Safe control: Long-term safety for stochastic systems
With the presence of stochastic uncertainties, a myopic controller that ensures safe probability in infinitesimal time intervals may allow the accumulation of unsafe probability over time and result in a small long-term safe probability. Meanwhile, increasing the outlook time horizon may lead to significant computation burdens and delayed reactions, which also compromises safety. To tackle this challenge, we define a new notion of forward invariance on ‘probability space’ as opposed to the safe regions on state space. This new notion allows the long-term safe probability to be framed into a forward invariance condition, which can be efficiently evaluated. We build upon this safety condition to propose a controller that works myopically yet can guarantee long-term safe probability or fast recovery probability. The proposed controller ensures the safe probability does not decrease over time and allows the designers to directly specify safe probability.
We also developed a long-term probabilistic safety certificate for multi-agent systems with stochastic uncertainties and information sharing constraints. This method works with safety and performance specifications specified by non-differentiable barrier functions in a distributed manner that intelligently coordinate multi-agent systems without centralized computing.
Safe Control: Rethinking Safe Control in the Presence of Self-Seeking Humans
Safe control methods are often designed to behave safely even in worst-case human uncertainties. Such design can cause more aggressive human behaviors that exploit its conservatism and result in greater risk for everyone. However, this issue has not been systematically investigated previously. We used an interaction-based payoff structure from evolutionary game theory to model how prior human-machine interaction experience causes behavioral and strategic changes in humans in the long term. The results demonstrate that deterministic worst-case safe control techniques and equilibrium-based stochastic methods can have worse safety and performance trade-offs than a basic method that mediates human strategic changes. This finding suggests an urgent need to fundamentally rethink the safe control framework used in human-technology interaction in pursuit of greater safety for all.
Risk estimation: Physics-informed learning for long-term risk estimation
Accurate estimates of long-term risk probabilities and their gradients are critical for many stochastic safe control methods. However, computing such risk probabilities in real-time and in unseen or changing environments is challenging. Monte Carlo (MC) methods cannot accurately evaluate the probabilitiesand their gradients as an infinitesimal devisor can amplify the sampling noise. We propose an efficient method to evaluate the probabilities of long-term risk and their gradients. The proposed method exploits the fact that long-term risk probability satisfies certain partial differential equations (PDEs), which characterize the neighboring relations between the probabilities, to integrate MC methods and physics-informed neural networks. Numerical results show the proposed method has better sample efficiency, generalizes well to unseen regions, and can adapt to systems with changing parameters. The proposed method can also accurately estimate the gradients of risk probabilities, which enables first- and second-order techniques on risk probabilities to be used for learning and control. We are interested in theoretical analysis and extension to end-to-end learning-based safe control with the proposed risk estimation framework.
Applications: Autonomous Driving with Uncertainties and Occlusions
We derive a sufficient condition to ensure long-term safe probability when there are uncertainties in system parameters. We test the proposed technique numerically in a few driving scenarios. The resulting control action systematically mediates behaviors based on uncertainties and can find safer actions even with large uncertainties.
We propose a probability-based predictive controller that is able to guarantee long-term safety under occlusions without being over-conservative. We verify our method with numerical and onboard experiments on a visual occluded pedestrian crossing scenario. We show that proposed controller can handle latent risks caused by on-road interactions in real-time, and is easy to design with transparency to the exposed risks.
Applications: A Learning and Control Perspective for Microfinance
We propose a novel control-theoretical microfinance model and propose an algorithm for learning loan approval policies.
Our methods can make robust decisions before enough loans are given to accurately estimate the default probability and credit scores by directly learning the optimal policy parameters. Our model accounts for missing information and group liability, and policy learning processes converge to optimal policy parameters in the presence of both. In addition, our algorithm can systematically optimize competing objectives such as risks, socio-economic impacts, and active and passive fairness among different groups.
Robust control: Achieving robustness in large-scale complex networks
A major challenge in the design of autonomous systems is to achieve robustness and efficiency despite using interconnected components with limited sensing, actuation, communication, and computation capabilities. To tackle this challenge, we develop the fundamental theory in learning and control for autonomous systems. Here, autonomous systems are broadly defined (human sensorimotor system, autonomous driving systems, see below for more examples). Below, we list some of the ongoing projects.
From optimality to robustness: Most non-asymptotic performance guarantees for reinforcement learning in linear dynamics are centered around optimality. However, optimality does not imply robustness (e.g. having stability margin), which is critical in achieving stable and safe performance when implemented physically. In this project, we aim to provide better robustness to the learning and control methods by borrowing tools from robust control.
