Woolley and Team Explore AI’s Potential for Enhancing Teams’ Collective Intelligence
By Dr. Emily Barrow DeJeu and Dr. Anita Williams Woolley
CI-Lab@CMU researchers explore AI-enhanced frameworks for boosting teams’ collective intelligence in ways that make remote, distributed collaborations smoother and more efficient.
The Problem: 21st-century professionals increasingly work on multiple remote, distributed teams, which makes managing collaboration difficult
Advances in collaborative technologies, such as file sharing and video conferencing applications, have made it easier for people to work together remotely. At the same time, the internet has created a profusion of information and a corresponding need for deeper specialization in many fields. As a result of these trends, today’s professionals often work on multiple large, geographically dispersed teams, which creates a host of new challenges.
One major reason that many professionals quickly burn out is related to multitasking. This kind of collaboration involves scheduling myriad meetings and trying to drive a project forward when team members are not easy to access and can prove exhausting. But even beyond logistical challenges, developing the ability to collaborate on a wide range of problems – which Dr. Woolley and her collaborators call collective intelligence – is very difficult when a team is large and its members all have different areas of expertise.
The Solution: AI can enhance established frameworks for boosting a team’s collective intelligence
Recognizing how challenging it is for 21st century teams to build collective intelligence, Woolley and her team developed a framework describing the components of collective intelligence, called TMS-CI, with an eye toward helping teams better coordinate their work. The TMS-CI framework articulates how three different socio-cognitive systems must come together for any team to work effectively:
- the transactive memory system, which helps team members keep track of each other’s expertise and skill sets
- the transactive attention system, which helps team members coordinate their task allocations efficiently and keep track of who is attending to what
- the transactive reasoning system, which helps teams develop and maintain alignment on shared goals and mutually beneficial outcomes, which maximizes member effort and commitment
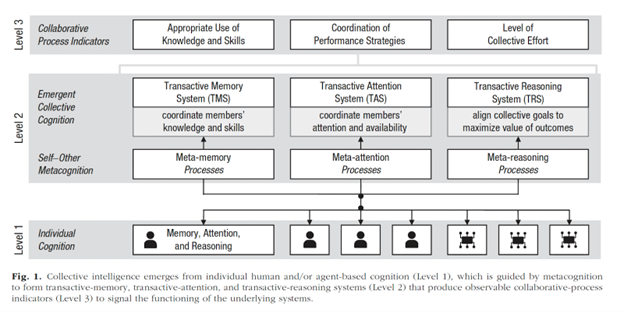
While Woolley’s framework predates the rise of generative AI, she and her collaborators are exploring the role that AI can play in supporting and enhancing each factor in the TMS-CI framework. They see exciting potential: for instance, they envision collaborators using AI tools to build a repository of shared knowledge they can regularly consult and that would grow with the team. Or consider the ways that AI tools could coordinate and regularly update popular scheduling applications, reducing the time and effort required to organize team meetings. AI tools could also facilitate team discussions about shared goals and priorities and assist with the inevitable negotiation that such prioritization requires.
There are limitations to integrating AI into a team’s collaborative processes, of course. Generative AI tools are not always trustworthy sources of information, and there’s a risk that team members might start to overly rely on AI tools, to the point that it jeopardizes the interpersonal communication and true collaborative effort that is necessary for building a thriving team. Even so, Woolley and her team are optimistic that recent advances in AI hold promise for further enhancing remote collaboration.
Case Study: An interdisciplinary CMU research team explores AI solutions for caregiving networks
In fact, she and her team have recently begun exploring specific AI applications: they recently embarked on a project to investigate the role that AI could play in caring for elderly adults by improving coordination between members of that adult’s care network. A caregiving network contributes substantially to helping elderly adults at home, but coordinating their joint efforts is vital: In addition to managing things like medication and meals, members of the caregiving network must be able to gather and share other kinds of information about the elderly adult’s mood, possible new symptoms, daily activities, etc.
Woolley and her team are testing the extent to which a generative AI agent, in the form of an app, can help distributed networks of caregivers coordinate asynchronously and keep track of these details, thereby ensuring vital information does not slip through the cracks.
Why It Matters: Generative AI tools are widely available, and integrating them into a collaboration could be relatively easy
While professionals are naturally limited by AI-related constraints within their organizations, experimenting with AI tools to enhance collaboration is relatively straightforward, thanks to their widespread availability and ease of use. For instance, if a team needs to plan an important presentation, instead of trying to coordinate multiple planning meetings, which can be time-consuming and frustrating, they could use AI tools to build and iteratively revise a repository of information for the presentation, which could, in turn, reduce the number of synchronous meetings they need to plan. Experimenting with AI interventions like these offers teams an accessible way to enhance their collective intelligence and streamline their collaborative processes.
For more on Dr. Woolley’s research on AI and collective intelligence, visit her Scholars@CMU page.