Astrophysics
Rupert Croft's interest is using AI to approach the inverse problem in cosmology: going from observational data to underlying physical quantities. A one dimensional example of this is shown in the Figure. The top panel shows a simulated observation of the "Lyman-alpha forest", the spectral absorption caused by hydrogen in intergalactic space. Where there is a high density of hydrogen causing absorption, the flux in the top panel is low. Where the (noisy) flux is consistent with zero (or saturated), the Universe is effectively hidden. The bottom panel shows a map of the the actual neutral hydrogen (blue line) corresponding to the spectrum in the top panel. There is a large spike which cannot be recovered by standard techniques (green and gray lines). A Neural Network trained on cosmological simulations uses the totality of information in the spectrum to reconstruct the hidden spike (orange line).
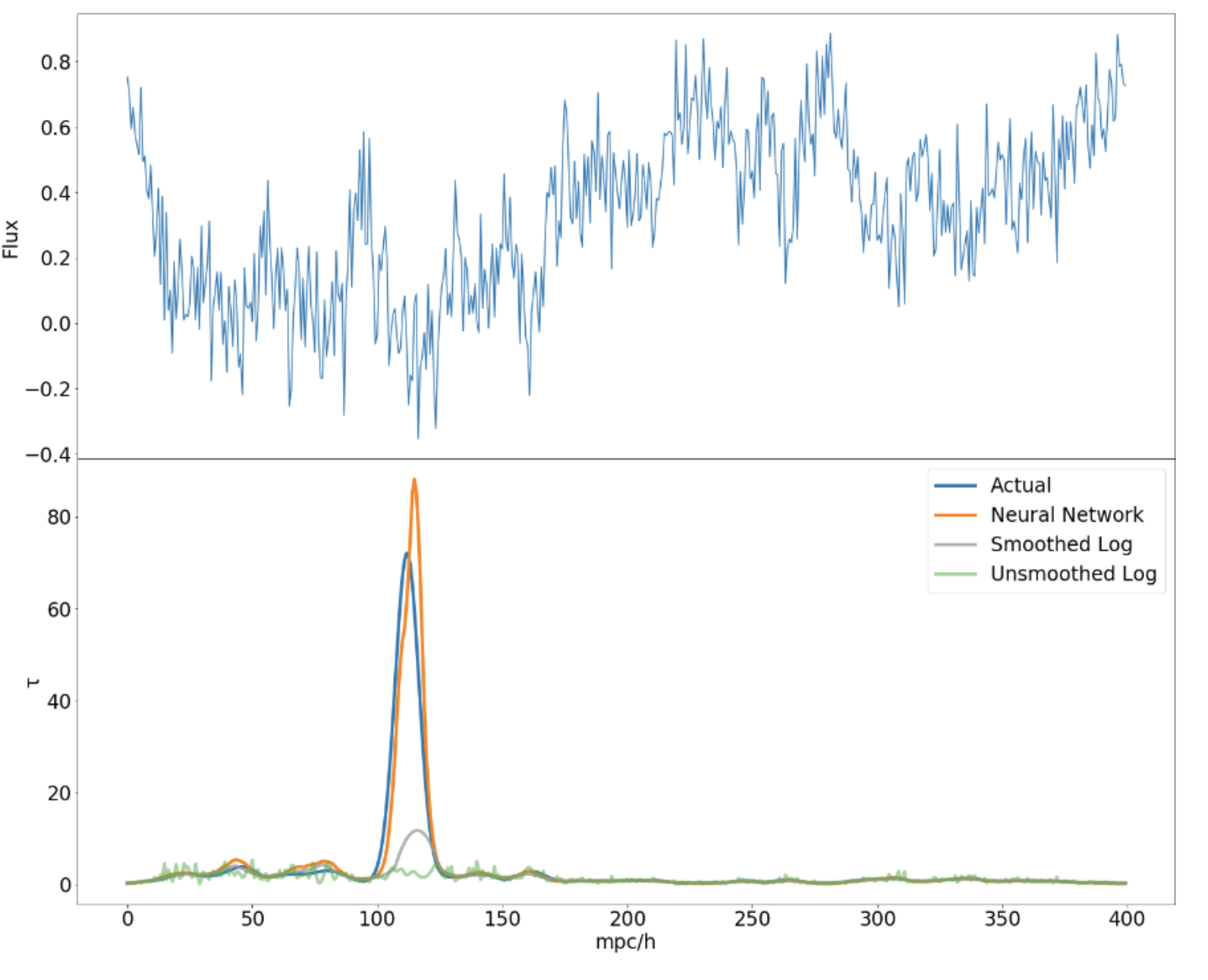
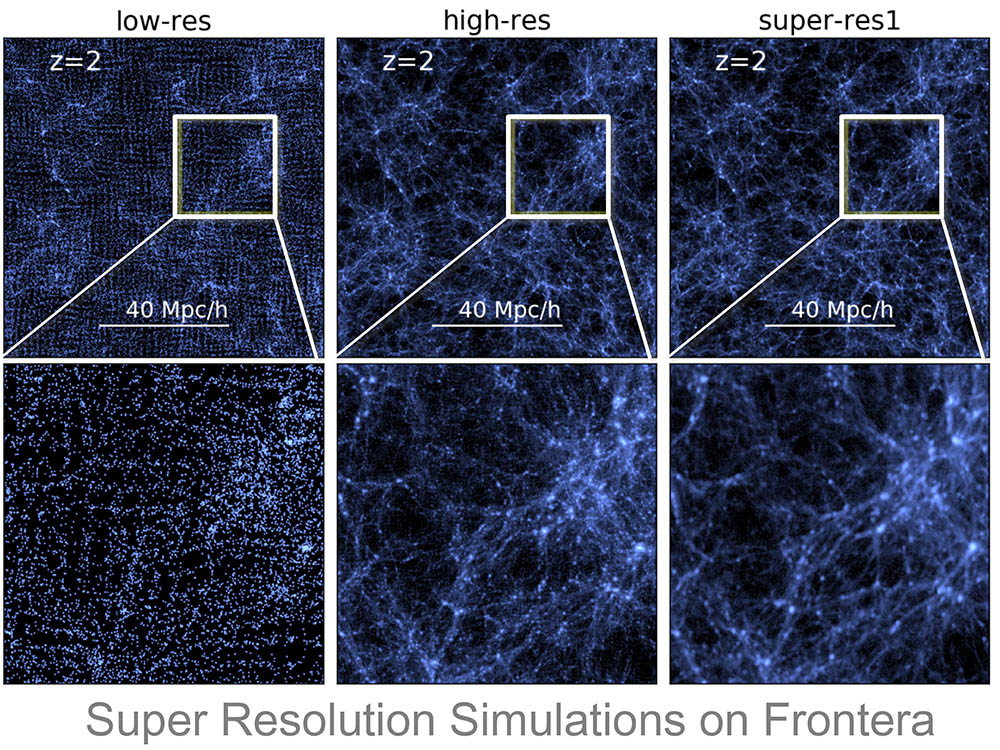
Tiziana Di Matteo's group is using NSF leadership HPC facilities to develop a new framework for cosmological simulations of galaxy formation. In concert with new and more powerful telescopes and satellites, they aim to combine expertise and existing super-scalable codes for petascale-plus cosmological hydrodynamic simulations with Machine Learning (ML) techniques to effectively create models on the scale of the observable Universe that incorporate information from higher resolution models of individual galaxies. This hybrid approach, which implies offloading our simulations to neural networks and other ML algorithms, will enable us to predict quasar, supermassive black holes and galaxy properties in a way which is statistically identical to full hydrodynamic models but with a significant speed up.
Cosmological data sets and analyses have high dimensionality. As examples, each of the hundreds of millions of galaxies in current surveys is observed in multiple "bands" so we might have 9 colors for each object. In this example, an important goal is to map these colors onto a "redshift" which indicates how distant the object is from us. We do this compression (9 colors down to one redshift estimate) with the aid of a Self Organizing Map (see figure). Each of the cells there consists of galaxies with very similar colors and therefore likely to be at similar redshifts. Scott Dodelson and Rachel Mandelbaum work on a wide variety of AI techniques like this to extract information about dark energy and dark matter from photometric galaxy surveys.
