartial in a way that humans inherently cannot, he says. But we’re forgetting that software and the algorithms that run it are all man-made and can be imbued with man’s biases and imperfections.
But what are the corrective measures? Datta and Young both agree that a combination of internal awareness and external oversight and accountability is key. Options range from an expansion of tools like AdFisher to regulations that penalize companies found to be in violation.
Citing the example set by the Human Rights Campaign, which every year publishes a Corporate Equality Index as a benchmarking tool for LGBTQ rights in the workplace, Young sees a similar opportunity in the information accountability space. He imagines there could be an organization that stress-tests a company’s marketing algorithms, patches any problematic holes, and then “certifies” that a company has reached a certain threshold of accountability, similar to how some businesses now become authorized retailers or “verified” vendors on PayPal.
On a personal level, though, what can you do when you are sitting in front of your computer screen, which you may be eyeing with increasing suspicion?
Take a page out of Datta’s and Young’s book. They both use Internet browser extensions that either inform them when their data are being shuffled off to marketers or block their information from being sent. Datta uses Privacy Badger, for example. (If you Google Privacy Badger, be prepared to receive ads for similar browser extensions!) A plug-in called Ghostery, which Young vouches for, shows users how many separate companies just collected their data when they load any given website. If he sees a long list begin to populate and he doesn’t absolutely need the information he’s searching for, he’ll close the site before the search is complete and make a mental note not to revisit.
As for why more people don’t take actions like this to safeguard their data, Datta says it’s a combination of the general public lacking a full understanding of potential consequences while also performing a kind of informal cost-benefit analysis—if you want to opt out of having your data collected, there’s very little you can do on the Internet. But the decision-making is fundamentally flawed, he warns: “Without understanding the consequences, a cost-benefit analysis can lead you to the wrong conclusion.”
Consider yourself informed.