CMU Energy Research Exhibition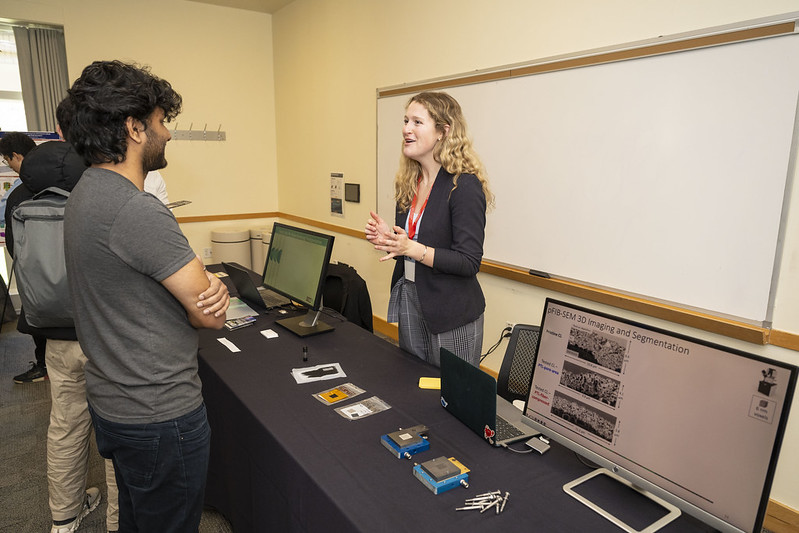
Across the hall from the main stage is the CMU Research Exhibition, highlighting our most exciting energy research and innovations in a more intimate format. Attendees will have the opportunity to interact with CMU researchers and view their findings and/or innovative technologies.
When: March 26, 2025, from 12:00-1:00 p.m. (during lunch)
Where: McKenna Peter Wright (across the hall from Rangos, the Energy Week main stage)
Research Exhibition Booths
AI-Driven Control for Energy-Efficient and Grid-Responsive Buildings | Using physics-informed reinforcement learning, we manage building energy use by optimizing renewable energy utilization while minimizing occupant discomfort. |
ML&AI Solutions for Uncertainty-Aware Grid Resilience and Sustainable Energy Planning |
Our research enhances grid resilience and energy planning in the face of growing uncertainties. From neural differential equations for outage prediction and intervention planning, to hierarchical uncertainty quantification for distributed energy growth, and generative decision-focused learning for resilient power operations, our work bridges predictive insights with strategic action for a more reliable and adaptive energy system. |
AI Calculations of Energy Materials |
Project 1: Become a perovskite solar cell designer for a day! Try out different materials and instantly see how the materials properties affect solar cell efficiency, calculated through the use of ML models. Project 2: Many foundation models for materials design have been created, but how good are they really? Find out how your favorite AI model compares and see if you can create a new battery material. Project 3: What if we can generate energy from waste heat? See how we can use AI to optimize the design of thermoelectric materials." |
Graph Neural Networks for Catalyst Discovery with the Open Catalyst Project |
Graph Neural Networks help us predict properties of materials, allowing us to find suitable catalysts for diverse energy applications. |
Smart AI: Making Data Collection and Processing More Efficient |
We use RL-driven strategies to learn to make smarter decisions about where to process data and how devices communicate, helping to save energy and improve performance. |
Examining the Role of Ride Sourcing Services During Rain: A Chicago Case Study |
We examine the role of ride-hailing during rain in the city of Chicago using non-parametric regression methods in a causal inference framework. |
Investigating Green Hydrogen Generating Processes Using Detection Films and Machine Vision |
We've developed a high-throughput photoreactor utilizing colorimetric detection films to study green hydrogen generation processes, with its data being used in machine learning models to predict potential hydrogen evolution catalysts. |
Understanding LLM Inference Costs Across Workloads and Settings |
We'll share insights into the energy considerations of large language model inference and energy optimizations. |