Interpretable Dynamic Programming Policies Informed by Managerial Insights with Application to Sequential Marketing Decision Making
Marketing managers need to dynamically decide upon a series of messages presented to consumers across many channels with the purpose of maximizing an objective like the probability a new account is opened. Dynamic programming offers a solution to this problem from which managers can derive recommendations about how today’s marketing actions effect future consumer choices. Estimation methods using non-parametric methods like Gaussian Processes can be used to estimate and interpolate the policy function. The problem is that these methods lack interpretability, which are important for managers to use these methods. Furthermore current methods ignore the insights that managers offer in terms of what the current policy is or what the policy function should be. To address these deficiencies our goal is to derive interpretable approximations of the policy function that can be communicated with managers, and in turn allow managers to form priors over these approximations that can inform on the posterior of the policy function. Our conjecture is that in noisy environments like our marketing context the prior information from managers is critical to improve the precision of the estimate of the policy function. It also offers a context for managers to understand and interpret the findings.
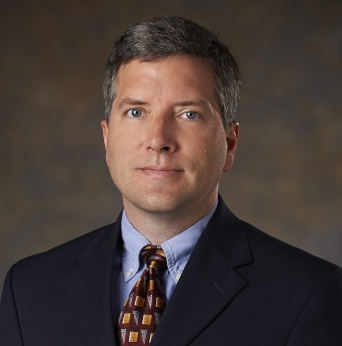