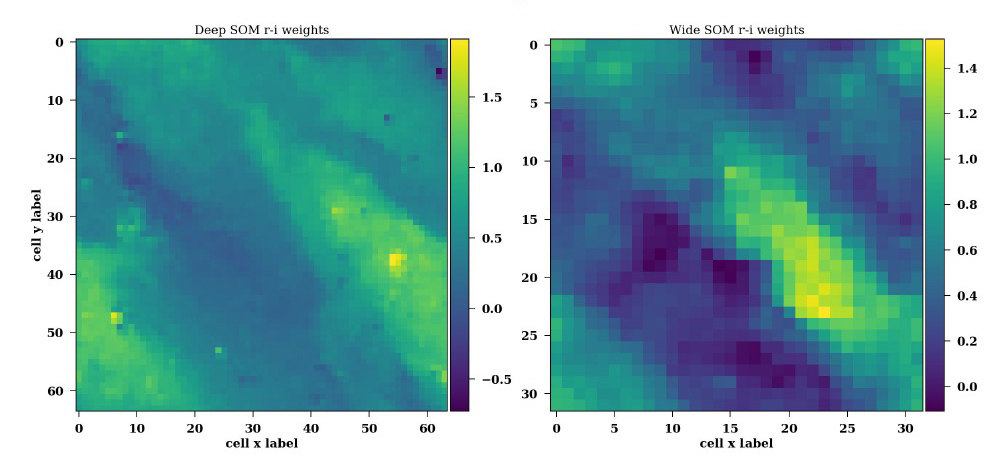
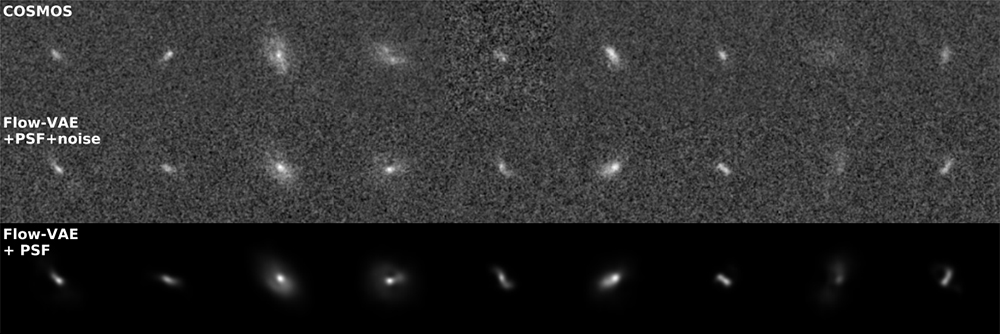
Rachel Mandelbaum's work includes the use of weak gravitational lensing and other analysis techniques, with projects that range from development of improved data analysis methods, to actual application of such methods to existing data. The figure depicts samples from real galaxies as observed with the Hubble Space Telescope (top), and random draws from the generative model (middle) with matching PSF and noise, conditioned on the size, magnitude, and redshift of the corresponding real galaxy. The bottom row shows the same generated light-profiles but without observational noise. Because of the conditioning, generated galaxies (middle) are consistent in appearance with the corresponding real galaxy. Image credit: Lanusse et al. (2020).
Where is the dark matter? Fritz Zwicky in 1933 postulated the existence of dark matter when he inferred the total mass of the Coma cluster from the motion of its galaxies and found it to be much larger than the visible mass. Vera Rubin in 1970 confirmed this from the rotation of stars and gas in Andromeda and other nearby galaxies. Today we think that dark matter makes up about 85% of the matter and 25% of the mass-energy of the Universe, but we have yet to map out its three-dimensional cosmic structure. Hy Trac's research group has applied novel machine learning approaches such as Bayesian Deep Learning, Convolution Neural Networks, and Support Distribution Machines to infer the masses of galaxy clusters (Ntampaka et al. 2015, Ntampaka et al. 2016, Ho et al. 2019, Ho et al. 2020). In upcoming work, they propose to use AI image recognition capabilities to learn the complex patterns between mass and light in high-resolution simulations, calibrated mock observations, and astronomical images taken at different wavelengths (e.g. x-ray, optical, millimeter, radio). Their primary science goal is to develop and apply modern AI approaches for cartographic discovery of dark matter from visible light. Knowing where dark matter is will help us to understand its nature and that of the Universe.
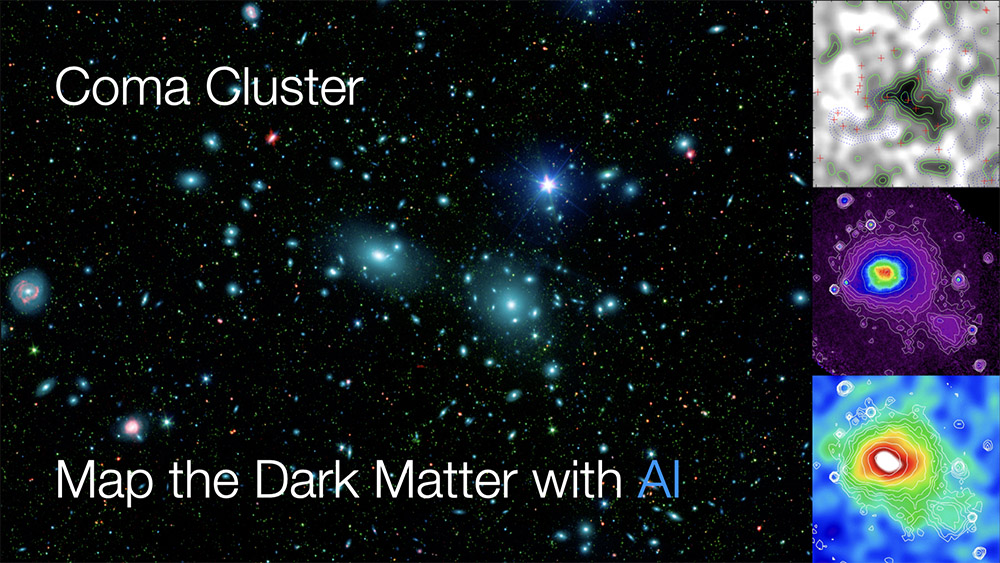