Predicting Financial Transactions in Context
Consumer behavior is without doubt influenced by many factors, but the exact nature and importance of these factors is often not clear. It could be that some users respond to broadcast news about a security threat by proactively working with their accounts (changing their weak password, for example). Others, on the other hand, might only react to a story about hacking that they come across in one of their social networks.
Some users might require information from different sources, some might react only to information that has been repeated over a certain period, and so on. None of these actions could be predicted well from previous account activity alone, because such internal data does only contain partial information, which is in reaction to external stimuli. Transaction and activity data, with sparse demographics and relationship markers has not been correlated with external data of different and heterogeneous types, such as national and local news, (social) media aggregates, or other relevant information streams.
While external data has been used to predict trends of customer behavior in general, and for groups of customers, so far it has not been possible to use it for improving predictions at the individual level, e.g. what is the likelihood that this specific customer will take a particular action, unless using customer segments and trends as proxies for individual behavior. We propose to create neural network sequence models that take both individual (e.g. historical account activity) and general data (national news) as input, and predict individual actions (e.g. future account activity), without requiring a hard assignment of customers into segments.
Our models will learn a latent clustering of customers, which can be used to understand and sanity check the model, but which will not be used directly in the decision process. Our predictions will be useful on their own merit, but could also become a factor in the context of fraud monitoring, risk modeling, or marketing activity. While not in scope of the current proposal, the original inspiration for this work is reports on the ability to predict economic activity by evaluating the degree to which parking lots are filled, and the ease with which such data can be acquired and processed with neural methods inspired by recent advances in speech processing using neural networks.
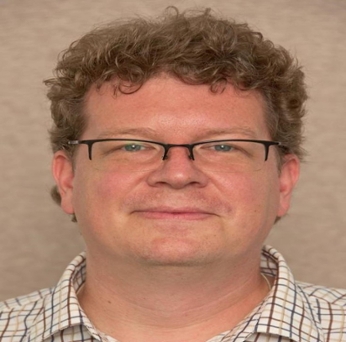