Effective architectures for integrating model-driven and data-driven control: Most existing methods either take a model-driven approach for systems with clear models or a data-driven approach for systems whose dynamics are hard to model. In contrast, human achieves remarkable robustness to varying dynamics/environment/tasks by using layered control architectures that exploit the knowledge on partial dynamics and the flexibility of model-free learning. Drawing insights from sensorimotor control, we study the effective layered architectures that integrate data-driven learning and model-driven control to achieve better robustness.
Neuroscience and biology: Integrating neurophysiology and sensorimotor
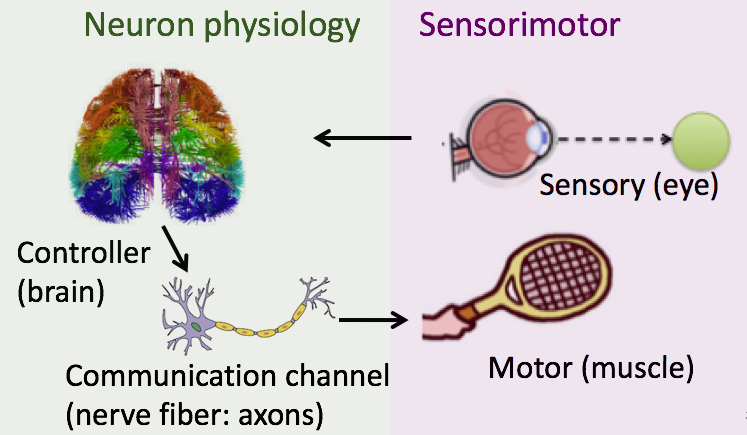
Neuroscience provides rich insights into the effective layered architectures that achieve remarkably robust control despite components that may be slow, inaccurate, distributed, or noisy. While enormous progress is being made in understanding both the components and overall system behavior, less progress has been made in connecting these due to the lack of theoretical foundations that capture the component level (neurophysiological) tradeoffs and the system level (sensorimotor control, which uses the component in control) from a holistic perspective. We develop the theoretical foundation to bridge this gap.
Through this bridge, we aim to interpret the existing insights on the component and system from a holistic perspective. In particular, we are excited that this perspective helps us understand what effective layered architectures allow for robust (fast and accurate) performance to be achieved even with slow or inaccurate components.
Our prior work made an initial step toward this objective by characterizing the tradeoffs between information rate and transmission delay in peripheral nerve fibers (hardware speed-accuracy-tradeoffs) and the resulting system speed-accuracy-tradeoffs in sensorimotor tasks such as visual tracking, driving, and reaching. The system SATs can be improved by having a broad distribution of delay and rate across the population of incoming sensory fibers within levels by (i.e. having a broad distribution within a single modality or single nerve bundle) or between layers (having a multimodal distribution across different modalities, such as a combination of visual and vestibular input for oculomotor stabilization). We term this concept as "diversity enabled sweet spot (DESS).'' Interestingly, DESS can be observed in a variety of systems, both natural and technological: the size principle for the recruitment of motor units, Fitts' Law in reaching, sensorimotor learning, immune response, transportation, smart grid.
Autonomous driving: attaining safety using affordable hardware
Autonomous driving can fundamentally change our life and transportation. However, there are tremendous challenges in ensuring safety and making autonomous vehicles more affordable and modular. We aim to enhance its safety and affordability using robust learning and control methods and bio-inspired architectures.
Past projects:
Smart grid: Enhancing scalability and efficiency in real-time scheduling
Large-scale service systems, such as power stations and cloud computing, present tremendous challenges in terms of controlling their strain on the power grid and the cloud. Meanwhile, these systems also present a great opportunity to exploit the flexibility in service speed and duration of each job. In view of these challenges and opportunities, we developed low-complexity online scheduling algorithms that balance system performance and quality-of-service. Our algorithms, when implemented in electric vehicle charging stations, can help achieve stable and predictable power consumption.
Security: Improving fault tolerance in estimation and inference
A cyber-physical system is vulnerable to various faults due to its distributed nature. Examples of these faults are hijacking, natural disasters, infrastructure wear, which can be caused by either malicious agents or unforeseen accidents. Therefore, the control of such systems should be equipped with safety-critical processes that tolerate a wide range of faults. We proposed both a state estimator and inference algorithms for system parameters, which have provable performance guarantees in the presence of sparse faults. Our algorithms, when incorporated into the control of cyber-physical systems, can help mitigate human injuries or economic damage to these faults.